Association between incubator standards and newborn nosocomial infection with machine-learning prediction
Highlight box
Key findings
• Health and safety standards for incubators play an important protective role in the control of newborn infections, and an early gestational age and incubator standards may be risk factors for nosocomial infections in newborns.
What is known and what is new?
• Incubators provide a comfortable environment with a suitable temperature and humidity for newborns, which effectively reduces the probability of newborns being infected by the external environment in the first few weeks after birth and enhances the immune resistance of newborns.
• We analyzed different the incubator standards and other risk factors associated with newborn nosocomial infection.
What is the implication, and what should change now?
• Our findings might help clinicians improve the health and safety standards for incubators, especially those used for early gestational age newborns. The extreme gradient boosting algorithm can be used to predict nosocomial infections in newborns.
Introduction
The nursing practices of medical workers are closely related to infection control in hospitals (1,2). Infection control is particularly important in neonatal pediatrics. Newborns need to be placed in a temperature-controlled environment, as the physiological functions of newborns are not fully developed, especially the thermoregulatory center, which develops gradually after birth (3,4). Neonates, especially preterm infants, are very prone to scleredema or dysplasia (5). The skin stratum corneum of newborn infants is thin and rich in blood vessels, which are easily damaged and infected (5,6). The barrier function of their skin can also be damaged (6). If proper care is not taken, it is easy to cause sepsis and cellulitis.
Incubators provide a comfortable environment with a suitable temperature and humidity for newborns, which can effectively reduce the probability of newborns being infected by the external environment in the first few weeks and enhance the immune resistance of newborns (7,8). Additionally, the “neutral temperature” provided by incubators means that newborns can maintain a normal body temperature, and a comfortable and appropriate environmental temperature and humidity protects the skin (9,10). At the appropriate temperature, the body consumes the least amount of oxygen, the metabolism is appropriate, and the external evaporation and heat dissipation are small (11-13). Thus, special attention must be paid to the health and safety standards for incubators. The management of incubators has an important effect on the prognosis of newborns. Thus, hospital management personnel need to work diligently, learn the causes and methods of hospital infection, understand the equipment structure of incubators, avoid potential risks, and constantly update their knowledge (14,15). Due to the high incidence, nosocomial infections (NIs) in newborns need to be correctly controlled to avoid the occurrence of neonatal infection events.
To date, research on the relationship between the health standards for incubators and newborn NIs has been limited. Thus, this study analyzed the standardized and reasonable prevention measures and health and safety standards for incubators, and explored risk factors for the new born NI. We present the following article in accordance with the STARD reporting checklist (available at https://tp.amegroups.com/article/view/10.21037/tp-23-171/rc).
Methods
Data set
In this retrospective research, we collected the basic and incubator information of 76 infants at the Heping Hospital Affiliated to Changzhi Medical College from January 2021 to January 2022. Among the 76 infants, 36 were infected during hospitalization, and the other 40 patients were not infected. The following basic information for the patients was collected: gestational age (weeks), gender, weight (g), incubator, paternal age (years), and maternal age (years). The sample size was calculated based on the prevalence of new born NI. We obtained the written informed consent from all patients’ legal guardian, and this study was approved by the ethics committee of Heping Hospital Affiliated to Changzhi Medical College (No. 356984125). The study was conducted in accordance with the Declaration of Helsinki (as revised in 2013).
Evaluation of new standards for incubators
An evaluation system of the health and safety standards was formulated for the newborn incubators, and the corresponding nursing practices for preventing infection were measured. A number of specific measures were employed. First, a hospital infection management team responsible for the health and safety management of the newborn incubators was established, and conscientious and responsible nurses who were responsible for the implementation of various strategies were selected. Second, a sound health and safety standard evaluation system for the incubators was established, the responsibility consciousness of the nursing staff was improved, and regular training and assessments of hospital infection-related knowledge were carried out. We also unified the clinical measures, improved the technical level of the nursing staff, and ensured the standardization of various operations. For example, the medical personnel had to wear soft and clean sanitary clothes, masks, and hats when in contact with the newborns. The time for which a mask could be worn could not exceed 4 h (16,17). Hands had to be washed before and after coming into contact with a newborn, and the relevant regulations strictly followed. Spot-check microbiological examinations of the hands of medical personnel were conducted every month to prevent hospital infection. Corresponding data record procedures were established to record the microbiological incubator tests, residual concentration of the disinfectant, and ultraviolet disinfection, and the factors relevant to NIs in children were analyzed. Test were conducted to ensure the bacteria culture quantity in the water tanks of the incubators and the water injection ports were ≤5 cuf/cm2. No pathogenic bacteria should be found in the incubators (18,19). Among the included infants, the new incubator standard was randomly introduced and there was no overlap between the conventional and the new standard patients. The infants without complete records were excluded.
Diagnosis of NI
The following measures were used for NI diagnosis: (I) evaluating the symptoms and indicators of an illness; (II) check for redness, swelling, pus, or the presence of an abscess at wounds and catheter entry points; (III) a complete physical examination and evaluation for any underlying diseases; (IV) laboratory examinations such as a complete blood count (CBC), which looks for an increase in white blood cells that fight infections, a urinalysis, which checks for white blood cells or other signs of blood in the urinary tract, and cultures of the infected area, blood, sputum, urine, or other body fluids or tissue to find the etiological organism.
Statistical analysis
Chi-square tests and t-tests were employed to examine variations in the demographic and socioeconomic variables between the 2 groups while evaluating the baseline data. The parameters connected to the patients’ ability to live with NIs were then found using Pearson correlation matrices. After that, we used a logistic regression to find the important risk variables connected to patients’ living conditions who had NIs and estimated odds ratios. (ORs). All the analyses were performed using R 4.1.2 software (American Statistical Computing R Foundation, Vienna, Austria). A bilateral P value <0.05 was considered statistically significant.
Logistic regression
A logistic regression is a supervised-learning algorithm for predicting relevant categorical target variables. If you have a large amount of data to classify, a logistic regression may be helpful (20). The principle of logistic regressions has been summarized as follows (21): binary logistic regressions estimate the likelihood that a characteristic of a binary variable is present, given the values of the covariates.
XGBoost
XGBoost is a distributed gradient boosting library that has been developed to be effective, adaptable, and portable. It uses the gradient boosting framework to construct machine learning algorithms. It offers a parallel tree augmentation to quickly and accurately address a variety of data science tasks. It has become popular recently, and due to its scalability, it dominates the applied machine-learning and Kaggle structured data competition. In the machine learning algorithms, we put infection status as the outcome variable, and all other covariates as the input variables.
Results
The sample of premature infants comprised an infected group with a mean [standard deviation (SD)] age of 32.4±4.71 weeks, and a non-infection group with a mean (SD) age of 35.8±3.75 weeks. In the infected group, 52.8% (n=19) of the patients were male, and in the non-infected group, 62.5% (n=25) were male. In the infected group, most infants weighed <3,000 g (2,656±1,021 g), and in the non-infected group most infants weighed >3,000 g (3,000±625 g). In the non-infected group, 87.5% (n=35) of the infants had a new standard incubator and in the infected group, 77.8% (n=28) had a conventional incubator. The paternal age of the infected group was 43.1±12.3 years and that of the non-infected group was 32.1±8.0 years. The maternal age of the infected group was 40.6±11.4 years and that of the non-infected group was 30.7±8.37 years (Table 1). In the differences analysis of the baseline data, gestational age, incubator type, paternal age and maternal age differed significantly among the 2 groups.
Table 1
Variables | Not infected (N=40) | Infected (N=36) | P value for T or chi-square test |
---|---|---|---|
Gestational age (weeks), mean ± SD | 35.8±3.75 | 32.4±4.71 | 0.001 |
Gender, n (%) | 0.532 | ||
Female | 15 (37.5) | 17 (47.2) | |
Male | 25 (62.5) | 19 (52.8) | |
Weight (g), mean ± SD | 3,000±625 | 2,656±1,021 | 0.086 |
Incubator, n (%) | <0.001 | ||
Conventional | 5 (12.5) | 28 (77.8) | |
New standard | 35 (87.5) | 8 (22.2) | |
Paternal age (years), mean ± SD | 32.1±8.00 | 43.1±12.3 | <0.001 |
Maternal age (years), mean ± SD | 30.7±8.37 | 40.6±11.4 | <0.001 |
SD, standard deviation.
After an exploratory analysis, the correlations of the 6 variables were analyzed by a Pearson correlation matrix for the further logistic regression (Figure 1). According to the matrix, only the paternal age and maternal age were correlated. The exploratory factor analysis confirmed a limited number of relevant covariates, and thus provided a good basis for the logistic regression.
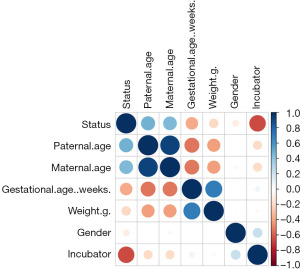
To further analyze the risk levels of the 6 factors, we conducted the logistic regression (Table 2) and found that infant infection was correlated with gestational age (β=−0.25394, P=0.045) and incubator type (β=−4.45336, P=0.0000223). These 2 factors were negatively correlated with infant infection.
Table 2
Variables | β | Standard error | Z value | P value |
---|---|---|---|---|
Gestational age (weeks) | –0.25394 | 0.132894 | –1.911 | 0.045* |
Male | 0.163905 | 0.816634 | 0.201 | 0.841 |
Weight (g) | 0.000507 | 0.00069 | 0.734 | 0.463 |
New standard incubator | –4.45336 | 1.050102 | –4.241 | 0.0000223* |
Paternal age (years) | 0.091865 | 0.078609 | 1.169 | 0.243 |
Maternal age (years) | 0.030938 | 0.091931 | 0.337 | 0.736 |
*, P<0.05.
Further, the logistic model OR results (Table 3) showed that gestational age [OR =0.77574, 95% confidence interval (CI): 0.583513–0.996354] and the new standard incubator (OR =0.011639, 95% CI: 0.000958–0.067897) may be protective factors for infant infections during hospitalization.
Table 3
Variables | OR | 95% CI |
---|---|---|
Gestational age (weeks) | 0.77574 | 0.583513–0.996354 |
Male | 1.178102 | 0.238722–6.339745 |
Weight (g) | 1.000507 | 0.999163–1.001942 |
New standard incubator | 0.011639 | 0.000958–0.067897 |
Paternal age (years) | 1.096217 | 0.942389–1.289826 |
Maternal age (years) | 1.031421 | 0.861315–1.244907 |
OR, odds ratio; 95% CI, 95% confidential interval.
To better prevent NI in newborns, we conducted a prediction using machine-learning algorithms, including Extreme gradient boosting (XGBoost), random forest (RF), support vector machine (SVM) and decision tree (DT). To determine which algorithm was best able to predict newborn NI, we compared the accuracy, kappa value, receiver operator characteristic (ROC) curve, and precision recall gain (PRG) curve. As Figure 2 shows, we found that XGBoost had the highest value in terms of its accuracy (0.92) and kappa value (0.84) among the 4 machine-learning algorithms.
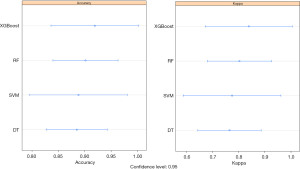
To further comprehensively evaluate the sensitivity and specificity of the XGBoost model, the ROC curve was adapted, and the area under the curve (AUC) of XGBoost had the highest value (0.93) (Figure 3).
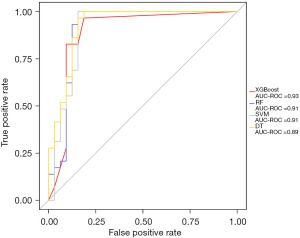
To examine the sensitivity and precision of the XGBoost model, the PRG curve was used and XGBoost had the best performance (AUC =0.86). All the results proved that XGBoost had the best prediction performance in terms of accuracy, sensitivity, specificity, and precision. Thus, we used XGBoost to predict NIs in newborns (Figure 4).
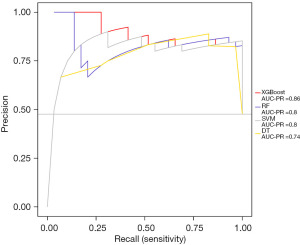
Discussion
In our research, the baseline analysis showed that gestational age, incubator type, paternal age, and maternal age differed significantly between the 2 groups. These factors might influence infection outcomes in infants. After the exploratory factor analysis that confirmed the limited correlated covariant, the included variables had a limited basis for logistic regression. The logistic regression showed that infant infection was negatively related with gestational age (β=−0.25394, P=0.045) and incubator type (β=−4.45336, P=0.0000223). Further, gestational age had an OR of 0.77574 (95% CI: 0.583513–0.996354) and the new standard incubator had an OR of 0.011639 (95% CI: 0.000958–0.067897). These 2 factors might be protective factors for infant infections during hospitalization.
The immune system of a newborn is immature. The phagocytosis of the reticuloendothelial system and leukocytes are weak. The serum complement is lower than that of an adult, as is the ability of leukocytes to kill fungi. The levels of immunoglobulin G and complement of the premature infant are lower than those of a full-term infant, and it is very easy to develop various infections (22). As newborns grow, the risk of infection decreases, which is consistent with our findings.
Gray reported that through the establishment of the corresponding evaluation standards, neonatology departments can strengthen the health and safety management of incubators. Controlling neonatal infections within a reasonable range also helps to improve the service quality of hospitals. Establishing a scientific evaluation system of health and safety standards for newborn incubators and formulating scientific intervention measures to prevent infection should improve the effect of NI control (23). This conclusion is consistent with our results.
Among the XGBoost, RF, SVM, and DT algorithms, XGBoost had the best performance in terms of accuracy, sensitivity, specificity, and precision. This appears to be the first study to examine the use of machine-learning algorithms in predicting newborn NI, and we found that XGBoost is a good prediction algorithm.
Conclusions
In conclusion, health and safety standards for incubators play an important protective role in newborn infection control, and an early gestational age and incubator standards may be risk factors for NIs in newborns. XGBoost can be used to predict NI in newborns. However, our study had some limitations. First, this study adopted a case-control design, rather than a cohort design, which may not be used for inferring causality. Second, the study sample size was limited, and the study was not conducted at multiple centers.
Acknowledgments
Funding: None.
Footnote
Reporting Checklist: The authors have completed the STARD reporting checklist. Available at https://tp.amegroups.com/article/view/10.21037/tp-23-171/rc
Data Sharing Statement: Available at https://tp.amegroups.com/article/view/10.21037/tp-23-171/dss
Peer Review File: Available at https://tp.amegroups.com/article/view/10.21037/tp-23-171/prf
Conflicts of Interest: All authors have completed the ICMJE uniform disclosure form (available at https://tp.amegroups.com/article/view/10.21037/tp-23-171/coif). The authors have no conflicts of interest to declare.
Ethical Statement: The authors are accountable for all aspects of the work in ensuring that questions related to the accuracy or integrity of any part of the work are appropriately investigated and resolved. The study was conducted in accordance with the Declaration of Helsinki (as revised in 2013). The study was approved by the ethics committee of Heping Hospital Affiliated to Changzhi Medical College (No. 356984125) and informed consent was taken from all the patients’ legal guardian.
Open Access Statement: This is an Open Access article distributed in accordance with the Creative Commons Attribution-NonCommercial-NoDerivs 4.0 International License (CC BY-NC-ND 4.0), which permits the non-commercial replication and distribution of the article with the strict proviso that no changes or edits are made and the original work is properly cited (including links to both the formal publication through the relevant DOI and the license). See: https://creativecommons.org/licenses/by-nc-nd/4.0/.
References
- Denkel LA, Schwab F, Clausmeyer J, et al. Effect of antiseptic bathing with chlorhexidine or octenidine on central line-associated bloodstream infections in intensive care patients: a cluster-randomized controlled trial. Clin Microbiol Infect 2022;28:825-31. [Crossref] [PubMed]
- Jacquot A, Labaune JM, Baum TP, et al. Rapid quantitative procalcitonin measurement to diagnose nosocomial infections in newborn infants. Arch Dis Child Fetal Neonatal Ed 2009;94:F345-8. [Crossref] [PubMed]
- Vaswani ND, Gupta N, Yadav R, et al. Seven versus Ten Days Antibiotics Course for Acute Pyogenic Meningitis in Children: A Randomized Controlled Trial. Indian J Pediatr 2021;88:246-51. [Crossref] [PubMed]
- Xia F, Li Q, Luo X, et al. Identification for heavy metals exposure on osteoarthritis among aging people and Machine learning for prediction: A study based on NHANES 2011-2020. Front Public Health 2022;10:906774. [Crossref] [PubMed]
- Xia F, Li Q, Luo X, et al. Machine learning model for depression based on heavy metals among aging people: A study with National Health and Nutrition Examination Survey 2017-2018. Front Public Health 2022;10:939758. [Crossref] [PubMed]
- Carneiro M, Adam MS, Machado JA, et al. The importance of infection prevention bundles related to construction/renovations in hospital environment. Am J Infect Control 2012;40:578-9. [Crossref] [PubMed]
- Ariffin N, Hasan H, Ramli N, et al. Comparison of antimicrobial resistance in neonatal and adult intensive care units in a tertiary teaching hospital. Am J Infect Control 2012;40:572-5. [Crossref] [PubMed]
- Xia F, Li Q, Luo X, et al. Association between urinary metals and leukocyte telomere length involving an artificial neural network prediction: Findings based on NHANES 1999-2002. Front Public Health 2022;10:963138. [Crossref] [PubMed]
- Chakraborty M, McGreal EP, Kotecha S. Acute lung injury in preterm newborn infants: mechanisms and management. Paediatr Respir Rev 2010;11:162-70; quiz 170. [Crossref] [PubMed]
- Cernada M, Aguar M, Brugada M, et al. Ventilator-associated pneumonia in newborn infants diagnosed with an invasive bronchoalveolar lavage technique: a prospective observational study. Pediatr Crit Care Med 2013;14:55-61. [Crossref] [PubMed]
- Thakkar RK, Penatzer J, Simon S, et al. Measures of Adaptive Immune Function Predict the Risk of Nosocomial Infection in Pediatric Burn Patients. J Burn Care Res 2022;43:1416-25. [Crossref] [PubMed]
- Wang K, Xia F, Li Q, et al. The Associations of Weekend Warrior Activity Patterns With the Visceral Adiposity Index in US Adults: Repeated Cross-sectional Study. JMIR Public Health Surveill 2023;9:e41973. [Crossref] [PubMed]
- Samane S, Yadollah ZP, Marzieh H, et al. Cue-based feeding and short-term health outcomes of premature infants in newborn intensive care units: a non-randomized trial. BMC Pediatr 2022;22:23. [Crossref] [PubMed]
- Buettcher M, Heininger U. Prospective surveillance of nosocomial viral infections during and after hospitalization at a university children's hospital. Pediatr Infect Dis J 2010;29:950-6. [Crossref] [PubMed]
- Zheng X, Shi J, Wu J. Analysis of factors and corresponding interactions influencing clinical management assistant ability using competency model in China. Medicine (Baltimore) 2020;99:e23516. [Crossref] [PubMed]
- Kelly KB, Banerjee A, Golob JF, et al. Where's the difference? Presentation of nosocomial infection in critically ill trauma versus general surgery patients. Surg Infect (Larchmt) 2014;15:377-81. [Crossref] [PubMed]
- Hosty J, Cheema A, Magdum AA. Necrotising fasciitis: clinical judgement and early multidisciplinary collaboration is the key. Age Ageing 2022;51:afab197. [Crossref] [PubMed]
- Jeong IS, Jeong JS, Choi EO. Nosocomial infection in a newborn intensive care unit (NICU), South Korea. BMC Infect Dis 2006;6:103. [Crossref] [PubMed]
- Webster J, Pritchard MA. Gowning by attendants and visitors in newborn nurseries for prevention of neonatal morbidity and mortality. Cochrane Database Syst Rev 2003;2003:CD003670. [Crossref] [PubMed]
- Shibahara M, Shibata E, Kinjo Y, et al. Impact of the Induction of Labor on Hemophilia Carriers and Their Newborn Infants. J Med Cases 2021;12:5-8. [Crossref] [PubMed]
- Joshi RD, Dhakal CK. Predicting Type 2 Diabetes Using Logistic Regression and Machine Learning Approaches. Int J Environ Res Public Health 2021;18:7346. [Crossref] [PubMed]
- Teng RJ, Wu TJ, Garrison RD, et al. Early neutropenia is not associated with an increased rate of nosocomial infection in very low-birth-weight infants. J Perinatol 2009;29:219-24. [Crossref] [PubMed]
- Gray PH, Flenady V. Cot-nursing versus incubator care for preterm infants. Cochrane Database Syst Rev 2011;CD003062. [Crossref] [PubMed]
(English Language Editor: L. Huleatt)