Bioinformatics analysis of the key genes in osteosarcoma metastasis and immune invasion
Introduction
Osteosarcoma is the most common and aggressive type of malignancy in bone tissue, and has a global incidence of approximately 3 cases per million. Osteosarcoma accounts for <0.2% of all malignant tumors and occurs mainly in children and adolescents, with a 2nd peak incidence above the age of 50 years (1-3). Surgical resection is the main treatment for osteosarcoma, but the survival rate of patients with osteosarcoma treated with surgery alone is only 15–17% (4).
The advent and application of neoadjuvant chemotherapy has led to more options and breakthroughs in the treatment of osteosarcoma; however, the overall survival (OS) rate of patients has not changed significantly (5). Approximately 20% of patients have combined metastatic lesions, especially pulmonary metastases, at the time of 1st diagnosis, and the 5-year survival rate of patients with osteosarcoma still has not improved significantly (6,7). Thus, the identification of potential biomarkers that can be used to assess the metastasis and prognosis of osteosarcoma has important clinical applications.
As early as 1891, surgeons adopted immunotherapy for patients with osteosarcoma, but its success remains controversial (8,9). A study of adjuvant immunotherapy in patients with resectable osteosarcoma treated with Bacillus Calmette Guerin vaccine and allogeneic tumor cells found that 18% of patients who received immunotherapy were cured or survived, while all patients who did not receive immunotherapy had recurrence (10). In pediatric patients with osteosarcoma, lymphocyte counts after chemotherapy were found to be positively associated with a good prognostic recovery (11). These therapies use both the innate and adaptive immune systems to restrain the ability of transformed cells to grow, to activate immune effector cells, and to induce immune antitumor activity. Thus, for the treatment of metastatic and recurrent osteosarcoma, immunotherapy may have great potential.
Osteosarcoma is a common and highly malignant tumor in the adolescent population, and the current status of biomarkers that effectively diagnose osteosarcoma and predict its prognosis, such as the occurrence of metastasis and susceptibility to drug therapy is unclear, which has limited further improvements in the survival of osteosarcoma patients. The bone microenvironment is a unique part of the immune system in which the intercellular crosstalk of immune cytokines is closely related to bone development and bone dynamic homeostasis. Transcriptome sequencing can address deep discovery of new genes, discovery of low-abundance transcripts, transcript mapping, regulation of variable splicing, metabolic pathway identification, gene family identification, and evolutionary analysis at the RNA level. Our study aims to identify biomarkers of osteosarcoma metastasis, construct relevant predictive models, and also predict potential therapeutic agents based on the key targets mined for subsequent osteosarcoma research. We present the following article in accordance with the TRIPOD reporting checklist (available at https://tp.amegroups.com/article/view/10.21037/tp-22-402/rc).
Methods
Data collection
We downloaded high-throughput transcriptomic data from the database of Therapeutically Applicable Research to Generate Effective Treatment (TARGET) program (https://ocg.cancer.gov/programs/target). A total of 87 patients containing transcriptomic and clinical data were collated, and a total of 84 patients were included in the follow-up survival analysis due to the absence of prognostic information in 3 cases. The Gene Expression Omnibus (GEO, http://www.ncbi.nlm.nih.gov/geo/) is a public functional genomics database. We searched the GEO database using osteosarcoma as a keyword, and datasets with sample size greater than >50 and containing clinical data were included, and finally the GSE21257 dataset was included. The study was conducted in accordance with the Declaration of Helsinki (as revised in 2013).
Differential expression analysis
After extracting the data sets for both GSE21257 and TARGET, the data sets were normalized using the limma package in R language. The limma package was used to analyze the differentially expressed genes (DEGs). The data sets were divided into the following 2 groups: (I) osteosarcoma without metastasis; and (II) osteosarcoma with metastasis. The threshold of patient significance was set as follows: variance multiples >0.5 and a P value <0.5. The results were visualized using the pheatmap package to construct heat maps for the top 100 DEGs and the ggplot2 package was used to construct volcano maps to visualize the distribution of the DEGs.
Identifying the core genes
Using the VennDiagram package in R language, DEGs from the GSE21257 and TARGET datasets were entered to obtain core genes for osteosarcoma metastasis and the results were visualized using veen plots. As a result, a total of 19 core genes for osteosarcoma metastasis were identified.
Protein-protein interaction (PPI) and gene-gene interaction (GGI)
The core genes of osteosarcoma metastasis were entered into the Search Tool for the Retrieval of Interacting Genes (STRING) database (https://string-db.org/) to construct a functional PPI network. Interactions with a composite score >0.4 were considered statistically significant, and the results were subsequently imported into Cytoscape (version 3.9.0) to visualize the results. The core genes for osteosarcoma metastasis were then imported into the online tool, GeneMinia (http://genemania.org/), to construct the GGI network.
Survival analysis
We used the TARGET database for the prognostic analysis of the core genes of osteosarcoma metastasis and conducted a log-rank test. The analysis was performed using the “survival” package and the “survminer” package of R language. Survival maps were constructed (a total of 12 prognosis-related core genes for osteosarcoma metastasis were identified).
Gene Ontology (GO) and Kyoto Encyclopedia of Genes and Genomes (KEGG) analyses
To determine the functions of the core genes of prognosis-associated osteosarcoma metastasis, we performed a GO annotation analysis and a KEGG pathway enrichment analysis using the R package clusterProfiler. The analysis covered the following 3 domains: biological process (BP), cellular component (CC), and molecular function (MF). A q value <0.05 indicated a statistically significant difference.
Micro-RNA (miRNA) target gene network prediction
In disease states, miRNAs affect gene expression through post-transcriptional control. This study used the miRWalk database (http://miRWalk.umm.uni-heidelberg.de/) to search for the miRNAs associated with genes central to prognosis-related osteosarcoma metastasis. miRWalk is a publicly available database of miRNA target genes, and includes data of predicted and experimentally validated miRNA-target interaction pairs from different species, including humans, mice, rats, dogs and bovine. We imported the core genes for prognosis-related osteosarcoma metastasis into the miRWalk database, set a score of 1, validated with miRDB (http://mirdb.org/) as a filter condition, and imported the results into Cytoscape (version 3.9.0) to construct a core gene regulatory network structure for prognosis-related osteosarcoma metastasis.
Construction of receiver operating characteristic (ROC) curves and metastasis diagnostic models
The ROC curves for the core genes of prognosis-related osteosarcoma metastasis were constructed using the “rms” package in R language to assess the diagnostic efficacy of the genes in both data sets. An area under the curve (AUC) of 0.6–0.7 indicated low efficacy, an AUC of 0.7–0.8 indicated moderate efficacy, and an AUC of >0.8 indicated high efficacy. Subsequently, the diagnostic model for diagnosing osteosarcoma metastasis was constructed using the survminer package and the RMS package, and the diagnostic efficacy of the model was assessed by the concordance index (C-index), and the accuracy of the model was assessed by the correction curve.
Construction of a prognostic model of metastasis
The prognosis-related core genes of osteosarcoma metastasis were obtained by a multivariate Cox proportional hazards regression analysis, which was implemented by the “surv” and “survminer” packages of R language. To construct the final risk model, 4 genes were identified. The risk score of each patient was then calculated based on the regression coefficients of the signature genes and the corresponding expression values. The risk scores were calculated as follows:
Risk score = expression of gene1 × β1 + expression of gene2 × β2 + … expression of Genen × βn (risk score = NPC2 × 0.28321 + CTSO × 0.38456 + APBB1IP × 0.559 + IGF2 × 0.26218).
Patients were divided into high- and low-risk groups based on the median risk scores. A Kaplan-Meier OS analysis was conducted followed by a log-rank test. The RMS package in R was used to construct the subject ROC curves and calculate the AUCs. The analyses were performed using the R package. A P value <0.05 was considered statistically significant.
Immune infiltration
We used the CIBERSORT online website (http://CIBERSORT.stanford.edu/) to predict the proportion of 22 immune cells in the data set of all samples. CIBERSORT was used to determine the proportion of immune cells by deconvolution and was experimentally validated. We set 100 replicates, and samples with P values <0.05 were considered statistically significant. We visualized the results through the ggplot2 package in R. The bar plot and heatmap reflect the relative content of the 22 immune cells in each sample. The correlation heatmap analyzed and visualized the correlation between immune cells. The violin plot shows the difference in the content of immune cells between the high- and low-risk groups.
Immuno-correlation analysis
The outcome matrix of immune infiltration was combined with the data set normalized to 12 core genes of prognosis-related osteosarcoma metastasis for the correlation analysis, and the results were visualized and analyzed using the ggcorrplot package in R language to construct bar plots to assess the correlations between the immune-related genes and immune cells. Correlation coefficients from 0.4 to 0.7 indicated moderate correlations and correlation coefficients >0.7 indicated high correlations.
Drug sensitivity analysis
Using the GeneCard database (https://www.genecards.org/), we searched for osteosarcoma therapeutic target genes using “osteosarcoma” as a keyword, and then intersected with 12 prognosis-related osteosarcoma metastasis core genes to obtain immune-related osteosarcoma therapeutic key genes. Finally, the Drug-Gene Interaction Database (DGIdb, https://www.dgidb.org/) was used to predict potential drugs targeting the intersecting genes, and Cytoscape was used to visualize the network modules.
Statistical analysis
All bioinformatics analyses in this study were performed using R software. The pheatmap package was applied to construct the expression heat map of important genes in osteosarcoma and metastatic osteosarcoma. Statistical tests were performed using the R language limma package to compare the differences in expression of important genes in osteosarcoma and metastatic osteosarcoma. the AUC values in the ROC curves were used to assess diagnostic efficacy and P<0.05 was considered a statistically significant difference.
Results
Osteosarcoma metastasis DEGs
The results showed that 176 genes were significantly upregulated and 112 genes were significantly downregulated in the TARGET data set. Conversely, 430 genes were upregulated and 715 genes were significantly downregulated in the GSE21257 data set (Figure 1).
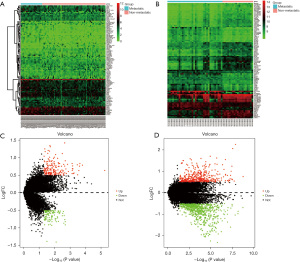
PPI and GGI network construction
The subsequent analyses of the co-expressed DEGs in the GSE21257 and TARGET data sets showed that 19 genes were significantly altered in both data sets (Figure 2A). PPI and GGI analyses of the 19 core genes were performed using the STRING database and the GeneMinia tool. The PPI network comprised 11 key proteins, such as lymphocyte cytosolic protein 2 (LCP2), MYC, and SELL, while in the GGI analysis, 37 interacting genes were enriched (Figure 2B,2C).
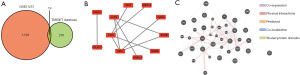
Survival analysis
We performed a survival analysis of the 19 core genes and found that patients with a low expression of APBB1IP, CCAAT/enhancer binding protein (CEBPA), CFI, CTSO, ecotropic viral integration site 2B (EVI2B), granzyme A (granzyme 1, cytotoxic T-lymphocyte-associated serine esterase 3) (GZMA), IGF2, LCP2, NPC2, and SELL had a better prognosis, while patients with MYC and prolyl 4-hydroxylase, alpha polypeptide I (P4HA1) expression had a better prognosis in Osteosarcoma, but the expression of calcium/calmodulin-dependent protein kinase I (CAMK1), collectin sub-family member 11 (COLEC11), growth arrest and DNA-damage-inducible, gamma interacting protein 1 (GADD45GIP1), glypican 1 (GPC1), heat shock 70 kDa protein 1A (HSPA1A), transgelin 2 (TAGLN2), and ubiquitin associated protein 1 (UAP1) did not affect the prognosis of patients (Figure 3).
GO and KEGG enrichment analysis of core DEGs
Using R software, biological function and pathway enrichment analyses were performed based on the core DEGs metastasized from osteosarcoma. The top 10 BPs, CCs, MFs and 10 KEGG pathways were selected according to the number and significance of gene enrichment, and bar and bubble plots were drawn (Figure 4A,4B). The main BPs included the regulation of cell division, secretory granule lumen, endopeptidase activity, immunological synapse, platelet activation, transcriptional misregulation in cancer, the Rap1 signaling pathway, and the MAPK signaling pathway (Figure 4A,4B).
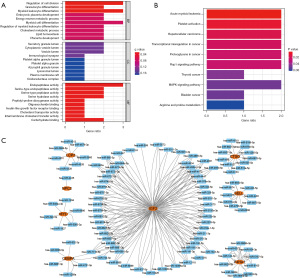
MiRNA target gene network prediction
To further explore the potential miRNAs of the core DEGs in osteosarcoma metastasis, we constructed a miRNA network comprising the following 8 key genes: LCP2, NPC2, MYC, CEBPA, IGF2, CTSO, SELL, and P4HA1 (Figure 4C).
Construction of ROC curves and prediction models for metastasis
To build a prediction model for metastasis, we first performed a diagnostic efficacy analysis of the core DEGs, and finally used 5 genes (i.e., EVI2B, CEBPA, LCP2, SELL and NPC2A) to construct models for the 2 data sets, and the results showed that the models all had good predictive efficacy (Figure 5).
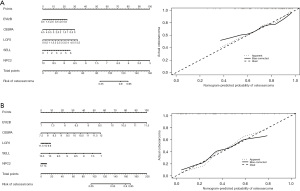
Construction of a prognostic model of metastasis
The prognosis-related core genes of osteosarcoma metastasis were identified by a multivariate Cox proportional hazards regression analysis, and a total of 4 genes (i.e., IGF2, CTSO, NPC2, and APBB1IP) were used to construct the final risk model. The risk scores of each patient were then calculated based on the regression coefficients of the signature genes and the corresponding expression values. Based on the median risk, the patients were divided into high- and low-risk groups, and the distribution of risk assessment, survival time, and status of patients were observed. The survival analysis showed that patients in the high-risk group had a worse prognosis than those in the low-risk group (P=0.00015). The ROC analysis showed that the AUCs were 0.790, 0.764, and 0.649 for 1-, 3-, and 5-year OS, respectively (Figure 6).
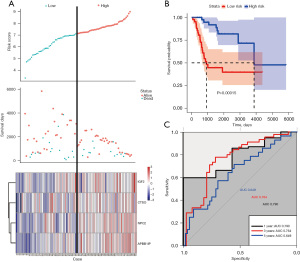
Immune cell infiltration analysis
To further explore the role of immune cells in osteosarcoma metastasis, we analyzed 22 immune cells from all the samples in TARGET and GSE21257 data sets. Macrophage infiltration was predominant in osteosarcoma samples compared to other immune cells (Figure 7A), and M0 macrophages were negatively correlated with T cells cluster of differentiation (CD)8, M1 macrophages, and M2 macrophages (Figure 7B). M0 macrophage levels were significantly higher in the low-risk group, and mast cell activation differed significantly between the high- and low-risk groups (Figure 7C).
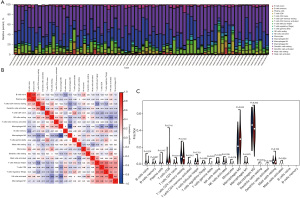
Immunological correlation analysis
The outcome matrix of immune infiltration was combined with the data set normalized to 12 core genes of prognostic relevance for osteosarcoma metastasis for the correlation analysis. The results showed that CEBPA and LCP2 were negatively correlated with M0 macrophages, and P4HA1 was positively correlated with M0 macrophages. LCP2 was positively correlated with M1 macrophages and M2 macrophages (Figure 8A).
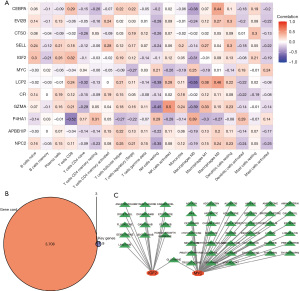
Drug sensitivity analysis
Using the GeneCard database (https://www.genecards.org/), the genes of the osteosarcoma therapeutic targets were searched using “osteosarcoma” as the keyword, and then intersected with the 12 prognosis-related core genes of osteosarcoma metastasis to identify further key genes for treatment. A drug sensitivity prediction analysis was then performed. The results showed that the IGF and MYC2 genes predicted >50 targeted therapeutic agents (Figure 8B,8C).
Discussion
Tumor metastasis is the spread of malignant tumor cells from the primary site, through blood vessels, lymphatic vessels, or body cavities, to other parts of the body for continued growth, and the occurrence of metastasis in malignant tumors is often the main cause of treatment failure and is a relevant cause of death in most patients (12,13). To achieve early diagnosis and treatment, the search for potential biomarkers is an essential task. In recent years, an increasing amount of microarray data from the GEO database has been used to analyze DEGs to reveal the mechanisms of development of various diseases. In this study, we examined the DEGs involved the development of metastasis in osteosarcoma based on the GSE21257 and TARGET data sets, and ultimately identified 5 key genes (i.e., EVI2B, CEBPA, LCP2, SELL, and NPC2A) by conducting biological function and PPI analyses.
EVI2B, also known as CD361, is a common viral integration site in retrovirus-induced leukemia in mice (14,15). Previous studies have shown that EVI2B is required for the granulocyte differentiation and function of hematopoietic progenitor cells and controls cell cycle progression and the survival of hematopoietic progenitor cells (16). EVI2B was found to predict the progression and prognosis of colorectal cancer (17). Related reports in osteosarcoma suggest that EVI2B is involved in the regulation of immune infiltrating cells and is positively correlated with multiple immune cells (18,19). CEBPA encodes a protein that regulates the expression of genes involved in cell-cycle regulation as well as body weight homeostasis, and mutations in CEBPA are associated with acute myeloid cell leukemia (20). Studies have shown that CEBPA expression is associated with the metabolism and prognosis of osteosarcoma and is a potential biomarker (21,22). LCP2 encodes an actin-binding protein that is involved in the regulation of various cellular signaling pathways. LCP2 protein activates T cells and promotes interferon gamma and interleukin-2 secretion, which is closely associated with metastasis and prognosis in patients with breast, gastric, and colon cancers (23-26). Ding et al. found that the expression of LCP2 was significantly reduced in the metastatic foci of osteosarcoma cells and was closely associated with patient prognosis (27). SELL and NPC2A have not been reported to play a role in osteosarcoma, which may be a direction for future research. In our study, osteosarcoma patients with high expression levels of EVI2B, CEBPA, LCP2, SELL, and NPC2A had a better prognosis in osteosarcoma. In this study, 19 DEGs were obtained by differential expression analyses of the GSE21257 and TARGET data sets. The GO functional annotation analysis and KEGG enrichment analysis showed that the DEGs were mainly enriched in substance metabolism, immunity, the MAPK signaling pathway, and the Rap1 signaling pathway. These are closely related to tumor development. The MAPK signaling pathway consists of MAP kinase-kinase-kinase (RAF1), extracellular signal-associated kinase (ERK), and MAP kinase-kinase (MEK), which transduce extracellular to nuclear signals through phosphorylation and are associated with tumor growth, proliferation, invasive migration, and vascular survival (28-31). Related studies have detected the activation of the MAPK signaling pathway in osteosarcoma lung metastasis and shown that it may be associated with cytokine secretion by macrophages (32,33). Additionally, it was found that the MAPK signaling pathway could be inhibited by knocking down calgranulin B (S100A9), thus inhibiting the growth of osteosarcoma cells (34). Studies have shown that the Rap1 signaling pathway is involved in tumor proliferation, invasion, and migration, regulating disease progression in osteosarcoma, and the expansion of tumor stem cells (35-37).
The tumor microenvironment (TME) of osteosarcoma is a complex environment composed of multiple components, including osteocytes, macrophages, mesenchymal stromal cells, and the extracellular matrix (38). Tumor cells and the TME interact with each other through various cytokines and chemokines (39), and an understanding of the immune microenvironment of osteosarcoma will help in the development of therapeutic regimens. Several studies have now confirmed that macrophages are a major component of the immune microenvironment of osteosarcoma. Further research has shown that M2 macrophages may inhibit osteosarcoma progression, and that all-trans retinoic acid inhibits pulmonary metastasis of osteosarcoma cells by suppressing M2-type macrophages (40-43). Additionally, it has been shown that M0 and M2 macrophages are significantly associated with the prognosis of patients with osteosarcoma (44). In our study, we also found that M0 macrophages were significantly elevated, LCP2 and CEBPA were positively correlated with the level of macrophage infiltration. Our findings are consistent with the above reports and suggest that these genes play an important role in disease progression in osteosarcoma, but the regulatory role of these cells and related genes needs to be further investigated.
The mainstay of treatment for osteosarcoma is surgery, but for patients with advanced stages and combined metastases, surgical resection combined with pharmacotherapy and radiation therapy are the main treatment options. Treatment for metastatic osteosarcoma is in its infancy, and tyrosine kinase inhibitors are used to treat osteosarcoma invasion and metastasis by inhibiting tumor angiogenesis (45). Metastatic osteosarcoma has a higher neoantigen load and immunogenicity than primary osteosarcoma, and programmed death receptor 1 expression is considered one of the most important biomarkers for checkpoint inhibitor therapy (33). Targeted and immunotherapy for osteosarcoma are promising therapeutic approaches, but on many occasions, the treatment results have not been satisfactory, which is probably due to the high cost of genome sequencing, a lack of knowledge about the mutated target genes, and reliance on clinical experience with drugs. We performed a drug sensitivity prediction analysis of the key genes involved in the pathogenesis of osteosarcoma and uncovered >50 potential therapeutic targets. Our findings should provide a basis for drug use in clinical treatment.
This study had a number of limitations. The data sources of this study were the GEO and TARGET databases; however, relatively few patients with osteosarcoma have been clinically sequenced for primary and metastatic foci, and there is a lack of key clinical information, which to some extent limits the analysis and interpretation of the data. Finally, due to the lack of corresponding clinical samples for verification and the improvement of basic experiments, this is the focus of our subsequent experimental research.
Conclusions
In summary, this study conducted a bioinformatic analysis to identify the DEGs and key genes associated with osteosarcoma metastasis, identified important immune infiltrating cells in osteosarcoma tissues (i.e., EVI2B, CEBPA, LCP2, SELL, and NPC2A) and constructed predictive risk models. Our results may provide new perspectives for the treatment of osteosarcoma.
Acknowledgments
Funding: None.
Footnote
Reporting Checklist: The authors have completed the TRIPOD reporting checklist. Available at https://tp.amegroups.com/article/view/10.21037/tp-22-402/rc
Conflicts of Interest: All authors have completed the ICMJE uniform disclosure form (available at https://tp.amegroups.com/article/view/10.21037/tp-22-402/coif). The authors have no conflicts of interest to declare.
Ethical Statement: The authors are accountable for all aspects of the work in ensuring that questions related to the accuracy or integrity of any part of the work are appropriately investigated and resolved. The study was conducted in accordance with the Declaration of Helsinki (as revised in 2013).
Open Access Statement: This is an Open Access article distributed in accordance with the Creative Commons Attribution-NonCommercial-NoDerivs 4.0 International License (CC BY-NC-ND 4.0), which permits the non-commercial replication and distribution of the article with the strict proviso that no changes or edits are made and the original work is properly cited (including links to both the formal publication through the relevant DOI and the license). See: https://creativecommons.org/licenses/by-nc-nd/4.0/.
References
- Mirabello L, Troisi RJ, Savage SA. Osteosarcoma incidence and survival rates from 1973 to 2004: data from the Surveillance, Epidemiology, and End Results Program. Cancer 2009;115:1531-43. [Crossref] [PubMed]
- Xie B, Li Y, Zhao R, et al. Identification of Key Genes and miRNAs in Osteosarcoma Patients with Chemoresistance by Bioinformatics Analysis. Biomed Res Int 2018;2018:4761064. [Crossref] [PubMed]
- Choi JH, Ro JY. The 2020 WHO Classification of Tumors of Bone: An Updated Review. Adv Anat Pathol 2021;28:119-38. [Crossref] [PubMed]
- Bernthal NM, Federman N, Eilber FR, et al. Long-term results (>25 years) of a randomized, prospective clinical trial evaluating chemotherapy in patients with high-grade, operable osteosarcoma. Cancer 2012;118:5888-93. [Crossref] [PubMed]
- Meyers PA, Healey JH, Chou AJ, et al. Addition of pamidronate to chemotherapy for the treatment of osteosarcoma. Cancer 2011;117:1736-44. [Crossref] [PubMed]
- Damron TA, Ward WG, Stewart A. Osteosarcoma, chondrosarcoma, and Ewing's sarcoma: National Cancer Data Base Report. Clin Orthop Relat Res 2007;40-7. [Crossref] [PubMed]
- Miller BJ, Cram P, Lynch CF, et al. Risk factors for metastatic disease at presentation with osteosarcoma: an analysis of the SEER database. J Bone Joint Surg Am 2013;95:e89. [Crossref] [PubMed]
- Coley WB. II. Contribution to the Knowledge of Sarcoma. Ann Surg 1891;14:199-220. [Crossref] [PubMed]
- Coley WB. The Treatment of Inoperable Sarcoma by Bacterial Toxins (the Mixed Toxins of the Streptococcus erysipelas and the Bacillus prodigiosus). Proc R Soc Med 1910;3:1-48. [Crossref] [PubMed]
- Eilber FR, Townsend C, Morton DL. Osteosarcoma. Results of treatment employing adjuvant immunotherapy. Clin Orthop Relat Res 1975;94-100. [Crossref] [PubMed]
- Moore C, Eslin D, Levy A, et al. Prognostic significance of early lymphocyte recovery in pediatric osteosarcoma. Pediatr Blood Cancer 2010;55:1096-102. [Crossref] [PubMed]
- Wu L, Hu B, Zhao B, et al. Circulating microRNA-422a is associated with lymphatic metastasis in lung cancer. Oncotarget 2017;8:42173-88. [Crossref] [PubMed]
- Xiong Y, Huang F, Li X, et al. CCL21/CCR7 interaction promotes cellular migration and invasion via modulation of the MEK/ERK1/2 signaling pathway and correlates with lymphatic metastatic spread and poor prognosis in urinary bladder cancer. Int J Oncol 2017;51:75-90. [Crossref] [PubMed]
- Buchberg AM, Bedigian HG, Jenkins NA, et al. Evi-2, a common integration site involved in murine myeloid leukemogenesis. Mol Cell Biol 1990;10:4658-66. [PubMed]
- Cawthon RM, Andersen LB, Buchberg AM, et al. cDNA sequence and genomic structure of EV12B, a gene lying within an intron of the neurofibromatosis type 1 gene. Genomics 1991;9:446-60. [Crossref] [PubMed]
- Zjablovskaja P, Kardosova M, Danek P, et al. EVI2B is a C/EBPα target gene required for granulocytic differentiation and functionality of hematopoietic progenitors. Cell Death Differ 2017;24:705-16. [Crossref] [PubMed]
- Yuan Y, Chen J, Wang J, et al. Identification Hub Genes in Colorectal Cancer by Integrating Weighted Gene Co-Expression Network Analysis and Clinical Validation in vivo and vitro. Front Oncol 2020;10:638. [Crossref] [PubMed]
- Matesanz-Isabel J, Sintes J, Llinàs L, et al. New B-cell CD molecules. Immunol Lett 2011;134:104-12. [Crossref] [PubMed]
- Yang B, Su Z, Chen G, et al. Identification of prognostic biomarkers associated with metastasis and immune infiltration in osteosarcoma. Oncol Lett 2021;21:180. [Crossref] [PubMed]
- Theilgaard-Mönch K, Pundhir S, Reckzeh K, et al. Transcription factor-driven coordination of cell cycle exit and lineage-specification in vivo during granulocytic differentiation: In memoriam Professor Niels Borregaard. Nat Commun 2022;13:3595. [Crossref] [PubMed]
- Wang J, Gong M, Xiong Z, et al. Bioinformatics integrated analysis to investigate candidate biomarkers and associated metabolites in osteosarcoma. J Orthop Surg Res 2021;16:432. [Crossref] [PubMed]
- Liu Z, Zhong Y, Meng S, et al. Identification of a seven-gene prognostic signature using the gene expression profile of osteosarcoma. Ann Transl Med 2022;10:53. [Crossref] [PubMed]
- Sommers CL, Menon RK, Grinberg A, et al. Knock-in mutation of the distal four tyrosines of linker for activation of T cells blocks murine T cell development. J Exp Med 2001;194:135-42. [Crossref] [PubMed]
- Maltzman JS, Kovoor L, Clements JL, et al. Conditional deletion reveals a cell-autonomous requirement of SLP-76 for thymocyte selection. J Exp Med 2005;202:893-900. [Crossref] [PubMed]
- Jiang H, Dong L, Gong F, et al. Inflammatory genes are novel prognostic biomarkers for colorectal cancer. Int J Mol Med 2018;42:368-80. [Crossref] [PubMed]
- Zhang J, Wang L, Xu X, et al. Transcriptome-Based Network Analysis Unveils Eight Immune-Related Genes as Molecular Signatures in the Immunomodulatory Subtype of Triple-Negative Breast Cancer. Front Oncol 2020;10:1787. [Crossref] [PubMed]
- Ding FP, Tian JY, Wu J, et al. Identification of key genes as predictive biomarkers for osteosarcoma metastasis using translational bioinformatics. Cancer Cell Int 2021;21:640. [Crossref] [PubMed]
- Chang L, Karin M. Mammalian MAP kinase signalling cascades. Nature 2001;410:37-40. [Crossref] [PubMed]
- Ding Y, Boguslawski EA, Berghuis BD, et al. Mitogen-activated protein kinase kinase signaling promotes growth and vascularization of fibrosarcoma. Mol Cancer Ther 2008;7:648-58. [Crossref] [PubMed]
- Tingting R, Wei G, Changliang P, et al. Arsenic trioxide inhibits osteosarcoma cell invasiveness via MAPK signaling pathway. Cancer Biol Ther 2010;10:251-7. [Crossref] [PubMed]
- Sasaki K, Hitora T, Nakamura O, et al. The role of MAPK pathway in bone and soft tissue tumors. Anticancer Res 2011;31:549-53. [PubMed]
- Wang C, Zhou X, Li W, et al. Macrophage migration inhibitory factor promotes osteosarcoma growth and lung metastasis through activating the RAS/MAPK pathway. Cancer Lett 2017;403:271-9. [Crossref] [PubMed]
- Wang D, Niu X, Wang Z, et al. Multiregion Sequencing Reveals the Genetic Heterogeneity and Evolutionary History of Osteosarcoma and Matched Pulmonary Metastases. Cancer Res 2019;79:7-20. [Crossref] [PubMed]
- Cheng S, Zhang X, Huang N, et al. Down-regulation of S100A9 inhibits osteosarcoma cell growth through inactivating MAPK and NF-κB signaling pathways. BMC Cancer 2016;16:253. [Crossref] [PubMed]
- Shi Z, Zhou H, Pan B, et al. Exploring the key genes and pathways of osteosarcoma with pulmonary metastasis using a gene expression microarray. Mol Med Rep 2017;16:7423-31. [Crossref] [PubMed]
- Wu J, Du W, Wang X, et al. Ras-related protein Rap2c promotes the migration and invasion of human osteosarcoma cells. Oncol Lett 2018;15:5352-8. [Crossref] [PubMed]
- Shah S, Brock EJ, Ji K, et al. Ras and Rap1: A tale of two GTPases. Semin Cancer Biol 2019;54:29-39. [Crossref] [PubMed]
- Corre I, Verrecchia F, Crenn V, et al. The Osteosarcoma Microenvironment: A Complex But Targetable Ecosystem. Cells 2020;9:976. [Crossref] [PubMed]
- Alfranca A, Martinez-Cruzado L, Tornin J, et al. Bone microenvironment signals in osteosarcoma development. Cell Mol Life Sci 2015;72:3097-113. [Crossref] [PubMed]
- Gomez-Brouchet A, Illac C, Gilhodes J, et al. CD163-positive tumor-associated macrophages and CD8-positive cytotoxic lymphocytes are powerful diagnostic markers for the therapeutic stratification of osteosarcoma patients: An immunohistochemical analysis of the biopsies fromthe French OS2006 phase 3 trial. Oncoimmunology 2017;6:e1331193. [Crossref] [PubMed]
- Li X, Chen Y, Liu X, et al. Tim3/Gal9 interactions between T cells and monocytes result in an immunosuppressive feedback loop that inhibits Th1 responses in osteosarcoma patients. Int Immunopharmacol 2017;44:153-9. [Crossref] [PubMed]
- Zhou Q, Xian M, Xiang S, et al. All-Trans Retinoic Acid Prevents Osteosarcoma Metastasis by Inhibiting M2 Polarization of Tumor-Associated Macrophages. Cancer Immunol Res 2017;5:547-59. [Crossref] [PubMed]
- Heymann MF, Lézot F, Heymann D. The contribution of immune infiltrates and the local microenvironment in the pathogenesis of osteosarcoma. Cell Immunol 2019;343:103711. [Crossref] [PubMed]
- Zhang C, Zheng JH, Lin ZH, et al. Profiles of immune cell infiltration and immune-related genes in the tumor microenvironment of osteosarcoma. Aging (Albany NY) 2020;12:3486-501. [Crossref] [PubMed]
- Shaikh AB, Li F, Li M, et al. Present Advances and Future Perspectives of Molecular Targeted Therapy for Osteosarcoma. Int J Mol Sci 2016;17:506. [Crossref] [PubMed]
(English Language Editor: L. Huleatt)