The potential mechanisms of neuroblastoma in children based on bioinformatics big data
Highlight box
Key findings
• The differential miRNAs may be related to the occurrence of neuroblastoma were screened.
What is known and what is new?
• In recent years, miRNAs have become a research hotspot, which is related to the occurrence and development of a variety of malignant tumors.
• The key genes screened and identified in this study are related to the occurrence, development, migration, and invasion of neuroblastoma cells.
What is the implication, and what should change now?
• Whether the key genes screened and identified in this study are related to the regulation of miRNA and whether miRNA can affect the occurrence and development of neuroblastomas through these genes warrant further investigation.
Introduction
Neuroblastoma (NB) is an embryonic malignant tumor originating from the primitive neural crest of the adrenal medulla and sympathetic ganglia. It is the most common extracranial solid tumor in children, accounting for 8–10% of childhood malignancies (1-3) and approximately 15% of childhood cancer-related deaths (4). At present, the true cause of NB is still unclear. Some genetic predisposing factors have been found to be related to the pathogenesis of NB. Nearly half of all patients with NB present with metastasis at the time of diagnosis, with poor prognosis. Primary abdominal neuroblastoma (ANB) tumors occur in the paraspinal ganglion or adrenal medulla, and metastatic dissemination occurs in the blood and lymphatic pathways (5-7). Its analogues play an important role in regulating many physiological processes of mammalian embryo development, cell differentiation, vision formation, reproduction, and immunity (8). The conversion are the only two reversible reactions in the retinol metabolic network, and this is the core link in the regulation of retinol metabolism (9). Retinol is stored as retinyl ester in cells. The levels of retinyl ester in cells is affected by the retinol substance inside and outside the cell and the physiological state of the cell. Recent studies have shown that the amount of retinoyl ester is decreased in bladder cancer, prostate cancer, kidney cancer, squamous cell carcinoma, and other tumor cells, and the degree of reduction is positively correlated with the degree of malignancy of tumor cells, indicating that the synthesis of retinoyl ester is closely related to the occurrence of tumors (10). Retinol dehydrogenase reductase in neuroblastoma cells can be induced by retinoic acid, which can increase the content of retinoyl ester in NB cells. It is thus an important regulator of retinol metabolism in neuroblastoma cells and is related to the occurrence of NBs (11-13).
NBs derived from the same migrating neural crest cells have a high potential for distant metastasis, and patients are often found to have bone marrow metastases at diagnosis. Surgical resection is still the most effective treatment for such patients, but for advanced cases and patients with distant metastasis, the prognosis is poor. Therefore, understanding the molecular mechanisms involved in the occurrence and development of NB bone marrow metastasis is crucial (14).
MicroRNA (miRNA) is a small non-coding RNA composed of about 20 nucleotides. It negatively regulates the mRNA expression of target genes at the post-transcriptional level. MiRNAs play an important role in embryonic development and tumorigenesis, and is closely related to patient prognosis (15). To date, the pathogenesis, differentiation, and metastatic mechanisms of NB have not been fully elucidated. Identification of the differentially expressed miRNAs in NB will contribute to the understanding, diagnosis, and treatment of the disease (16). In this current study, the miRNA expression in neuroblastoma samples and normal fetal adrenal neuroblastoma tissue samples were retrieved from the Gene Expression Omnibus (GEO) database and analyzed for differential expression. Target genes of the differentially expressed miRNAs with |log fold change (FC)| ≥4 were predicted and analyzed for functional enrichment. A protein interaction network was constructed to screen the core genes. The data presented herein will provide novel ideas for understanding the predictive value of miRNAs in NB. We present the following article in accordance with the TRIPOD reporting checklist (available at https://tp.amegroups.com/article/view/10.21037/tp-22-504/rc).
Methods
Data sources and methods
The GEO database (https://www.ncbi.nlm.nih.gov) is a genome database created by the National Center for Biotechnology Information (NCBI). It is the largest and most comprehensive public gene database in the world. The gene chip numbered GSE121513 was downloaded from the GEO database, including 117 samples in the dataset. The miRNA expression in 8 embryonic stem cell lines, 7 normal fetal adrenal NB samples, 7 normal fetal adrenal cortical samples, and 95 NB samples were included. In this study, 95 NB samples were selected as the experimental group and 7 normal fetal adrenal NB samples were selected as the control group. The study was conducted in accordance with the Declaration of Helsinki (as revised in 2013).
Data set arrangement and differential expression analysis
(I) Sample pretreatment: The GSE121513 matrix data file was downloaded from the GEO database, and the data of 95 NB samples (experimental group) and 7 normal fetal adrenal neuroblastoma samples (control group) were collected. Perl script was used to merge the two groups of data matrices, and the expression matrix file needed for this study was obtained. (II) Differentially expressed miRNA analysis: The preprocessed gene expression matrix file was extracted using the Read.table function in the R software package (V3.6.3), and the normalizeBetweenArrays function in the Limma software package was used to standardize the data. The IF cycle was used to determine whether the data needed log2 conversion, and if so, log2 conversion was carried out. The Model.matrix function in the LimmA program package was used to define groups, the lmFit function was used to establish linear models, and the eBayes function was used to conduct Bayes test for multiple test correction. Subset and other functions were used to analyze the differentially expressed miRNAs in neuroblastoma samples and normal fetal adrenal neuroblastoma samples. Differential miRNA screening criteria were as follows: P<0.05 and difference ratio change |logFC| ≥1. Volcano plots were constructed to visualize the differences in gene expression using R language ggplot2 package and heat maps were constructed using the R heatmap package.
Prediction of miRNA target genes
The target genes of the differentially expressed miRNAs were predicted using Targetscan, miRDB, and microRNA.org online tools (using |logFC| ≥4 as the criteria).
Gene Ontology and Kyoto Encyclopedia of Genes and Genomes enrichment analysis
The DAVID online analysis platform (https://david.ncifCRf.gov/) was used to perform GO biological function enrichment analysis and KEGG signaling pathway enrichment analysis on target genes. The target genes were annotated by GO function analysis according to biological process, cellular component, and molecular function. P<0.05 was considered statistically significant.
Construction of the protein interaction network and screening of the core target genes
The STRING data library (http://db.org/) was used to construct a protein-protein interaction (PPI) network of the differentially expressed miRNA target genes. The Cytoscape software and the Network Analyzer plug-in were used to screen out the key target genes with more nodes to construct the PPI core network.
Screening of hub genes and construction of the hub module
CytoHubba, a plug-in of Cytoscape, was used to identify the hub genes in the PPI network modules. The maximal clique centrality (MCC) in the PPI network was calculated and the top 10 genes were defined as hub genes. The Molecular Complex detection (MCODE) method in Cytoscape plug-in was used to analyze the whole network of genes in the PPI network module. The gene modules were extracted and the seed genes were obtained. A MCODE score >5 and number of nodes >10 were used as truncation criteria to screen the hub modules. The default parameters were used for MCODE analysis (degree cutoff =2, node score cutoff =0.2, K core =2, and maximum depth =100).
Statistical analysis
R software was used in the statistical analyses. The ROC model was constructed to analyse the predictive values. The differences of all categorical variables were analyzed by using χ2 analysis, while the differences of the continuous data were analyzed by using the t-tests. P<0.05 indicated that the difference was significant (two tail).
Results
Differentially expressed miRNAs
Neuroblastoma and normal fetal adrenal gland nerve cell tissue were compared to identify the differentially expressed miRNAs (Figures 1,2).
Gene Ontology and Kyoto Encyclopedia of Genes and Genomes analysis
GO functional enrichment analysis showed that differential miRNAs were mainly located in the extracellular matrix region of proteins, and were involved in biological processes such as cell response to amino acid stimulation, collagen catabolic process, negative regulation of cytoplasmic translation, and extracellular matrix organization. The differentially expressed miRNAs were also involved in binding to the 3’-untranslated region (UTR) of mRNAs, inhibiting translation factor activity by binding to nucleic acid, structural components of the extracellular matrix, metal ion binding, and zinc ion binding.
KEGG pathway analysis showed that the differential miRNAs may be related to RNA degradation, mucin-type O-glycan biosynthesis, adhesion pathway, phosphatidylinositol-3-kinase (PI3K)-Akt signaling pathway, dorsoventral axis formation, protein digestion and absorption, and extracellular matrix (ECM) receptor interaction mediated signaling pathway events (Figure 3).
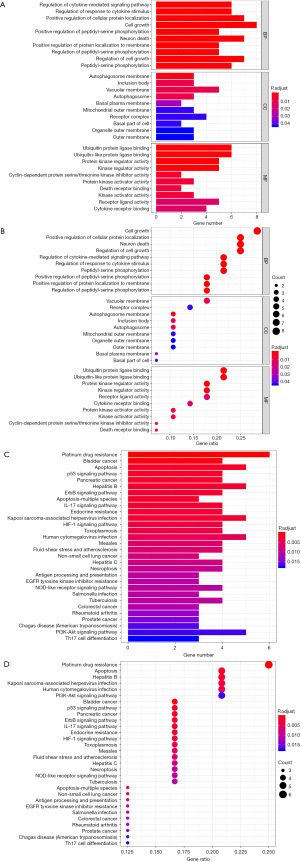
Target gene protein interaction network and core target genes
A total of 602 target genes were analyzed and the key target genes with the most nodes were used to construct the PPI core network map (Figure 4).
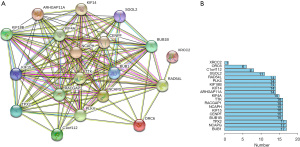
Analysis of the cell signaling pathway
The cell signal transduction pathway is composed of specific cells that secrete and release intercellular and intracellular information substances (Such as transport carriers and transport pathways) and target cells (Including specific receptors, etc.) (Figure 5).
Risk curve analysis
The “PHEATMap” package in R software was used to rank and collate the downloaded The Cancer Genome Atlas (TCGA) data, calculate the risk coefficient of patients with neuroblastoma, calculate the distribution matrix of survival and death patients, analyze the differential expression of immune infiltration-related prognostic Long non-coding RNA (lncRNA), and successfully construct the prognosis model of neuroblastoma. From left to right, we found that with the increase of the expression of lncRNA quantity, the prognosis of patients with neuroblastoma risk coefficient also rose, while deaths were focused on the right side of the high risk group of regional (Figure 6).
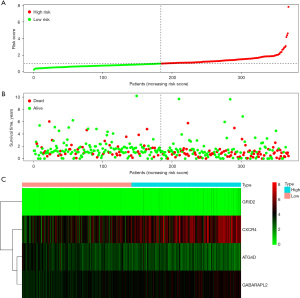
Receiver operating characteristic (ROC) curve analysis of the prognostic model
A prognostic model of neuroblastoma was successfully constructed through TCGA public database, and ROC curve analysis was used to verify the possibility and accuracy of the model. The area under the ROC curve was significantly greater than 0.7, indicating that compared with other clinically relevant indicators, the higher prediction accuracy of this model has certain predictive value for the clinical prognosis of patients with neuroblastoma (Figure 7).
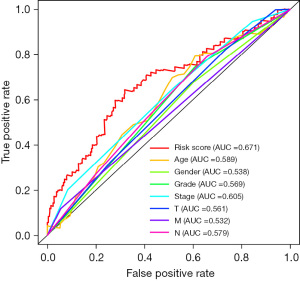
Discussion
Previous studies have found that target genes can inhibit the migration, proliferation, and metastasis of colon cancer and thyroid cancer (17). MiR-135b can promote the proliferation and metastasis of breast cancer, gastric cancer, and other tumors through the effect of target genes, and inhibit the occurrence, development, and metastasis of melanoma and other tumors (18-20). In this study, target genes of the differentially expressed miRNAs in neuroblastoma samples were identified and their functions were annotated. The target genes of the differentially expressed miRNAs were mainly located in the extracellular matrix region of proteins (21) and were related to cell response to amino acid stimulation, collagen catabolic process, negative regulation of cytoplasmic translation, Mrna 3'-UTR binding, and inhibition of translation factor activity by binding to nucleic acid (22). Furthermore, the differentially expressed miRNAs were involved in RNA dehydrolysis, mucin-type O-glycan biosynthesis, adhesion pathway, PI3K-Akt signaling pathway, protein digestion and absorption, and other processes. Some reports have shown that PTEN/PI3K/Akt signaling pathway is related to cell proliferation, apoptosis, and angiogenesis, and is involved in the invasion and metastasis of gastric cancer (23-26).
The PPI network was constructed using STRING, and the 10 core genes with highest correlation were screened using the Cytoscape software (27). Interleukin-6 (IL-6) is an inflammatory cytokine involved in a variety of biological processes, including immune dysregulation in diseases and cancer. IL-6 affects the occurrence and development of cancer by affecting the apoptosis, survival, proliferation, invasion, and metastasis of cancer cells. Studies comparing high-, low-, and intermediate-risk neuroblastoma patients found that the levels of IL-6 in high-risk neuroblastoma was significantly increased (P<0.001), and elevated IL-6 levels were associated with poor prognosis in high-risk neuroblastoma patients (28-30). IL-6 can promote the growth of neuroblastoma cells and contribute to the formation of a bone marrow microenvironment that is conducive to the progression of metastatic neuroblastoma, thus promoting the bone metastasis of neuroblastoma. Anti-IL-6 therapy has been used to treat immunological disorders and cancer, and has been shown to be effective in Castleman’s disease and inflammatory diseases (such as rheumatoid arthritis) without significant toxicity (31).
NB is derived from intrinsic migratory neural crest cells and has a high potential for distant metastasis, and patients often present with bone marrow metastases at the time of diagnosis (32). Surgical resection is still the most effective treatment for the disease, but the prognosis is poor for advanced cases and patients with distant metastases. Therefore, it is of great significance to study the molecular mechanisms of NB bone marrow metastasis (33,34). The differentially expressed genes in NB samples were imported into the STRING database and a PPI map network was constructed using Cytoscape software. The MCC algorithm in CytoHubba plug-in was used to analyze the top 10 hub genes.
Limitations
Only 7 normal fetal adrenal NB samples were selected as the control group. It was relatively few. Further study was still needed.
Conclusions
In summary, the key genes screened and identified in this study are related to the occurrence, development, migration, and invasion of neuroblastoma cells. However, whether they are related to the regulation of miRNA and whether miRNA can affect the occurrence and development of neuroblastomas through these genes warrant further investigation. Core genes are related to the proliferation and migration of other tumors, and their relationship with neuroblastoma also requires further study (35). The results of this analysis provide a valuable reference for elucidating the molecular mechanisms of miRNA in the occurrence and development of neuroblastoma, and provide new ideas and a theoretical basis for studying the pathogenesis, differentiation, and metastasis of NB.
Acknowledgments
Funding: None.
Footnote
Reporting Checklist: The authors have completed the TRIPOD reporting checklist. Available at https://tp.amegroups.com/article/view/10.21037/tp-22-504/rc
Conflicts of Interest: All authors have completed the ICMJE uniform disclosure form (available at https://tp.amegroups.com/article/view/10.21037/tp-22-504/coif). The authors have no conflicts of interest to declare.
Ethical Statement: The authors are accountable for all aspects of the work in ensuring that questions related to the accuracy or integrity of any part of the work are appropriately investigated and resolved. The study was conducted in accordance with the Declaration of Helsinki (as revised in 2013).
Open Access Statement: This is an Open Access article distributed in accordance with the Creative Commons Attribution-NonCommercial-NoDerivs 4.0 International License (CC BY-NC-ND 4.0), which permits the non-commercial replication and distribution of the article with the strict proviso that no changes or edits are made and the original work is properly cited (including links to both the formal publication through the relevant DOI and the license). See: https://creativecommons.org/licenses/by-nc-nd/4.0/.
References
- Rombo SE, Ursino D. Integrative bioinformatics and omics data source interoperability in the next-generation sequencing era-Editorial. Brief Bioinform 2021;22:1-2. [Crossref] [PubMed]
- Costa MC, Gabriel AF, Enguita FJ. Bioinformatics Research Methodology of Non-coding RNAs in Cardiovascular Diseases. Adv Exp Med Biol 2020;1229:49-64. [Crossref] [PubMed]
- Smaïl-Tabbone M, Rance B. Section Editors for the IMIA Yearbook Section on Bioinformatics and Translational Informatics. Contributions from the 2019 Literature on Bioinformatics and Translational Informatics. Yearb Med Inform 2020;29:188-92. [Crossref] [PubMed]
- Chen C, Hou J, Tanner JJ, et al. Bioinformatics Methods for Mass Spectrometry-Based Proteomics Data Analysis. Int J Mol Sci 2020;21:2873. [Crossref] [PubMed]
- Zhang Y, Zheng J. Bioinformatics of Metalloproteins and Metalloproteomes. Molecules 2020;25:3366. [Crossref] [PubMed]
- Ali MM, Hamid M, Saleem M, et al. Status of Bioinformatics Education in South Asia: Past and Present. Biomed Res Int 2021;2021:5568262. [Crossref] [PubMed]
- Pittard WS, Villaveces CK, Li S. A Bioinformatics Primer to Data Science, with Examples for Metabolomics. Methods Mol Biol 2020;2104:245-63. [Crossref] [PubMed]
- Auslander N, Gussow AB, Koonin EV. Incorporating Machine Learning into Established Bioinformatics Frameworks. Int J Mol Sci 2021;22:2903. [Crossref] [PubMed]
- Paul P, Antonydhason V, Gopal J, et al. Bioinformatics for Renal and Urinary Proteomics: Call for Aggrandization. Int J Mol Sci 2020;21:961. [Crossref] [PubMed]
- Rotroff DM. A Bioinformatics Crash Course for Interpreting Genomics Data. Chest 2020;158:S113-23. [Crossref] [PubMed]
- Branco I, Choupina A. Bioinformatics: new tools and applications in life science and personalized medicine. Appl Microbiol Biotechnol 2021;105:937-51. [Crossref] [PubMed]
- Van Camp PJ, Haslam DB, Porollo A. Bioinformatics Approaches to the Understanding of Molecular Mechanisms in Antimicrobial Resistance. Int J Mol Sci 2020;21:1363. [Crossref] [PubMed]
- Wu L, Zheng Y, Liu J, et al. Comprehensive evaluation of the efficacy and safety of LPV/r drugs in the treatment of SARS and MERS to provide potential treatment options for COVID-19. Aging (Albany NY) 2021;13:10833-52. [Crossref] [PubMed]
- Nakashima S, Nacher JC, Song J, et al. An Overview of Bioinformatics Methods for Analyzing Autism Spectrum Disorders. Curr Pharm Des 2020;25:4552-9. [Crossref] [PubMed]
- Feero WG. Bioinformatics, Sequencing Accuracy, and the Credibility of Clinical Genomics. JAMA 2020;324:1945-7. [Crossref] [PubMed]
- Medema MH. The year 2020 in natural product bioinformatics: an overview of the latest tools and databases. Nat Prod Rep 2021;38:301-6. [Crossref] [PubMed]
- Sevigny JL, Norenburg JL, Leasi F. A Bioinformatics Tutorial for Comparative Development Genomics in Diverse Meiofauna. Methods Mol Biol 2021;2219:289-305. [Crossref] [PubMed]
- Wu L, Zheng Y, Ruan X, et al. Long-chain noncoding ribonucleic acids affect the survival and prognosis of patients with esophageal adenocarcinoma through the autophagy pathway: construction of a prognostic model. Anticancer Drugs 2022;33:e590-603. [Crossref] [PubMed]
- Wratten L, Wilm A, Göke J. Reproducible, scalable, and shareable analysis pipelines with bioinformatics workflow managers. Nat Methods 2021;18:1161-8. [Crossref] [PubMed]
- Zafar A, Wang W, Liu G, et al. Molecular targeting therapies for neuroblastoma: Progress and challenges. Med Res Rev 2021;41:961-1021. [Crossref] [PubMed]
- Irwin MS, Naranjo A, Zhang FF, et al. Revised Neuroblastoma Risk Classification System: A Report From the Children's Oncology Group. J Clin Oncol 2021;39:3229-41. [Crossref] [PubMed]
- Wienke J, Dierselhuis MP, Tytgat GAM, et al. The immune landscape of neuroblastoma: Challenges and opportunities for novel therapeutic strategies in pediatric oncology. Eur J Cancer 2021;144:123-50. [Crossref] [PubMed]
- Floros KV, Cai J, Jacob S, et al. MYCN-Amplified Neuroblastoma Is Addicted to Iron and Vulnerable to Inhibition of the System Xc-/Glutathione Axis. Cancer Res 2021;81:1896-908. [Crossref] [PubMed]
- Zafar A, Wang W, Liu G, et al. Targeting the p53-MDM2 pathway for neuroblastoma therapy: Rays of hope. Cancer Lett 2021;496:16-29. [Crossref] [PubMed]
- Wu L, Zhong Y, Wu D, et al. Immunomodulatory Factor TIM3 of Cytolytic Active Genes Affected the Survival and Prognosis of Lung Adenocarcinoma Patients by Multi-Omics Analysis. Biomedicines 2022;10:2248. [Crossref] [PubMed]
- Kamili A, Atkinson C, Trahair TN, et al. Mouse models of high-risk neuroblastoma. Cancer Metastasis Rev 2020;39:261-74. [Crossref] [PubMed]
- Kildisiute G, Kholosy WM, Young MD, et al. Tumor to normal single-cell mRNA comparisons reveal a pan-neuroblastoma cancer cell. Sci Adv 2021;7:eabd3311. [Crossref] [PubMed]
- Lundberg KI, Treis D, Johnsen JI. Neuroblastoma Heterogeneity, Plasticity, and Emerging Therapies. Curr Oncol Rep 2022;24:1053-62. [Crossref] [PubMed]
- Tonini GP, Capasso M. Genetic predisposition and chromosome instability in neuroblastoma. Cancer Metastasis Rev 2020;39:275-85. [Crossref] [PubMed]
- Olivera GG, Urtasun A, Sendra L, et al. Pharmacogenetics in Neuroblastoma: What Can Already Be Clinically Implemented and What Is Coming Next? Int J Mol Sci 2021;22:9815. [Crossref] [PubMed]
- Nolan JC, Frawley T, Tighe J, et al. Preclinical models for neuroblastoma: Advances and challenges. Cancer Lett 2020;474:53-62. [Crossref] [PubMed]
- Wu L, Zhong Y, Yu X, et al. Selective poly adenylation predicts the efficacy of immunotherapy in patients with lung adenocarcinoma by multiple omics research. Anticancer Drugs 2022;33:943-59. [Crossref] [PubMed]
- Kovalevich J, Santerre M, Langford D. Considerations for the Use of SH-SY5Y Neuroblastoma Cells in Neurobiology. Methods Mol Biol 2021;2311:9-23. [Crossref] [PubMed]
- Hochheuser C, Windt LJ, Kunze NY, et al. Mesenchymal Stromal Cells in Neuroblastoma: Exploring Crosstalk and Therapeutic Implications. Stem Cells Dev 2021;30:59-78. [Crossref] [PubMed]
- Mastrangelo S, Attinà G, Ruggiero A. 131-I-metaiodobenzylguanidine and chemotherapy for advanced neuroblastoma. Expert Rev Clin Pharmacol 2021;14:1325-7. [Crossref] [PubMed]
(English Language Editor: J. Jones)