Identification of epidermal growth factor receptor as an immune-related biomarker in epilepsy using multi-transcriptome data
Highlight box
Key findings
• The pathophysiology of epilepsy was correlated with EGFR, and thus, EGFR could be a novel biomarker of juvenile focal epilepsies. This finding provides promising therapeutic targets for epilepsy.
What is known and what is new?
• Recent studies have indicated that an interrelationship between immunity and inflammatory processes and epilepsy. However, the immune-related mechanisms of epilepsy are still not precisely understood.
• This study aimed to explore immune-related mechanisms in epilepsy disorders, highlight the role of immune cells at the molecular level in epilepsy, and provide therapeutic targets for patients with epilepsy. In this study, we used bioinformatics to discover that EGFR could be a novel biomarker of juvenile focal epilepsies.
What are the implications, and what should change now?
• If we can confirm that EGFR could be a novel biomarker of juvenile focal epilepsies, EGFR will provide a new therapeutic direction for the clinical treatment of epilepsy.
Introduction
During the last decades, due to the increasing use of MRI in epilepsy, cortical malformations have been “reconsidered” as one of the most frequent etiologies of focal epileptic seizures in both pediatric and young adults population (1). Children with lesional epilepsy are more likely to have focal cortical dysplasia (FCD), which is a prominent cause of refractory epilepsy (2-4). The primary neuropathological alteration in epilepsy is neuronal injury; status epilepticus damages the nervous system and hippocampal neurons both chronically and suddenly, resulting in hypoxia, ischemia, inflammation, and edema (5-10). Studies on the pathophysiology of epilepsy reveal that inflammatory cell infiltration and molecular regulatory mechanisms in injured neuronal tissues are crucial elements in epileptogenesis (11,12); additionally, studies have also shown that the beginning of seizures and epileptogenesis are mediated by inflammatory mediators secreted by peripheral immune cells and brain cell. Immune response and inflammatory mediators contribute to epileptogenesis while reducing the seizure threshold in individuals (13,14). Currently, several studies have revealed the immune-related biomarkers in epilepsy (15,16). These studies have explored the expression of these molecules in epilepsy, but lack in-depth exploration of immunity.
The expression of inhibitory receptors or their ligands, changes in immune cell function or quantity, activation of proinflammatory cytokines and chemokines, and epileptogenesis are all assumed to be influenced by immune system elements (17-19). In the elderly, T helper cell 17 (Th17) activation was suspected in autoimmune epilepsy pathogenesis (20). In another study, Cluster of differentiation 8+T cell (CD8+ T cell)-initiated attack caused astrogliosis and microglial activation, consequently inducing temporal lobe epilepsy (21). Also, elevated macrophage migration inhibitory factor levels in plasma contributed to detrimental autoimmunity, neuroinflammation, and epilepsy (22). However, the distribution of immune cell infiltration remains unexplained.
Long non-coding RNA (lncRNA) demonstrates a relationship between the congenital immune response and neuroinflammation in the development of epilepsy. In epilepsy models, lncRNAs regulate gene expression by competitively binding to microRNAs (miRNAs) (23-25). Recent study has shown that the lncRNA ZNF883 inhibits NLRP3 ubiquitination and promotes epilepsy through upregulating USP47 (26), and lncRNA ZFAS1 might contribute to the progression of epilepsy by regulating the miR-15a-5p/OXSR1/NF-κB pathway. However, this was only briefly examined in recent research based on the association between infiltrating immune cells and immune-related competitive endogenous RNA (ceRNA) regulatory networks in epilepsy.
In the present study, various bioinformatics-related methods were utilized to identify differentially expressed lncRNAs (DElncRNAs) and differentially expressed genes (DEGs) as well as to create a ceRNA network. Single Sample Gene Set Enrichment Analysis (ssGSEA) and the CIBERSORT algorithm were used to identify immune cell infiltration in epileptic tissues. Immune-related genes (IRGs) were overlapped with DEGs in the ceRNA network to filter for differentially expressed IRGs (DEIRGs). After constructing a protein-protein interaction (PPI) network, the core IRGs were obtained by intersecting the degree and maximum neighborhood component (MNC) topological analysis methods of the “cytoHubba” (Cytoscape 3.8.2 plug-in from cytoscape.org.). Correlations between key immune genes and immune cells were assessed using Pearson correlation. Finally, the core IRGs were validated using clinical tissue samples to identify new therapeutic targets for epilepsy. Our findings will provide new insights into the mechanism of epilepsy development and highlight a promising prognostic and treatment marker for epilepsy patients. We present the following article in accordance with the MDAR and ARRIVE reporting checklists (available at https://tp.amegroups.com/article/view/10.21037/tp-23-196/rc).
Methods
Sample collection
The Neurosurgery Department at Chongqing Medical University contributed five surgical specimens obtained from individuals with FCD-related secondary epilepsy. The samples were transported in liquid nitrogen dry shippers after procedural cooling (programmed cooling) and finally stored in a freezer at −80 ℃. Control samples were provided by the Forensic Department of Medical Medicine at Chongqing Medical University. These included two dead human cases with no history or signs of neurological or psychiatric diseases and whose living brain tissue was isolated and preserved within 12 h of death. The clinical characteristics of the patients and controls as well as relevant information are described in Table S1. This study was approved by the Ethics Committee of the Affiliated Children’s Hospital of Chongqing Medical University (approval number #2021-295), and informed consent was obtained from all participants. The study was conducted in accordance with the Declaration of Helsinki (as revised in 2013).
The Chongqing Medical University’s Laboratory Animal Center provided male C57BL/6 J mice, which were kept in a specific-pathogen-free environment. We established a mouse model of kainic acid (KA)-induced epilepsy (27). Anesthetized 4-week-old mice were intraperitoneally injected with 20 mg/kg KA (28), while control mice were injected with saline. At least one behavioral spontaneous recurrent motor seizure observed 28 d after injection was considered a successful model of chronic epilepsy. All mice were euthanized 28 d after surgery. Experiments were performed under the approval granted by the Ethics Committee of the Affiliated Children’s Hospital of Chongqing Medical University, in compliance with national guidelines for the care and use of animals. A protocol was prepared before the study without registration.
Isolation, quality assessment, and library creation of RNA
Total RNA was isolated from brain tissue using the TRIzol reagent (Invitrogen, Carlsbad, CA, USA). A NanoDrop spectrophotometer and an Agilent 2100 bioanalyzer (Thermo Fisher Scientific, MA, USA) were used to measure the RNA quantity and concentration. RNA of desired quality was kept at −80 ℃ to sequence messenger RNA (mRNA) and lncRNA and conduct future studies. Shanghai Life Gene Technology Co. Ltd. (Shanghai, China) performed the library preparation and light sequencing.
Bioinformatics analysis
The “DEseq2” package in R software (Version 4.1.0) was used to analyze the RNA sequencing (RNA-seq) (lncRNA and mRNA) data for differential gene expression. Results with |log2FoldChange| >2 and a corrected P-adj <0.05 were considered significant.
The lncRNA-mediated ceRNA network was constructed based on the interactions generated from the miRcode, starBase2.0, miRDB, miRTarBase, TargetScan, and ENCORI databases. Due to an absence of miRNA-related information, the Mircode database was used to predict interactions among miRNAs of DElncRNA. miRNA-mRNA interactions were predicted using the starBase 2.0, miRDB, miRTarBase, TargetScan, and ENCORI databases to identify the target genes.
Gene Ontology (GO) and Kyoto Encyclopedia of Genes and Genomes (KEGG) analyses were performed using the R package. Genes in the key module were analyzed using the species and human parameters. The GO terms of biological processes, cellular components, and molecular functions, and KEGG pathway datasets were downloaded.
CIBERSORT and ssGSEA were employed to estimate immune cell infiltration. CIBERSORT was used to analyze the proportion of 22 infiltrating immune cells in epileptic and normal tissues, whereas the ssGSEA scores were applied to determine the immune infiltration level in each database.
Further, the IRG sets comprised the overlapping genes of the DEGs in the ceRNA network and the immune-related genes from the ImmPort database.
Data from the STRING database were used to construct the PPI network of DEIRGs. The core genes in a network were subsequently predicted and explored via the intersection of degree and MNC topological analysis methods of the “cytoHubba” plugin in Cytoscape. Metascape (http://metascape.org) revealed the underlying mechanism of key ceRNAs (EGFR, GRB2, KRAS, FOS, ESR1, MAPK1, MAPK14, MAPK8, and PPARG).
Pearson’s correlation was employed to clarify the molecular mechanism of epileptogenesis and epilepsy development by determining the correlations between the core genes and immune cells. Statistical significance was set at P<0.05.
Identification and validation of core IRGs
The core IRG validation step was carried out using the GSE205661 dataset. Epilepsy gene expression profiles were gathered using the Gene Expression Omnibus (GEO) database. Expression array, epilepsy, and Homo sapiens were the inclusion criteria.
Western blotting was utilized to assess the expression of core IRGs [epidermal growth factor receptor (EGFR)] and whole protein samples in KA-induced epilepsy and control mouse extracts. Their concentrations were quantified using a Pierce BCA Protein Assay Kit (Thermo Fisher Scientific), after which 15 g of protein was loaded. The membranes were incubated with specific primary antibodies against EGFR (Abcam, dilution 1:5,000) and glyceraldehyde-3-phosphate dehydrogenase (GAPDH) (Abcam, dilution 1:8,000) for 16 hours at 4 ℃. The membranes were then incubated for 1 h at room temperature with goat anti-rabbit immunoglobulin G (IgG) conjugated to horseradish peroxidase (Santa Cruz, dilution 1:5,000).
Statistical analyses
GraphPad Prism 8.0 was used to conduct statistical analysis (GraphPad Software, La Jolla, CA, USA). Inter-group differences were examined using a two-tailed unpaired t-test. Statistical significance was set at P<0.05.
Results
Differential expression analyses and identification
Figure 1 provides a schematic representation of the research process. Functional lncRNAs and mRNAs related to FCD-associated epilepsy were isolated from RNA-seq data. DElncRNAs and DEmRNAs had strict inclusion criteria (|log2FoldChange|2, P.adj<0.05) and were presented in a volcano map and hierarchical cluster heatmap (Figure 2). In the aggregate, 3,538 DEmRNAs (2,997 upregulated and 541 downregulated) and 1,179 DElncRNAs (930 upregulated and 249 downregulated) were chosen for our study (Figure 2).
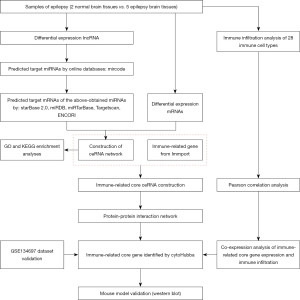
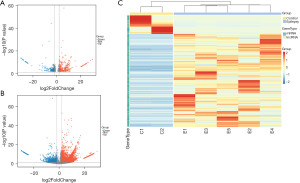
Construction of the lncRNA-mediated ceRNA network
The interaction between DElncRNAs and miRNAs was first predicted using the online Mircode database; a total of 17,881 lncRNA-miRNA pairs containing 164 DElncRNAs (Figure 3A) and 208 predicted miRNAs were acquired. To further explore potential target genes of the predicted miRNAs, we obtained 3,474 miRNA-mRNA pairs from the ENCORI, miRDB, miRTarBase, and TargetScan databases (Figure 3B). Based on the integration of 1,179 DElncRNAs and 3,538 DEmRNAs from human tissue RNA-seq, 164 DElncRNAs, 44 DEmiRNAs, and 412 DEmRNAs were incorporated into the ceRNA network.
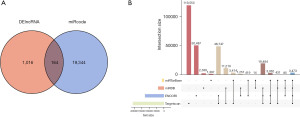
GO and KEGG analyses
GO and KEGG pathway analyses were performed to identify the biological activities and signaling pathways of mRNA in the ceRNA networks. According to the GO analysis, inflammatory response; neural apoptotic processes; leukocyte proliferation, activation, and participation; myeloid cell activation; T-cell activation regulation; and other immune-related processes were all enriched in DEGs (Figure 4A). The enrichment terms of cellular components were concentrated in the vesicle membrane; cellular activities, including immune antigen presentation and neuronal synaptic plasticity, depended on vesicular recycling transport (Figure 4B). Regarding molecular function, serine/threonine protein kinase activity was the primary mediator (Figure 4C).
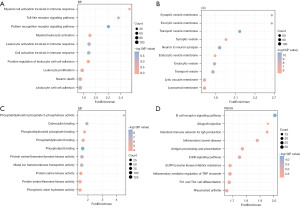
KEGG signaling pathway analysis (Figure 4D) showed that the DEGs were mainly enriched in immune-related pathways (B-cell receptor signaling, TH1 and TH2 cell differentiation) and immune- and inflammation-related diseases (inflammatory bowel disease, rheumatoid arthritis, antigen processing and presentation). Collectively, these findings established that immunoregulation is significantly involved in the pathogenesis of epilepsy.
Assessment of infiltrating immune cells
We evaluated the biological activities and signaling pathways especially immune response of mRNA in the ceRNA networks using GO and KEGG analysis. The findings established that immunoregulation is significantly involved in the pathogenesis of epilepsy. Based on this, we employed CIBERSORT and ssGSEA to estimate immune cell infiltration between the control and epileptic samples. A significant difference in the degree of immune infiltration was observed in the control and epileptic samples. The immune cell composition of invading tissues was evaluated (Figure 5A). As shown in Figure 5B, 28 immune cell types that infiltrated immune cell subpopulations in the epilepsy and control samples were depicted in a heatmap. Figure 5C shows the relationship among the 28 immune cells. A significant difference in the degree of immune infiltration was observed in the control and epileptic samples at a ratio of 9:28. To visualize the different expression levels of the 28 immune-infiltrating cells in both groups, violin plots were created using the “ggplot2” package in R (Figure 5D). Immune cell infiltration was lower in the epilepsy samples than in the controls, with type 1 T helper cells being the most prevalent.
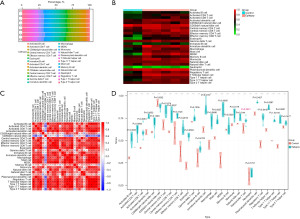
Construction of core IRGs and PPI networks
The intersection of lncRNA-related DEmRNAs and IRGs (obtained from the ImmPort database) was examined using the Venn method, and 40 immune-related DEmRNAs were discovered. This analysis helped create the immune-related core ceRNA network (Figure 6A). Using the STRING database, we created PPI networks to further investigate the interactions among immune-related DEmRNAs (Figure 6B). The networks had 375 edges and 40 nodes. The degree of connection and patterns of gene expression were represented by node size and color, respectively. Portions of the PPI networks with extensive connected regions were more likely to participate in biological regulation. Hub genes in subnetworks often play important roles. The intersection of degree (Figure 6C) and MNC topology (Figure 6D) analysis methods of the “cytoHubba” plugin in Cytoscape were applied to predict and explore the core genes. The results demonstrated that the key ceRNAs may be involved in endocrine resistance, prolactin signaling pathway, and MAPK cascade (Figure 6E). Darker colors signified higher scores, and EGFR was selected as the core IRG for follow-up studies. A total of 38 lncRNAs, one miRNA (MIR27A), and one mRNA (EGFR) formed the immune-related core ceRNA network (Figure 6F).
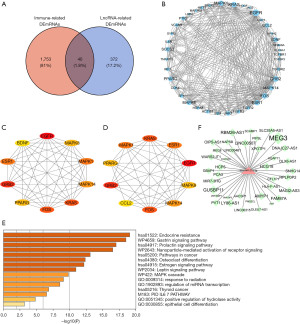
Correlation analysis
Immune cell counts in epileptic tissues were higher than those in normal tissues, and a correlation between EGFR and infiltrating immune cells was observed (Table 1). Correlated pairs with a P<0.05 were identified via the t-test used in this investigation (Figure 7). The final results showed that type 1 T helper cells were considered to be important immune cells associated with epilepsy formation and that the expression of the EGFR gene was positively correlated with EGFR as well as immature dendritic cells (R=0.857, P=0.024), mast cells (R=0.821, P=0.034), myeloid-derived suppressor cells (MDSC) (R=0.786, P=0.048), and plasmacytoid dendritic cells (R=0.857, P=0.024), and negatively correlated with CD56dim natural killer cells (R=−0.929, P=0.007) (Figure 7).
Table 1
Immune cell types | EGFR | |
---|---|---|
r | P | |
Activated B cell | 0.464 | 0.302 |
Activated CD4 T cell | 0.679 | 0.110 |
Activated CD8 T cell | 0.750 | 0.066 |
Activated dendritic cell | 0.464 | 0.302 |
CD56bright natural killer cell | −0.107 | 0.840 |
CD56dim natural killer cell | −0.929 | 0.007 |
Central memory CD4 T cell | 0.393 | 0.396 |
Central memory CD8 T cell | 0.643 | 0.139 |
Effector memory CD4 T cell | 0.321 | 0.498 |
Effector memory CD8 T cell | 0.750 | 0.066 |
Eosinophil | 0.393 | 0.396 |
Gamma delta T cell | −0.536 | 0.236 |
Immature B cell | 0.393 | 0.396 |
Immature dendritic cell | 0.857 | 0.024 |
Macrophage | 0.321 | 0.498 |
Mast cell | 0.821 | 0.034 |
MDSC | 0.786 | 0.048 |
Memory B cell | −0.286 | 0.556 |
Monocyte | −0.036 | 0.963 |
Natural killer cell | 0.000 | 1.000 |
Natural killer T cell | 0.143 | 0.783 |
Neutrophil | 0.214 | 0.662 |
Plasmacytoid dendritic cell | 0.857 | 0.024 |
Regulatory T cell | 0.679 | 0.110 |
T follicular helper cell | 0.500 | 0.267 |
Type 1 T helper cell | 0.357 | 0.444 |
Type 17 T helper cell | 0.643 | 0.139 |
Type 2 T helper cell | 0.000 | 1.000 |
EGFR, epidermal growth factor receptor.
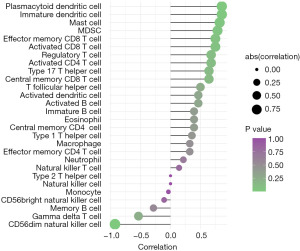
Identification and validation of EGFR in epilepsy
First, we used the GSE134697 dataset to validate the diagnostic value of EGFR in epilepsy. Further analysis demonstrated that EGFR was substantially expressed in epilepsy compared to the control (Figure 8A), corroborating previous studies. Second, we determined the EGFR expression in animal epilepsy models using western blot (Figure 8B), and the findings were consistent with the sequencing results.
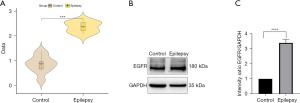
Discussion
Epilepsy is characterized by brief episodes of brain dysfunction caused by abrupt and aberrant discharges of brain neurons (9,29). The most frequent cause of juvenile focal epilepsies requiring surgical intervention is FCD (30,31). Despite developments in surgical and pharmacological therapies, FCD-associated epilepsy progression remains inefficiently controlled. The involvement of a dysregulated ceRNA network in epilepsy is only partially understood. Hence, understanding the molecular mechanisms underlying pathogenesis is pivotal to improving pediatric focal epilepsy treatments. Therefore, RNA-seq data were used to analyze the lncRNA and mRNA expressions in pediatric focal epilepsy and normal brain tissues. Finding new immunological targets for epilepsy treatment will require more research on the relationship between immune response in the brain as well as epilepsy development and prognosis (32,33).
The innate and adaptive immune responses were observed in the brain tissues of (33) patients with temporal lobe epilepsy and experimental models, according to previous investigations (34-37). Immune system components, including changes in immune cell function or number, proinflammatory cytokine and chemokine production, and the expression of inhibitory receptors or their ligands, contribute to epilepsy onset and progression (17-19). The existing literatures have been reported that microglial activation in the hippocampus presents in hippocampal sclerosis (31,38-40). In human hippocampal sclerosis specimens, reactive astrocytes and surviving neurons showed signs of nuclear factor kappa B overexpression (41). B (CD20+) and T (CD3+) lymphocytes were found in perivascular temporal lobe epilepsy and scattered in the brain parenchyma, causing neurotoxicity and glial cell damage (19). These studies demonstrate the connection between inflammatory and immunological processes and epilepsy.
In the present study, we obtained brain tissues from individuals with cortical dysplasia epilepsy and sequenced them to investigate the immune landscape of epilepsy and discover novel biomarkers as potential therapeutic targets. According to the KEGG and GO analyses of the ceRNA network, TH1 and TH2 cell differentiation and B-cell receptor signaling were implicated in pathological stress response and immunoregulatory mechanisms in seizures. These results were consistent with those of Rodgers et al. The cortical innate immune response enhanced the excitability of local and central brain neurons, causing seizures and focal seizures, respectively. Further, interleukin-1 release promoted neuronal excitability owing to Toll-like receptor 4 (TLR4)’s response to danger signals, which are linked to brain injury and central nervous system (CNS) infections (42).
Our research also investigated immune cell infiltration in cortical dysplasia epilepsy. Based on the variations between groups when immune cell abundances were evaluated, we established an immune-related core ceRNA regulatory network. Higher immune scores in epileptic surgery samples compared to normal samples implied that epilepsy development is linked to immune-related pathway factors. Immune infiltration analysis revealed that immune cells were abundant in epileptic samples; activated B and CD8 T cells, central memory CD8 T cells, eosinophils, immature dendritic cells, mast cells, T follicular helper cells, Type 1 T helper cells, and Th17 cells demonstrated that the activation of high-mobility group protein B1 (HMGB1)/chemokine C-X-C motif ligand 12 (CXCL12)-mediated immunity and Th17 cells played a key role in the pathogenesis of epilepsy (20) and that varying attacks on hippocampal neurons from CD8+ T cells can induce temporal lobe epilepsy (21). Furthermore, Th17-associated circulating proinflammatory cytokines are elevated in the sera of epileptic tissues (43), which is consistent with our results. Therefore, analyzing the relevant immune characteristics in patients with epilepsy can elucidate the pathogenesis and treatment of epilepsy.
Peripheral nerve resident mast cells serve as the first line of activation at the injured site in neuropathic pain, enabling the recruitment of neutrophils and macrophages (44). Following temporary cerebral ischemia, cerebral mast cells can damage the blood-brain barrier by controlling acute microvascular gelatinase activity (45). Histamine and other neuron-stimulant molecules, such as tumor necrosis factor-α (TNF-α) and interleukin-2, induce seizures in susceptible children (46). Mast cell-glia communication mediates inflammatory response and CNS disorders, including neuropathic pain, epilepsy, and neurodegenerative diseases (47). As localized tissue acidification occurs during epilepsy and cerebral ischemia, histamine directly increases neuronal death under enhanced synaptic neurotransmission conditions. This evidence illustrates that mast cell involvement in the immune response to achieve neuroinflammatory regulation has a potential regulatory role in epilepsy. Further, It was reported that EGFR was significantly associated with the risk of epilepsy occurrence (48), and overexpression of EGFR caused by focal copy number gains was identified in epilepsy (49). EGFR belongs to the receptor tyrosine kinase family. DNA methyltransferase 3 alpha (DNMT3) may regulate EGFR involved in cortical development, neuronal plasticity, and epileptogenesis in patients with type II FCD (50). Mice that underwent brain-specific EGFR ablation were found to be sensitive to KA-induced seizures and showed signs of neurodegeneration (50,51). EGFR inhibitor was identified as a novel antiepileptic choice (52). Therefore, we hypothesized that an immunological signal of epilepsy included a link between mast cells and EGFR.
To that end, we used western blotting to determine the correlation between core IRG (EGFR) and invading immune cells in a mouse epilepsy model. The results suggested the involvement of EGFR in the initiation, maintenance, and progression of epilepsy. EGFR was significantly associated with mast cells, and immune cell infiltration was abundant in epileptic tissue. Additionally, mast cells and EGFR gene expression exhibited a positive correlation (R=0.857, P=0.0027).
However, the present study had some limitations. Based on the ssGSEA, the immune cell infiltration analysis used incomplete cell types. Furthermore, the fundamental mechanisms governing the ceRNA network and immune cells remain unknown, necessitating additional functional biological studies with larger sample sizes.
Conclusions
In this study, an immune-related ceRNA network was initially developed in epilepsy, consisting of 38 lncRNAs, one miRNA (hsa-miR-27a-3p), and one mRNA (EGFR). Immune cell infiltration was examined to determine the abundance and quantity of the immune cells. EGFR was positively correlated with immature dendritic cells, mast cells, MDSC and plasmacytoid dendritic cells, and negatively correlated with CD56dim natural killer cells. These findings provide new insights into the pathogenesis and progression of epilepsy as well as novel potential therapeutic targets. In the future, new drugs could be developed for these therapeutic targets to delay the progression of epilepsy.
Acknowledgments
Funding: This study was supported by the National Natural Science Foundation of China (No.81971217) and the Scientific Research Project of the National Clinical Research Center of Child Health and Disorders (No. NCRC-2019-GP-17).
Footnote
Reporting Checklist: The authors have completed the MDAR and ARRIVE reporting checklists. Available at https://tp.amegroups.com/article/view/10.21037/tp-23-196/rc
Data Sharing Statement: Available at https://tp.amegroups.com/article/view/10.21037/tp-23-196/dss
Peer Review File: Available at https://tp.amegroups.com/article/view/10.21037/tp-23-196/prf
Conflicts of Interest: All authors have completed the ICMJE uniform disclosure form (available at https://tp.amegroups.com/article/view/10.21037/tp-23-196/coif). The authors have no conflicts of interest to declare.
Ethical Statement: The authors are accountable for all aspects of the work, including ensuring that any questions related to the accuracy or integrity of any part of the work have been appropriately investigated and resolved. All patients who participated in this study signed an informed consent form, and this study was approved by the Ethics Committee of the Affiliated Children’s Hospital of Chongqing Medical University (approval number #2021-295). The study was conducted in accordance with the Declaration of Helsinki (as revised in 2013). Experiments were performed under the approval granted by the Ethics Committee of the Affiliated Children’s Hospital of Chongqing Medical University, in compliance with national guidelines for the care and use of animals.
Open Access Statement: This is an Open Access article distributed in accordance with the Creative Commons Attribution-NonCommercial-NoDerivs 4.0 International License (CC BY-NC-ND 4.0), which permits the non-commercial replication and distribution of the article with the strict proviso that no changes or edits are made and the original work is properly cited (including links to both the formal publication through the relevant DOI and the license). See: https://creativecommons.org/licenses/by-nc-nd/4.0/.
References
- De Vito A, Mankad K, Pujar S, et al. Narrative review of epilepsy: getting the most out of your neuroimaging. Transl Pediatr 2021;10:1078-99. [Crossref] [PubMed]
- Blümcke I, Vinters HV, Armstrong D, et al. Malformations of cortical development and epilepsies: neuropathological findings with emphasis on focal cortical dysplasia. Epileptic Disord 2009;11:181-93. [PubMed]
- Iffland PH 2nd, Crino PB. Focal Cortical Dysplasia: Gene Mutations, Cell Signaling, and Therapeutic Implications. Annu Rev Pathol 2017;12:547-71. [Crossref] [PubMed]
- Zhang Z, Liu Q, Liu M, et al. Upregulation of HMGB1-TLR4 inflammatory pathway in focal cortical dysplasia type II. J Neuroinflammation 2018;15:27. [Crossref] [PubMed]
- Chang BS, Lowenstein DH. Practice parameter: antiepileptic drug prophylaxis in severe traumatic brain injury: report of the Quality Standards Subcommittee of the American Academy of Neurology. Neurology 2003;60:10-6. [Crossref] [PubMed]
- Farrell JS, Wolff MD, Teskey GC. Neurodegeneration and Pathology in Epilepsy: Clinical and Basic Perspectives. Adv Neurobiol 2017;15:317-34. [Crossref] [PubMed]
- McGinnity CJ, Smith AB, Yaakub SN, et al. Decreased functional connectivity within a language subnetwork in benign epilepsy with centrotemporal spikes. Epilepsia Open 2017;2:214-25. [Crossref] [PubMed]
- Mehvari-Habibabadi J, Basiratnia R, Moein H, et al. Prognostic value of ictal onset patterns in postsurgical outcome of temporal lobe epilepsy. Iran J Neurol 2017;16:185-91. [PubMed]
- Thijs RD, Surges R, O'Brien TJ, et al. Epilepsy in adults. Lancet 2019;393:689-701. [Crossref] [PubMed]
- Viguera AC, Fan Y, Thompson NR, et al. Prevalence and Predictors of Depression Among Patients With Epilepsy, Stroke, and Multiple Sclerosis Using the Cleveland Clinic Knowledge Program Within the Neurological Institute. Psychosomatics 2018;59:369-78. [Crossref] [PubMed]
- Hou Y, Chen Z, Wang L, et al. Characterization of Immune-Related Genes and Immune Infiltration Features in Epilepsy by Multi-Transcriptome Data. J Inflamm Res 2022;15:2855-76. [Crossref] [PubMed]
- Rodriguez AS, Engel T, Palfi A, et al. Tubby-like protein 1 (Tulp1) is a target of microRNA-134 and is down-regulated in experimental epilepsy. Int J Physiol Pathophysiol Pharmacol 2017;9:178-87. [PubMed]
- Vezzani A, French J, Bartfai T, et al. The role of inflammation in epilepsy. Nat Rev Neurol 2011;7:31-40. [Crossref] [PubMed]
- Rijkers K, Majoie HJ, Hoogland G, et al. The role of interleukin-1 in seizures and epilepsy: a critical review. Exp Neurol 2009;216:258-71. [Crossref] [PubMed]
- Ayanoğlu M, Çevik Ö, Erdoğan Ö, et al. TARC and Septin 7 can be better monitoring biomarkers than CX3CL1, sICAM5, and IRF5 in children with seizure-free epilepsy with monotherapy and drug-resistant epilepsy. Int J Neurosci 2022; Epub ahead of print. [Crossref] [PubMed]
- Basnyat P, Pesu M, Söderqvist M, et al. Chronically reduced IL-10 plasma levels are associated with hippocampal sclerosis in temporal lobe epilepsy patients. BMC Neurol 2020;20:241. [Crossref] [PubMed]
- Tang H, Wang X. PD-1 Is an Immune-Inflammatory Potential Biomarker in Cerebrospinal Fluid and Serum of Intractable Epilepsy. Biomed Res Int 2021;2021:7973123. [Crossref] [PubMed]
- Gnatek Y, Zimmerman G, Goll Y, et al. Acetylcholinesterase loosens the brain's cholinergic anti-inflammatory response and promotes epileptogenesis. Front Mol Neurosci 2012;5:66. [Crossref] [PubMed]
- Gales JM, Prayson RA. Chronic inflammation in refractory hippocampal sclerosis-related temporal lobe epilepsy. Ann Diagn Pathol 2017;30:12-6. [Crossref] [PubMed]
- Han Y, Yang L, Liu X, et al. HMGB1/CXCL12-Mediated Immunity and Th17 Cells Might Underlie Highly Suspected Autoimmune Epilepsy in Elderly Individuals. Neuropsychiatr Dis Treat 2020;16:1285-93. [Crossref] [PubMed]
- Pitsch J, van Loo KMJ, Gallus M, et al. CD8(+) T-Lymphocyte-Driven Limbic Encephalitis Results in Temporal Lobe Epilepsy. Ann Neurol 2021;89:666-85. [Crossref] [PubMed]
- Benedek G, Abed El Latif M, Miller K, et al. Macrophage migration inhibitory factor in Nodding syndrome. PLoS Negl Trop Dis 2021;15:e0009821. [Crossref] [PubMed]
- Wan Y, Yang ZQ. LncRNA NEAT1 affects inflammatory response by targeting miR-129-5p and regulating Notch signaling pathway in epilepsy. Cell Cycle 2020;19:419-31. [Crossref] [PubMed]
- Cai X, Long L, Zeng C, et al. LncRNA ILF3-AS1 mediated the occurrence of epilepsy through suppressing hippocampal miR-212 expression. Aging (Albany NY) 2020;12:8413-22. [Crossref] [PubMed]
- Han CL, Liu YP, Guo CJ, et al. The lncRNA H19 binding to let-7b promotes hippocampal glial cell activation and epileptic seizures by targeting Stat3 in a rat model of temporal lobe epilepsy. Cell Prolif 2020;53:e12856. [Crossref] [PubMed]
- Gong L, Han Y, Chen R, et al. LncRNA ZNF883-Mediated NLRP3 Inflammasome Activation and Epilepsy Development Involve USP47 Upregulation. Mol Neurobiol 2022;59:5207-21. [Crossref] [PubMed]
- Wu W, Li Y, Wei Y, et al. Microglial depletion aggravates the severity of acute and chronic seizures in mice. Brain Behav Immun 2020;89:245-55. [Crossref] [PubMed]
- Li X, Yang C, Shi Y, et al. Abnormal neuronal damage and inflammation in the hippocampus of kainic acid-induced epilepsy mice. Cell Biochem Funct 2021;39:791-801. [Crossref] [PubMed]
- Goldberg EM, Coulter DA. Mechanisms of epileptogenesis: a convergence on neural circuit dysfunction. Nat Rev Neurosci 2013;14:337-49. [Crossref] [PubMed]
- Guerrini R, Barba C. Focal cortical dysplasia: an update on diagnosis and treatment. Expert Rev Neurother 2021;21:1213-24. [Crossref] [PubMed]
- Perucca P, Bahlo M, Berkovic SF. The Genetics of Epilepsy. Annu Rev Genomics Hum Genet 2020;21:205-30. [Crossref] [PubMed]
- Cordero-Arreola J, West RM, Mendoza-Torreblanca J, et al. The Role of Innate Immune System Receptors in Epilepsy Research. CNS Neurol Disord Drug Targets 2017;16:749-62. [Crossref] [PubMed]
- Aarli JA. Epilepsy and the immune system. Arch Neurol 2000;57:1689-92. [Crossref] [PubMed]
- Vezzani A, Lang B, Aronica E. Immunity and Inflammation in Epilepsy. Cold Spring Harb Perspect Med 2015;6:a022699. [Crossref] [PubMed]
- Granata T, Cross H, Theodore W, et al. Immune-mediated epilepsies. Epilepsia 2011;52:5-11. [Crossref] [PubMed]
- Zattoni M, Mura ML, Deprez F, et al. Brain infiltration of leukocytes contributes to the pathophysiology of temporal lobe epilepsy. J Neurosci 2011;31:4037-50. [Crossref] [PubMed]
- Silverberg J, Ginsburg D, Orman R, et al. Lymphocyte infiltration of neocortex and hippocampus after a single brief seizure in mice. Brain Behav Immun 2010;24:263-72. [Crossref] [PubMed]
- Sheng JG, Boop FA, Mrak RE, et al. Increased neuronal beta-amyloid precursor protein expression in human temporal lobe epilepsy: association with interleukin-1 alpha immunoreactivity. J Neurochem 1994;63:1872-9. [Crossref] [PubMed]
- Beach TG, Woodhurst WB, MacDonald DB, et al. Reactive microglia in hippocampal sclerosis associated with human temporal lobe epilepsy. Neurosci Lett 1995;191:27-30. [Crossref] [PubMed]
- Ravizza T, Gagliardi B, Noé F, et al. Innate and adaptive immunity during epileptogenesis and spontaneous seizures: evidence from experimental models and human temporal lobe epilepsy. Neurobiol Dis 2008;29:142-60. [Crossref] [PubMed]
- Crespel A, Coubes P, Rousset MC, et al. Inflammatory reactions in human medial temporal lobe epilepsy with hippocampal sclerosis. Brain Res 2002;952:159-69. [Crossref] [PubMed]
- Rodgers KM, Hutchinson MR, Northcutt A, et al. The cortical innate immune response increases local neuronal excitability leading to seizures. Brain 2009;132:2478-86. [Crossref] [PubMed]
- Ouédraogo O, Rébillard RM, Jamann H, et al. Increased frequency of proinflammatory CD4 T cells and pathological levels of serum neurofilament light chain in adult drug-resistant epilepsy. Epilepsia 2021;62:176-89. [Crossref] [PubMed]
- Zuo Y, Perkins NM, Tracey DJ, et al. Inflammation and hyperalgesia induced by nerve injury in the rat: a key role of mast cells. Pain 2003;105:467-79. [Crossref] [PubMed]
- Mattila OS, Strbian D, Saksi J, et al. Cerebral mast cells mediate blood-brain barrier disruption in acute experimental ischemic stroke through perivascular gelatinase activation. Stroke 2011;42:3600-5. [Crossref] [PubMed]
- Girolamo F, Coppola C, Ribatti D. Immunoregulatory effect of mast cells influenced by microbes in neurodegenerative diseases. Brain Behav Immun 2017;65:68-89. [Crossref] [PubMed]
- Skaper SD, Facci L. Mast cell-glia axis in neuroinflammation and therapeutic potential of the anandamide congener palmitoylethanolamide. Philos Trans R Soc Lond B Biol Sci 2012;367:3312-25. [Crossref] [PubMed]
- Pan SP, Zheng XL, Zhang N, et al. A novel nomogram for predicting the risk of epilepsy occurrence after operative in gliomas patients without preoperative epilepsy history. Epilepsy Res 2021;174:106641. [Crossref] [PubMed]
- Vasudevaraja V, Rodriguez JH, Pelorosso C, et al. Somatic Focal Copy Number Gains of Noncoding Regions of Receptor Tyrosine Kinase Genes in Treatment-Resistant Epilepsy. J Neuropathol Exp Neurol 2021;80:160-8. [Crossref] [PubMed]
- Aronica E, Crino PB. Inflammation in epilepsy: clinical observations. Epilepsia 2011;52:26-32. [Crossref] [PubMed]
- Robson JP, Wagner B, Glitzner E, et al. Impaired neural stem cell expansion and hypersensitivity to epileptic seizures in mice lacking the EGFR in the brain. Febs j 2018;285:3175-96. [Crossref] [PubMed]
- Liu J, Sternberg AR, Ghiasvand S, et al. Epilepsy-on-a-Chip System for Antiepileptic Drug Discovery. IEEE Trans Biomed Eng 2019;66:1231-41. [Crossref] [PubMed]
(English Language Editor: A. Kassem)