Altered gut microbiota and microbial metabolism in children with hepatic glycogen storage disease: a case-control study
Highlight box
Key findings
• Our data revealed a gut microbiota dysbiosis characterized by lower bacterial biodiversity and richness, and altered microbial metabolisms with increased abundances of primary BAs and decreased concentrations of SCFAs in hepatic GSD children as compared to that of the healthy children.
What is known and what is new?
• Studies have demonstrated that gut microbiota dysbiosis correlated with altered metabolism are implicated in liver metabolic diseases.
• Our data indicated that the altered bacterial genera were correlated with the changes of both fecal BAs and SCFAs in hepatic GSD children.
What is the implication, and what should change now?
• Future studies are needed to investigate the driver of altered gut microbiota and microbial metabolism in hepatic GSD children mediated by either the genetic defect, disease status, or diet therapy.
Introduction
Glycogen storage disease (GSD) is a group of hereditary metabolic disorders caused by the deficiency of enzymes involved in glycogen synthesis or glycolysis. The overall incidence of GSD is estimated at 1 in 20,000 to 43,000 live births (1). A total of 16 GSD types (GSD 0–XV) have been reported according to the different enzyme deficiencies, affected tissue, and clinical symptoms (2). The majority of the GSD types are inherited in an autosomal recessive mode, with the exception of X-linked GSD type IXa (1).
Since glycogen is mainly stored in the liver and muscle, GSD may affect either the liver (hepatic GSD) or the muscles (muscle GSD), or both (1). Hypoglycemia and hepatomegaly are the major manifestations of hepatic GSD (3). In addition, hepatic GSD may present with several metabolic abnormalities, including hyperlipidemia, hyperlactatemia, and hyperuricemia (3,4). GSD I, GSD III, and GSD IX are the most common types, accounting for 80% of hepatic GSD cases, which result from glucose 6-phosphatase enzyme (G6PC), glycogen debranching enzyme (AGL), and phosphorylase kinase (PHKA2) deficiency, respectively (1). A variety of complications, such as delayed growth, osteoporosis, anemia, hepatocellular adenoma, and chronic kidney disease may occur in patients with GSD caused by long-term metabolic abnormalities (3,5-7). The current standard therapies for hepatic GSD are nutritional intervention [e.g., uncooked cornstarch (UCCS)] and symptomatic supportive treatment (3,5-7).
The human gut microbiota is an intricate microbial community consisting of trillions of microbes and millions of functional genes, which is significantly vital to human health. Its composition can be influenced by diet, lifestyle, medications, and genetics (8). The gut microbiota plays a crucial role in regulation of host metabolism through the facilitation of nutrients and energy harvest from the intake of food and metabolite production (8). For instance, gut microbial cells affect the host glucose metabolism by regulating the bile acids (BAs) metabolism (9) and fermentation of short chain fatty acids (SCFAs) substrates (10). BAs can feedback their own synthesis and regulate glucose metabolism by triggering farnesoid X receptor (FXR) and G protein-coupled BA receptor 5 (TGR5) signaling (11). SCFA are formatted through fermentation of non-digestible carbohydrates by gut microbiota such as acetate, propionate, and butyrate (10). It has been reported that SCFAs regulate hepatic lipid and systemic glucose homeostasis through free fatty acid receptor 2/3 (FFAR 2/3) and peroxisome proliferator-activated receptor-γ (PPAR-γ) (10). Propionate is a substrate for gluconeogenesis in the liver, and the increased propionate flux through the liver reduces intrahepatic triglyceride (TG) level (12).
Previous studies had revealed that the gut microbiota is associated with clinical manifestations present in GSD, including obesity, liver disease, and enteropathy, implicating its important role in the disease progression of GSD (13,14). Studies found that the abundances of bacteria genera Akkermansia, Faecalibacterium, Oscillibacter, and Alistipes were decreased, whereas that of Lactobacillus reuteri was increased in people with obesity as compared with people of normal weight (15,16). The gut microbiota can affect the adiposity and glucose metabolism in the human body (17,18). Increased relative abundances of Anaerobacter, Streptococcus, Lactobacillus, and Escherichia, whereas decreased abundances of Odoribacter, Flavonifaractor, Oscillibacter, and Alistipes spp. were identified in non-alcoholic fatty liver disease (NAFLD) patients in comparison to healthy controls (HCs) (19). Recent studies have shown that the relative abundances of Enterobacteriaceae and Veillonellaceae families were increased, whereas the beneficial genera Faecalibacterium and Oscillospira were reduced in hepatic GSD patients (20,21). Therefore, investigating the associations between gut microbiota and hepatic GSD may implicate the potential clinical significance of the microbial-targeted interventions in hepatic GSD.
In the current study, we aimed to explore the features of the gut microbiota composition and microbial metabolites (SCFAs, BAs) in a group of pediatric hepatic GSD patients from China. Our data may help to further understand the associations between hepatic GSD and gut microbiota and its metabolites. We present the following article in accordance with the STROBE reporting checklist (available at https://tp.amegroups.com/article/view/10.21037/tp-22-293/rc).
Methods
Study cohort and fecal samples collection
A total of 22 pediatric hepatic GSD patients (Ia =11, III =8, IXa =3) diagnosed between February 2014 and July 2018 were enrolled from Shanghai Children’s Hospital, China (Table 1). The diagnosis of hepatic GSD was based on the guidelines of diagnosis and management of GSD (5-7). The inclusion criteria were as follows: hepatic GSD children confirmed by genetic diagnosis and/or liver biopsy pathology with the following clinical features: hypoglycemia, hepatomegaly with or without splenomegaly, growth and development delay, metabolic abnormalities of hyperlipidemia, hyperuricemia, and lactic academia (22). The exclusion criteria were as follows: patients aged less than 3 years at the time of fecal sample collection, or those who had taken antibiotics within 3 months before feces collection. All patients were routinely treated with UCCS or other necessary symptomatic management. Clinical characteristics, including demographics, clinical features, and the findings of blood and genetic tests at the time of diagnosis were retrospectively extracted from the medical records. A total of 16 healthy children who had attended the Shanghai Children’s Hospital for a health check were enrolled as the control group. The inclusion criteria of the control group were children without any previous history of chronic disease and clinically relevant GSD or symptoms of other metabolic diseases. Individuals who had taken antibiotics or any other medications within 6 months before fecal sample collection were excluded from the study. The baseline characteristics of the two groups were gender- and age-matched using the chi-squared test and Mann-Whitney U test, respectively. There were no statistical differences in gender (male 14/22, 63.6% vs. 10/16, 62.5%; P=0.98) and age [5.3 years, interquartile range (IQR) 3.5–8.7 vs. 5.0 years, IQR 5.0–6.7; P=1.00] at the time of fecal sample collection between the GSD group and the control group. A single fecal sample was obtained from each HC and GSD individual during routine follow-up. All fecal samples were stored at −80 ℃ until performing 16S ribosomal RNA (rRNA) gene sequencing and metabolites quantification.
Table 1
Characteristics | GSD Ia (n=11) | GSD III (n=8) | GSD IXa (n=3) | P value# |
---|---|---|---|---|
Male | 6 (54.5) | 6 (75.0) | 3 (100.0) | |
Age of onset (years) | 1.00 (0.63, 2,17) | 0.87 (0.83, 1.95) | 1.66 (0.89, 2.29) | 0.906 |
Age at diagnosis (years) | 2.08 (0.99, 3.37) | 1.79 (0.21, 2.71) | 2.17 (2.08, 2.59) | 0.820 |
Disease course (months) | 5.00 (2.00, 12.00) | 1.00 (0.25, 6.50) | 1.00 (0.52, 12.50) | 0.502 |
Height (Z score) | −1.10 (−1.80, −1.00) | 0.20 (−0.7, 0.50) | −0.20 (−1.00, 0.70) | 0.061 |
Liver size (cm) | 7.00 (5.65, 8.45) | 7.15 (4.00, 10.85) | 5.30 (4.90, 6.40) | 0.657 |
Blood biochemistry | ||||
ALT (U/L) | 168.00 (143.00, 210.50) | 658.00 (498.00, 759.00) | 350.00 (206.50, 445.00) | 0.002 |
AST (U/L) | 301.00 (276.50, 356.00) | 997.50 (674.50, 1,247.50) | 275.00 (173.00, 511.00) | 0.002 |
CK (U/L) | 48.00 (29.00, 90.00) | 530.00 (392.50, 853.50) | 90.00 (86.00, 114.00) | 0.002 |
TG (mmol/L) | 6.39 (3.90, 8.24) | 3.39 (1.89, 5.14) | 3.09 (2.14, 3.18) | 0.025 |
TC (mmol/L) | 4.76 (4.19, 5.18) | 4.53 (3.31, 5.75) | 4.46 (4.08, 4.62) | 0.722 |
HDL (mmol/L) | 0.74 (0.72, 0.96) | 0.40 (0.39, 0.88) | 0.78 (0.68, 0.79) | 0.722 |
LDL (mmol/L) | 2.68 (1.56, 3,17) | 1.76 (1.195, 1.97) | 2.86 (2.60, 3.02) | 0.171 |
Blood ammonia (μmol/L) | 42.00 (36.50, 54.50) | 56.50 (53.00, 61.00) | 46.00 (35.50, 47.50) | 0.244 |
Lactic acid (mmol/L) | 6.00 (4.55, 8.60) | 2.70 (1.30, 3.70) | 1.50 (1.10, 1.55) | 0.002 |
Uric acid (μmol/L) | 444.00 (368.00, 489.00) | 350.50 (251.00, 360.50) | 318.00 (260.50, 352.50) | 0.028 |
Fasting glucose (mmol/L) | 2.60 (1.85, 3.95) | 2.15 (1.56, 3.00) | 3.80 (3.35, 4.20) | 0.225 |
Abdominal ultrasound | ||||
Hepatomegaly | 11/11 (100.0) | 11/11 (100.0) | 11/11 (100.0) | |
Nephromegaly | 3/11 (27.3) | 0 | 0 | |
Liver biopsy | 5/11 (33.3) | 3/8 (41.7) | 2/3 (42.8) | |
Steatosis (+) | 4 | 1 | 1 | |
PAS staining (+) | 3 | 1 | 2 | |
Liver fibrosis (+) | 3 | 1 | 1 | |
Liver cirrhosis (+) | 1 | 0 | 0 |
Data were shown as the median and IQR (25th, 75th) or n (%). #, data were compared by the nonparametric Kruskal-Wallis H test. GSD, glycogen storage disease; ALT, alanine transaminase; AST, aspartate transaminase; CK, creatine kinase; TG, triglyceride; TC, total cholesterol; HDL, high-density lipoprotein; LDL, low-density lipoprotein; PAS, periodic acid-Schiff; IQR, interquartile range.
The study was conducted in accordance with the Declaration of Helsinki (as revised in 2013). The study was approved by the Ethical Review Board of Shanghai Children’s Hospital (No. 2021R119-E01), and informed consent was taken from the parent or legal guardian of all the participants.
DNA extraction, polymerase chain reaction amplification, and 16S rRNA sequencing
Genomic DNA was extracted from fecal samples using the QIAamp DNA Stool Mini Kit (Qiagen, Hilden, Germany). Bacterial 16S rRNA gene fragments (V3–V4) were amplified by using primers 338F (5'-ACTCCTACGGGAGGCAGCAG-3') and 806R (5'-GGACTACHVGGGTWTCTAAT-3'). The polymerase chain reaction (PCR) products were purified using the AxyPrep DNA Gel Extraction Kit (Axygen Biosciences, Union City, CA, USA). Purified amplicons were subjected to paired-end sequencing on an Illumina MiSeq PE300 platform (Illumina, Inc., San Diego, CA, USA) at Majorbio Bio-Pharm Technology Co., Ltd. (Shanghai, China).
16S rRNA gene sequences analysis
The raw 16S rRNA gene sequences were merged with FLASH (v1.2.11) (23) and quality filtered with fastp (0.19.6) (24). Then, the high-quality sequences were denoised using DADA2 (25) to obtain unique operational taxonomic unit (OTU) and further analyzed in the Qiime2 (version 2020.2) (26) pipeline with recommended parameters. Taxonomic assignment of OTU was performed using the Blast consensus taxonomy classifier implemented in Qiime2 and the SILVA 16S rRNA database (v138). Majorbio Cloud Platform was used to analyze the bacterial community composition data. The alpha diversity of the fecal microbiome was measured using the observed species richness (Sobs), abundance-based coverage estimator (ACE), Chao, and Shannon indexes. The beta diversity was calculated through principal coordinate analysis (PCoA) to Bray-Curtis distance, unweighted and weighted UniFrac metric. The relative abundance of taxa difference was analyzed using Wilcoxon rank-sum test between 2 groups.
Fecal BAs measurement
A total of 47 BAs were measured by targeted metabolomics profiling using ultra-high performance liquid chromatography-tandem mass spectrometry (UHPLC-MS/MS) as described in the literature (27). In brief, 25 mg feces were accurately weighted and then added to 200 µL extraction solution (methanol: water =4:1) by vortex for 30 seconds. The mixture was ultrasonicated at 5 ℃, 40 KHz for 30 minutes, settled at −20 ℃ for 30 minutes, and then centrifuged at 13,000 ×g at 4 ℃ for 15 minutes. Next, 100 µL of supernatant was collected and blow dried with a nitrogen blower. The precipitate was reconstituted with 30 µL acetonitrile solution. Finally, 1 µL supernatant was transferred to sample vial for BAs analysis using AB SCIEX QTRAP 6500+ UHPLC-MS/MS system (UHPLC-Qtrap; AB SCIEX, Framingham, MA, USA) and Waters BEH C18 (150 mm × 2.1 mm, 1.7 µm; Waters, Milford, MA, USA) liquid chromatography column (AB SCIEX, MA, USA). Data analysis was performed by default parameters used in AB SCIEX quantitative software OS for automatic identification and integration of each ion fragment with manual inspection assisted. The concentration of BA was calculated by the mass spectrum peak area according to the linear regression standard curve drawn with the standard solution. The differences of BAs profiling between groups were assessed by PCoA and partial least squares discriminant analysis (PLS-DA).
Fecal SCFAs quantification
Gas chromatography-mass spectrometry (GC-MS) was used to measure the fecal SCFAs levels as previously described (28). In brief, the SCFAs were extracted from 100 mg feces by adding a 900 µL methanol and 100 µL 2-ethylbutyric acid (1,000 µg/mL) as an internal standard. The mixture was homogenized at −10 ℃ for 3 minutes and was ultrasonicated for 30 minutes, and was then allowed to settle at −20 ℃ for 30 minutes. A total of 200 µL of supernatant was transferred to a 1.5 mL tube after centrifugation at 13,000 ×g at 4 ℃ for 15 minutes, after which, 50 mg anhydrous sodium sulfate was added to the tube, followed by vortex and centrifugation at 13,000 ×g, 4 ℃ for 15 minutes. Finally, the supernatant was transferred to a sample vial for SCFAs quantification by using Agilent 8890B-5977B GC/MS system and HP-FFAP (30 m × 0.25 mm × 0.25 µm) capillary column (Agilent Technologies, Santa Clara, CA, USA).
Statistical analysis
Statistical analysis was performed using SPSS 25.0 software IBM Corp., Armonk, NY, USA). Quantitative data were expressed as the median and IQR (25th–75th) or number with percentage, as appropriate. The comparability of the baseline characteristics between the two groups was tested by chi-squared test for gender, and Mann-Whitney U test for age. The data were compared with the nonparametric Mann-Whitney U (two groups) or Kruskal-Wallis H test (multiple groups). The association between bacteria taxa, SCFA, and BAs was analyzed by using Spearman correlation analysis with a two-sided P value. A P value of <0.05 was considered statistically significant.
Results
Characteristics of pediatric hepatic GSD
As shown in Table 1, of the 22 patients with hepatic GSD enrolled in the study, 15 (15/22, 68.2%) were boys. Patients were divided into 3 groups according to disease type based on genetic testing results, GSD Ia (11 patients), GSD III (8 patients), and GSD IXa (3 patients) group. There were no statistically significant differences in age at onset, disease course, height Z score, and liver size at the time of diagnosis among the three groups. Blood biochemistry tests revealed higher levels of alanine transaminase (ALT), aspartate transaminase (AST), and creatine kinase (CK) in patients with GSD III than in patients with GSD Ia and IXa. The levels of TG, lactic acid, and uric acid were significantly higher in patients with GSD Ia than patients with GSD III and IXa. Abdominal ultrasonography revealed hepatomegaly in all patients, and 3 GSD Ia patients had nephromegaly. Liver biopsy was performed in 5 patients with GSD Ia, 3 patients with GSD III, and 2 patients with GSD IXa. In the group GSD Ia, 4 patients had hepatocyte steatosis, 3 patients were periodic acid-Schiff (PAS) staining positive, 3 patients had fibrosis, and 1 patient had cirrhosis. Similar findings were observed in liver biopsy of patients with GSD III and GSD IXa, but none of them had developed cirrhosis at the time of diagnosis. All the enrolled patients were routinely treated with UCCS after the diagnosis of GSD. The duration of the UCCS treatment of each patient at the time of fecal sample collection is listed in Table 2.
Table 2
Patient no. | Gender | Age (years) | GSD type | Duration (years) |
---|---|---|---|---|
1 | Male | 5.3 | Ia | 2.8 |
2 | Female | 6.4 | Ia | 5.9 |
3 | Female | 5.2 | Ia | 4.3 |
4 | Female | 5.3 | Ia | 4.2 |
5 | Male | 3.3 | Ia | 2.3 |
6 | Male | 8.8 | Ia | 8.2 |
7 | Female | 14.3 | Ia | 7.2 |
8 | Male | 4.6 | Ia | 0.8 |
9 | Male | 11 | Ia | 8.9 |
10 | Female | 11 | Ia | 7.0 |
11 | Male | 10 | Ia | 8.0 |
12 | Female | 3.3 | III | 2.4 |
13 | Male | 4 | III | 2.0 |
14 | Male | 5.3 | III | 3.8 |
15 | Female | 4 | III | 1.1 |
16 | Male | 3.3 | III | 2.0 |
17 | Male | 15 | III | 13.7 |
18 | Male | 8.5 | III | 7.3 |
19 | Female | 4.6 | III | 0.6 |
20 | Male | 7.3 | IXa | 5.6 |
21 | Male | 3.5 | IXa | 1.4 |
22 | Male | 3.8 | IXa | 1.0 |
UCCS, uncooked cornstarch; GSD, glycogen storage disease.
Altered gut microbiota in hepatic GSD children
To characterize the gut microbiota composition associated with pediatric hepatic GSD, we performed 16S rRNA gene sequencing on 38 fecal samples from 22 hepatic GSD patients and 16 HCs. As shown in Figure 1A, alpha diversity revealed both richness and diversity, measured by the observed species (Sobs, P=0.011), ACE (P=0.011), Chao (P=0.011), and Shannon (P<0.001) indexes, were significantly lower in hepatic GSD patients than in HCs. Beta diversity analysis, represented by PCoA calculated on unweighted UniFrac distances (P=0.011), weighted UniFrac, and Bray-Curtis distance generated from genus-level taxa, showed a separation between the microbial community of hepatic GSD patients and the HCs (Figure 1B). As shown in Figure S1A,S1B and Figure 2A,2B, taxonomic profiles showed that the relative abundances of dominated phyla Firmicutes (P=0.030) and Bacteroidetes (P=0.029), families Lachnospiraceae (P=0.012), Ruminococcaceae (P=0.008), Peptostreptococcaceare (P=0.031), and Prevotellaceae (P=0.047), were decreased, whereas phyla Actinobacteria (P=0.033), Proteobacteria (P=0.049), families Bifidobacteriaceae (P=0.030), Lactobacillaceae (P=0.034), and Veillonellaceae (P=0.033), were increased in pediatric hepatic GSD patients as compared with the HCs. Inter-group comparisons identified significantly lower relative abundances of genera Blautia (P=0.017), Eubacterium_hallii_group (P=0.032), Faecalibacterium (P=0.017), Ruminococcus_troques_group (P=0.018), Dorea (P=0.002), Fusicatenibacter (P=0.013), Turicibacter (P=0.023), Prevotella (P=0.016), unclassified_f_Lachnospiraceae (P=0.001), and Anaerostipes (P=0.016), yet significantly higher abundances of genera Lactobacillus (P=0.011), Enterobater (P=0.034), Veillonella (P=0.014), Klebsiella (P=0.021), Megamonas (P=0.013), and Ruminococcus_gnavus_group (P=0.023) in hepatic GSD patients in comparison with the HCs (Figure S1C, Figure 2C).
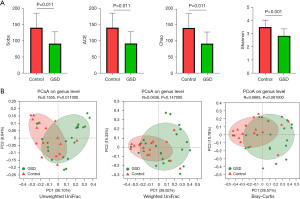
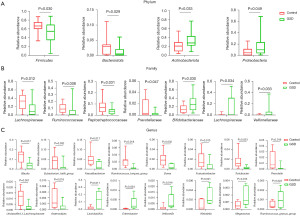
In order to investigate the relationship of UCCS treatment with gut microbiota composition in hepatic GSD patients, we divided the patients into three groups (<2 years, 2–5 years, >5 years) based on the duration of the UCCS intake. Microbial genera of relative abundance >0.1% were selected for the analysis. As shown in Figure 3, UCCS treatment (<2 years) was negatively associated with the relative abundance of genera Enterococcus (R=−0.35); UCCS intake within 2–5 years was positively associated with the abundance of Intestinibacter (R=0.51), whereas it was negatively associated with the relative abundance of genera Streptococcus (R=−0.32). In addition, long-term (>5 years) UCCS treatment was positively associated with the abundances of genera Terrisporobacter (R=0.66) and Sarcina (R=0.42) and negatively associated with the abundance of genera Megasphaera (R=−0.21).

Metabolomics changes in hepatic GSD children
We used a targeted metabolomics approach to determine fecal BA profiles of 47 BAs in both hepatic GSD children and HCs (Table S1). Both the PCoA (Figure 4A, P=0.002) and PLS-DA (Figure 4B, R=0.618) plots revealed significant differences in distribution patterns of BAs between the two groups. The relative abundances of primary BAs were increased in hepatic GSD children as compared to that of HCs (Figure 4C, P=0.009), and significantly for cholic acid (CA; P=0.021), 12-ketochenodeoxycholic acid (12-KCDCA; P=0.046), 3-dehydrocholic acid (3-DHCA; P=0.028), chenodeoxycholic acid-3-glucuronide (CDCA-3Gln; P=0.009), and glycochenodeoxycholic acid (GCDCA; P=0.038) in comparison with the HCs (Figure 4D). The decreased relative abundances of secondary BAs were significantly for lithocholic acid (LCA; P=0.004), deoxycholic acid (DCA; P=0.003), isolithocholic acid (isoLCA; P=0.002), 12-ketolithocholic acid (12-KLCA; P<0.001), dehydrolithocholic acid (DHLCA; P<0.001), glycodeoxycholic acid (GDCA; P<0.001), nordeoxycholic acid (NorDCA; P=0.003), glycolithocholic acid (GLCA; P=0.006), and taurolithocholic acid (TLCA; P=0.008) as compared to that of HCs (Figure 4E).
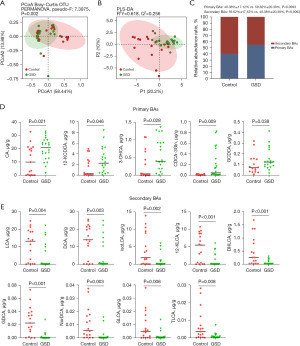
Furthermore, the levels of several major SCFAs were measured in the fecal samples collected from both hepatic GSD patients and HCs. As shown in Figure 5, the levels of propionic acid (P=0.001), valeric acid (P=0.011), isobutyric acid (P<0.001), and isovaleric acid (P<0.001) were significantly decreased in hepatic GSD children as compared to that of HCs. No statistical differences were observed in acetic acid, butanoic acid, isohexanoic acid, and hexanoic acid levels between the two groups.
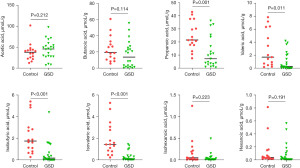
Covariance between GSD-related gut bacteria and metabolites
To explore the associations between the gut bacteria and metabolites in GSD children, we calculated the Spearman rank correlations coefficient for the 16 significantly altered bacteria genera, fecal BAs, and SCFAs. Bacteria genera that decreased in GSD children, including Blautia, Eubacterium_hallii_group, Dorea, Anaerostipes, Ruminococcus_troques_group, unclassified_f_Lachnospiraceae, and Turicibacter, were positively correlated with the BA levels of ursodeoxycholic acid (UDCA), DCA, GLCA, omega-MCA, LCA, CDCA, isoLCA, 12-KLCA, DHLCA, 3-beta-UDCA, UCA, 3-beta-CA, SCFAs of butyric acid, propionic acid, isobutyric acid, and valeric acid, and negatively correlated with BAs of CDCA-3Gln, CDCA-24A-beta-Glu, GCA, GCDCA, CA, GHCA, TCA, TCDCA, T-beta-MCA, AlloCA, T-omega-MCA, and 7-DHCA (Figure 6A). In contrast, bacteria genera that increased in GSD children, such as Lactobacillus was positively correlated with the BA levels of CDCA-3Gln, CDCA-24A-beta-Glu, and negatively correlated with the BA levels of 3-beta-CA, 3-beta-UDCA, DHLCA, murideoxycholic acid (MDCA), SCFAs of butyric acid, propionic acid, isobutyric acid, and valeric acid, respectively (Figure 6B). The correlation coefficients R and P values were provided in the online supporting data (see available online: https://cdn.amegroups.cn/static/public/tp-22-293-1.xlsx).
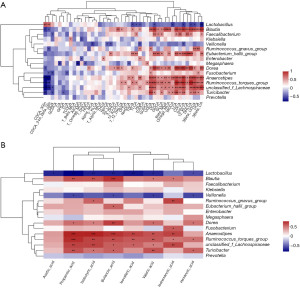
Discussion
The gut microbiota plays a critical role in host physiology and metabolism (29). Available evidences have demonstrated that the altered gut microbiota may contribute to the pathogenesis of human metabolic disorders, including obesity, type 2 diabetes, and metabolic liver diseases (30). For instance, the gut microbiota of NAFLD patients was characterized by increased relative abundances of Anaerobacter, Streptococcus, Lactobacillus, and Escherichia, whereas decreased abundances of Odoribacter, Flavonifaractor, Oscillibacter, and Alistipes spp. as compared to HCs (19). In non-alcoholic steatohepatitis (NASH) patients, the relative abundances of Proteobacteria, Enterobacteriaceae and Escherichia spp. were found to be increased (31). Recently, 2 studies showed that altered gut microbiota was also present in patients with hepatic GSD, suggesting the associations of gut microbiota and hepatic GSD (20,21).
In the current study, we firstly investigated the gut microbiota composition of hepatic GSD children in an Asian population. We demonstrated that the hepatic GSD-associated gut microbiota dysbiosis was characterized by decreases of both richness and biodiversity. The changes of bacteria taxa abundances, including deceased phyla Firmicutes and Bacteroidetes, families Lachnospiraceae, Ruminococcaceae, and Peptostreptococcaceare, genera Blautia, Romboutsia, Faecalibacterium, and increased phyla Actinobacteria, Proteobacteria, Fusobacteria, families Bifidobacteriaceae, Enterobacteriaceae, Lactobacillaceae, Veillonellaceae, and Clostridiaceae, genera Bifidobacterium, Escherichia-Shigella, and Lactobacillu were similar with previous reported data in GSD patients (20,21). Interestingly, an identical pattern of gut microbiota community changes has been observed in various liver metabolic diseases, such as increased Proteobacteria, Enterobacteriaceae, Lactobacillus, Escherichia, and decreased Firmicutes, Faecalibacterium (19,31), which further indicates the strong associations between gut microbiota and host liver metabolism. For instance, genera Prevotella that was decreased in hepatic GSD children from our data, was reported to be associated with dietary fiber-induced improvement in host glucose metabolism (32).
The gut microbiota may be influenced by diet therapy in hepatic GSD patients. A previous study showed that gut microbial richness was negatively correlated with total carbohydrate but positively with sugar intake in hepatic GSD patients (20). In addition, Ceccarani et al. reported that fiber intake was correlated positively with genera Odoribacter and Parabacteroides, and that starch intake positively correlated with genera Veillonella, Citrobacter and Akkermansia yet negatively correlated with genera Coprococcus and Clostridium in GSD patients (21). The diet therapies of the enrolled hepatic GSD patients included cornstarch therapy and dietary restrictions according to the guidelines (4,5). Foods containing high sucrose (fructose and glucose) and lactose (galactose and glucose), organ meats, and fatty and processed meats are avoided. Starchy food, including brown and white rice, Chinese yam, broad bean, and white potatoes are the major sources of the carbohydrates, and lean poultry, beef, pork, fish are the major sources of protein and fat. All participants were routinely treated with UCCS after the diagnosis of GSD. We showed that long-term (>5 years) UCCS treatment was positively associated with the relative abundances of genera Terrisporobacter and Sarcina, whereas it was negatively associated with the relative abundances of genera Megasphaera. Nevertheless, future studies are needed to further explore the correlation of diet therapy and gut microbiota in GSD patients.
In addition to facilitating the digestion of food and the absorption of dietary lipids and fat-soluble vitamins in the gut, BAs have multiple endocrine functions as regulation of hepatic glucose and lipid metabolism (33). As pivotal signaling molecules, BAs bind to the nuclear hormone FXR and TGR5 in multiple organs that regulate intestinal incretin secretion, hepatic gluconeogenesis, and glycogen synthesis (11). Alterations of the metabolism of BAs are implicated in various metabolic diseases (11). We showed that the BAs metabolism was changed by increasing abundances of primary BAs (significantly for CA, 12-KCDCA, 3-DHCA, CDCA-3Gln, and GCDCA) in hepatic GSD children as compared to healthy children. Since primary BAs are efficacious endogenous agonists of FXR in the liver (34), the increased primary BAs ratio in the BAs pool may lead to an over-activation of FXR signaling. However, the exact role of FXR in the regulation of glucose metabolism is still controversial. TGR5 is activated by the secondary BAs TLCA and DCA (34). It has been reported that TGR5 activation can protect against the liver injury caused by BAs overload during liver regeneration (35). The decreased levels of TLCA and DCA may be involved in liver damage through impairing the TGR5 signaling in hepatic GSD patients. Nevertheless, the effect of altered BAs metabolism and its mechanisms in hepatic GSD are largely unknown. In addition, it has been reported that SCFAs have a potential regulatory role in glucose homeostasis (10). Several major SCFAs, including acetic acid, propionic acid, and butanoic acid, regulate hepatic glucose homeostasis through PPAR-γ signaling-mediated effects on gluconeogenesis (36). It has also been shown that butyrate induces the expression of gluconeogenic genes involved in the action of propionate in intestinal gluconeogenesis regulation (37). In addition, butyrate can improve liver glycogen metabolism in type 2 diabetes model mice (38). Our data revealed that the levels of butanoic acid and propionic acid were significantly decreased in hepatic GSD children, suggesting that the changes of SCFAs may play a role in the hepatic glucose metabolism in hepatic GSD.
Since the liver health, BAs metabolism, SCFAs, and gut microbiota composition are strongly correlated, we further explored the covariance between the altered bacteria genera, fecal BAs, and SCFAs in hepatic GSD children. We found that several bacteria genera that were decreased in GSD children were correlated with the deceasing levels of secondary BAs, including DCA, LCA, and isoLCA, as well as butyric acid, propionic acid, isobutyric acid, and valeric acid. However, the complex interactions of gut microbiota, microbial metabolites, and liver glucose metabolism still need to be investigated in the future.
The current study had several limitations. First, this was a single-center study with a small cohort. Studies with large number of GSD patients are further needed. Second, we only evaluated the gut microbiota by 16S rRNA sequencing. Third, gut microbiota and metabolites were determined only in a single fecal sample. Longitudinal studies dedicated to the dynamics of the microbiota and metabolome are needed to understand the microbiota and metabolome in GSD. Fourth, the criteria of dividing the patients into three groups based on the sample size appropriate for the statistical analysis may cause bias in the investigation of the association of UCCS intake duration with gut microbiota composition. The relationship of UCCS treatment with gut microbiota composition in hepatic GSD patients is needed to be further validated in large cohorts. Considering a potential concern that some or most of these metabolic/microbiota changes and their correlation may be merely an outcome of UCCS diet in GSD patients, it is important to conduct studies to explore the correlation of metabolic/microbiota changes between untreated GSD patients and healthy individuals in the future. Finally, the driver of the microbiota and metabolome changes mediated by either the genetic defect, disease status, or diets in GSD was undetermined in this study. Due to the limited number of enrolled patients, we did not divide them into poor metabolic control vs. optimal metabolic control groups. Since the HCs did not consume UCCS and did not undergo dietary restriction, studies comparing within the GSD groups rather than comparing with the general public are warranted in the future.
Conclusions
In summary, hepatic GSD children are presented with a significant gut microbiota dysbiosis correlated with altered BAs metabolism, as well as changes of fecal SCFAs concentrations. Despite the undetermined driver of these changes mediated by either the genetic defect, disease status, or diet (e.g., UCSS), this study may shed light on the exploration of microbial-targeted therapies, such as antibiotics, pre/probiotics, polyphenols, and fecal microbiome transplantation (FMT), in the management of hepatic GSD.
Acknowledgments
Funding: This work was supported by the grants from the National Natural Science Foundation of China (Nos. 81870373 and 81900472), and the Natural Science Foundation of Shanghai (No. 22ZR1451800).
Footnote
Reporting Checklist: The authors have completed the STROBE reporting checklist. Available at https://tp.amegroups.com/article/view/10.21037/tp-22-293/rc
Data Sharing Statement: Available at https://tp.amegroups.com/article/view/10.21037/tp-22-293/dss
Conflicts of Interest: All authors have completed the ICMJE uniform disclosure form (available at https://tp.amegroups.com/article/view/10.21037/tp-22-293/coif). The authors have no conflicts of interest to declare.
Ethical Statement: The authors are accountable for all aspects of the work in ensuring that questions related to the accuracy or integrity of any part of the work are appropriately investigated and resolved. The study was conducted in accordance with the Declaration of Helsinki (as revised in 2013). The study was approved by the Ethical Review Board of Shanghai Children’s Hospital (No. 2021R119-E01), and informed consent was taken from the parent or legal guardian of all the participants.
Open Access Statement: This is an Open Access article distributed in accordance with the Creative Commons Attribution-NonCommercial-NoDerivs 4.0 International License (CC BY-NC-ND 4.0), which permits the non-commercial replication and distribution of the article with the strict proviso that no changes or edits are made and the original work is properly cited (including links to both the formal publication through the relevant DOI and the license). See: https://creativecommons.org/licenses/by-nc-nd/4.0/.
References
- Kanungo S, Wells K, Tribett T, et al. Glycogen metabolism and glycogen storage disorders. Ann Transl Med 2018;6:474. [Crossref] [PubMed]
- Vega AI, Medrano C, Navarrete R, et al. Molecular diagnosis of glycogen storage disease and disorders with overlapping clinical symptoms by massive parallel sequencing. Genet Med 2016;18:1037-43. [Crossref] [PubMed]
- Hicks J, Wartchow E, Mierau G. Glycogen storage diseases: a brief review and update on clinical features, genetic abnormalities, pathologic features, and treatment. Ultrastruct Pathol 2011;35:183-96. [Crossref] [PubMed]
- Ross KM, Ferrecchia IA, Dahlberg KR, et al. Dietary Management of the Glycogen Storage Diseases: Evolution of Treatment and Ongoing Controversies. Adv Nutr 2020;11:439-46. [Crossref] [PubMed]
- Kishnani PS, Austin SL, Abdenur JE, et al. Diagnosis and management of glycogen storage disease type I: a practice guideline of the American College of Medical Genetics and Genomics. Genet Med 2014;16:e1. [Crossref] [PubMed]
- Kishnani PS, Austin SL, Arn P, et al. Glycogen storage disease type III diagnosis and management guidelines. Genet Med 2010;12:446-63. [Crossref] [PubMed]
- Kishnani PS, Goldstein J, Austin SL, et al. Diagnosis and management of glycogen storage diseases type VI and IX: a clinical practice resource of the American College of Medical Genetics and Genomics (ACMG). Genet Med 2019;21:772-89. [Crossref] [PubMed]
- Structure, function and diversity of the healthy human microbiome. Nature 2012;486:207-14. [Crossref] [PubMed]
- Sayin SI, Wahlström A, Felin J, et al. Gut microbiota regulates bile acid metabolism by reducing the levels of tauro-beta-muricholic acid, a naturally occurring FXR antagonist. Cell Metab 2013;17:225-35. [Crossref] [PubMed]
- Morrison DJ, Preston T. Formation of short chain fatty acids by the gut microbiota and their impact on human metabolism. Gut Microbes 2016;7:189-200. [Crossref] [PubMed]
- Shapiro H, Kolodziejczyk AA, Halstuch D, et al. Bile acids in glucose metabolism in health and disease. J Exp Med 2018;215:383-96. [Crossref] [PubMed]
- den Besten G, van Eunen K, Groen AK, et al. The role of short-chain fatty acids in the interplay between diet, gut microbiota, and host energy metabolism. J Lipid Res 2013;54:2325-40. [Crossref] [PubMed]
- Marchesi JR, Adams DH, Fava F, et al. The gut microbiota and host health: a new clinical frontier. Gut 2016;65:330-9. [Crossref] [PubMed]
- Geng J, Ni Q, Sun W, et al. The links between gut microbiota and obesity and obesity related diseases. Biomed Pharmacother 2022;147:112678. [Crossref] [PubMed]
- Thingholm LB, Rühlemann MC, Koch M, et al. Obese Individuals with and without Type 2 Diabetes Show Different Gut Microbial Functional Capacity and Composition. Cell Host Microbe 2019;26:252-64.e10. [Crossref] [PubMed]
- Million M, Maraninchi M, Henry M, et al. Obesity-associated gut microbiota is enriched in Lactobacillus reuteri and depleted in Bifidobacterium animalis and Methanobrevibacter smithii. Int J Obes (Lond) 2012;36:817-25. [Crossref] [PubMed]
- Le Chatelier E, Nielsen T, Qin J, et al. Richness of human gut microbiome correlates with metabolic markers. Nature 2013;500:541-6. [Crossref] [PubMed]
- Utzschneider KM, Kratz M, Damman CJ, et al. Mechanisms Linking the Gut Microbiome and Glucose Metabolism. J Clin Endocrinol Metab 2016;101:1445-54. [Crossref] [PubMed]
- Jiang W, Wu N, Wang X, et al. Dysbiosis gut microbiota associated with inflammation and impaired mucosal immune function in intestine of humans with non-alcoholic fatty liver disease. Sci Rep 2015;5:8096. [Crossref] [PubMed]
- Colonetti K, Bento Dos Santos B, Nalin T, et al. Hepatic glycogen storage diseases are associated to microbial dysbiosis. PLoS One 2019;14:e0214582. [Crossref] [PubMed]
- Ceccarani C, Bassanini G, Montanari C, et al. Proteobacteria Overgrowth and Butyrate-Producing Taxa Depletion in the Gut Microbiota of Glycogen Storage Disease Type 1 Patients. Metabolites 2020;10:133. [Crossref] [PubMed]
- Liang Y, Du C, Wei H, et al. Genotypic and clinical analysis of 49 Chinese children with hepatic glycogen storage diseases. Mol Genet Genomic Med 2020;8:e1444. [Crossref] [PubMed]
- Magoč T, Salzberg SL. FLASH: fast length adjustment of short reads to improve genome assemblies. Bioinformatics 2011;27:2957-63. [Crossref] [PubMed]
- Chen S, Zhou Y, Chen Y, et al. fastp: an ultra-fast all-in-one FASTQ preprocessor. Bioinformatics 2018;34:i884-90. [Crossref] [PubMed]
- Callahan BJ, McMurdie PJ, Rosen MJ, et al. DADA2: High-resolution sample inference from Illumina amplicon data. Nat Methods 2016;13:581-3. [Crossref] [PubMed]
- Bolyen E, Rideout JR, Dillon MR, et al. Reproducible, interactive, scalable and extensible microbiome data science using QIIME 2. Nat Biotechnol 2019;37:852-7. [Crossref] [PubMed]
- Tian Y, Cai J, Allman EL, et al. Quantitative Analysis of Bile Acid with UHPLC-MS/MS. Methods Mol Biol 2021;2194:291-300. [Crossref] [PubMed]
- Zhang S, Wang H, Zhu MJ. A sensitive GC/MS detection method for analyzing microbial metabolites short chain fatty acids in fecal and serum samples. Talanta 2019;196:249-54. [Crossref] [PubMed]
- Tremaroli V, Bäckhed F. Functional interactions between the gut microbiota and host metabolism. Nature 2012;489:242-9. [Crossref] [PubMed]
- Fan Y, Pedersen O. Gut microbiota in human metabolic health and disease. Nat Rev Microbiol 2021;19:55-71. [Crossref] [PubMed]
- Zhu L, Baker SS, Gill C, et al. Characterization of gut microbiomes in nonalcoholic steatohepatitis (NASH) patients: a connection between endogenous alcohol and NASH. Hepatology 2013;57:601-9. [Crossref] [PubMed]
- Kovatcheva-Datchary P, Nilsson A, Akrami R, et al. Dietary Fiber-Induced Improvement in Glucose Metabolism Is Associated with Increased Abundance of Prevotella. Cell Metab 2015;22:971-82. [Crossref] [PubMed]
- de Aguiar Vallim TQ, Tarling EJ, Edwards PA. Pleiotropic roles of bile acids in metabolism. Cell Metab 2013;17:657-69. [Crossref] [PubMed]
- Chiang JYL, Ferrell JM. Bile Acid Metabolism in Liver Pathobiology. Gene Expr 2018;18:71-87. [Crossref] [PubMed]
- Péan N, Doignon I, Garcin I, et al. The receptor TGR5 protects the liver from bile acid overload during liver regeneration in mice. Hepatology 2013;58:1451-60. [Crossref] [PubMed]
- den Besten G, Bleeker A, Gerding A, et al. Short-Chain Fatty Acids Protect Against High-Fat Diet-Induced Obesity via a PPARγ-Dependent Switch From Lipogenesis to Fat Oxidation. Diabetes 2015;64:2398-408. [Crossref] [PubMed]
- Soty M, Penhoat A, Amigo-Correig M, et al. A gut-brain neural circuit controlled by intestinal gluconeogenesis is crucial in metabolic health. Mol Metab 2015;4:106-17. [Crossref] [PubMed]
- Zhang WQ, Zhao TT, Gui DK, et al. Sodium Butyrate Improves Liver Glycogen Metabolism in Type 2 Diabetes Mellitus. J Agric Food Chem 2019;67:7694-705. [Crossref] [PubMed]
(English Language Editor: J. Jones)