Screening of key genes in childhood asthma based on bioinformatics analysis
Highlight box
Key findings
• This study screened the key genes CXCL12, MMP9, and WNT2 of childhood asthma using a bioinformatics analysis and machine-learning algorithm.
What is known and what is new?
• The key genes of childhood asthma have not yet been determined, which may be related to the lack of comprehensive exploration of gene expression and the use of reasonable algorithms.
• This study used bioinformatics analysis tools and machine-learning algorithms to analyze the gene expression profile data of children with uncontrolled asthma symptoms and children with controlled asthma symptoms and identified the key genes related to childhood asthma.
What is the implication, and what should change now?
• The findings of this study may guide the diagnosis of pediatric asthma patients, extend understandings of the molecular mechanisms of pediatric asthma, and lead to the development of new drugs.
Introduction
Asthma is mainly characterized by recurrent airway obstruction and bronchospasm, and the symptoms in the acute attack stage have a serious effect on children’s physical and mental health (1,2). Currently, asthma requires long-term complex treatment strategies and may rapidly deteriorate in a short period of time (3). Childhood asthma is closely related to genetic and allergic factors (2,3). Following genome-wide association studies and subsequent validation studies, the gene mutation sites related to childhood asthma have been identified, and research has shown that the ORM1-Like Protein 3 (ORMDL3)/gasdermin A (GSDMA) locus on chromosome 17q12 is closely related to childhood asthma (4).
Several studies have examined the relationship between gene expression and childhood asthma (5-7). The adrenoceptor beta 2 (ADRB2) gene is closely related to the pathogenesis of childhood asthma (5). The interleukin 33 (IL-33)/interleukin 1 receptor-like 1 (IL-1RL1) pathway plays an important role in the pathogenesis of childhood asthma (6). Rigoli et al. expressed the view that genes variants with environmental factors contributes to the occurrence of childhood asthma (7). However, the key genes that can serve as diagnostic markers for childhood asthma have not yet been identified. This may be related to the lack of comprehensive exploration on gene expression and the use of reasonable algorithms. Most previous studies have only elucidated the role of a certain gene or mechanism pathway in asthma, and are unable to efficiently, accurately, and comprehensively screen diagnostic biomarkers. The advancement of whole genome sequencing technology provides an opportunity to comprehensively screen key genes for childhood asthma from a macro perspective and determine serological diagnostic markers. Thus, screening the key genes in children with asthma based on whole transcriptome sequencing results could provide a basis for understanding how susceptible individuals develop allergic diseases, and is of great significance for exploring new targets for exploring diagnostic markers of diseases.
This study used bioinformatics analysis tools and machine-learning algorithms to analyze the gene expression profiling data of children with asthma to screen out the key genes associated with childhood asthma and test the diagnostic efficacy of key genes in childhood asthma. This article is presented in accordance with the STREGA reporting checklist (available at https://tp.amegroups.com/article/view/10.21037/tp-23-204/rc).
Methods
Data download
The whole transcriptome sequencing results (GSE188424) of childhood asthma plasma samples were downloaded from the Gene Expression Omnibus (GEO) database. There were 43 controlled pediatric asthma serum samples and 46 uncontrolled pediatric asthma serum samples. The study was conducted in accordance with the Declaration of Helsinki (as revised in 2013).
Differential analysis
R software (v.3.5.1) and R package (AT&T Bell Laboratories) were used to screen the differentially expressed genes (DEGs) in the serum samples of the asthmatic and non-asthmatic children. Due to the small sample size of this study. We used a t-test based on small sample data with random variance model correction to screen DEGs. The following screening criteria were set: a fold change (FC) >2 times, and an adjusted P value <0.05.
Enrichment analysis
Kyoto Encyclopedia of Genes and Genomes (KEGG) and Gene Ontology (GO) enrichment analyses were performed on the DEGs using the R software (AT&T Bell Laboratories) and R package.
Key gene screening
We used the weighted gene co-expression network analysis (WGCNA) package in R software (AT&T Bell Laboratories) to screen hub genes. First, the correlations among all the genes were calculated and a topological overlap matrix (TOM) was constructed. The diss TOM between the genes was calculated using the following formula: diss TOM =1− TOM. A phylogenetic clustering tree was then established based on the hierarchical clustering of dissTOM; that is, genes with similar expression were divided into the same modules. The minimum value of the module gene was set to 30, and the generation eigenvector (GE) value was calculated, and the modules with high similarity were clustered and merged.
This study used 2 approaches to identify the modules associated with the clinical phenotypes. The first method calculated the correlation coefficient between the module characteristic gene and the disease trait, and the P value of each module to determine the key module. The second method calculated the gene significance (GS) and module significance (MS) to identify the hub genes. The hub genes. were screened using the following criteria as the standard: MM >0.8, and GS >0.5.
Least absolute shrinkage and selection operator (LASSO) regression analysis
In this study, a penalty model was constructed by a LASSO regression analysis, and the genes in the gene module were further screened.
Evaluation of diagnostic efficacy
The receiver operating characteristic (ROC) curves were used to evaluate the diagnostic efficacy of the key genes for asthma in children with non-asthma. The larger the area under the curve, the better the diagnostic performance of the genes.
Statistical analysis
R software (v.3.5.1, AT&T Bell Laboratories) and related R packages were used for the statistical analysis in this study. A 2-sided P value <0.05 indicated statistical significance.
Results
Screening of DEGs
We screened a total of 171 DEGs in the controlled and uncontrolled samples. Compared to the controlled samples, 118 genes were downregulated and 53 genes were upregulated in the uncontrolled samples. A heat map and volcano map of the DEGs are shown in Figures 1,2, respectively.
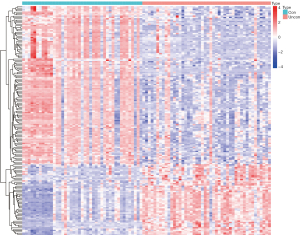
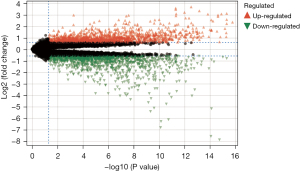
GO enrichment analysis
The GO enrichment analysis showed that the DEGs were significantly enriched in a number of biological process items, including leukocyte chemotaxis, tissue homeostasis, macrophage chemotaxis, inflammatory responses, and hormone metabolism processes. Additionally, the DEGs were significantly enriched in a number of cellular component items including the collagen-containing extracellular matrix, endoplasmic reticulum lumen, base of cells, apical portion of cells, and collagen trimers. The DEGs were also significantly enriched in a number of molecular function items including the extracellular matrix structural composition, glycosaminoglycan binding, peptidase-regulated activity, extracellular matrix structural composition, and imparting tensile strength (Figure 3).
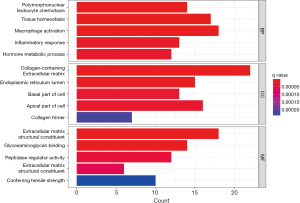
KEGG enrichment analysis
The KEGG enrichment analysis showed that DEGs were significantly enriched in a number of pathways, including the cytokine-cytokine receptor interaction, tumor necrosis factor signaling pathway, nuclear factor-kappa beta (NF-κB) signaling pathway, rheumatoid arthritis, chemokine signaling pathway, interleukin 17 (IL-17) signaling pathway, gastric acid secretion, and toll-like receptor signaling pathway (Figure 4).
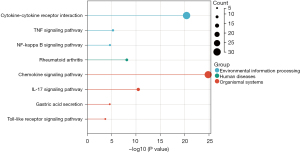
WGCNA screening of the co-expressed genes
In this study, we conducted a WGCNA to screen the co-expressed genes associated with the disease. We set a soft threshold of β =5, used the dynamic clipping tree method to initially identify the modules, merged the similar modules, set the minimum number of genes for each gene network module to 30, and ultimately obtained 8 modules, of which the gray modules could not be aggregated with the other modules. Gene set. As Figure 5 shows, we calculated the correlations among different modules for both the disease-controlled and uncontrolled clinical phenotypes. The absolute value of the correlation coefficient between the green module and the clinical phenotype was the largest. The green module were positively correlated with the uncontrolled clinical phenotype (r=0.81, P=5e-22). As Figure 6 shows, to ensure the accuracy of the screening of the key modules, we re-screened the key modules using another method, and found that the green module had the largest GS value (Figure 7). We identified the green module as the key module. The genes in the green module may promote the development and progression of childhood asthma. In this study, using |MM| >0.8 and |GS| >0.5 as the criteria, 148 hub genes were screened in the green module (Figure 8). The hub genes and DEGs had 30 overlapping genes (Figure 9).
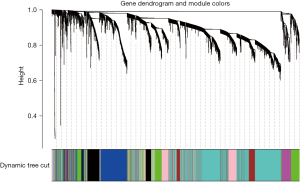
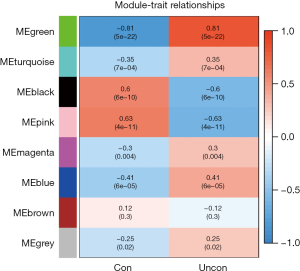
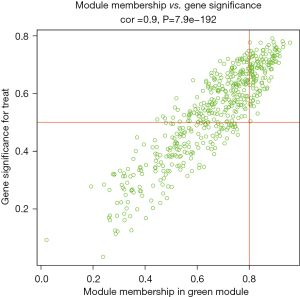
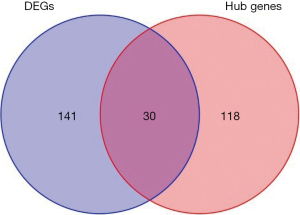
LASSO regression analysis to screen key genes
In this study, based on the above analysis results, a penalty function was constructed by a LASSO regression analysis to further screen the key genes, and a total of 3 genes [i.e., CXCL12, matrix metallopeptidase 9 (MMP9), and WNT2] were identified (Figure 10). CXCL12, MMP9, and WNT2 were upregulated in the uncontrolled samples (Figure 11). The areas under the ROC curves for CXCL12, MMP9, and WNT2 were 0.895, 0.936, and 0.928, respectively (Figure 12).
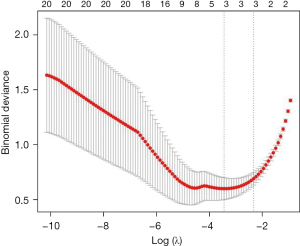
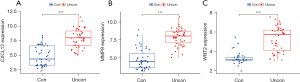
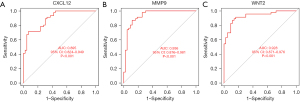
Discussion
Asthma is the most common chronic respiratory disease in children, and its morbidity and mortality rates continue to increase each year (1,3); thus, asthma represents a serious health and economic burden worldwide and seriously affects the quality of life of patients (8-11). The etiology of asthma is still unclear, but it is generally believed that it is closely related to immune, neurological, mental, endocrine, and genetic factors, and abnormal signaling pathways (12,13). The unclear pathogenesis causes serious difficulties in clinical treatment, and research on its underlying molecular mechanisms is of great significance.
We screened the DEGs of different clinical phenotypes of childhood asthma using bioinformatics technology and constructed a clinical phenotype and gene co-expression network of childhood asthma using a WGCNA. Based on the overlapping genes obtained by the using 2 methods, we identified the key genes by a LASSO regression analysis. We identified a total of 171 DEGs. The GO and KEGG enrichment analyses showed that these DEGs were significantly enriched in the inflammation-related pathways. The chemokie interaction pathway and the IL-17 signaling pathway are considered closely related to the occurrence and progression of asthma (14-16). IL-17, a hallmark cytokine produced by T-helper 17 (Th17) cells, plays a key role in host defense responses against invasion by microorganisms and in the pathogenesis of autoimmune diseases and allergic syndromes. IL-17 activates multiple downstream signal transduction pathways, including NF-κB, mitogen-activated protein kinase, and cytosine-cytosine-adenosine-adenosine-thymidine-enhancer-binding proteins, thereby inducing the gene expression of antimicrobial peptides, pro-inflammatory chemokines, cytokines, and matrix metalloproteinases (17). Blocking the IL-17 signaling pathway effectively reduces asthmatic airway inflammation (17). The chemokine signaling pathway is a signal transduction pathway formed by a combination of chemokines and their corresponding receptors. Cell chemokines are important regulators of airway hyperresponsiveness, immune cell infiltration, and inflammatory responses.
In this study, 3 key genes were identified; that is, CXCL12, MMP9, and WNT2. All 3 key genes were highly expressed in the uncontrolled samples and showed good diagnostic performance for the clinical phenotypes. CXCL12 is a classic chemokine that is associated with the occurrence of various diseases, including asthma, lung injury, and osteoarthritis. Janssens et al. (18) showed that CXCL12 recruits neutrophils to the site of inflammation through the NF-κB signaling pathway, thereby aggravating the airway inflammatory response. The use of CXCL12 neutralizing antibodies has been shown to prevent the onset of the disease or delay the progression of the disease (18). MicroRNA-23a is considered a regulator in the process of airway wall remodeling, and its mechanism of action is to inhibit the expression of CXCL12, which reduces inflammation and relieves asthma symptoms and is thus a potential therapeutic target (19). Another study (20) confirmed that miR-135b may suppress the immune response of Th17 cells by targeting CXCL12, thereby alleviating asthma airway inflammation and hyperresponsiveness.
The main function of MMP9 is to degrade and remodel the dynamic balance of the extracellular matrix, which is closely related to the release and activity of chemokines, and is involved in various inflammatory responses (21,22). MMP9 is secreted from cells to extracellular in the form of zymogen and can be activated by a series of protease cascades in vivo (21,22). MMP9 decomposes structural complexes in the respiratory tract and lung, such as the basement membrane, and is involved in the reconstruction of the respiratory tract and lung. It also regulates the activities of other proteases and cytokines, degrades antitrypsin and protects neutrophil elastase (21,22) MMP9 participates in angiogenesis by releasing vascular endothelial growth factor (23). No studies have directly linked MMP9 to childhood asthma; however, our analysis suggests that MMP9 is a key gene in childhood asthma.
WNT2 is related to the occurrence and progression of tumors (24). A previous study also suggested that WNT2 activates the NF-κB signaling pathway (25). WNT2 may recruit inflammatory cells through the NF-κB signaling pathway in childhood asthma (25). To date, few studies have been conducted on the relationship between the expression of WNT2 and childhood asthma. Thus, the role of WNT2 in childhood asthma requires further study.
There are some shortcomings in this study. Firstly, this study only screened key genes for childhood asthma based on bioinformatics analysis and evaluated diagnostic efficacy. However, there is a lack of external data for verification. It is still necessary to verify the diagnostic efficacy of key genes in clinical samples. Secondly, this study failed to elucidate the role of key genes in the onset and progression of childhood asthma. In vivo and in vitro experiments are still needed to explore the potential pathogenic mechanisms of key genes.
Conclusions
In conclusion, this study identified the key genes CXCL12, MMP9, and WNT2 in childhood asthma using a bioinformatics analysis and machine-learning algorithm. The findings of this study may guide the diagnosis of pediatric asthma patients, extend understandings of the molecular mechanisms of pediatric asthma, and lead to the development of new drugs.
Acknowledgments
Funding: None.
Footnote
Reporting Checklist: The authors have completed the STREGA reporting checklist. Available at https://tp.amegroups.com/article/view/10.21037/tp-23-204/rc
Peer Review File: Available at https://tp.amegroups.com/article/view/10.21037/tp-23-204/prf
Conflicts of Interest: All authors have completed the ICMJE uniform disclosure form (available at https://tp.amegroups.com/article/view/10.21037/tp-23-204/coif). The authors have no conflicts of interest to declare.
Ethical Statement: The authors are accountable for all aspects of the work, including ensuring that any questions related to the accuracy or integrity of any part of the work have been appropriately investigated and resolved. The study was conducted in accordance with the Declaration of Helsinki (as revised in 2013).
Open Access Statement: This is an Open Access article distributed in accordance with the Creative Commons Attribution-NonCommercial-NoDerivs 4.0 International License (CC BY-NC-ND 4.0), which permits the non-commercial replication and distribution of the article with the strict proviso that no changes or edits are made and the original work is properly cited (including links to both the formal publication through the relevant DOI and the license). See: https://creativecommons.org/licenses/by-nc-nd/4.0/.
References
- Nuzzi G, Di Cicco M, Trambusti I, et al. Primary Prevention of Pediatric Asthma through Nutritional Interventions. Nutrients 2022;14:754. [Crossref] [PubMed]
- Trikamjee T, Comberiati P, Peter J. Pediatric asthma in developing countries: challenges and future directions. Curr Opin Allergy Clin Immunol 2022;22:80-5. [Crossref] [PubMed]
- He S, Lin W, Zhong J, et al. Independent risk factors of asthma exacerbations: 3-year follow-up in a single-center prospective cohort study. Ann Transl Med 2022;10:1353. [Crossref] [PubMed]
- Moffatt MF, Kabesch M, Liang L, et al. Genetic variants regulating ORMDL3 expression contribute to the risk of childhood asthma. Nature 2007;448:470-3. [Crossref] [PubMed]
- Zhang YQ, Zhu KR. The C79G Polymorphism of the beta2-Adrenergic Receptor Gene, ADRB2, and Susceptibility to Pediatric Asthma: Meta-Analysis from Review of the Literature. Med Sci Monit 2019;25:4005-13. [Crossref] [PubMed]
- Saikumar Jayalatha AK, Hesse L, Ketelaar ME, et al. The central role of IL-33/IL-1RL1 pathway in asthma: From pathogenesis to intervention. Pharmacol Ther 2021;225:107847. [Crossref] [PubMed]
- Rigoli L, Briuglia S, Caimmi S, et al. Gene-environment interaction in childhood asthma. Int J Immunopathol Pharmacol 2011;24:41-7. [Crossref] [PubMed]
- Stern J, Pier J, Litonjua AA. Asthma epidemiology and risk factors. Semin Immunopathol 2020;42:5-15. [Crossref] [PubMed]
- Azmeh R, Greydanus DE, Agana MG, et al. Update in Pediatric Asthma: Selected Issues. Dis Mon 2020;66:100886. [Crossref] [PubMed]
- Asher MI, García-Marcos L, Pearce NE, et al. Trends in worldwide asthma prevalence. Eur Respir J 2020;56:2002094. [Crossref] [PubMed]
- Martin J, Townshend J, Brodlie M. Diagnosis and management of asthma in children. BMJ Paediatr Open 2022;6:e001277. [Crossref] [PubMed]
- Gans MD, Gavrilova T. Understanding the immunology of asthma: Pathophysiology, biomarkers, and treatments for asthma endotypes. Paediatr Respir Rev 2020;36:118-27. [Crossref] [PubMed]
- Pijnenburg MW, Fleming L. Advances in understanding and reducing the burden of severe asthma in children. Lancet Respir Med 2020;8:1032-44. [Crossref] [PubMed]
- Wei Q, Liao J, Jiang M, et al. Relationship between Th17-mediated immunity and airway inflammation in childhood neutrophilic asthma. Allergy Asthma Clin Immunol 2021;17:4. [Crossref] [PubMed]
- Steinke JW, Lawrence MG, Teague WG, et al. Bronchoalveolar lavage cytokine patterns in children with severe neutrophilic and paucigranulocytic asthma. J Allergy Clin Immunol 2021;147:686-693.e3. [Crossref] [PubMed]
- Manni ML, Robinson KM, Alcorn JF. A tale of two cytokines: IL-17 and IL-22 in asthma and infection. Expert Rev Respir Med 2014;8:25-42. [Crossref] [PubMed]
- Agarwal A, Singh M, Chatterjee BP, et al. Interplay of T Helper 17 Cells with CD4(+)CD25(high) FOXP3(+) Tregs in Regulation of Allergic Asthma in Pediatric Patients. Int J Pediatr 2014;2014:636238. [Crossref] [PubMed]
- Janssens R, Struyf S, Proost P. Pathological roles of the homeostatic chemokine CXCL12. Cytokine Growth Factor Rev 2018;44:51-68. [Crossref] [PubMed]
- Jin A, Bao R, Roth M, et al. microRNA-23a contributes to asthma by targeting BCL2 in airway epithelial cells and CXCL12 in fibroblasts. J Cell Physiol 2019;234:21153-65. [Crossref] [PubMed]
- Liu Y, Huo SG, Xu L, et al. MiR-135b Alleviates Airway Inflammation in Asthmatic Children and Experimental Mice with Asthma via Regulating CXCL12. Immunol Invest 2022;51:496-510. [Crossref] [PubMed]
- Zhang H, Liu L, Jiang C, et al. MMP9 protects against LPS-induced inflammation in osteoblasts. Innate Immun 2020;26:259-69. [Crossref] [PubMed]
- Mondal S, Adhikari N, Banerjee S, et al. Matrix metalloproteinase-9 (MMP-9) and its inhibitors in cancer: A minireview. Eur J Med Chem 2020;194:112260. [Crossref] [PubMed]
- Larsson P, Syed KA, Semenas J, et al. The functional interlink between AR and MMP9/VEGF signaling axis is mediated through PIP5K1alpha/pAKT in prostate cancer. Int J Cancer 2020;146:1686-99. [Crossref] [PubMed]
- Unterleuthner D, Neuhold P, Schwarz K, et al. Cancer-associated fibroblast-derived WNT2 increases tumor angiogenesis in colon cancer. Angiogenesis 2020;23:159-77. [Crossref] [PubMed]
- Yin C, Ye Z, Wu J, et al. Elevated Wnt2 and Wnt4 activate NF-kappaB signaling to promote cardiac fibrosis by cooperation of Fzd4/2 and LRP6 following myocardial infarction. EBioMedicine 2021;74:103745. [Crossref] [PubMed]
(English Language Editor: L. Huleatt)