Association of gut microbiota, plasma and fecal metabolite profiles with intellectual development in school-age children
Highlight box
Key findings
• Certain plasma metabolites concentrations but not the gut microbiota composition nor fecal metabolites are correlated with intelligence in normal school-age children.
What is known and what is new?
• Altered microbiome composition is correlated with cognitive function in early life.
• Specific plasma metabolites are significantly associated with processing speed index of children aged 6–9 years.
What is the implication, and what should change now?
• These findings appear to provide a greater insight into the underlying mechanisms of the gut-microbiota-brain axis for future research.
Introduction
The number of microbes inhabiting in the human gastrointestinal tract has been estimated to exceed 1014 and the types include bacteria, fungi, viruses, and protozoa. These bacterial cells can reach a density of up to 1012 per mL, which is the highest bacterial density of any known microbial ecosystem (1). Observational findings from the past two decades have suggested that the intestinal microbiota may play a vital role in regulating human health (2). A growing body of evidence has suggested a role for the microbiota in the etiology of mental disorders. The microbiota-gut-brain axis has been shown to be a bidirectional communication system that is connected via neural, immune, endocrine, and metabolic pathways (3).
Intellectual development in children plays a significant role in shaping later life and is inversely associated with several somatic health outcomes in middle and later life (4-6). Animal studies have revealed that the gut microbiota influences brain development and cognitive function (7,8). Moreover, human studies have linked altered microbiome composition to changes in cognitive function. Several factors that influence the development and composition of the intestinal microbiota and brain development and function have been identified, including gestational age at birth and feeding method (breast milk or formula) (9). Cohort studies have reported that the infant microbiome is correlated with cognitive function and brain volume at 2–3 years of age (10-12), at which most bacterial groups have reached a stable population size. However, some microbial groups can take a longer period to reach such a steady state (13). Despite these findings, few studies have assessed the relationship between the microbiota and cognitive function in young children, whose cognitive outcomes are more advanced and easier to evaluate than those of infants and whose gut microbiota is more stable. The role of blood metabolomics, which can be used to characterize many small molecules in the context of physiological stimuli or in disease states (14,15), in brain function has been previously established in the context of Alzheimer’s disease, mild cognitive impairment, and cognitive decline (16-18). Meanwhile, gut bacterial metabolite groups are suggested to have potential protective or aggravating effect on brain function (19). However, little is currently known about the association of specific blood or fecal metabolites with childhood intelligence.
This current study explored the association of the gut microbiota, plasma and fecal metabolites with intelligence assessed by the Wechsler Intelligence Scale for Children-Fourth Edition (WISC-IV) in Chinese children aged 6–9 years. We present this article in accordance with the STROBE reporting checklist (available at https://tp.amegroups.com/article/view/10.21037/tp-22-610/rc).
Methods
Participant selection
This cross-sectional study enrolled healthy, typically developing children aged 6–9 years in urban Guangzhou, China from 2015 to 2017, as reported previously (20). Five hundred and twenty-one children who met the inclusion criteria were recruited through invitation letters, advertisements, and referrals. The exclusion criteria were: (I) twin and preterm births, (II) history of serious disease or mental or physical disability, (III) receiving any dietary intervention or medical treatment, and (IV) history of receiving antibiotic, probiotic, or prebiotic treatment or any other medical treatment within the 3 months prior to the study. Children lacking intelligence test data and gut microbiota or plasma/fecal metabolite measurements data were not included. A total of 452 children were included after applying the exclusion criteria. Furthermore, 410, 446 and 401 participants were included in the final analyses of microbial and plasma/fecal metabolic profiles, respectively (Figure S1). This study was conducted in accordance to the Declaration of Helsinki (as revised in 2013) and was approved by the ethics committee of the School of Public Health at Sun Yat-sen University (No. 201549). Written informed consent was obtained from parents or legal guardians of the participants.
Data collection
Plasma and fecal sample collection
Stool specimens from 90% of the participants were collected during the assessment of intelligence. The samples were stored at −80 ℃ within 10 min of collection until DNA extraction as described previously (21). Stool samples from the remaining 10% of the participants were collected at home with the assistance of the guardians and delivered to research facility in coolers with ice packs within 12 hours.
Fasting blood samples were collected by registered nurses into a tube with anticoagulant and delivered to laboratories on ice within 2 hours of collection. Plasma samples were collected after centrifugation and frozen at −80 ℃ until used for testing.
Measurement of gut microbiota
Gut microbial data were obtained from fecal samples using previously described DNA extraction and high-throughput sequencing methods (20). First, DNA was extracted from 250-mg stool samples using a QIAamp Fast DNA Stool Mini kit (Qiagen, Hilden, Germany). Next, the DNA was amplified by a two-step polymerase chain reaction (PCR) using the 16S rRNA gene primers 341F (5'-CCT AYG GGR BGC ASC AG-3') and 806R (5'-GGA CTA CNN GGG TAT CTA AT-3'), targeting the bacterial V3-V4 region. Thermal cycling conditions: 1 min at 98 ℃; 30 cycles of 10 s at 98 ℃, 30 s at 50 ℃, and 60 s at 72 ℃; and 5 min at 7 ℃. Each sample was randomly fragmented and libraries were constructed using the TruSeq DNA PCR-Free Sample Preparation Kit with dual indexing and sequenced on the HiSeq 2500 platform (Illumina, USA) to produce 250-bp paired-end reads. After filtering with FLASH (v1.2.7, http://ccb.jhu.edu/software/FLASH/), paired-end reads were assigned to each sample using their unique barcodes. Chimeric sequences were detected and putative sequences were removed. Operational taxonomic unit (OTU) grouping was performed using the UPARSE package (v7.0.1001, http://drive5.com/uparse/) with a standard similarity threshold of 97%. OTUs were taxonomically assigned by the Mothur classifier at a confidence threshold of 80%, based on the Ribosomal Database Project database (22). Taxonomic information was annotated for each representative sequence against the SILVA database (version 128, https://www.arb-silva.de). Alpha-diversity measures were calculated based on the rarefied OTU counts. The indicators of alpha-diversity included the abundance-based coverage estimator (ACE), Chao1 index, Simpson and Shannon index. All samples were sequenced together and at the same laboratory. Bioinformatics analysis was performed by Novogene Bioinformatics Technology Co., Ltd. (Tianjin, China).
Measurement of plasma and fecal metabolomic
Targeted metabolomic analysis was conducted using the Q300 Metabolite Assay Kit (Human Metabolomics Institute, Inc., Shenzhen, China) in accordance with the published method (23), with minor modifications. Details for chemicals, reagents and steps of targeted metabolomics analysis were described in Appendix 1. In brief, 25 µL plasma or fecal extract was added to a 96-well plate and transferred to the Biomek 4000 workstation (Beckman Coulter, Inc., Brea, CA, USA) for partial internal standards adding and vortex. Derivatization was carried out (60 min, 30 ℃) for supernatant obtained after centrifuging (Allegra X-15R, Beckman Coulter, Inc., Indianapolis, IN, USA). Then, internal standards for each well were added after diluting by methanol solution. Serial dilutions of the derivatized stock standards were added to the remaining wells. Finally, plasma and fecal metabolites were measured using ultra-performance liquid chromatography coupled with tandem mass spectrometry (UPLC-MS/MS; ACQUITY UPLC-Xevo TQ-S, Waters Corp., Milford, MA, USA). MassLynx 4.1 software was used to controlled the entire LC-MS system. All chromatographic separations were performed with an ACQUITY BEH C18 column (1.7 µm, 100 mm × 2.1 mm internal dimensions) (Waters Corp.). TMBQ (v1.0, Human Metabolomics Institute, Inc.) was used to process the raw data files generated by UPLC-MS/MS and perform peak integration, calibration, and quantification for each metabolite.
Assessment of intelligence in children
The Chinese version of the WISC-IV was used to assess cognitive development outcomes in children. The WISC-IV has 15 subtests, 10 of which are core subtests used in this study. Raw scores were converted into scaled scores based on an age group-specific metric with a mean of 10 and standard deviation of 3, as described in the WISC-IV manual (24). The scaled scores were then used to derive four composite scores: Verbal Comprehension Index (VCI), Perceptual Reasoning Index (PRI), Working Memory Index (WMI), and Processing Speed Index (PSI). These four indexes were summed to calculate the composite Full-Scale Intelligence Quotient (FSIQ).
Covariates
Participant characteristics, such as household income, parental education level, use of calcium and multivitamin supplements, and method of delivery were collected through face-to-face interviews conducted by trained interviewers. A quantitative food frequency questionnaire (FFQ), in which 79 food items were classified into 20 food groups, was used to assess the diets of the children over the year preceding the study, as described previously (20). The China Food Composition Table [2009] (25) was used to calculate dietary energy and fiber intake. Physical activity level was assessed using a 3-day physical activity questionnaire and calculated by combining the metabolic equivalent scores (MET; Kcal/kg/h) for each type of physical activity after multiplying each by its duration per day (h/d).
Statistical analysis
Basic descriptive characteristics were presented as the median and interquartile range for continuous variables and count and percentage for categorical variables. Analysis of variance (ANOVA) was used for continuous data. Categorical data was analyzed by Pearson’s Chi-square test and Fisher’s exact test. For the microbial sequencing data, low-abundance microbiota compositions (<0.01%) were filtered out, leaving genera and species in >10% of the samples. Restricted maximum likelihood (REML) models implemented in the software package omics-data-based complex trait analysis (OSCA v.0.45, https://yanglab.westlake.edu.cn/software/osca) were used to assess the association of the overall microbial and metabolic profiles with the cognitive measures (26). REML evaluates whether the microbial or metabolic profile is related to the test variables in terms of the proportion of explained variance. Covariates that were potential confounders for these analyses were assessed using a separate REML model for each potential confounding variable: age and sex of the child, type of birth (vaginal delivery vs. caesarean section), calcium supplement use, multivitamin use, maternal and paternal education level, household income, physical activity level, and energy and fiber intake. Participants with incomplete data mentioned above were not included in the REML model. Next, the cognition REML models were rerun to account for the identified confounding variables.
The multivariate methods with unbiased variable selection in R (MUVR) algorithm was used to identify key gut microbiota genera and species, plasma and fecal metabolites for significant relationships (27). Read counts of microbiota data were normalized using the variance stabilizing transformation in the DESeq2 R package. For the metabolomic data, plasma and fecal metabolite concentrations were log10-transformed using MetaboAnalyst 5.0 (https://www.metaboanalyst.ca) (28). When running the MUVR models, partial least squares (PLS) regression was used with nRep=100, nOuter=8, and varRatio =0.8 for the repeated double cross-validation procedure. For each of the inner training models, variables were ranked by de facto standard techniques such as variable importance of projection for PLS analysis. Then, the top 10 variables associated with intelligence were identified and spearman correlation analysis was conducted. Subsequently, multiple linear analyses were carried out after adjusting for age, sex, covariates identified using REML models (P<0.05) and controlling for the false discovery rate (FDR) using the Benjamini-Hochberg method (29).
All statistical analyses were carried out using R software (v4.0.4) and IBM SPSS Statistics software (v26, SPSS Inc., Chicago, IL, USA). All P values were calculated using two-sided tests and P values <0.05 were considered statistically significant.
Results
Participant characteristics
A total of 452 participants (410 for microbiota composition analysis, 446 and 401 for plasma and fecal metabolites analyses) were included in this study. Demographic characteristics are summarized in Table 1. The median [interquartile range] age at WISC-IV assessment was 8.1 [1.5] years. Energy intake and physical activity level were higher for males than for females (P<0.001). Females scored higher in PSI than males (P=0.002). No significant differences were observed in age, fiber intake, delivery mode, household income, parental education level, use of calcium and multivitamin supplements, or other intelligence test scores (P: 0.088–0.984).
Table 1
Characteristics | Total (n=452) | Males (n=257) | Females (n=195) | P† |
---|---|---|---|---|
Age (years) | 8.1 [1.5] | 8.0 [1.5] | 8.2 [1.4] | 0.428 |
Energy intake (Kcal/day)‡ | 1,363 [556] | 1,436 [563] | 1,268 [552] | <0.001* |
MET (Kcal/day/kg)‡ | 39.1 [4.9] | 40.0 [5.4] | 38.2 [4.6] | <0.001* |
Fiber intake (g)‡ | 6.9 [4.0] | 7.0 [3.9] | 6.6 [4.1] | 0.777 |
Delivery mode | ||||
Vaginal | 229 (50.7) | 121 (47.1) | 108 (55.4) | 0.088 |
Cesarean | 223 (49.3) | 136 (52.9) | 87 (44.6) | |
Household income | ||||
<8,000 Yuan/month | 77 (17.0) | 44 (17.1) | 33 (16.9) | 0.984 |
8,000–15,000 Yuan/month | 141 (31.2) | 82 (31.9) | 59 (30.3) | |
>15,000 Yuan/month | 154 (34.1) | 86 (33.5) | 68 (34.9) | |
No response | 80 (17.7) | 45 (17.5) | 35 (17.9) | |
Maternal education level | ||||
Secondary or less | 173 (38.3) | 97 (37.7) | 76 (39.0) | 0.126 |
University | 238 (52.7) | 130 (50.6) | 108 (55.4) | |
Postgraduate or above | 39 (8.6) | 28 (10.9) | 11 (5.6) | |
No response | 2 (0.4) | 2 (0.8) | 0 (0.0) | |
Paternal education level | ||||
Secondary or less | 180 (39.8) | 101 (39.3) | 79 (40.5) | 0.703 |
University | 209 (46.2) | 116 (45.1) | 93 (47.7) | |
Postgraduate or above | 59 (13.1) | 37 (14.4) | 22 (11.3) | |
No response | 4 (0.9) | 3 (1.2) | 1 (0.5) | |
Use of calcium supplement | ||||
Yes | 183 (40.5) | 111 (43.2) | 72 (36.9) | 0.209 |
No | 269 (59.5) | 146 (56.8) | 123 (63.1) | |
Use of multivitamin supplement | ||||
Yes | 78 (17.3) | 45 (17.5) | 33 (16.9) | 0.901 |
No | 374 (82.7) | 212 (82.5) | 162 (83.1) | |
VCI | 108 [22] | 108 [20] | 108 [20] | 0.471 |
PRI | 106 [16] | 108 [15] | 106 [16] | 0.648 |
WMI | 100 [16] | 100 [18] | 100 [15] | 0.967 |
PSI | 102 [15] | 102 [15] | 104 [15] | 0.002* |
FSIQ | 106 [15] | 106 [15] | 106 [16] | 0.737 |
Data are given as median [interquartile range] or number (percentage). Physical activity level is represented by MET. *, P<0.05. †, comparison between males and females. ‡, n=451 (nmissing=1). MET, metabolic equivalent; VCI, Verbal Comprehension Index; PRI, Perceptual Reasoning Index; WMI, Working Memory Index; PSI, Processing Speed Index; FSIQ, Full-Scale Intelligence Quotient.
Correlation between microbiota composition and intelligence scores
After quality and content filtering, 114 genera and 97 species were retained for downstream analyses. After adding covariates significant associated with microbiota composition (Table S1), cognition REML models showed that the overall microbiota composition was not associated with either subscale or total cognitive function [V(O)/Vp: 0–4.86%, P: 0.059–0.500, Table 2] at genus and species level. Among the 28 genera and 18 species identified using MUVR, spearman correlation and multiple linear analysis were carried out (Figures S2,S3). No significant correlation was found between intelligence scores with selected microbiota counts at genus and species level after adjusting for age, covariates identified in REML models and FDR correction (β =−0.14 to 0.16, PFDR=0.058–0.993). As shown in Figure S4, no significant correlations were identified between the Shannon, Simpson, ACE, and Chao1 indexes and intelligence test scores (β =−0.09 to 0.02, PFDR=0.720–0.898) after adjusting for confounders.
Table 2
Phenotype | V(O)/Vp (% ) | SE (%) | P |
---|---|---|---|
Microbiota at genus level | |||
VCI | 2.85 | 3.64 | 0.188 |
PRI | 0.18 | 1.40 | 0.438 |
WMI | 0.00 | 1.40 | 0.500 |
PSI | 0.00 | 1.89 | 0.500 |
FSIQ | 1.20 | 2.13 | 0.207 |
Microbiota at species level | |||
VCI | 1.16 | 3.10 | 0.339 |
PRI | 4.64 | 3.88 | 0.087 |
WMI | 0.00 | 3.42 | 0.500 |
PSI | 4.86 | 3.78 | 0.059 |
FSIQ | 1.67 | 3.47 | 0.310 |
Alpha-diversity | |||
VCI | 0.06 | 0.66 | 0.475 |
PRI | 0.00 | 0.45 | 0.500 |
WMI | 0.00 | 0.53 | 0.500 |
PSI | 0.00 | 0.76 | 0.500 |
FSIQ | 0.18 | 0.74 | 0.396 |
Plasma metabolite† | |||
VCI | 0.00 | 2.09 | 0.500 |
PRI | 8.09 | 5.29 | 0.060 |
WMI | 0.00 | 2.09 | 0.500 |
PSI | 8.64 | 5.07 | 0.008* |
FSIQ | 0.00 | 1.94 | 0.500 |
Fecal metabolite | |||
VCI | 2.12 | 2.90 | 0.121 |
PRI | 2.04 | 3.64 | 0.290 |
WMI | 0.00 | 2.27 | 0.500 |
PSI | 1.12 | 1.92 | 0.177 |
FSIQ | 0.00 | 1.56 | 0.500 |
Model of microbiota at genus level composition adjusted for sex, maternal education level, physical activity level. Model of microbiota at species level composition adjusted for sex, fiber intake. Model of alpha-diversity adjusted for fiber intake. Model of plasma metabolite adjusted for age, sex, maternal and paternal education level, household income, energy intake. Explained variance estimated using REML models. *, P<0.05. †, N=444 in model of plasma metabolite. V(O)/Vp, explained variance; SE, standard error; VCI, Verbal Comprehension Index; PRI, Perceptual Reasoning Index; WMI, Working Memory Index; PSI, Processing Speed Index; FSIQ, Full-Scale Intelligence Quotient; REML, restricted maximum likelihood.
Correlation of plasma metabolites with intelligence scores
A total of 200 plasma metabolites were identified. Of the assessed covariates, age, sex, energy intake, parental education level and household income showed a significant association with the plasma metabolite in REML models (Table S1) and were added in further analysis as covariates. Only one subscale of intelligence test, PSI was found to be associated with overall plasma metabolite concentrations [V(O)/Vp: 8.64%, P=0.008, Table 2]. Among the 34 metabolites identified using MUVR (Figure 1), 24 metabolites, including three kinds of carnitines, four amino acids, one benzenoid, one carbohydrate, seven fatty acids (FAs), and eight organic acids were significantly correlated with intelligence scores (r=−0.17 to 0.15, P: <0.001–0.042, Figure 2A). After adjusting for covariates selected in REML models and correcting for FDR, the plasma concentrations of benzoic acid, azelaic acid, adipic acid, suberic acid, and malonic acid were found to be positively associated with PSI (β =0.16–0.19, PFDR=0.006–0.024, Figure 2B), whereas pyruvic acid was negatively associated with both PSI and FSIQ (β =−0.17 to −0.14, PFDR=0.014–0.030). The co-occurrence network of metabolites demonstrated that malonic acid, linoleic acid, azelaic acid and adipic acid were the core nodes in key plasma metabolites (Figure 3).
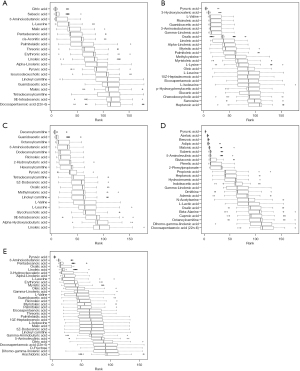
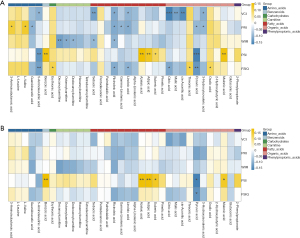
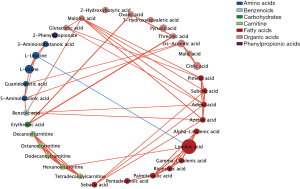
Correlation of fecal metabolites with intelligence scores
We identified a total of 212 fecal metabolites. None of the covariates assessed in REML models were found to be significantly associated with the fecal metabolites and included in cognition REML model (Table S1). No subscale and total scores of intelligence test were found to be associated with overall fecal metabolite concentrations [V(O)/Vp: 0–2.12%, P: 0.121–0.500, Table 2] in cognition REML model. Among the 35 metabolites identified using MUVR, 23 metabolites, including four kinds of amino acids, one benzenoids, four bile acids, one carbohydrate, one carnitine, eight FAs, one imidazole, and three organic acids were significantly correlated with intelligence scores (r=−0.13 to 0.16, P: <0.001–0.039, Figure S5A). No significant correlation remained after adjusting for age, sex and correcting for FDR as shown in Figure S5B (β =−0.14 to 0.16, PFDR=0.096–0.996).
Discussion
The current study suggests that the concentrations of several metabolites in blood (e.g., adipic acid and suberic acid), but not the overall microbiota composition and fecal metabolites, are associated with intellectual development in children aged 6–9 years.
Association of gut microbiota composition with intelligence in children
Numerous animal model studies have identified significant interactions between an altered gut microbiota and changes in cognitive behavior (8). Germ-free rodents have displayed impaired short-term recognition and working memory and altered social behavior (30). Despite an increasing interest in the influence of gut microbiota on human brain development, most previous studies have focused on adults, infants, and young children. A correlational study of 20 obese and 19 non-obese adults showed significant correlations between the Shannon index and brain microstructure, as shown by magnetic resonance imaging (31). In a cohort study by Carlson et al. that included 89 typically developing children, higher alpha-diversity, as assessed by the Chao1 index, the total number of observed species, and Faith’s phylogenetic diversity of gut microbiota at 1 year of age were associated with lower overall composite, visual reception, and expressive language scores at 2 years of age (12). Streit et al. similarly reported significantly negative correlations between alpha-diversity metrics (e.g., Shannon index and Faith’s phylogenetic diversity index) and cognition as assessed by the Wechsler Preschool and Primary Scale of Intelligence in 308 children aged 45 months (11). However, a study conducted by Sordillo et al. did not observe any statistically significant associations between the Ages & Stages Questionnaire (ASQ-3) score and gut microbial diversity in 3-year-old children (32). Similarly, no associations were observed between cognitive performance and gut microbiota diversity in this current study.
In terms of microbial abundance, a greater relative abundance of specific microbes such as Actinobacteria, Prevotella, Verrucomicrobia, and Lentisphaerae have been reported to be associated with enhanced cognitive flexibility and executive function in adults (33). Interventional studies to improve cognition or brain function in adults have generally resulted in improved visuospatial memory, verbal learning, memory, and attentional vigilance (33). A cross-sectional study of 89 1-year-old infants reported that infants with a greater relative abundance of Bacteroides demonstrated improved subsequent neurodevelopmental performance in early learning and cognition (12). Similarly, a cohort study of 309 children showed that a decreased abundance of Bacteroides at 3–6 months of age was associated with reduced communication, personal, and social scores as assessed by the ASQ-3 at 3 years of age (32). Additionally, taxa within the order Clostridiales were found to have lower abundance in normally developing children. A recent study of 308 children aged 45 months identified a strong negative association between cognitive function and the relative abundance of an unidentified genus of the family Enterobacteriaceae (11). Several observational and clinical studies in elderly individuals have also reported a significant relationship between altered proportions of certain bacteria (e.g., Firmicutes and Proteobacteria) and mild cognitive impairment (34,35). However, this study did not identify any significant correlations between intelligence scores and specific gut microbes on genus and species level. Differences in the participants’ age and ethnicity, method of brain function evaluation, type of gene sequencing used for microbiota composition assessment, study design, and the covariates applied for adjustment may collectively contribute to the heterogeneity of these studies.
Association of circulating metabolites with intelligence in children
This study identified specific circulating metabolites that are significantly correlated with PSI in children. Processing speed is defined as the speed at which an individual perceives and reacts to stimuli with reasonable accuracy. Faster processing speed is thought to improve cognitive and academic progression by allowing for greater allocation of attention to higher-level tasks in the context of early skill and knowledge acquisition (36). Most of the metabolites identified are medium-chain FAs, which can permeate the blood-brain barrier and be oxidized within the brain (37). Higher plasma concentrations of adipic acid have been shown to be significantly associated with better PSI. Adipic acid is a dicarboxylic acid and has been shown to inhibit the activity of the enzyme gamma-amino butyric acid (GABA) transaminase in mouse brains, resulting in an increase in cerebral GABA concentrations in in vitro systems (38). GABA is the principal inhibitory neurotransmitter in the human nervous system and plays a fundamental functional role in the central nervous system (39). Previous studies have noted the importance of GABA as a modulator of memory encoding, suggesting that greater frontal GABA concentrations may be associated with superior cognitive performance in elderly population (40). However, no correlations were identified between GABA concentrations and intelligence scores in the present study while plasma levels of GABA may reflect GABA activity in brain. Another dicarboxylic acid, suberic acid, was found to be positively correlated with PSI in this study; the concentration of suberic acid has previously been reported as a good discriminator between children with autism spectrum disorder and neurotypical children (41). Organic acids are important metabolites and play a role in the major metabolic pathways of FAs, proteins, and neurotransmitters and in several pathways involved in energy production, oxidative damage, and intestinal dysbiosis (42). Organic acid metabolic disorders can lead to the accumulation of toxic metabolites, energy synthesis disorders, mitochondrial dysfunction, and neuronal cell apoptosis, resulting in structural damage to the brain and nerves and ganglioside and synaptic plasticity abnormalities. This can lead to decreased learning and memory abilities and even intellectual disabilities (43). An in vitro study showed that malonic acid exerts anti-inflammatory effects and may protect against inflammatory reactions caused by the activation of microglia, which can lead to neurodegeneration and cause diseases such as Alzheimer’s and Parkinson’s disease (44). A positive correlation between malonic acid concentrations and PSI was also identified, suggesting that malonic acid may benefit cognitive development. Further studies are required to validate the role and mechanism of these metabolites in shaping neurobiological function.
Association of fecal metabolites with intelligence in children
Metabolites transformed by gut microbiota such as short-chain FAs can act on the blood-brain barrier, directly on brain neurons. These microbial-derived metabolites with signaling functions are essential to brain function (45). Changes in fecal levels of short-chain FAs, amino acids, polyphenolic and other metabolites were reported in patients with neurodevelopmental and neurodegenerative disorders such as autism spectrum disorder, Alzheimer’s and Parkinson’s disease (19). For cognitive development, no significant correlations between specific gut microbes and intelligence scores were identified in this study. And no evidence has been found to indicate the fecal metabolites, which largely reflects gut microbial composition and its function (46), are related to children’s intelligence performance. Based on the theory of microbiota-gut-brain axis, further research should investigate whether fecal metabolome is associated with brain function and intellectual development.
Strengths and limitations
In contrast to previous studies on the relationship between the intestinal microbiota and cognitive development of 1–3-year-old infants, this current study focused on children aged 6–9 years. Older children were chosen for the study as their microbiota composition is thought to be more stable and their cognitive outcomes are more advanced and easier to evaluate. However, there are several limitations in this study. First, the assessment of the infant gut microbiota at a single time-point in this study does not fully capture the early-life evolution of the dynamic microbiota, which could be an important factor that affects neurocognitive outcomes. Second, multimodal approaches using imaging and other tools might be better suited to exploring the relationship between gut microbes and intelligence than the single intelligence test used in this study (10). Third, although multiple confounders were adjusted, other factors that were not taken into account in the current study, such as allergic status, may also be associated with the gut microbiota composition or intelligence development (47). As such, certain confounding biases may have been overlooked. Fourth, our analyses only included children aged 6–9 years living in urban Guangzhou, China. Relatively high level of subjects’ homogeneity such as region and parental education level in the current study might play a role in the lack of associations. Therefore, the results of this study should be interpreted with caution when being considered for different populations. Increasing the diversity of the subjects in future research will be needed, including family education background and regions with different dietary habits, to enhance the extrapolation of the results in this study. Lastly, around 10% of fecal samples were not collected on site. However, sensitivity analysis excluding these samples revealed similar association (data not shown), which suggests that different methods of feces collection have no impact on our findings.
Conclusions
In conclusion, this study has demonstrated an association between specific plasma metabolites and IQ in children aged 6–9 years.
Acknowledgments
We are grateful to the study participants. We want to thank Huanchang Yan, Yuting Hao, Yongxin Chen, Jiahua Zhang, Wenyi Guan, Ye Tong, Zhicong Zeng and Gaopei Huang from School of Public Health of Southern Medical University for their help in sample collection.
Funding: This study was supported by the Natural Science Foundation of Guangdong Province, China (No. 2021A1515011163), the Guangzhou Science and Technology Planning Project (No. 202102020967), and the National Natural Science Foundation of China (No. 81903347).
Footnote
Reporting Checklist: The authors have completed the STROBE reporting checklist. Available at https://tp.amegroups.com/article/view/10.21037/tp-22-610/rc
Data Sharing Statement: Available at https://tp.amegroups.com/article/view/10.21037/tp-22-610/dss
Conflicts of Interest: All authors have completed the ICMJE uniform disclosure form (available at https://tp.amegroups.com/article/view/10.21037/tp-22-610/coif). KZ and GX are current employees of Human Metabolomics Institute, Inc. The other authors have no conflicts of interest to declare.
Ethical Statement: The authors are accountable for all aspects of the work in ensuring that questions related to the accuracy or integrity of any part of the work are appropriately investigated and resolved. The study was conducted in accordance with the Declaration of Helsinki (as revised in 2013). The study was approved by the ethics committee of the School of Public Health at Sun Yat-sen University (No. 201549) and informed consent was obtained from parents or legal guardians of the participants before enrollment.
Open Access Statement: This is an Open Access article distributed in accordance with the Creative Commons Attribution-NonCommercial-NoDerivs 4.0 International License (CC BY-NC-ND 4.0), which permits the non-commercial replication and distribution of the article with the strict proviso that no changes or edits are made and the original work is properly cited (including links to both the formal publication through the relevant DOI and the license). See: https://creativecommons.org/licenses/by-nc-nd/4.0/.
References
- Thursby E, Juge N. Introduction to the human gut microbiota. Biochem J 2017;474:1823-36. [Crossref] [PubMed]
- Fan Y, Pedersen O. Gut microbiota in human metabolic health and disease. Nat Rev Microbiol 2021;19:55-71. [Crossref] [PubMed]
- Cryan JF, O’Riordan KJ, Cowan CSM, et al. The Microbiota-Gut-Brain Axis. Physiol Rev 2019;99:1877-2013. [Crossref] [PubMed]
- Koenen KC, Moffitt TE, Roberts AL, et al. Childhood IQ and adult mental disorders: a test of the cognitive reserve hypothesis. Am J Psychiatry 2009;166:50-7. [Crossref] [PubMed]
- Dobson KG, Chow CH, Morrison KM, et al. Associations Between Childhood Cognition and Cardiovascular Events in Adulthood: A Systematic Review and Meta-analysis. Can J Cardiol 2017;33:232-42. [Crossref] [PubMed]
- Calvin CM, Batty GD, Der G, et al. Childhood intelligence in relation to major causes of death in 68 year follow-up: prospective population study. BMJ 2017;357:j2708. [Crossref] [PubMed]
- Diaz Heijtz R, Wang S, Anuar F, et al. Normal gut microbiota modulates brain development and behavior. Proc Natl Acad Sci U S A 2011;108:3047-52. [Crossref] [PubMed]
- Alemohammad SMA, Noori SMR, Samarbafzadeh E, et al. The role of the gut microbiota and nutrition on spatial learning and spatial memory: a mini review based on animal studies. Mol Biol Rep 2022;49:1551-63. [Crossref] [PubMed]
- Hou L, Li X, Yan P, et al. Impact of the Duration of Breastfeeding on the Intelligence of Children: A Systematic Review with Network Meta-Analysis. Breastfeed Med 2021;16:687-96. [Crossref] [PubMed]
- Gao W, Salzwedel AP, Carlson AL, et al. Gut microbiome and brain functional connectivity in infants-a preliminary study focusing on the amygdala. Psychopharmacology (Berl) 2019;236:1641-51. [Crossref] [PubMed]
- Streit F, Prandovszky E, Send T, et al. Microbiome profiles are associated with cognitive functioning in 45-month-old children. Brain Behav Immun 2021;98:151-60. [Crossref] [PubMed]
- Carlson AL, Xia K, Azcarate-Peril MA, et al. Infant Gut Microbiome Associated With Cognitive Development. Biol Psychiatry 2018;83:148-59. [Crossref] [PubMed]
- Cheng J, Ringel-Kulka T, Heikamp-de Jong I, et al. Discordant temporal development of bacterial phyla and the emergence of core in the fecal microbiota of young children. ISME J 2016;10:1002-14. [Crossref] [PubMed]
- Oberacher H, Arnhard K, Linhart C, et al. Targeted Metabolomic Analysis of Soluble Lysates from Platelets of Patients with Mild Cognitive Impairment and Alzheimer’s Disease Compared to Healthy Controls: Is PC aeC40:4 a Promising Diagnostic Tool? J Alzheimers Dis 2017;57:493-504. [Crossref] [PubMed]
- Xu H, Valenzuela N, Fai S, et al. Targeted lipidomics – advances in profiling lysophosphocholine and platelet-activating factor second messengers. FEBS J 2013;280:5652-67. [Crossref] [PubMed]
- Bressler J, Yu B, Mosley TH, et al. Metabolomics and cognition in African American adults in midlife: the atherosclerosis risk in communities study. Transl Psychiatry 2017;7:e1173. [Crossref] [PubMed]
- Orešič M, Hyötyläinen T, Herukka SK, et al. Metabolome in progression to Alzheimer’s disease. Transl Psychiatry 2011;1:e57. [Crossref] [PubMed]
- van der Lee SJ, Teunissen CE, Pool R, et al. Circulating metabolites and general cognitive ability and dementia: Evidence from 11 cohort studies. Alzheimers Dement 2018;14:707-22. [Crossref] [PubMed]
- Tran SM, Mohajeri MH. The Role of Gut Bacterial Metabolites in Brain Development, Aging and Disease. Nutrients 2021;13:732. [Crossref] [PubMed]
- Wei Y, Liang J, Su Y, et al. The associations of the gut microbiome composition and short-chain fatty acid concentrations with body fat distribution in children. Clin Nutr 2021;40:3379-90. [Crossref] [PubMed]
- Chen F, Li Q, Chen Y, et al. Association of the gut microbiota and fecal short-chain fatty acids with skeletal muscle mass and strength in children. FASEB J 2022;36:e22109. [Crossref] [PubMed]
- Fugmann M, Breier M, Rottenkolber M, et al. The stool microbiota of insulin resistant women with recent gestational diabetes, a high risk group for type 2 diabetes. Sci Rep 2015;5:13212. [Crossref] [PubMed]
- Xie G, Wang L, Chen T, et al. A Metabolite Array Technology for Precision Medicine. Anal Chem 2021;93:5709-17. [Crossref] [PubMed]
- Wechsler D. The Wechsler Intelligence Scale for Children-Fourth Edition. The Psychological Corporation; 2003.
- Yang Y, Wang G, Pan X. China food composition. Beijing: Peking University Medical Press; 2009:795-9.
- Zhang F, Chen W, Zhu Z, et al. OSCA: a tool for omic-data-based complex trait analysis. Genome Biol 2019;20:107. [Crossref] [PubMed]
- Shi L, Westerhuis JA, Rosén J, et al. Variable selection and validation in multivariate modelling. Bioinformatics 2019;35:972-80. [Crossref] [PubMed]
- Pang Z, Chong J, Zhou G, et al. MetaboAnalyst 5.0: narrowing the gap between raw spectra and functional insights. Nucleic Acids Res 2021;49:W388-96. [Crossref] [PubMed]
- Ye F, Gao X, Wang Z, et al. Comparison of gut microbiota in autism spectrum disorders and neurotypical boys in China: A case-control study. Synth Syst Biotechnol 2021;6:120-6. [Crossref] [PubMed]
- Allen AP, Dinan TG, Clarke G, et al. A psychology of the human brain-gut-microbiome axis. Soc Personal Psychol Compass 2017;11:e12309. [Crossref] [PubMed]
- Fernandez-Real JM, Serino M, Blasco G, et al. Gut Microbiota Interacts With Brain Microstructure and Function. J Clin Endocrinol Metab 2015;100:4505-13. [Crossref] [PubMed]
- Sordillo JE, Korrick S, Laranjo N, et al. Association of the Infant Gut Microbiome With Early Childhood Neurodevelopmental Outcomes: An Ancillary Study to the VDAART Randomized Clinical Trial. JAMA Netw Open 2019;2:e190905. [Crossref] [PubMed]
- Tooley KL. Effects of the Human Gut Microbiota on Cognitive Performance, Brain Structure and Function: A Narrative Review. Nutrients 2020;12:3009. [Crossref] [PubMed]
- Manderino L, Carroll I, Azcarate-Peril MA, et al. Preliminary Evidence for an Association Between the Composition of the Gut Microbiome and Cognitive Function in Neurologically Healthy Older Adults. J Int Neuropsychol Soc 2017;23:700-5. [Crossref] [PubMed]
- Nagpal R, Neth BJ, Wang S, et al. Modified Mediterranean-ketogenic diet modulates gut microbiome and short-chain fatty acids in association with Alzheimer’s disease markers in subjects with mild cognitive impairment. EbioMedicine 2019;47:529-42. [Crossref] [PubMed]
- Floyd RG, Keith TZ, Taub GE, et al. Cattell-Horn-Carroll cognitive abilities and their effects on reading decoding skills: g has indirect effects, more specific abilities have direct effects. School Psychology Quarterly 2007;22:200-33. [Crossref]
- Roopashree PG, Shetty SS, Kumari NS. Effect of medium chain fatty acid in human health and disease. Journal of Functional Foods 2021;87:104724. [Crossref]
- Boonstra E, de Kleijn R, Colzato LS, et al. Neurotransmitters as food supplements: the effects of GABA on brain and behavior. Front Psychol 2015;6:1520. [Crossref] [PubMed]
- Buzsáki G, Kaila K, Raichle M. Inhibition and brain work. Neuron 2007;56:771-83. [Crossref] [PubMed]
- Porges EC, Woods AJ, Edden RA, et al. Frontal Gamma-Aminobutyric Acid Concentrations Are Associated With Cognitive Performance in Older Adults. Biol Psychiatry Cogn Neurosci Neuroimaging 2017;2:38-44. [Crossref] [PubMed]
- Khan ZUN, Chand P, Majid H, et al. Urinary metabolomics using gas chromatography-mass spectrometry: potential biomarkers for autism spectrum disorder. BMC Neurol 2022;22:101. [Crossref] [PubMed]
- Jones PM, Bennett MJ. Urine organic acid analysis for inherited metabolic disease using gas chromatography-mass spectrometry. Methods Mol Biol 2010;603:423-31. [Crossref] [PubMed]
- Gabbi P, Ribeiro LR, Jessié Martins G, et al. Methylmalonate Induces Inflammatory and Apoptotic Potential: A Link to Glial Activation and Neurological Dysfunction. J Neuropathol Exp Neurol 2017;76:160-78. [Crossref] [PubMed]
- Lee H, Jang JH, Kim SJ. Malonic acid suppresses lipopolysaccharide-induced BV2 microglia cell activation by inhibiting the p38 MAPK/NF-κB pathway. Anim Cells Syst (Seoul) 2021;25:110-8. [Crossref] [PubMed]
- Parker A, Fonseca S, Carding SR. Gut microbes and metabolites as modulators of blood-brain barrier integrity and brain health. Gut Microbes 2020;11:135-57. [Crossref] [PubMed]
- Zierer J, Jackson MA, Kastenmüller G, et al. The fecal metabolome as a functional readout of the gut microbiome. Nat Genet 2018;50:790-5. [Crossref] [PubMed]
- Papapostolou G, Kiotseridis H, Romberg K, et al. Cognitive dysfunction and quality of life during pollen season in children with seasonal allergic rhinitis. Pediatr Allergy Immunol 2021;32:67-76. [Crossref] [PubMed]