Urine metabolites and viral pneumonia among children: a case-control study in China
Highlight box
Key findings
• Twenty-four metabolites were selected as potential biomarkers for four types of viral pneumonia.
What is known, and what is new?
• It is known that metabolites in urine are the end products of normal and pathologic cellular processes.
• This study identified differences between urine metabolites of children with four types of viral pneumonia and obtained a better understanding of the pathophysiological processes involved in the development of viral pneumonia.
What is the implication, and what should change now?
• Our results imply there may be a common effect across viruses.
• This finding may lead to the discovery of new treatments for viral infections and bring us closer to the development of new antiviral drugs.
Introduction
The coronavirus disease (COVID-19) was declared a pandemic in March 2020 (1) and has had a profound impact on human health and society. Despite extensive efforts, no universally approved drugs for the treatment of COVID-19 are currently available. Traditional Chinese medicine (TCM), with its focuses on the interaction between the pathogen and host, holds promise in treating this disease. However, current recommendations of TCM-based treatments (Trial Version 7) (2) mainly involve existing resources such as Chinese patent medicines and various soups, and there is a lack of newly developed therapeutic drugs (3). To address this gap, we explored a universal pattern of human response to different viral species that could help restore health in facing various viral infections using a common model.
Previous research by our team has shown promising results in the clinical and experimental studies of a modified Chinese medicine derived from the famous ma-xing-shi-gan (4). This medicine has demonstrated effectiveness against respiratory syncytial virus (RSV), influenza virus (IFV), parainfluenza virus (PIV), adenovirus (ADV) (5), and even severe acute respiratory syndrome coronavirus 2 (SARS-CoV-2) (6). The broad antiviral efficacy of this medicine suggests the possibility of a specific common pathway for viral infections.
Urine, a complex biological fluid, contains various components, including metabolic intermediates that reflect the activity of specific metabolic processes. In recent years, urine metabolomics has been widely applied in the study of pneumonia (7). Our research group has successfully employed urine metabolomics techniques and obtained preliminary results (8). Urine collection is a simple, safe, and noninvasive method, particularly suitable for children, as it imposes no adverse effects. While the appearance of metabolites in urine can be influenced by diet, the implementation of standardized diets in hospitals can help minimize this confounding factor.
This study aimed to identify alterations in urine metabolites of children with viral pneumonia compared with normal controls, providing insights into the pathophysiological processes involved in the onset and progression of viral pneumonia. Specifically, we aimed to identify common effects or biomarkers across various viruses. This research has the potential to contribute to the development of a new drug treatment for viral pneumonia. We present this article in accordance with the MDAR reporting checklist (available at https://tp.amegroups.com/article/view/10.21037/tp-23-199/rc).
Methods
Study subjects
All participants were recruited from the Affiliated Hospital of Nanjing University of Traditional Chinese Medicine and Beijing Children’s Hospital between July 1, 2013, and July 1, 2016. Participants with congenital/acquired genetic metabolic disorders, heart failure, respiratory failure, toxic encephalopathy, exudative pleurisy, severe abnormalities of liver and kidney function, and severe primary diseases with heart, liver, kidney, and hematopoietic system disorders were excluded from the study. Furthermore, participants with mental illness were also excluded from the study. In the case group, we included all participants with confirmed diagnosis of pneumonia and had clarification of several single viral infections studied in the text. Moreover, we excluded patients with clear comorbidity of other viral bacterial infections. All patients with viral pneumonia were identified based on the operative virus test performed on a pharyngeal swab or fiberoptic bronchoscopy washing fluid; however, it did not define the exact type of the virus. Sex- and age-matched normal controls were chosen in this study. The study was conducted in accordance with the Declaration of Helsinki (as revised in 2013). Informed consent was taken from all the patients’ guardians. The study was approved by Institutional Review Board (IRB) of The Affiliated Hospital of Nanjing University of Traditional Chinese Medicine (No. 2013NL-KS15) and Beijing Children’s Hospital was informed and agreed the study.
In total, 96 cases with viral pneumonia and 31 sex- and age-matched normal control subjects were included in this study. Among the case group, there were 30 patients diagnosed with RSV pneumonia, 23 patients with ADV pneumonia, 24 patients with PIV pneumonia, and 19 patients with IFV pneumonia.
Samples
Patients were clinically diagnosed with pneumonia on an outpatient basis, admitted to the ward with an inpatient certificate issued by the outpatient physician, and urine samples were taken on an empty stomach the morning following the patient’s admission (without treatment). Afterwards, positive virological test results were obtained, and those that met the inclusion criteria we returned to include the aforementioned urine samples in the study. The classification, which is based directly on the virological test results, single positive ones are included in the appropriate grouping.
After fasting overnight, the first urine sample was collected from each participant early the next morning. The collected urine samples were stored in sterile 10 mL centrifuge tubes and centrifuged at 5,000×g for 10 min at 4 ℃. And then they were filtered through a 0.45 µm filter, and finally stored at −80 ℃ until analyzed. All operational procedures, including sample collection and storage, were performed in accordance with the relevant guidelines and regulations.
Chemicals and reagents
Methanol acetonitrile [Merck KGaA (Darmstadt, Germany)], formic acid [ROE Scientific Inc. (Newark, DE, USA)], and 1-desoxymethylsphinganine-d5 [Avanti Polar Lipids (USA)] were used. Milli-Q water was used to dilute the urine samples and prepare the 1-desoxymethylsphinganine-d5 solution.
Sample preparation and analysis
The urine sample was brought to room temperature and 90 µL was measured out. An amount of 190 µg/mL. 1-desoxymethylsphinganine-d5 was added to the methanol solution (10 µL) as an internal standard, and 100 µL of pure water was added to dilute the urine sample. The sample was centrifuged at an oscillatory vortex of 17,000 rpm for 10 min. The supernatant (150 µL) was removed into a 1.5 µL Eppendorf (EP) tube. On further centrifugation, 90 µL of supernatant was poured into the 1.5 µL EP tubes for testing. Quality control (QC) samples were prepared by pooling aliquots of the urine samples of the same viral pneumonia and were processed similarly to the experimental samples. During analyses of the samples, one QC sample was run after every 10 injections.
The analysis was performed on an Linear Trap Quadrupole (LTQ)/Orbitrap XLr two-dimensional linear ion trap high-resolution electrostatic field-combined mass spectrometer (Thermo Fisher, USA) connected to an UltiMate 3000 RS (UPLC, Dionex, USA) as described in previous studies (8,9).
Data processing
Raw data obtained from the Xcalibur 2.2 software (Thermo Fisher Scientific) were transferred to the format of “XZML” using Proteo Wizard converter (http://proteowizard.sourceforge.net/downloads.shtml). We planned to follow a traditional metabolomic workflow, such as comparative analysis, MS database search, targeted MS acquisition, comparison mass Spectrometry (MS) spectra, and identification of the mater and huge handing job. Hence, we chose XCMS (X-ray Charge Mobility Spectrometer, a software of R-based platform for raw liquid chromatography-mass spectrometry [LC-MS] data processing and visualization). Moreover, the XCMS Online, an innovative platform processing untargeted metabolomics by LC-MS, provides a complete metabolomics workflow, including feature detection, retention time correction, alignment, annotation, and statistical analysis (10,11).
We set the parameters as follows: polarity: positive; retention time format: minutes; feature detection method: centWave; ppm: 2.5; snthr: 10; peakwidth: 5–20; mzdiff: 0.01; prefilter peaks: 3; prefilter intensity: 5,000; noise: 1,000. We selected 10 ion peaks for the mass-charge ratio and relative time (RT) ratio and compared them for stability verification. As a result, the change in the mass-charge ratio relative standard deviation was 0.000–0.000%, and that of the RT relative standard deviation was 0.144–5.135%. This showed that the system platform was stable and the data were reliable. We determined from the total ion flow superposition diagram of the four study groups and NC that the peak time and ion peak form of each group were comparable, and the ion peak form was stable, indicating the stability of the liquid platform state.
The Metabolite and Tandem Mass Spectrometry (METLIN) database (https://metlin.scripps.edu) has over 500,000 molecular standards with MS/MS data at multiple energies and in pos/neg modes that could be used for metabolite m/z matches as described in previous studies (12,13). XCMS Online searched and compared the ion dissociation spectrum (MS/MS) and matched similar collision energy (eV), peak (max: 30 peaks) M/Z, and positive mode and used the common adducts M + H, M + NH4, M + Na, M + H-H2O, M + H-2H2O, M + K, M + ACN + H, M + ACN + Na, M + 2Na-H, M + 2H, M + 3H, M + H + Na, M + 2H + Na, M + 2Na, M + 2Na + H, and M+. The METLIN parts per million (ppm) error threshold was 2 (14). The XCMS Online database uses a special technique named after the Mummichog fish. The Mummichog method maps all possible metabolite matches to the network based on the m/z feature but does not identify the metabolites (15).
Statistical analysis
ANOVA was used to test the difference of demographic and clinical parameters between four case groups and the control group. In order to identify the differentially expressed urinary metabolites, principal component analysis, non-metric multidimensional scaling, dynamic time warping, and a one-to-one interpolated warp function chromatographic alignment algorithm (16) were performed in this study. XCMS Online version 2.2.0, XCMS version 1.47.3, and CAMERA version 1.27.1. were used to evaluating urine metabolite, and compared cases with ADV, IFV, RSV, and PIV with those of normal control subjects. Databases, such as METLIN, Human Metabolome Database (HMDB), Urine Metabolome Database (UMDB), Lipid Metabolites and Pathways Strategy (LIPID), and Metabolic Atlas Projects (MAPs), were used to analyze the metabolic pathways. P value <0.05 was defined as statistical significant.
Results
Characteristics of study subjects
In total, 127 urine samples were obtained from 30, 23, 24, and 19 patients infected with RSV, ADV, PIV, and IFV, respectively, and 31 control subjects. The samples underwent untargeted metabolomic analysis. The detailed demographic and clinical parameters, including age and sex, showed no significant differences among the four case groups and the control groups (P>0.05). There were 75 males in total case group (n=96) and 20 males in the control group (n=31). The mean ± standard deviation (SD) age of the total case group and the control group were 1.56±1.37 and 1.87±1.02, respectively.
Identification of differentially expressed urinary metabolites
In total, 948 metabolites were identified by XCMS Online. After false discovery rate (FDR) correction, 24 typical metabolites were selected as potential markers. Table 1 and the figures show 24 typical potential markers (Table 1, Figures 1-6). Three viruses, including ADV, RSV, and IFV, affected the systemic metabolome, but IFV does not. Two-oxoglutarate was upregulated, and aspartate was downregulated in the ADV, RSV, and IFV-infected samples.
Table 1
ID | Compound ID | MZ | Boxplot ID | Name | Match form |
---|---|---|---|---|---|
1 | C00141 | 155.0103 | 423 | 3-methyl-2-oxobutanoic acid; 3-methyl-2-oxobutyric acid; 3-methyl-2-oxobutanoate; 2-oxo-3-methylbutanoate; 2-oxoisovalerate; 2-oxoisopentanoate; alpha-ketovaline; 2-ketovaline; 2-keto-3-methylbutyric acid | M+K1+ |
2 | C05604 | 218.0424 | 677 | 2-carboxy-2,3-dihydro-5,6-dihydroxyindole | M+Na1+ |
196.0605 | 144 | M+H1+ | |||
3 | C02470 | 206.0447 | 264 | Xanthurenic acid; xanthurenate | M+H1+ |
4 | C03232 | 184.9854 | 62 | 3-phosphonooxypyruvate; 3-phosphonooxypyruvic acid; 3-phosphohydroxypyruvate; 3-phosphohydroxypyruvic acid | M+H1+ |
5 | C06002 | 140.9957 | 68 | (S)-methylmalonate semialdehyde | M+K1+ |
103.0388 | 1215 | M+H1+ | |||
85.0282 | 837 | M-H2O+H1+ | |||
6 | C03684 | 220.0817 | 137 | 6-pyruvoyltetrahydropterin | M-H2O+H1+ |
238.0923 | 793 | M+H1+ | |||
7 | CE2102 | 357.1295 | 174 | Lipoyllysine | M+Na1+ |
8 | C05844 | 255.0652 | 665 | 5-L-glutamyl-taurine; 5-glutamyl-taurine | M+H1+ |
293.02 | 254 | M+K1+ | |||
238.0382 | 1022 | M-NH3+H1+ | |||
9 | CE4890 | 216.0995 | 475 | N-methylsalsolinol | M+Na1+ |
232.0735 | 130 | M+K1+ | |||
194.1178 | 217 | M+H1+ | |||
195.1209 | 268 | M(Cl3)+H1+ | |||
10 | C00624 | 190.071 | 252 | N-acetyl-L-glutamate; N-acetyl-L-glutamic acid | M+H1+ |
172.0604 | 250 | M-H2O+H1+ | |||
212.053 | 366 | M+Na1+ | |||
11 | CE1936 | 185.1649 | 703 | Spermine dialdehyde | M-H2O+H1+ |
203.1753 | 287 | M+H1+ | |||
12 | C03793 | 190.163 | 236 | N6,N6,N6-trimethyl-L-lysine | M(Cl3)+H1+ |
171.149 | 469 | M-H2O+H1+ | |||
211.1416 | 843 | M+Na1+ | |||
171.1491 | 544 | M-H2O+H1+ | |||
189.1599 | 305 | M+H1+ | |||
13 | C04244 | 222.0973 | 862 | 6-lactoyl-5,6,7,8-tetrahydropterin | M-H2O+H1+ |
223.0843 | 819 | M-NH3+H1+ | |||
262.0924 | 214 | M+Na1+ | |||
14 | C16604 | 232.0735 | 130 | 2-hydroxyiminostilbene | M+Na1+ |
15 | C04133 | 307.9908 | 239 | N-acetyl-l-glutamate 5-phosphate; n-acetyl-l-glutamyl 5-phosphate | M+K1+ |
292.0169 | 204 | M+Na1+ | |||
16 | C00086 | 98.9952 | 183 | Urea; carbamide | M+K1+ |
83.0213 | 112 | M+Na1+ | |||
17 | CE5536 | 202.0474 | 285 | Adrenochrome | M+Na1+ |
180.0655 | 243 | M+H1+ | |||
162.0549 | 1194 | M-H2O+H1+ | |||
181.0688 | 283 | M(Cl3)+H1+ | |||
18 | C07646 | 293.02 | 254 | Carboxyphosphamide; carboxycyclophosphamide | M+H1+ |
19 | C00049 | 134.0447 | 249 | L-aspartate | M+H1+ |
20 | C00214 | 226.0711 | 940 | Thymidine; deoxythymidine | M-NH3+H1+ |
243.0978 | 891 | M+H1+ | |||
265.0812 | 50 | M+Na1+ | |||
21 | C05931 | 248.0767 | 306 | N-succinyl-L-glutamate; (2S)-2-(3-carboxypropanoylamino) pentanedioic acid | M+H1+ |
22 | C01042 | 198.0373 | 300 | N-acetyl-l-aspartate | M+Na1+ |
158.0447 | 196 | M-H2O+H1+ | |||
176.0554 | 44 | M+H1+ | |||
23 | C05932 | 214.071 | 121 | N-succinyl-l-glutamate 5-semialdehyde; (2S)-2-(3-carboxypropanoylamino)-5-oxopentanoic acid | M-H2O+H1+ |
232.0817 | 1132 | M+H1+ | |||
24 | C00147 | 158.0447 | 190 | Adenine; 6-aminopurine | M+Na1+ |
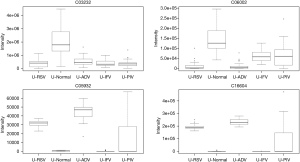
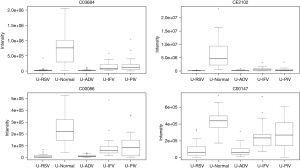
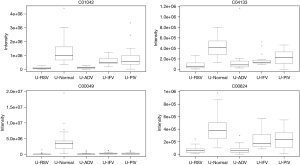
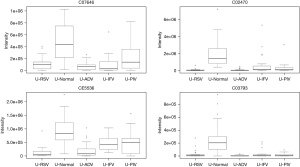
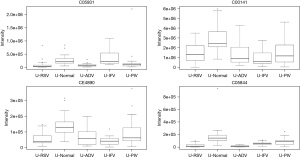
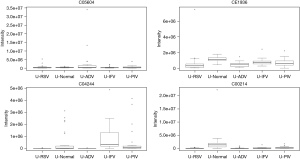
Pathways for identified metabolites
The significantly enriched pathways are shown in Table 2. The tyrosine metabolism and urea cycle/amino group metabolism are the top pathways enriched for identified metabolites. Moreover, the top six modules of our identified metabolites are shown in Table 3.
Table 2
Pathways | Overlap size | Pathway size | P value |
---|---|---|---|
Tyrosine metabolism | 13 | 42 | 0.00214 |
Urea cycle/amino group metabolism | 6 | 21 | 0.01558 |
Aspartate and asparagine metabolism | 9 | 35 | 0.01695 |
Carbon fixation | 2 | 3 | 0.01766 |
Lipoate metabolism | 2 | 3 | 0.01766 |
Methionine and cysteine metabolism | 4 | 13 | 0.02376 |
Arginine and proline metabolism | 4 | 14 | 0.03403 |
Alanine and aspartate metabolism | 4 | 14 | 0.03403 |
Pyrimidine metabolism | 5 | 19 | 0.0353 |
Drug metabolism - cytochrome P450 | 4 | 15 | 0.04751 |
Lysine metabolism | 3 | 10 | 0.04993 |
Table 3
Module | P value | Size | Members (ID) | Top pathways |
---|---|---|---|---|
Module_1 | 0.0015 | 16 | C00232, C03232, C05931, C02470, C00349, C00141, C00109, C00164, C05645, C00026, C03793, C01180, C00049, C06002, C05932, C01042 | (6, ‘Aspartate and asparagine metabolism’); (5, ‘Valine, leucine and isoleucine degradation’); (4, ‘Butanoate metabolism’) |
Module_2 | 0.0026 | 5 | C00364, C12739, C00147, C11736, C00214 | (2, ‘Pyrimidine metabolism’); (2, ‘Drug metabolism - other enzymes’); (1, ‘Purine metabolism’) |
Module_3 | 0.0205 | 11 | C00026, C03232, C02470, C00349, C00141, C00109, C00164, C05645, C03793, C00232, C06002 | (5, ‘Valine, leucine and isoleucine degradation’); (4, ‘Butanoate metabolism’); (3, ‘Tryptophan metabolism’) |
Module_4 | 0.0241 | 4 | C04244, C00835, C02953, C03684 | (2, ‘Biopterin metabolism’) |
Module_5 | 0.0333 | 4 | C05587, C07645, C07646, C05577 | (2, ‘Tyrosine metabolism’); (2, ‘Drug metabolism - cytochrome P450’) |
Module_6 | 0.0493 | 4 | C00355, C00268, C05604, C00822 | (4, ‘Tyrosine metabolism’); (1, ‘Tryptophan metabolism’); (1, ‘Biopterin metabolism’) |
Discussion
This study identified 948 metabolites using the Mummichog technique on the XCMS Online platform, of which 24 were identified as putative biomarkers. The aim of this study is to look across specific virus types and check whether body has the same response patterns to different viruses. In fact, this is a bit different from most current researches, which prefer to figure out the specific virus that causes the disease, explore its reproduction process, the pathogenic process, and then conduct research on treatment and drugs for a single virus, and of course, once it succeeds, it is a so-called effective drug, such as oseltamivir for the flu virus. However, this is a long, expensive and costly research with a high chance of failure. So, this paper tried to study from another aspect, if all viruses have the same pattern for human pathogenesis, one particular drug might be used to treat many kinds of viral infections. It was found that the human body really has one or more identical metabolic patterns for different viral infections in this study. We believe that such a study is some kinds of different from other common molecules studies that illustrate a certain point of a disease and also has some inspiration for future drug research.
We identified 24 typical metabolites were selected as potential markers and further differences in 2-oxoglutarate among the groups. Patients infected with RSV, ADV, and PIV showed upregulation, while those infected with IFV and PIV showed downregulation. Two-oxoglutaric acid is a precursor of glutamine and hydroxyproline, the latter being the most abundant amino acid of collagen (17). 2-oxoglutarate-dependent dioxygenase enzymes catalyze the hydroxylation of a wide range of metabolic processes, such as transcriptional regulation, nucleic acid modification/repair, fatty acid metabolism, and secondary metabolite biosynthesis in plant and animal development (18), including those of medicinally important antibiotics. Members of this family regulate the hypoxic response and epigenetic processes of the cells; demethylation of histones and DNA have been identified in mammalian cells (19). Hypoxia leads to acute lung injury and causes large numbers of deaths worldwide. Thus, 2-oxoglutarate could be a potential therapeutic target.
L-aspartate had a lower concentration in the pneumonia samples. During the progression of viral infection, L-aspartate may be helpful for recovery; a previous report showed that it reduced oxidative stress in mouse kidneys (20). Another review reported that L-aspartate reduced the levels of blood ammonia and helped in the prevention and treatment of hepatic encephalopathy. It has been proposed that aspartate serves as a neurotransmitter (21). However, glutamate alone accounts for neurotransmission at excitatory synapses in the hippocampus, with no role for aspartate. Hence, the significance of L-aspartate in infection and the self-inflammatory response needs further study.
In this module, several pathways were involved: aspartate and asparagine metabolism; alanine, leucine, and isoleucine degradation; and butanoate metabolism. As we know, the main pathogenic paramyxoviruses, such as PIV, RSV, measles, mumps, and IFV, belong to the orthomyxoviridae family. Hence, we speculate that there may be some changes in similar metabolites after infection by these viruses at a molecular level, irrespective of the specific type of viruses. However, this requires further study at the molecular level.
Because of the limited sample size of current study, further studies with larger, independent cohorts of patients are required to validate these findings. Further research is warranted on the mechanisms of IFV and the other three viruses.
Conclusions
To the best of our knowledge, this is the first study to elucidate the urine profiles of children with viral pneumonia and 31 age- and sex-matched NCs using LC-MS. We identified the typical metabolites and found that some connected pathways were significantly altered in children with viral pneumonia. We also identified 24 metabolites with the highest sensitivities as potential biomarkers. Furthermore, specially, our results suggests that three viruses, including ADV, RSV, and IFV, affect the systemic metabolome, but IFV does not. Two-oxoglutarate was upregulated, and aspartate was downregulated in the ADV, RSV, and IFV-infected samples. Our findings may lead to the discovery of new treatments for viral infections and bring us closer to the development of new antiviral drugs.
Acknowledgments
Funding: This study was supported by Beijing Key Discipline of Pediatric, Spleen, and Stomach Diseases Foundation (No. JingZhong II-8).
Footnote
Reporting Checklist: The authors have completed the MDAR reporting checklist. Available at https://tp.amegroups.com/article/view/10.21037/tp-23-199/rc
Data Sharing Statement: Available at https://tp.amegroups.com/article/view/10.21037/tp-23-199/dss
Peer Review File: Available at https://tp.amegroups.com/article/view/10.21037/tp-23-199/prf
Conflicts of Interest: All authors have completed the ICMJE uniform disclosure form (available at https://tp.amegroups.com/article/view/10.21037/tp-23-199/coif). The authors have no conflicts of interest to declare.
Ethical Statement: The authors are accountable for all aspects of the work in ensuring that questions related to the accuracy or integrity of any part of the work are appropriately investigated and resolved. The study was conducted in accordance with the Declaration of Helsinki (as revised in 2013). The study was approved by IRB of The Affiliated Hospital of Nanjing University of Traditional Chinese Medicine(No. 2013NL-KS15) and Beijing Children’s Hospital was informed and agreed the study. Informed consent was taken from all the patients’ guardians.
Open Access Statement: This is an Open Access article distributed in accordance with the Creative Commons Attribution-NonCommercial-NoDerivs 4.0 International License (CC BY-NC-ND 4.0), which permits the non-commercial replication and distribution of the article with the strict proviso that no changes or edits are made and the original work is properly cited (including links to both the formal publication through the relevant DOI and the license). See: https://creativecommons.org/licenses/by-nc-nd/4.0/.
References
- Zhou Y, Zhang L, Xie YH, et al. Advancements in detection of SARS-CoV-2 infection for confronting COVID-19 pandemics. Lab Invest 2022;102:4-13. [Crossref] [PubMed]
- Zhang Y, Liu Y, Li M, et al. Timely treatment and higher compliance to traditional Chinese medicine: New influencing factors for reducing severe COVID-19 based on retrospective cohorts in 2020 and 2021. Pharmacol Res 2022;178:106174. [Crossref] [PubMed]
- Yang Z, Liu Y, Wang L, et al. Traditional Chinese medicine against COVID-19: Role of the gut microbiota. Biomed Pharmacother 2022;149:112787. [Crossref] [PubMed]
- Song J, Zhao J, Cai X, et al. Lianhuaqingwen capsule inhibits non-lethal doses of influenza virus-induced secondary Staphylococcus aureus infection in mice. J Ethnopharmacol 2022;298:115653. [Crossref] [PubMed]
- Gu W, Zhao Y, Yang L, et al. A new perspective to improve the treatment of Lianhuaqingwen on COVID-19 and prevent the environmental health risk of medication. Environ Sci Pollut Res Int 2022;29:74208-24. [Crossref] [PubMed]
- Ji Z, Hu H, Qiang X, et al. Traditional Chinese Medicine for COVID-19: A Network Meta-Analysis and Systematic Review. Am J Chin Med 2022;50:883-925. [Crossref] [PubMed]
- Bi X, Liu W, Ding X, et al. Proteomic and metabolomic profiling of urine uncovers immune responses in patients with COVID-19. Cell Rep 2022;38:110271. [Crossref] [PubMed]
- Liu X, Li J, Hao X, et al. LC-MS-Based Urine Metabolomics Analysis for the Diagnosis and Monitoring of Medulloblastoma. Front Oncol 2022;12:949513. [Crossref] [PubMed]
- Tan J, Li Y, Gao D, et al. A liquid chromatography-mass spectroscopy-based untargeted metabolomic study of the rat cochlear nucleus at various stages of maturity. Hear Res 2022;426:108645. [Crossref] [PubMed]
- Doğan HO, Şenol O, Karadağ A, et al. Metabolomic profiling in ankylosing spondylitis using time-of-flight mass spectrometry. Clin Nutr ESPEN 2022;50:124-32. [Crossref] [PubMed]
- Bosman P, Pichon V, Acevedo AC, et al. Untargeted Metabolomic Approach to Study the Impact of Aging on Salivary Metabolome in Women. Metabolites 2022;12:986. [Crossref] [PubMed]
- Montis A, Souard F, Delporte C, et al. Targeted and Untargeted Mass Spectrometry-Based Metabolomics for Chemical Profiling of Three Coffee Species. Molecules 2022;27:3152. [Crossref] [PubMed]
- Bauermeister A, Mannochio-Russo H, Costa-Lotufo LV, et al. Mass spectrometry-based metabolomics in microbiome investigations. Nat Rev Microbiol 2022;20:143-60. [Crossref] [PubMed]
- Zhao JJ, Zhang Y, Wang XC, et al. A new platform for untargeted UHPLC-HRMS data analysis to address the time-shift problem. Anal Chim Acta 2022;1193:339393. [Crossref] [PubMed]
- Ito M, Hano T, Kono K, et al. Desorption of polycyclic aromatic hydrocarbons from polyethylene microplastics in two morphologically different digestive tracts of marine teleosts: Gastric red seabream (Pagrus major) and agastric mummichog (Fundulus heteroclitus). Environ Pollut 2022;308:119589. [Crossref] [PubMed]
- Amiri-Dashatan N, Yekta RF, Koushki M, et al. Metabolomic study of serum in patients with invasive ductal breast carcinoma with LC-MS/MS approach. Int J Biol Markers 2022;37:349-59. [Crossref] [PubMed]
- Kviatkovsky SA, Hickner RC, Ormsbee MJ. Collagen peptide supplementation for pain and function: is it effective? Curr Opin Clin Nutr Metab Care 2022;25:401-6. [Crossref] [PubMed]
- Liu H, Xie Y, Wang X, et al. Exploring links between 2-oxoglutarate-dependent oxygenases and Alzheimer's disease. Alzheimers Dement 2022;18:2637-68. [Crossref] [PubMed]
- Muzammal M, Di Cerbo A, Almusalami EM, et al. In Silico Analysis of the L-2-Hydroxyglutarate Dehydrogenase Gene Mutations and Their Biological Impact on Disease Etiology. Genes (Basel) 2022;13:698. [Crossref] [PubMed]
- Jain A, Sharma BC, Mahajan B, et al. L-ornithine L-aspartate in acute treatment of severe hepatic encephalopathy: A double-blind randomized controlled trial. Hepatology 2022;75:1194-203. [Crossref] [PubMed]
- Teleanu RI, Niculescu AG, Roza E, et al. Neurotransmitters-Key Factors in Neurological and Neurodegenerative Disorders of the Central Nervous System. Int J Mol Sci 2022;23:5954. [Crossref] [PubMed]
(English Language Editor: D. Fitzgerald)