Pathogenesis of bronchopulmonary dysplasia in preterm neonates revealed by an RNA sequencing interaction network analysis
Highlight box
Key findings
• We revealed the
What is known and what is new?
• The aberration of lncRNA-miRNA-mRNA triplet can disrupt gene expression patterns and lead to the development of disease.
• This study identified 1 key upregulated lncRNA (i.e.,
What is the implication, and what should change now?
• This key lncRNA gene could serve as a promising diagnostic biomarker for prenatal examinations.
Introduction
The chronic lung condition known as bronchopulmonary dysplasia (BPD) is most commonly found in preterm infants, and individuals with respiratory distress syndrome and other conditions that require prolonged mechanical ventilation (1). The occurrence of BPD is complicated, but it is essentially caused by injury to the developing lung due to oxygen toxicity, inflammation, and other factors (2,3). Since the first report of BPD, its phenotype has evolved from a fibrocystic disease that initially affected late preterm infants to parenchymal development impairment and vascular growth disorder, mainly affecting infants born before 29 weeks of gestation (4). BPD is mainly caused by genetic predispositions, lung hypoplasia, acute lung injury, and deviant post-injury repair (5,6). Recently, the incidence of BPD has been increasing year by year, mainly due to chronic respiratory diseases in infancy (1,7). The incidence of BPD in premature infants <28 weeks of gestation is estimated to be 48–68% (1). The molecular mechanism of BPD remains unclear, and as a result, it has become a major problem for neonatologists worldwide. Thus, exploring the molecular mechanism and the clinical biomarkers of BPD is of great significance in improving the survival and treatment of BPD neonatal infants.
Recent years, the potential of RNAs as disease markers and as powerful innovative therapeutics has been widely recognized. RNA sequencing (RNA-seq) is quantitative to be used to determine RNA expression levels, allowing for a robust comparison between diseased and healthy tissues (8). Long non-coding RNAs (lncRNAs) are present in all mammalian cells (9). They are crucial in controlling molecular processes, such as gene expression, transcription, and RNA processing (10). There is increasing evidence that lncRNAs are essential for cell growth and development, and in the onset and development of many disorders (11). Decade-long research on the crosstalk between lncRNAs and mRNAs has revealed multiple layers of regulatory circuits in cells, including protein stability and messenger RNA (mRNA) turnover (12).
LncRNAs and microRNAs (miRNAs) mutually regulate their levels, and commonly affect mRNAs (13). Conversely, mRNAs also affect the expression of lncRNAs in multiple ways (14). For example, the muscle-specific lncRNA linc-MD1 enhances the production of MAML1 and MEF2C mRNA by inhibiting the binding of miR-133 and miR-135 to their targets. This interaction is called the lncRNA-miRNA-mRNA triplet, and its aberration can disrupt gene expression patterns and lead to the development of disease (15).
In this study, we performed RNA-seq on peripheral blood samples collected from the enrolled preterm neonatal BPD patients. We also conducted a series of bioinformatics analyses to determine the key lncRNA in BPD, and preliminarily explored a blood-based BPD diagnosis and prediction model. The differentially expressed mRNAs and miRNAs that were strongly correlated with the key lncRNA were examined to determine the molecular mechanism. We sought to comprehensively explore the potential mechanism and clinical biomarkers of BPD. Finally, we demonstrated for the first time that MERGE.57185.1 is involved in the progression of BPD by regulating hsa-miR-6833-5p and eukaryotic translation initiation factor 5A (EIF5A). We present the following article in accordance with the STREGA reporting checklist (available at https://tp.amegroups.com/article/view/10.21037/tp-22-590/rc).
Methods
Blood sample collection
The data of 3 preterm neonates with BPD and 3 age-matched preterm neonates without BPD were collected for RNA-seq. BPD was diagnosed based on the National Institute of Child Health and Human Development guidelines at Dongguan Children’s Hospital Affiliated to Guangdong Medical University from June 2020 to September 2020. The following diagnostic criteria were used: (I) oxygen therapy is still required after 36 weeks corrected gestational age; and (II) imaging scans show typical features of BPD, such as texture enhancement, decreased permeability, emphysema, and cystic changes. Any neonates with congenital heart disease, infectious disease, pneumothorax, or other lung diseases were excluded from the study. The study was conducted in accordance with the Declaration of Helsinki (as revised in 2013). This study was approved by the Clinical Ethics Committee of Dongguan Children’s Hospital Affiliated to Guangdong Medical University (No. LL2022083101). The neonates’ families signed the written informed consent form.
RNA-seq and Identification of differentially expressed lncRNAs, mRNAs, and miRNAs
RNA extraction from 3 BPD and 3 age-matched neonates without BPD was used for transcriptomic profiling by RNA-seq. RNA was transcribed into cDNA using the SMARter Ultra Low Amplification kit (Clonetech, Mountain, CA, USA). The quality of cDNA was assessed using Agilent Bioanalyzer 2100 (Santa Clara, CA, USA) and quantity was determined with the Qubit Flourometer (Life Technologies, Grand Island, NY, USA). Libraries were pooled and sequenced on the Illumina HiSeq 2500 machine (Illumina, San Diego, CA, USA) to generate reads/sample. Reads generated from the sequencer were aligned using the TopHat algorithm and expression values were summarized using HTSeq. Based on the bulk RNA-seq data, the differentially expressed lncRNAs, mRNAs, and miRNAs were identified, and a correction for the multiple comparisons was performed using the limma method. The thresholds for screening were as follows: P<0.05, fold change (FC) >2 for upregulation and FC <0.5 for downregulation. Volcano plots of the gene expression profiles were plotted by R package ggplots to present the results.
Weighted gene co-expression network analysis (WGCNA)
Next, we conducted a WGCNA with the R program to create co-expression networks using the lncRNAs from the RNA-seq data. A soft threshold power with a scale-free R2 value of 0.85 and a slope close to 1 was used to transform the adjacency matrix into a topological overlap matrix. To create a compact network, we integrated the modules with a high degree of similarity by setting the soft threshold power for module recognition and network development to 18. Correlations between modules and disease states were used to assess the module-trait connections. The Pearson coefficients between the module eigengenes and disease status were calculated for each module to identify the modules significantly associated with BPD (P<0.05). The modules significantly correlated with the disease states were considered modules of interest. The dark-red module was considered the key module.
The identification of the key lncRNA
Venn diagrams of the dark-red module genes and differentially expressed lncRNAs were generated using the Venn diagram package in R software to select the candidate genes. Next, the Sangerbox 3.0 website was used (http://vip.sangerbox.com/home.html) to perform the gene expression analysis on the candidate genes. The lncRNA with the most significant result was considered the key lncRNA. Finally, the co-expression network of the key lncRNA and differentially expressed mRNAs and miRNAs was constructed using Cytoscape software, and the correlated genes then underwent a Gene Ontology [GO; biological process (BP), molecular function (MF), cellular component (CC)] annotation analysis and a Kyoto Encyclopedia of Gene and Genome (KEGG) pathway enrichment analysis.
Gene set enrichment analysis (GSEA)
We performed a Spearman correlation analysis on the key lncRNA and its correlated miRNAs and mRNAs to identify the key miRNA and mRNA using the Sangerbox 3.0 website (http://vip.sangerbox.com/home.html). Next, their correlations with the key lncRNA and expression levels in BPD and normal tissues were investigated. The biological pathways of the key mRNA were also explored by a GSEA.
Statistical analysis
The study was carried out independently and repeatedly for at least three times. The data were analyzed by SPSS 19.0 and represented by mean ± standard deviation. The differences of the data were analyzed by student t-test. P<0.05 was considered to have statistical differences.
Results
The differentially expressed lncRNAs, miRNAs, and mRNAs
RNA-seq was attempted and successfully completed in 3 preterm neonates with BPD and 3 age-matched preterm neonates without BPD. There was no significant difference in the mean gestational age at birth and the corrected gestational age at the time of blood sample collection between the two groups (28.6±2.1 vs. 31.4±0.2 weeks, P=0.147; 39.6±2.4 vs. 36.6±2.3 weeks, P=0.195). We applied limma to screen for reliable differently expressed genes (DEGs) based on the RNA-seq data. In total, 158 significantly differentially expressed lncRNAs were identified, including 53 upregulated and 105 downregulated lncRNAs (Figure 1A,1B). In terms of the miRNAs, 5 upregulated and 8 downregulated differentially expressed miRNAs were identified (Figure 1C,1D). As for the mRNAs, 21 upregulated and 20 downregulated differentially expressed mRNAs were identified (Figure 1E,1F). In the volcano maps, the upregulated genes are represented by red plots, and the downregulated genes are represented by blue plots. In the heatmaps, blue represents the BPD group, and red represents the control group.
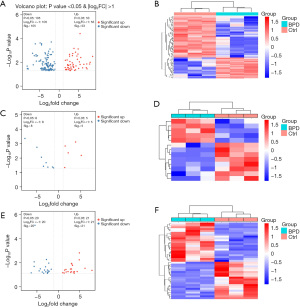
The dark-red module was identified as the key module by the WGCNA of the lncRNA genes in the RNA-seq data
The WGCNA software package was used to identify the key module. A scale-free network was generated by setting the soft threshold power to 18 (scale-free R2=0.85) (Figure 2A). The gene clustering dendrogram and module-trait relationship are shown in Figure 2B-2D. Ultimately, 22 modules were identified, of which, the dark-red module had the strongest correlation with BPD. Thus, the dark-red module was considered the key module (Figure 2E).
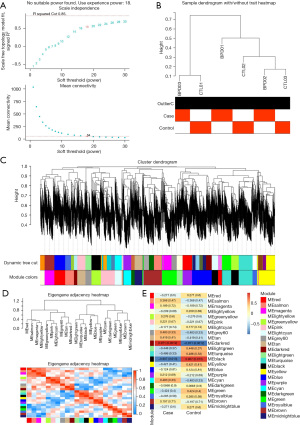
MERGE.57185.1 is the key lncRNA in BPD
From the Venn diagram in Figure 3A, a total of 21 overlapping genes were identified from the key module and differentially expressed lncRNAs. These overlapping genes were all highly expressed in the BPD group, but lowly expressed in the control group (Figure 3B,3C). Among them, MERGE.57185.1 had the most significant P value (P=0.00067), and thus was selected as the key lncRNA in this study. Next, we combined the differentially expressed miRNAs and mRNAs to construct a MERGE.57185.1-miRNAs-mRNAs co-expression network (P<0.05; Figure 3D). The genes connected by the red lines represent the mRNAs and miRNAs co-expressed with the lncRNAs.
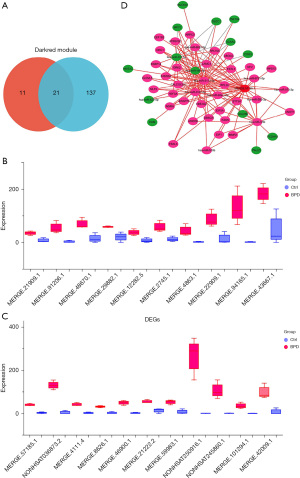
Biological and functional enrichment analyses of the co-expressed mRNAs and miRNAs
To examine the biological roles of the co-expressed mRNAs and miRNAs, we conducted GO functional and KEGG pathway analyses. The GO analysis results showed that the co-expressed mRNAs were mainly related to the cellular protein metabolic process (BP), ribosome (CC), and RNA binding (MF) (Figure 4A-4C). The KEGG pathway analysis results showed that the co-expressed mRNAs were mainly related to ribosome, Coronavirus disease, tight junction, fatty acid biosynthesis, and protein processing in the endoplasmic reticulum (Figure 4D). Additionally, the co-expressed miRNAs were mainly involved in the BPs of organophosphate metabolic process and thymus epithelium morphogenesis (Figure 4E), and the MF of phosphatidylinositol-4,5-bisphosphate 5-phosphatase activity (Figure 4F).
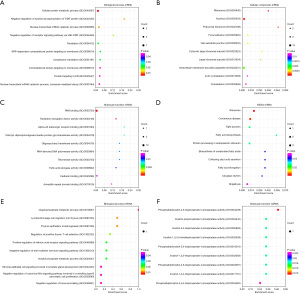
Hsa-miR-6833-5p and EIF5A are the key miRNA and mRNA, respectively, of MERGE.57185.1 in BPD
A Spearman correlation analysis was then performed on the MERGE.57185.1 and co-expressed mRNAs and miRNAs (Figure 5A). The results showed that hsa-miR-6833-5p and EIF5A (a mRNA and miRNA, respectively) had the strongest correlations with MERGE.57185.1. In terms of the correlation results, MERGE.57185.1 was negatively correlated with hsa-miR-6833-5p (r=−0.96; Figure 5B), but positively correlated with EIF5A (r=0.99; Figure 5C). We also analyzed the levels of hsa-miR-6833-5p and EIF5A in BPD. The expression level of hsa-miR-6833-5p was lower in BPD (Figure 5D), but the expression level of EIF5A was higher in BPD (Figure 5E). Moreover, the EIF5A-enriched GSEA pathways included pyruvate metabolism, focal adhesion, beta alanine metabolism, the mechanistic target of rapamycin (mTOR) signaling pathway, the transforming growth factor-β (TGF-β) signaling pathway, and the chemokine signaling pathway (Figure 5F).
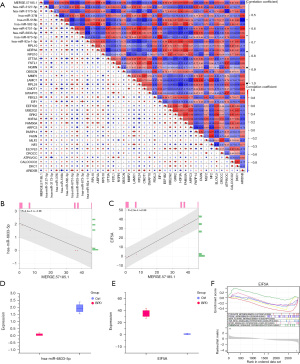
Discussion
Lung hypoplasia due to BPD may lead to respiratory insufficiency, which is the main cause of perinatal mortality in neonates (16). Currently, BPD is an overwhelming problem for preterm infants, but its exact mechanism at the molecular level is still unclear (4,17). Thus, exploring the molecular mechanisms and clinic biomarkers of BPD is of great value in improving the diagnosis and treatment of BPD. In this study, we took blood samples from 3 neonates with BPD and 3 controls for RNA-seq and performed a series of bioinformatics analyses to explore the mechanism of BPD in a lncRNA-miRNA-mRNA network.
LncRNAs play a number of roles in the emergence and progression of human illnesses (18,19). LncRNAs also play significant regulatory roles in the pathophysiology and progression of the lung (20,21). For example, in chronic obstructive pulmonary disease, the maternally expressed gene 3 is significantly overexpressed, which further promotes the apoptosis of human lung microvascular endothelial cells by upregulating the expression of pro-apoptotic proteins (22). Recently, it was reported that 882 lncRNAs were upregulated and 887 lncRNAs were downregulated in lung tissues from a mouse model of BPD compared to controls, which suggests that lncRNAs play crucial roles in the progression of BPD (23). In the current investigation, we discovered that infants with BPD had considerably higher expression levels of MERGE.57185.1 in their peripheral blood samples than infants without BPD. Thus, similar to a few recent studies, we identified MERGE.57185.1 as the key lncRNA. But there is no relevant report on the function of lncRNA-MERGE. 57185.1 in the current literature.
Next, we constructed the MERGE.57185.1 co-expression network of differentially expressed miRNAs and mRNAs, and then performed robust biological enrichment analyses of the co-expressed genes. The enrichment results indicated that these co-expressed mRNAs were mainly associated with GO terms, such as membrane-targeted co-translation proteins and membrane-targeted signal recognition particle (SRP)-dependent co-translation proteins, and KEGG pathways, such as cadherin binding and oligosaccharide transferase activity. This suggests that these mRNAs are closely related to the translation of proteins. In addition, the main enrichment pathways of the miRNAs included phosphoinositide metabolism, the retinoic acid receptor signaling pathway and inositol-1,3,4,5-tetraphosphate 5-phosphatase activity.
Spengler et al. showed that inositol derivatives maintained alveolar-capillary barrier functions, reduced alveolar epithelial cell apoptosis, and pulmonary edema in a neonatal piglet acute respiratory distress syndrome (ARDS) model (24). In recent research, an experimental emphysema rat model was used to stimulate alveolar regeneration when exogenous retinoic acid, a bioactive derivative of vitamin A, was administered (25). Fernandes-Silva et al. showed that retinoic acid plays a crucial role during lung developmental phases and pulmonary vascular development (26). Both human infant and animal studies have demonstrated that myo-inositol may play a critical role in development of both the central and peripheral respiratory neural control system (27).
The results of the Spearman correlation analysis showed that hsa-miR-6833-5p and EIF5A had the strongest correlation with MERGE.57185.1. There are few reports on hsa-miR-6833-5p; however, Lan et al. conducted a transcriptome analysis and showed that hsa-miR-6833-5p was a key mediating miRNA in type 2 diabetes (28). In our study hsa-miR-6833-5p was lowly expressed in BPD and negatively correlated with MERGE.57185.1.
EIF5A is an unique amino acid of the well-known EIF family and is related to translation factor initiation and elongation, which is necessary for cell proliferation (29,30). Li et al. investigated the relationship between hepatocellular carcinoma (HCC) and EIF5A, and found that EIF5A was abundant in HCC cell lines (31). In our study, EIF5A was upregulated in BPD and positively correlated with the expression of MERGE.57185.1. Further, we showed that EIF5A was the key factor of BPD, and our results are consistent with those of previous research results on severe sepsis and acute lung injury (32).
We also specifically examined the enrichment pathways of EIF5A in GSEA, which mainly included the TGF-β and the chemokine signaling pathways. The TGF-β signaling pathway has an important function in pulmonary vascular development (33,34). The findings of Jin et al. suggest that disrupting the relationship between the TGF-β-ALK1-Smad1/5 and TGF-β-ALK5-Smad2/3 pathways in lung endothelial cells may result in the occurrence of BPD (35). Taken together, we conclude that the MERGE.57185.1/hsa-miR-6833-5p/EIF5A axis is present in the progression of BPD.
Our study had some limitations. First, our sample size was small. However, the clinical manifestations of the selected subjects are very typical and representative. Second, our findings have not yet been verified in animal experiments, but we intend to conduct supplementary research in the future.
Conclusions
In conclusion, our research shows that MERGE. 57185.1 may play an important role in the pathogenesis of BPD, mainly in the stage of lung and pulmonary vascular developmental phases, through lncRNA-miRNA-mRNA network. Thus, MERGE.57185.1 can be used as a diagnostic biomarker for the screening and prediction of neonatal BPD. Our findings may help to elucidate the mechanisms of BPD and identify a new clinical biomarker for the diagnosis and therapy of BPD neonates.
Acknowledgments
Funding: This work was supported by the Dongguan Social Science and Technology Development Project (No. 20221800905362).
Footnote
Reporting Checklist: The authors have completed the STREGA reporting checklist. Available at https://tp.amegroups.com/article/view/10.21037/tp-22-590/rc
Data Sharing Statement: Available at https://tp.amegroups.com/article/view/10.21037/tp-22-590/dss
Conflicts of Interest: All authors have completed the ICMJE uniform disclosure form (available at https://tp.amegroups.com/article/view/10.21037/tp-22-590/coif). All authors report funding support from the Dongguan Social Science and Technology Development Project (No. 20221800905362). The authors have no other conflicts of interest to declare.
Ethical Statement: The authors are accountable for all aspects of the work in ensuring that questions related to the accuracy or integrity of any part of the work are appropriately investigated and resolved. The study was conducted in accordance with the Declaration of Helsinki (as revised in 2013). This study was approved by the Clinical Ethics Committee of Dongguan Children’s Hospital Affiliated to Guangdong Medical University (No. LL2022083101). The neonates’ family signed the written informed consent form.
Open Access Statement: This is an Open Access article distributed in accordance with the Creative Commons Attribution-NonCommercial-NoDerivs 4.0 International License (CC BY-NC-ND 4.0), which permits the non-commercial replication and distribution of the article with the strict proviso that no changes or edits are made and the original work is properly cited (including links to both the formal publication through the relevant DOI and the license). See: https://creativecommons.org/licenses/by-nc-nd/4.0/.
References
- Davidson LM, Berkelhamer SK. Bronchopulmonary Dysplasia: Chronic Lung Disease of Infancy and Long-Term Pulmonary Outcomes. J Clin Med 2017;6:4. [Crossref] [PubMed]
- Cannavò L, Perrone S, Viola V, et al. Oxidative Stress and Respiratory Diseases in Preterm Newborns. Int J Mol Sci 2021;22:12504. [Crossref] [PubMed]
- Surate Solaligue DE, Rodríguez-Castillo JA, Ahlbrecht K, et al. Recent advances in our understanding of the mechanisms of late lung development and bronchopulmonary dysplasia. Am J Physiol Lung Cell Mol Physiol 2017;313:L1101-53. [Crossref] [PubMed]
- Gilfillan M, Bhandari A, Bhandari V. Diagnosis and management of bronchopulmonary dysplasia. BMJ 2021;375:n1974. [Crossref] [PubMed]
- Hwang JS, Rehan VK. Recent Advances in Bronchopulmonary Dysplasia: Pathophysiology, Prevention, and Treatment. Lung 2018;196:129-38. [Crossref] [PubMed]
- Abdellatif MAK, Eyada E, Rabie W, et al. Genetic and Biochemical Predictors of Neonatal Bronchopulmonary Dysplasia. J Pediatr Genet 2022;11:173-8. [Crossref] [PubMed]
- Thébaud B, Goss KN, Laughon M, et al. Bronchopulmonary dysplasia. Nat Rev Dis Primers 2019;5:78. [Crossref] [PubMed]
- Lucchinetti E, Zaugg M. RNA Sequencing. Anesthesiology 2020;133:976-8. [Crossref] [PubMed]
- Andergassen D, Rinn JL. From genotype to phenotype: genetics of mammalian long non-coding RNAs in vivo. Nat Rev Genet 2022;23:229-43. [Crossref] [PubMed]
- Sun Q, Hao Q, Prasanth KV. Nuclear Long Noncoding RNAs: Key Regulators of Gene Expression. Trends Genet 2018;34:142-57. [Crossref] [PubMed]
- Xu Y, Ge Z, Zhang E, et al. The lncRNA TUG1 modulates proliferation in trophoblast cells via epigenetic suppression of RND3. Cell Death Dis 2017;8:e3104. [Crossref] [PubMed]
- Zhang P, Lu Y, Kong Z, et al. Androgen-responsive lncRNA LINC00304 promotes cell cycle and proliferation via regulating CCNA1. Prostate 2019;79:994-1006. [Crossref] [PubMed]
- Zhang J, Liu L, Li J, et al. LncmiRSRN: identification and analysis of long non-coding RNA related miRNA sponge regulatory network in human cancer. Bioinformatics 2018;34:4232-40. [Crossref] [PubMed]
- Ransohoff JD, Wei Y, Khavari PA. The functions and unique features of long intergenic non-coding RNA. Nat Rev Mol Cell Biol 2018;19:143-57. [Crossref] [PubMed]
- Cesana M, Cacchiarelli D, Legnini I, et al. A long noncoding RNA controls muscle differentiation by functioning as a competing endogenous RNA. Cell 2011;147:358-69. [Crossref] [PubMed]
- Principi N, Di Pietro GM, Esposito S. Bronchopulmonary dysplasia: clinical aspects and preventive and therapeutic strategies. J Transl Med 2018;16:36. [Crossref] [PubMed]
- Pasha AB, Chen XQ, Zhou GP. Bronchopulmonary dysplasia: Pathogenesis and treatment. Exp Ther Med 2018;16:4315-21. [Crossref] [PubMed]
- Tsagakis I, Douka K, Birds I, et al. Long non-coding RNAs in development and disease: conservation to mechanisms. J Pathol 2020;250:480-95. [Crossref] [PubMed]
- Lin W, Zhou Q, Wang CQ, et al. LncRNAs regulate metabolism in cancer. Int J Biol Sci 2020;16:1194-206. [Crossref] [PubMed]
- Li Y, Yin Z, Fan J, et al. The roles of exosomal miRNAs and lncRNAs in lung diseases. Signal Transduct Target Ther 2019;4:47. [Crossref] [PubMed]
- Lin S, Zhen Y, Guan Y, et al. Roles of Wnt/β-Catenin Signaling Pathway Regulatory Long Non-Coding RNAs in the Pathogenesis of Non-Small Cell Lung Cancer. Cancer Manag Res 2020;12:4181-91. [Crossref] [PubMed]
- Zhang L, Bai X, Yan W. LncRNA-MALAT1, as a biomarker of neonatal BPD, exacerbates the pathogenesis of BPD by targeting miR-206. Am J Transl Res 2021;13:462-79.
- Bao TP, Wu R, Cheng HP, et al. Differential expression of long non-coding RNAs in hyperoxia-induced bronchopulmonary dysplasia. Cell Biochem Funct 2016;34:299-309. [Crossref] [PubMed]
- Spengler D, Winoto-Morbach S, Kupsch S, et al. Novel therapeutic roles for surfactant-inositols and -phosphatidylglycerols in a neonatal piglet ARDS model: a translational study. Am J Physiol Lung Cell Mol Physiol 2018;314:L32-53. [Crossref] [PubMed]
- Hind M, Maden M. Retinoic acid induces alveolar regeneration in the adult mouse lung. Eur Respir J 2004;23:20-7. [Crossref] [PubMed]
- Fernandes-Silva H, Araújo-Silva H, Correia-Pinto J, et al. Retinoic Acid: A Key Regulator of Lung Development. Biomolecules 2020;10:152. [Crossref] [PubMed]
- MacFarlane PM, Di Fiore JM. Myo-inositol Effects on the Developing Respiratory Neural Control System. Adv Exp Med Biol 2018;1071:159-66. [Crossref] [PubMed]
- Lan X, Han J, Wang B, et al. Integrated analysis of transcriptome profiling of lncRNAs and mRNAs in livers of type 2 diabetes mellitus. Physiol Genomics 2022;54:86-97. [Crossref] [PubMed]
- Lubas M, Harder LM, Kumsta C, et al. eIF5A is required for autophagy by mediating ATG3 translation. EMBO Rep 2018;19:e46072. [Crossref] [PubMed]
- Dever TE, Dinman JD, Green R. Translation Elongation and Recoding in Eukaryotes. Cold Spring Harb Perspect Biol 2018;10:a032649. [Crossref] [PubMed]
- Li L, Li X, Zhang Q, et al. EIF5A expression and its role as a potential diagnostic biomarker in hepatocellular carcinoma. J Cancer 2021;12:4774-9. [Crossref] [PubMed]
- Moore CC, Martin EN, Lee G, et al. Eukaryotic translation initiation factor 5A small interference RNA-liposome complexes reduce inflammation and increase survival in murine models of severe sepsis and acute lung injury. J Infect Dis 2008;198:1407-14. [Crossref] [PubMed]
- Goumans MJ, Liu Z, ten Dijke P. TGF-beta signaling in vascular biology and dysfunction. Cell Res 2009;19:116-27. [Crossref] [PubMed]
- Zhang N, Dong M, Luo Y, et al. Danshensu prevents hypoxic pulmonary hypertension in rats by inhibiting the proliferation of pulmonary artery smooth muscle cells via TGF-β-smad3-associated pathway. Eur J Pharmacol 2018;820:1-7. [Crossref] [PubMed]
- Jin M, Lee J, Lee KY, et al. Alteration of TGF-β-ALK-Smad signaling in hyperoxia-induced bronchopulmonary dysplasia model of newborn rats. Exp Lung Res 2016;42:354-64. [Crossref] [PubMed]
(English Language Editor: L. Huleatt)