Estimating the individual singleton preterm birth risk: nomogram establishment and independent validation
Highlight box
Key findings
• Factors affecting PTB are multi-dimensional, and the 3 most significant factors were identified as hypertensive eclampsia, assisted reproductive technology, and history of PTB.
What is known and what is new?
• Although nomograms for PTB had been established in previous studies, they were developed based on limited samples and variables.
• Male sex of the newborn, maternal age and BMI, marital status, mother’s race, parental level of education, prenatal care starting time, smoking, history of PTB, hypertensive eclampsia, infertility treatment, diabetes, hypertension, history of cesarean, birth circumstances, live birth order, gonorrhea, syphilis, and chlamydia were identified as independent predictors for PTB.
• A multidimensional comprehensive prediction model incorporating large data was established to predict the individualized probabilities of PTB.
What is the implication, and what should change now?
• Nomograms were developed according to multidimensional factors, which could be useful to physicians for improved early identification of PTB and making clinical decisions.
Introduction
Preterm birth (PTB) is defined as birth before 37 weeks’ gestation, which occurs in approximately 10% of births in the United States (US) (1). It is one of the leading causes of perinatal mortality and morbidity (2), accounting for approximately 18% of all pediatric deaths and 35% of deaths among newborns (3). An accurate and individualized assessment of the probability of PTB can assist early prevention and formulation of individualized treatment strategies for high-risk women, and improve neonatal outcomes. A nomogram including weighted calculation of various PTB-related factors can be used for individualized and accurate prediction of PTB, which is of great practical value for the clinical assessment of PTB. Although previous studies have established nomograms for PTB (4-8), they have been developed based on limited samples and variables. Factors affecting PTB are multi-dimensional, including the basic status of the mother, health status before pregnancy, complications of pregnancy, history of delivery and PTB, infertility and its treatment approach, sexually transmitted infections, living environment, and so on (9-14). Therefore, a more multidimensional prediction model incorporating large data is needed to improve the prediction of PTB. The National Vital Statistics System (NVSS) from the US is one of the largest and most comprehensive databases in the world, which records birth data in detail with objective authenticity and representativeness. Our study aimed to use the data from the NVSS database to develop and independently validate a more multidimensional nomogram for the estimation of individualized PTB risk based on multidimensional variables and the largest sample size to date. We present this article in accordance with the TRIPOD reporting checklist (available at https://tp.amegroups.com/article/view/10.21037/tp-22-611/rc).
Methods
This study used data from the NVSS in the US, a database that contains the birth and death records from 50 states and the District of Columbia. We obtained birth data from January 1, 2016, to December 31, 2020. All cases with complete information were included in this study. The related data can be obtained online: https://www.cdc.gov/nchs/data_access/vitalstatsonline.htm. All cases were divided into a PTB group and a full-term birth (FTB) group according to the gestational week at birth (37 weeks). Due to the data being publicly available and the study does not involve human participants, the institutional Review Board of Children’s Hospital of Fudan University deemed this study exempt from review and waived the informed consent requirement. The study was conducted in accordance with the Declaration of Helsinki (as revised in 2013).
The primary outcome was the risk of PTB. The secondary outcome was the risk of varying degrees of PTB (moderately and late PTB, very PTB, and extremely PTB). The relevant variables were as follows: maternal age (<20, 21–30, 31–40, and >40 years), sex (male and female), maternal body mass index (BMI) before pregnancy (underweight, normal, overweight, and obese), marital status (married and unmarried), mother’s race [White, Black, American Indian/Alaskan Native (AIAN), Asian, Native Hawaiian or Other Pacific Islander (NHOPI), and more than 1 race], parental education attainment (< high school, high school, associate degree, and ≥ bachelor’s degree), prenatal care starting time (1st to 3rd month, 4th to 6th month, 7th to final month, and no prenatal care), smoking (no smoking, smoking before pregnancy, and smoking during pregnancy), history of PTB and cesareans, hypertensive eclampsia, infertility and its treatment [no treatment, fertility-enhancing drugs, assisted reproductive technology (ART), and other treatments], diabetes (pre-pregnancy diabetes, gestational diabetes, and no diabetes), hypertension (pre-pregnancy hypertension, gestational hypertension, and no hypertension), live birth order, mother’s nativity (born in the US, born outside the US, and unknown), gonorrhea, syphilis, chlamydia, hepatitis B, and hepatitis C.
To better understand the different degrees of PTB, we divided the newborns into 3 subgroups: moderately and late PTB group (32 weeks ≤ gestational weeks <37 weeks), very PTB group (28 weeks ≤ gestational weeks <32 weeks), and extremely PTB group (22 weeks ≤ gestational weeks <28 weeks). We compared the differences in these abovementioned factors between the PTB and FTB groups by univariate logistic regression analyses, respectively. Multivariate logistic regression analyses were used to identify the independent predictors. We used the final data (16,294,529 samples) to establish 4 risk prediction nomograms, and then used the US Territories data from Puerto Rico, Virgin Islands, Guam, American Samoa, and Northern Marianas to perform external validation (54,708 samples). Further, we used the concordance index (C-index), calibration plots, and receiver operating characteristic (ROC) curves to validate the performance of these models.
Statistical analysis
Statistical analysis was performed using IBM 23.0 software (IBM Corp., Armonk, NY, USA) and R (version 4.1.2; R Foundation for Statistical Computing, Vienna, Austria). Univariate analyses were conducted to assess the association of PTB with factors. All statistical tests were 2-sided with 0.05 significance levels. Multivariate logistic regression analyses were used to identify the independent predictors for PTB. The prediction nomograms were developed by R based on a multivariate logistic regression model. The calibration curves were used to evaluate the consistency of the nomograms. The aptitude of each nomogram for predicting PTB risk was appraised by the C-index and the area under the receiver operating characteristic curve (AUC).
Results
A total of 16,294,529 samples were enrolled, as shown in Figure 1. The detailed information is listed in Table 1. Univariate analysis revealed that 20 variables had relationship with PTB. Table 2 displays the multivariate logistic regression analysis results, showing that male sex of the newborn [odds ratio (OR): 0.910; 95% confidence interval (CI): 0.907–0.913; P<0.001], maternal age <20 (OR: 1.155; 95% CI: 1.144–1.166; P<0.001), maternal BMI (OR: 1.224; 95% CI: 1.212–1.236; P<0.001), marital status (OR: 1.142; 95% CI: 1.137–1.146; P<0.001), mother’s race (OR: 1.425; 95% CI: 1.418–1.432; P<0.001), mother’s level of education (OR: 1.167; 95% CI: 1.158–1.176; P<0.001), father’s level of education (OR: 1.180; 95% CI: 1.172–1.189; P=0.047), prenatal care starting time (OR: 2.284; 95% CI: 2.256–2.312; P<0.001), smoking (OR: 1.208; 95% CI: 1.199–1.217; P<0.001), history of PTB (OR: 2.771; 95% CI: 2.752–2.790; P<0.001), hypertensive eclampsia (OR: 4.075; 95% CI: 3.987–4.164; P<0.001), infertility treatment (OR: 2.977; 95% CI: 2.940–3.014; P<0.001), diabetes (OR: 2.015; 95% CI: 1.988–2.042; P<0.001), hypertension (OR: 2.547; 95% CI: 2.534–2.561; P<0.001), history of cesarean (OR: 1.089; 95% CI: 1.084–1.094; P<0.001), nativity (OR: 0.978; 95% CI: 0.973–0.982; P<0.001), live birth order (OR: 1.029; 95% CI: 1.024–1.034; P<0.001), gonorrhea (OR: 1.140; 95% CI: 1.100–1.181; P<0.001), syphilis (OR: 1.095; 95% CI: 1.040–1.153; P<0.001), and chlamydia (OR: 1.028; 95% CI: 1.014–1.042; P<0.001) were independent predictors for PTB. In addition, the comparison of characteristics of different degrees of PTB and FTB are listed in Table S1. The detailed information from multivariate logistic regression analysis of these 3 models is shown in Tables S2-S4.
Table 1
Variables | FTB group, N (%) | PTB group, N (%) | P value |
---|---|---|---|
All population | 14,518,114 (100.0) | 1,776,415 (100.0) | – |
Year | <0.001 | ||
2016 | 3,021,461 (20.8) | 356,284 (20.1) | |
2017 | 2,954,513 (20.4) | 356,739 (20.1) | |
2018 | 2,913,994 (20.1) | 354,401 (20.0) | |
2019 | 2,873,800 (19.8) | 366,091 (20.6) | |
2020 | 2,754,346 (18.9) | 342,900 (19.3) | |
Maternal age | <0.001 | ||
<20 | 528,824 (3.6) | 76,366 (4.3) | |
20–30 | 6,835,689 (47.1) | 785,330 (44.2) | |
31–40 | 6,679,838 (46.0) | 824,490 (46.4) | |
>40 | 473,763 (3.3) | 90,229 (5.1) | |
Sex of newborn | <0.001 | ||
Male | 7,124,839 (49.1) | 829,014 (46.7) | |
Female | 7,393,275 (50.9) | 947,401 (53.3) | |
Mother’s education | <0.001 | ||
< High school | 1,525,921 (10.5) | 234,230 (13.2) | |
High school | 3,357,889 (23.1) | 462,173 (26.0) | |
AD | 4,086,022 (28.1) | 515,623 (29.0) | |
≥ BD | 5,376,325 (37.0) | 541,797 (30.5) | |
Unknown | 171,957 (1.2) | 22,592 (1.3) | |
Smoking | <0.001 | ||
No smoking | 13,555,015 (93.4) | 1,622,400 (91.3) | |
Smoking cessation | 270,926 (1.9) | 33,000 (1.9) | |
Smoking | 692,173 (4.8) | 121,015 (6.8) | |
History of PTB | <0.001 | ||
Yes | 374,940 (2.6) | 148,322 (8.3) | |
No | 14,143,174 (97.4) | 1,628,093 (91.7) | |
Diabetes | <0.001 | ||
Pre-pregnancy | 112,194 (0.8) | 164,153 (9.2) | |
Gestational | 970,722 (6.7) | 38,168 (0.2) | |
No | 13,435,198 (92.5) | 1,574,094 (88.6) | |
Chlamydia | <0.001 | ||
Yes | 185,898 (1.3) | 28,629 (1.6) | |
No | 14,313,866 (98.6) | 1,740,816 (98.0) | |
Unknown | 18,350 (0.1) | 6,790 (0.4) | |
Gonorrhea | <0.001 | ||
Yes | 23,365 (0.2) | 4,516 (0.3) | |
No | 14,476,399 (99.7) | 1,764,929 (99.4) | |
Unknown | 18,350 (0.1) | 6,790 (0.4) | |
Hepatitis B | <0.001 | ||
Yes | 30,763 (0.2) | 4,102 (0.2) | |
No | 14,469,001 (99.7) | 1,765,343 (99.4) | |
Unknown | 18,350 (0.1) | 6,790 (0.4) | |
Prenatal care starting time | <0.001 | ||
1st to 3rd month | 11,670,225 (80.4) | 1,334,440 (75.1) | |
4th to 6th month | 2,130,762 (14.7) | 328,269 (18.5) | |
7th to final month | 561,230 (3.9) | 67,417 (3.8) | |
No prenatal care | 155,897 (1.1) | 46,289 (2.6) | |
Mother’s race | <0.001 | ||
White | 11,126,214 (76.6) | 1,260,596 (70.9) | |
Black | 1,778,873 (12.3) | 326,829 (18.4) | |
AIAN | 118,473 (0.8) | 17,701 (1.0) | |
Asian | 1095,352 (7.5) | 118,769 (6.7) | |
NHOPI | 41,768 (0.3) | 7,147 (0.4) | |
>1 race | 357,434 (2.5) | 45,373 (2.6) | |
Maternal BMI | <0.001 | ||
Normal | 6,187,556 (42.6) | 669,411 (37.7) | |
Underweight | 429,259 (2.9) | 57,838 (3.3) | |
Overweight | 3,828,984 (26.4) | 458,299 (25.8) | |
Obesity | 3,827,848 (26.4) | 551,396 (31.0) | |
Unknown | 244,467 (1.7) | 39,441 (2.2) | |
Infertility treatment | <0.001 | ||
No | 14,255,558 (98.2) | 1,696,659 (95.5) | |
FEDT | 108,543 (0.7) | 30,778 (1.7) | |
ART | 138,200 (1.0) | 44,664 (2.5) | |
Other treatments | 15,813 (0.1) | 4,314 (0.2) | |
Previous cesareans | <0.001 | ||
Yes | 2,213,891 (15.2) | 329,463 (18.5) | |
No | 12,304,223 (84.8) | 1,446,952 (81.5) | |
Father’s education | <0.001 | ||
< High school | 1,776,843 (12.3) | 260,640 (14.7) | |
High school | 4149519 (28.6) | 573,027 (32.3) | |
AD | 3,694,278 (25.4) | 443,923 (25.0) | |
≥ BD | 4,539,764 (31.3) | 444,003 (25.0) | |
Unknown | 357,710 (2.5) | 54,822 (3.1) | |
Mother’s nativity | <0.001 | ||
In the US | 11,032,782 (76.0) | 1,354,549 (76.3) | |
Outside the US | 3,465,698 (23.9) | 419,127 (23.6) | |
Unknown | 19,634 (0.1) | 2,739 (0.2) | |
Hypertension eclampsia | <0.001 | ||
Yes | 26,125 (0.2) | 15,391 (0.9) | |
No | 14,491,989 (99.8) | 1,761,024 (99.1) | |
Hypertension | <0.001 | ||
Pre-pregnancy | 245,404 (1.7) | 76,365 (4.3) | |
Gestational | 886,156 (6.1) | 258,871 (14.6) | |
No | 13,386,554 (92.2) | 1,441,179 (81.1) | |
Marital status | <0.001 | ||
Married | 8,888,021 (61.2) | 1,005,385 (56.6) | |
Unmarried | 4,127,076 (28.4) | 618,577 (34.8) | |
Unknown | 1,503,017 (10.4) | 152,453 (8.6) | |
Syphilis | <0.001 | ||
Yes | 11,995 (0.1) | 2,318 (0.1) | |
No | 14,487,769 (99.8) | 1,767,127 (99.5) | |
Unknown | 18,350 (0.1) | 6,790 (0.4) | |
Hepatitis C | <0.001 | ||
Yes | 44,229 (0.3) | 9,891 (0.6) | |
No | 14,455,535 (99.6) | 1,759,554 (99.1) | |
Unknown | 18,350 (0.1) | 6,790 (0.4) | |
Live birth order | <0.001 | ||
1 | 5,555,199 (38.3) | 610,729 (34.4) | |
2 | 4,813,305 (33.2) | 551,339 (31.0) | |
3 | 2,457,445 (16.9) | 324,556 (18.3) | |
4 | 1,004,828 (6.9) | 160,607 (9.0) | |
5 | 371,942 (2.6) | 69,344 (3.9) | |
6 | 151,213 (1.0) | 29,738 (1.7) | |
7 | 68,075 (0.5) | 13,425 (0.8) | |
≥8 | 76,534 (0.5) | 13,945 (0.8) | |
Unknown | 19,573 (0.1) | 2,732 (0.2) |
FTB, full-term birth; PTB, preterm birth; AD, associate degree; BD, bachelor’s degree; US, United States; AIAN, American Indian/Alaskan Native; NHOPI, Native Hawaiian or Other Pacific Islander; BMI, body mass index; FEDT, fertility-enhancing drugs therapy; ART, assisted reproductive technology.
Table 2
Factor | OR | 95% CI | P value |
---|---|---|---|
Sex (female vs. male) | 0.910 | 0.907–0.913 | <0.001 |
Maternal age (year) | |||
<20 vs. 20–30 | 1.155 | 1.144–1.166 | <0.001 |
31–40 vs. 20–30 | 1.108 | 1.104–1.113 | <0.001 |
>40 vs. 20–30 | 1.389 | 1.377–1.402 | <0.001 |
Maternal BMI | |||
Underweight vs. normal | 1.224 | 1.212–1.236 | <0.001 |
Overweight vs. normal | 0.984 | 0.980–0.988 | <0.001 |
Obesity vs. normal | 0.998 | 0.993–1.002 | <0.001 |
Marital status (unmarried vs. married) | 1.142 | 1.137–1.146 | <0.001 |
Race of mother | |||
Black vs. White | 1.425 | 1.418–1.432 | <0.001 |
AIAN vs. White | 1.074 | 1.056–1.093 | <0.001 |
Asian vs. White | 1.113 | 1.104–1.122 | <0.001 |
NHOPI vs. White | 1.310 | 1.272–1.348 | <0.001 |
>1 race vs. White | 1.067 | 1.055–1.079 | <0.001 |
Mother’s education | |||
< High school vs. ≥ bachelor’s degree | 1.167 | 1.158–1.176 | <0.001 |
High school vs. ≥ bachelor’s degree | 1.115 | 1.108–1.122 | <0.001 |
Associate degree vs. ≥ bachelor’s degree | 1.080 | 1.075–1.086 | <0.001 |
Father’s education | |||
<High school vs. ≥ bachelor’s degree | 1.180 | 1.172–1.189 | 0.047 |
High school vs. ≥ bachelor’s degree | 1.173 | 1.166–1.180 | <0.001 |
Associate degree vs. ≥ bachelor’s degree | 1.099 | 1.093–1.105 | <0.001 |
Prenatal care starting time | |||
4th to 6th month vs. 1st to 3rd month | 1.249 | 1.243–1.255 | <0.001 |
7th to final month vs. 1st to 3rd month | 0.994 | 0.985–1.003 | 0.217 |
No vs. 1st to 3rd month | 2.284 | 2.256–2.312 | <0.001 |
Smoking | |||
Smoking cessation vs. no smoking | 0.943 | 0.932–0.955 | <0.001 |
Smoking vs. no smoking | 1.208 | 1.199–1.217 | <0.001 |
History of preterm (yes vs. no) | 2.771 | 2.752–2.790 | <0.001 |
Hypertensive eclampsia (yes vs. no) | 4.075 | 3.987–4.164 | <0.001 |
Infertility treatment | |||
Other treatments vs. no | 2.550 | 2.462–2.642 | <0.001 |
FEDT vs. no | 2.728 | 2.690–2.766 | <0.001 |
ART vs. no | 2.977 | 2.940–3.014 | <0.001 |
Diabetes | |||
Pre-pregnancy diabetes vs. no | 2.015 | 1.988–2.042 | <0.001 |
Gestational diabetes vs. no | 1.234 | 1.226–1.241 | <0.001 |
Hypertension | |||
Pre-pregnancy hypertension vs. no | 2.243 | 2.222–2.264 | <0.001 |
Gestational hypertension vs. no | 2.547 | 2.534–2.561 | <0.001 |
History of cesareans (yes vs. no) | 1.089 | 1.084–1.094 | <0.001 |
Live birth order | |||
2 vs. 1 | 1.029 | 1.024–1.034 | <0.001 |
3 vs. 1 | 1.092 | 1.086–1.098 | <0.001 |
4 vs. 1 | 1.206 | 1.198–1.215 | <0.001 |
5 vs. 1 | 1.284 | 1.271–1.297 | <0.001 |
6 vs. 1 | 1.271 | 1.253–1.290 | <0.001 |
7 vs. 1 | 1.219 | 1.193–1.245 | <0.001 |
≥8 vs. 1 | 1.081 | 1.059–1.104 | <0.001 |
Nativity | |||
Not in the US vs. in the US | 0.978 | 0.973–0.982 | <0.001 |
Unknown vs. in the US | 0.987 | 0.943–1.034 | 0.585 |
Gonorrhea (yes vs. no) | 1.140 | 1.100–1.181 | <0.001 |
Syphilis (yes vs. no) | 1.095 | 1.040–1.153 | <0.001 |
Chlamydia (yes vs. no) | 1.028 | 1.014–1.042 | <0.001 |
PTB, preterm birth; OR, odds ratio; CI, confidence interval; BMI, body mass index; AIAN, American Indian/Alaskan Native; NHOPI, Native Hawaiian or Other Pacific Islander; FEDT, fertility-enhancing drugs therapy; ART, assisted reproductive technology; US, United States.
A final nomogram was established (Figure 2). The variables that were found to increase the probability of PTB included sex (male newborn), maternal age (<20 years, >40 years), maternal BMI (underweight, overweight, and obesity), marital status (unmarried), mother’s race (non-white), mother’s and father’s level of education (< high school), prenatal care starting time (not at all, 4th to 6th month, and 7th to final month), smoking, history of PTB, hypertensive eclampsia, infertility treatment, diabetes, hypertension, history of cesarean, live-birth order, nativity (not in the US), gonorrhea, syphilis, and chlamydia. The detailed score of each variable in the model and the prediction PTB probability corresponding to the total point are shown in Table S5. The score of each variable in the moderately and late PTB, very PTB, and extremely PTB risk model and the prediction probability corresponding to the total points are shown in Table S6, Table S7, and Table S8, respectively. To use the nomogram, first, locate a specific point of an individual patient on each variable axis; second, draw a vertical line upwards in the variable axis, with each variable corresponding to a specific point on the Points scale (top); third, sum all the points of each variable, and locate on the Total Points scale (bottom); forth, draw a vertical line downwards, corresponding to the PTB probability axis to determine the PTB probability. We found that the risk variables were identical in 3 models (PTB model, moderately and late PTB model, and very PTB model), whereas the weights of variables were different with different contribution degrees. We also found that hypertensive eclampsia (1st) and infertility treatment (2nd) were the top 2 contributors in all these 3 models. In addition, a slight difference between the extremely PTB model and the other 3 models was revealed, with syphilis and chlamydia not included in the extremely PTB model since they were not independent predictors. The top 2 contributors in the extremely PTB model were prenatal care starting time (1st) and infertility treatment (2nd).
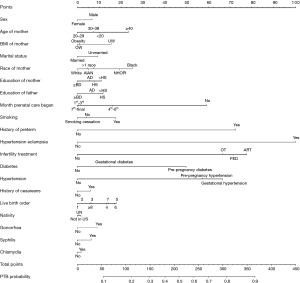
The C-index and the AUC under the ROC curves in the training set and validation set were 0.652 (95% CI: 0.652–0.653, Figure 3A) and 0.642 (95% CI: 0.635–0.649, Figure 3B), respectively. For the predicted PTB risk plots in both the training set and validation set (Figure 3C,3D), both of these lines slightly strayed from the ideal reference line toward the low end of the outcome scale; however the observed and optimism-corrected lines were nearly consistent, indicating that this model’s predictions were consistent with the expectations.
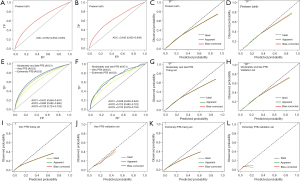
In addition, we established 3 prediction nomograms for moderately and late PTB, very PTB, and extremely PTB, respectively (Figures S1-S3). In the training set, the C-index and the AUC were 0.647 (95% CI: 0.646–0.647, Figure 3E) in moderately and late PTB, 0.693 (95% CI: 0.692–0.695, Figure 3E) in very PTB, and 0.726 (95% CI: 0.724–0.728, Figure 3E) in extremely PTB. In the validation set, the C-index and the AUC were 0.638 (95% CI: 0.630–0.645, Figure 3F) in moderately and late PTB, 0.702 (95% CI: 0.682–0.722, Figure 3F) in very PTB, and 0.705 (95% CI: 0.676–0.734, Figure 3F) in extremely PTB. The calibration curves in both the training set and validation set showed that the predicted value approximated the observed value in these 3 models (Figure 3G-3L). Overall, our external validation results showed that these prediction nomograms had moderate consistency and discrimination, and that they had reliable predictive performance.
Discussion
The goal of this study was to develop and independently validate prediction models for PTB based on a large population and multidimensional analyses. The contribution degree of different variables to the model from high to low was hypertensive eclampsia, infertility treatment, history of PTB, hypertension, prenatal care starting time, smoking, diabetes, mother’s race, maternal age, live-birth order, smoking, maternal BMI, father’s level of education, mother’s level of education, marital status, gonorrhea, sex of the newborn, syphilis, history of cesareans, chlamydia, and nativity. In addition, 3 nomograms for moderately and late PTB, very PTB, and extremely PTB were developed for further predicting different degrees of PTB probabilities, respectively. These models could predict the probability of PTB individually and provide a reference for clinicians in individualized decision making.
Hypertensive eclampsia is one of the most common life-threatening maternal diseases worldwide and is closely associated with PTB, increasing the risk of PTB (13,15). In our study, 0.9% of women had hypertensive eclampsia in the PTB group, which was significantly higher than in the FTB group. Hypertensive eclampsia was shown to be an independent predictor for PTB. In addition, in 3 of our nomograms, hypertensive eclampsia had the greatest contribution to PTB.
As the incidence of infertility increases, more parents undergo infertility treatment, such as fertility-enhancing drugs and ART. However, infertility treatment may increase the risk of PTB (16). In our study, we found that 4.4% of children of parents who underwent infertility treatment experienced PTB, which was much higher than that in FTB group (0.8%). Of the parents who underwent infertility treatment, 56.0% received ART and 38.6% received fertility-enhancing drug treatment, and infertility treatment was confirmed as an independent predictor, which was consistent with previous studies (17,18). To summarize, infertility treatment could increase the PTB risk, and ART has the greatest impact on PTB in this regard.
An increased risk of PTB also is observed in women with a history of PTB and cesarean (1,19). Williams et al. found that compared to women without cesarean delivery history, women with cesarean delivery history in their first pregnancy were 14% more likely to have a PTB in their second pregnancy (19). Koire et al. found that a history of PTB can increase the risk of PTB (1). Our findings also supported their views that the history of PTB and cesarean had relationship with PTB.
Diabetes and hypertension have also been shown to be associated with PTB (20-22), with an increasing PTB rate in mothers with diabetes and hypertension. Their findings were similar to ours; these factors were independent predictors for PTB in our study. In addition, some common infections during pregnancy are associated with PTB. The mechanism of maternal infection/inflammation leading to PTB may be related to intrauterine inflammation (23,24). There is a significant association between chlamydia infection and chorioamnionitis, and chlamydia may lead to chorioamnionitis (25). In addition, there may be a relationship between chorioamnionitis and gonorrhea (25). Chorioamnionitis was found to be an independent predictor of PTB in a previous study (26), and chlamydia and gonorrhea might lead to chorioamnionitis to increase PTB risk. Gao et al. also concluded that maternal sexually transmitted infections could increase the risk of PTB (27). Their results were consistent with our findings that gonorrhea, syphilis, and chlamydia are independent predictors of PTB.
Smoking during pregnancy can endanger the unborn child’s health, and has long been considered a risk factor for PTB (28,29). Cessation of smoking cessation can reduce such risk (10). In our study, smoking during pregnancy was shown to increase the risk of PTB compared with smoking cessation, and smoking was an independent predictor of PTB. In the US, prenatal care is associated with a lower PTB rate, and increased prenatal care participation may reduce PTB rates (30). The majority of women in our study received prenatal care, and the PTB rate was higher among women who did not receive prenatal care; the earlier prenatal care was initiated, the lower the incidence of PTB.
In addition to the relevant factors discussed above, the sex of the newborn, maternal age, marital status, mother’s race, and nativity have long been associated with PTB (4,7,12), and were also confirmed as independent predictors for PTB in our study. In addition to these variables, maternal BMI was shown to have a relationship with PTB: compared with mothers with a normal maternal BMI, those who were underweight, overweight, or obese had a higher PTB rates.
A similar PTB nomogram was developed by Mehta-Lee et al. with 192,208 samples (6), which was established based on 9 factors. Although our results supported their conclusion that the factors mentioned in their study are of significance, their nomogram did not include some variables which we found to be closely associated with PTB, such as the sex of the newborn, maternal BMI, marital status, education levels of parents, prenatal care starting time, hypertension eclampsia, infertility treatment, hypertension, history of cesareans, live-birth order, nativity, and some sexually transmitted diseases (27).
We established a multidimensional model to provide an individualized prediction of PTB. To further predict the probability of different degrees of PTB, moderately and late PTB, very PTB, and extremely PTB nomograms were also established. In addition, we also found 20 identical risk factors in the PTB, moderately and late PTB, and very PTB models, which supported the commonality of these 3 models. Although the contribution degree of each factor was different, hypertensive eclampsia (1st) and infertility treatment (2nd) were the top 2 contributors in all 3 models. The extremely PTB model was slightly different from the other 3 models, whereby syphilis and chlamydia were not independent predictors for extremely PTB. These results may be due to only 0.1% (127 samples) and 1.8% (1,697 samples) of women in the extremely PTB group having syphilis and chlamydia. In addition, the population samples were extremely limited compared with the FTB group. In addition, the prenatal care starting time (1st) had the largest contribution to the extremely PTB model, however, infertility treatment and hypertensive eclampsia (which were the top 2 contributors in the other 3 models) still contributed significantly to the extremely PTB model.
Although the consistency of these models ranged from 64.7% to 72.8%, the calibration curves showed that these 4 models had relatively good discrimination, indicating that the predicted values approximated the observed values. The validation findings showed that our models had reliable predictive performance. Therefore, our models can help physicians estimate the individualized PTB probability, the mothers with high predicted PTB risk can be screened out, and the physicians allocate more attention to them. For example, for mothers with higher model prediction-based PTB probability, physicians can make individualized obstetric examination plans, and provide them with personalized advice, such as smoking cessation, to decrease the PTB risk.
This study had several limitations. Firstly, the nomograms were established using data from the US, reducing their applicability in other countries, particularly those with different development levels or health care systems. Therefore, we will use data from different institutions and other countries for validation in future studies. Secondly, in previous studies, some other factors were associated with PTB, such as cervical length, vaginal bleeding (7), maternal thyroid function (31), preeclampsia (13), maternal marijuana use (32), and so on. These factors were not included in our nomograms as such data was absent in the NVSS database. Thirdly, the accuracy of the models is not perfect. Despite these limitations, the models were developed and validated based on the largest sample size known to date, the variables included in the models are multidimensional, and the population data is from different regions of the US, making our models more applicable and representative. In addition, the models allow us to not only predict the probability of PTB, but also to estimate the probability of different degrees of PTB.
Conclusions
Factors affecting PTB are multi-dimensional, and in this study, the 3 most significant factors were hypertensive eclampsia, ART, and history of PTB. We established 4 nomograms to predict the individual probability of PTB based on a multidimensional large sample size dataset, which can provide an individual PTB possibility estimation rather than a group estimation.
Acknowledgments
Funding: None.
Footnote
Reporting Checklist: The authors have completed the TRIPOD reporting checklist. Available at https://tp.amegroups.com/article/view/10.21037/tp-22-611/rc
Peer Review File: Available at https://tp.amegroups.com/article/view/10.21037/tp-22-611/prf
Conflicts of Interest: All authors have completed the ICMJE uniform disclosure form (available at https://tp.amegroups.com/article/view/10.21037/tp-22-611/coif). The authors have no conflicts of interest to declare.
Ethical Statement: The authors are accountable for all aspects of the work in ensuring that questions related to the accuracy or integrity of any part of the work are appropriately investigated and resolved. Due to the data being publicly available and the study does not involve human participants, the institutional Review Board of Children’s Hospital of Fudan University deemed this study exempt from review and waived the informed consent requirement. The study was conducted in accordance with the Declaration of Helsinki (as revised in 2013).
Open Access Statement: This is an Open Access article distributed in accordance with the Creative Commons Attribution-NonCommercial-NoDerivs 4.0 International License (CC BY-NC-ND 4.0), which permits the non-commercial replication and distribution of the article with the strict proviso that no changes or edits are made and the original work is properly cited (including links to both the formal publication through the relevant DOI and the license). See: https://creativecommons.org/licenses/by-nc-nd/4.0/.
References
- Koire A, Chu DM, Aagaard K. Family history is a predictor of current preterm birth. Am J Obstet Gynecol MFM 2021;3:100277. [Crossref] [PubMed]
- Gioan M, Fenollar F, Loundou A, et al. Development of a nomogram for individual preterm birth risk evaluation. J Gynecol Obstet Hum Reprod 2018;47:545-8. [Crossref] [PubMed]
- Oh KJ, Romero R, Park JY, et al. Evidence that antibiotic administration is effective in the treatment of a subset of patients with intra-amniotic infection/inflammation presenting with cervical insufficiency. Am J Obstet Gynecol 2019;221:140.e1-140.e18. [Crossref] [PubMed]
- Meng Y, Lin J, Fan J. A Novel Nomogram for Predicting the Risk of Premature Delivery Based on the Thyroid Function in Pregnant Women. Front Endocrinol (Lausanne) 2021;12:793650. [Crossref] [PubMed]
- Zhang J, Zhan W, Lin Y, et al. Development and external validation of a nomogram for predicting preterm birth at < 32 weeks in twin pregnancy. Sci Rep 2021;11:12430. [Crossref] [PubMed]
- Mehta-Lee SS, Palma A, Bernstein PS, et al. A Preconception Nomogram to Predict Preterm Delivery. Matern Child Health J 2017;21:118-27. [Crossref] [PubMed]
- Mailath-Pokorny M, Polterauer S, Kohl M, et al. Individualized assessment of preterm birth risk using two modified prediction models. Eur J Obstet Gynecol Reprod Biol 2015;186:42-8. [Crossref] [PubMed]
- Dabi Y, Nedellec S, Bonneau C, et al. Clinical validation of a model predicting the risk of preterm delivery. PLoS One 2017;12:e0171801. [Crossref] [PubMed]
- Crump C, Sundquist J, Sundquist K. Preterm birth and risk of type 1 and type 2 diabetes: a national cohort study. Diabetologia 2020;63:508-18. [Crossref] [PubMed]
- Soneji S, Beltrán-Sánchez H. Association of Maternal Cigarette Smoking and Smoking Cessation With Preterm Birth. JAMA Netw Open 2019;2:e192514. [Crossref] [PubMed]
- Ebisutani K, Wang CK, Jun Ahn H, et al. Utility of Routine Testing for Chlamydia and Gonorrhea in the Setting of Preterm Delivery or Premature Preterm Rupture of Membranes. Hawaii J Health Soc Welf 2021;80:134-9. [PubMed]
- Tingleff T, Vikanes Å, Räisänen S, et al. Risk of preterm birth in relation to history of preterm birth: a population-based registry study of 213 335 women in Norway. BJOG 2022;129:900-7. [Crossref] [PubMed]
- An H, Jin M, Li Z, et al. Impact of gestational hypertension and pre-eclampsia on preterm birth in China: a large prospective cohort study. BMJ Open 2022;12:e058068. [Crossref] [PubMed]
- Defilipo ÉC, Chagas PSC, Drumond CM, et al. Factors associated with premature birth: a case-control study. Rev Paul Pediatr 2022;40:e2020486. [Crossref] [PubMed]
- Lisonkova S, Bone JN, Muraca GM, et al. Incidence and risk factors for severe preeclampsia, hemolysis, elevated liver enzymes, and low platelet count syndrome, and eclampsia at preterm and term gestation: a population-based study. Am J Obstet Gynecol 2021;225:538.e1-538.e19. [Crossref] [PubMed]
- Sanders JN, Simonsen SE, Porucznik CA, et al. Fertility treatments and the risk of preterm birth among women with subfertility: a linked-data retrospective cohort study. Reprod Health 2022;19:83. [Crossref] [PubMed]
- Wang R, Shi Q, Jia B, et al. Association of Preterm Singleton Birth With Fertility Treatment in the US. JAMA Netw Open 2022;5:e2147782. [Crossref] [PubMed]
- Farley GJ, Sauer MV, Brandt JS, et al. Singleton pregnancies conceived with infertility treatments and the risk of neonatal and infant mortality. Fertil Steril 2021;116:1515-23. [Crossref] [PubMed]
- Williams CM, Asaolu I, Chavan NR, et al. Previous cesarean delivery associated with subsequent preterm birth in the United States. Eur J Obstet Gynecol Reprod Biol 2018;229:88-93. [Crossref] [PubMed]
- Chen X, Gissler M, Lavebratt C. Association of maternal polycystic ovary syndrome and diabetes with preterm birth and offspring birth size: a population-based cohort study. Hum Reprod 2022;37:1311-23. [Crossref] [PubMed]
- Kong L, Nilsson IAK, Gissler M, et al. Associations of Maternal Diabetes and Body Mass Index With Offspring Birth Weight and Prematurity. JAMA Pediatr 2019;173:371-8. [Crossref] [PubMed]
- Cao X, Zu D, Liu Y. Effects of Interaction Between Gestational Hypertension and History of Preterm Birth on the Risk of Preterm Birth: An Analysis Based on the National Vital Statistics System Database. Med Sci Monit 2022;28:e935094. [Crossref] [PubMed]
- Nadeau HC, Subramaniam A, Andrews WW. Infection and preterm birth. Semin Fetal Neonatal Med 2016;21:100-5. [Crossref] [PubMed]
- Gomez-Lopez N, Galaz J, Miller D, et al. The immunobiology of preterm labor and birth: intra-amniotic inflammation or breakdown of maternal-fetal homeostasis. Reproduction 2022;164:R11-45. [Crossref] [PubMed]
- Fuchs E, Dwiggins M, Lokken E, et al. Influence of Sexually Transmitted Infections in Pregnant Adolescents on Preterm Birth and Chorioamnionitis. Infect Dis Obstet Gynecol 2020;2020:1908392. [Crossref] [PubMed]
- Lee JE, Dan K, Kim HJ, et al. Plasma proteomic analysis to identify potential biomarkers of histologic chorioamnionitis in women with preterm premature rupture of membranes. PLoS One 2022;17:e0270884. [Crossref] [PubMed]
- Gao R, Liu B, Yang W, et al. Association of Maternal Sexually Transmitted Infections With Risk of Preterm Birth in the United States. JAMA Netw Open 2021;4:e2133413. [Crossref] [PubMed]
- Liu B, Xu G, Sun Y, et al. Maternal cigarette smoking before and during pregnancy and the risk of preterm birth: A dose-response analysis of 25 million mother-infant pairs. PLoS Med 2020;17:e1003158. [Crossref] [PubMed]
- Curtin SC, Matthews TJ. Smoking Prevalence and Cessation Before and During Pregnancy: Data From the Birth Certificate, 2014. Natl Vital Stat Rep 2016;65:1-14. [PubMed]
- Tang MB, Kung PT, Chiu LT, et al. Comparison of the use of prenatal care services and the risk of preterm birth between pregnant women with disabilities and those without disabilities: A nationwide cohort study. Front Public Health 2023;11:1090051. [Crossref] [PubMed]
- Wu JN, Peng T, Xie F, et al. Association of thyroid dysfunction and autoantibody positivity with the risk of preterm birth: a hospital-based cohort study. BMC Pregnancy Childbirth 2022;22:473. [Crossref] [PubMed]
- Conner SN, Bedell V, Lipsey K, et al. Maternal Marijuana Use and Adverse Neonatal Outcomes: A Systematic Review and Meta-analysis. Obstet Gynecol 2016;128:713-23. [Crossref] [PubMed]