Association of gut microbiota composition and craniosynostosis
Highlight box
Key findings
• Gut microbiota was associated with craniosynostosis (CS) risk, and several taxa and specific functional pathways with significantly altered abundance have been identified in CS patients.
What is known and what is new?
• Previous studies have reported that gut microbiota biodiversity was relevant with osteoporosis and bone-related diseases.
• This study is the first population study to assess the association between gut microbiota diversity and CS using 16S rRNA sequencing. In this study, we found CS group had a smaller number of OTUs and bacterial taxa at each level of classification compare with non-CS group. In comparation with control group, the CS group had more abundant Staphylococcales and Lactobacillales at the order level, Enterococcaceae and Staphylococcaceae at the family level, Enterococcus and Staphylococcus at the genus level.
What is the implication, and what should change now?
• These findings can provide epidemiologic evidence to demonstrate the underlying microbiota associated mechanism in the CS pathology, and develop microbiota modification therapy for the treatment and early prevention of CS.
Introduction
Craniosynostosis (CS) is a birth defect in which one or more bone sutures connecting the skull are closed prematurely, subsequently resulting in cranial deformity, increased intracranial pressure, mental retardation, and facial deformities (1,2). Sagittal suture, one of the most common CS, accounts for 40–55%, followed by coronal synostosis (20–25%) (3). The disease has been reported to occur 1/10,000–5/10,000 in countries such as the United States, France and Australia, and up to 1/2,500 globally (4,5).
Previous studies have shown that genetic and environmental factors play major roles in the development of CS, among which transforming growth factor (TGF), fibroblast growth factor/fibroblast growth factor receptor (FGF/FGFR), and Wnt signaling pathway variants have been clearly reported to affect the occurrence of CS (2,6). Alderman et al. (7) and Källén et al. (8) found that maternal smoking during pregnancy was closely associated with CS. Other factors such as parental education, occupation or maternal age may also influence the development of CS (9). However, the risk factors and molecular mechanisms of most CS, especially non-syndromic CS, remain unclear. In comparation with syndromic CS, non-syndromic CS is more common and is found only in the skull, and does not involve changes in other parts of the body (10). In recent years, with the development of high-throughput sequencing technology, studies have reported that gut microbiota biodiversity is relevant to osteoporosis and bone-related diseases with low bone mineral density (BMD) (11-13). However, there are few studies on gut microbiota association with CS.
Gut microbiota, or microbiome is an important ecosystem in the human digestive tract, which is regarded as a multicellular organ in the body. When the microecological disorder occurs in the gut microbiota, the immune system and/or endocrine system of the body can be disrupted, thereby causing a series of diseases (14,15). The gut microbiota, comprising of 1014 bacteria, is regulated by 5 million genes, 66.6% of which in the human body is unique to each individua (16,17). Recently, the study of the association between gut microbiota and various diseases has become a hot topic in medical research. In terms of bone metabolism, Weaver et al. found that changes in gut microbiota induced by diet (such as prebiotics) increased fiber fermentation, and then produced short-chain fatty acids to promote bone development (18).
However, to date, the epidemiological evidence of gut microbiota and CS is very weak. Therefore, it is very necessary and urgent to investigate the association between gut microbiota and CS. To this end, we analyzed the gut microbiota specificity of CS and explored the possible mechanism of these microbiota by 16S rRNA gene sequencing based on 30 non-syndromic CS patients who met the inclusion criteria and 30 non-CS. We present this article in accordance with the STROBE reporting checklist (available at https://tp.amegroups.com/article/view/10.21037/tp-23-76/rc).
Methods
Sample collection, DNA extraction and PCR experiments
The study was conducted in accordance with the Declaration of Helsinki (as revised in 2013). The study was approved by the Ethics Committee of Children’s Hospital of Nanjing Medical University (No. 201908226-1), and informed consent was taken by participants’ parents or legal guardians. Participants was initially included in this study when the patients were diagnosed with sagittal suture synostosis. A total of 93 CS subjects and 71 non-CS subjects with head injury treated in Children’s Hospital of Nanjing Medical University of Jiangsu Province from June 2021 to March 2022 were initially included in this study. Participants were excluded for the following reasons: (I) pre-existing known syndromic disease; (II) diagnosed with metopic suture, coronal suture, or lambdoid suture; (III) diagnosed with chromosomal abnormalities or some common mutations in FGF/FGFR and Wnt signaling pathway; (IV) ineligible sample quality or sequencing requirements (such as quality of DNA extracted). Thirty CS patients and 30 non-CS were finally enrolled in the present study. Fecal samples were collected in 2 mL EP tubes, frozen, then transported to the laboratory within 4 hours and stored at −80 ℃ until DNA was extracted.
The QIAamp Fast DNA Stool Mini Kit (QIAGEN, Chatsworth, CA, USA) was used to extract and purify total genomic DNA from fecal samples. The amount of extracted DNA was then assessed using SpectraMax190 (Molecular Devices, Sunnyvale, CA, USA). DNA integrity was detected by 1% agarose-gel electrophoresis and stored at −20 ℃ for PCR amplification.
After cleaning with AMPure XP beads (Beckman Coulter, Shanghai, China), 16S rRNA gene amplicons were connected to dual exponential and Illumina sequencing adapters. Then, Nextera XT Index Kit (Illumina China, Shanghai, China) was used for PCR amplification. This study used bacterial genomic DNA as a template to amplify the V3-V4 hypervariable region of 16S rRNA gene with the 2× KAPA HiFi HotStart ReadyMix (Shanghai Dobio, Shanghai, China) primers set at 341F (5'-CCTAYGGGRBGCASCAG-3') and 806R (5'-GGACTACNNGGGTATCTAAT-3'). Each sample was amplified three times by polymerase chain reaction (PCR) and the PCR products were purified using AMPure XP beads. Then, the products were quantified and standardized by Agilent Technologies 2100 Bioanalyzer (Agilent, Shanghai, China) to form a sequencing library (SMRT Bell). The completed library was inspected for quality first, and the qualified library was sequenced using PacBio Sequel. Data from PacBio Sequel was in bam format, which was transformed into circular consensus sequencing (CCS) files with SMRT Link analysis software. Data of different samples was identified by Barcode sequence and converted to FASTQ format.
Sequence denoising or clustering
In this study, Vsearch method was used to perform a series of operations on the reads obtained such as primers removal, splicing, quality filtering, weight removal, chimerism removal and clustering. These sequences would be aggregated and quantified as the manner of operational taxonomic units (OTUs). Then, OTUs was used as the basic unit of calculation for further analysis. According to the algorithm principle, the sequence with 97% identity and the highest frequency was the representative sequence of OTUs and will also be used for various subsequent analyses (19).
Statistical analysis
In this study, QIIME2 was used to analyze the species composition and visualized results in the samples. Wilcoxon rank test was used to compare inter-sample alpha diversity index (such as Chao1, Observed species indices, Shannon, Simpson, Michaelis Menten fit, Pielou’s evenness, and Good’s coverage index), and Principal Coordinate Analysis (PCoA) and Nonmetric Multidimensional Scaling (NMDS) methods were used to analyze inter-group beta diversity. Linear discriminant analysis effect size (LEfSe) was used to analyze the distribution of bacteria at different classification levels and identify robust differential species, namely biomarkers. For Kyoto Encyclopedia of Genes and Genomes (KEGG) pathway enrichment analyses, differential proteins were used to perform enrichment tests of KEGG pathways using two-tailed Fisher’s exact test method with a filter value of 0.05 in the FDR (false discovery rate) term filter mode and removal of duplicates. Phylogenetic Investigation of Communities by Reconstruction of Unobserved States (PICRUSt2) was adopted to predict sample functional abundance. R software (V4.1.1) was used for data sorting and analysis. Significant difference was set at two-sided P<0.05.
Results
From June 2021 to March 2022, fecal samples from 30 CS patients (26 males; 4 females) and 30 non-CS (23 males; 7 females) were included in the final sequencing. A total of 8,059,719 sequences were detected in this study, among which the length of the included high-quality sequences was about 400–430 BP (Figure S1). As can be seen from Table 1, the distribution of age and sex between CS group and non-CS group was not significantly different (P=0.068 for age; P=0.317 for sex). In the CS group, there were 42 species, 121 genera, 56 families, 34 orders, 16 classes, and 11 phyla. The CS group have significantly lower levels of family, genus, and species than non-CS group (All P<0.05).
Table 1
Variables | Non-craniosynostosis (n=30) | Craniosynostosis (n=30) | P value |
---|---|---|---|
Age [medium (IQR)], months | 11.0 (9.0, 12.0) | 4.5 (3.2, 13.0) | 0.068 |
Gender, n (%) | 0.317 | ||
Female | 7 (23.33) | 4 (13.33) | |
Male | 23 (76.67) | 26 (86.67) | |
Phylum, n | 13 | 11 | 0.286 |
Class, n | 18 | 16 | 0.339 |
Order, n | 37 | 34 | 0.073 |
Family, n | 62 | 56 | 0.002 |
Genus, n | 139 | 121 | 0.001 |
Species, n | 70 | 42 | 0.009 |
OTU, n | 2041 | 1664 | 0.6098 |
IQR, inter-quartile range; OTU, operational taxonomic units.
As is shown in the Figure 1A, the CS group had a lower number of bacterial species at all classification levels than the non-CS group. Moreover, we found that the CS group had a relatively lower proportion of species and genus, but a higher distribution at all other levels than the non-CS group. To further explore the distribution of bacteria in CS group, we compared the two groups at the genus level. And we observed that the relative abundance of Bifidobacterium, Escherichia Shigella, Veillonella, Streptococcus, Faecalibacterium and Clostridium_sensu_stricto_1 was higher in the CS group, while the abundance of Bacteroides and Subdoligranulum were opposite (Figure 1B). Furthermore, we found that Bifidobacterium occupied the largest proportion in both order and family level, and the enrichment degree of CS group was higher than that of non-CS group (Figure S2). Additionally, Figure 1C shows the relative distribution of different classification levels of each fecal sample in the CS group (F_397-F_486) and the non-CS group (F_139-F_207).
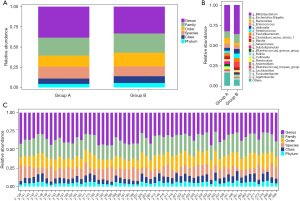
As shown in Figure 2A, the curve rose sharply to a flat state with the increase of the number of samples, indicating that the amount of sequencing data was reasonable, and the included sample size was sufficient to reflect the species composition of the community. The results of Rank abundance curve showed that the curve of the CS group was steeper and less uniform than non-CS group, that is, there was a larger difference in the distribution of flora abundance (Figure 2B). Although there were no statistically significant differences in Pielou’s evenness, Shannon and Simpson indexes between the two groups (all P>0.05), but most alpha-diversity indexes including Chao1 (P=0.026), Good’s coverage (P=0.042), Michaelis-Menten fit (P=0.018) and Observed Specie (P=0.021) achieve significance, indicating gut microbiota in CS group have changed (Figure 2C-2I).
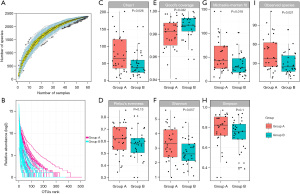
Based on the PCoA, we can observe the plane distance and distribution between different samples (Figure 3A-3C). Then, we further calculated the Bray-Curtis distance to reflect the sample differential distance in the distance matrix to the greatest extent (Figure 3D). Figure 3E showed that the similarity of samples in the same group was relatively high, but there were still some samples with no significant difference between groups based on the unweighted pair-group method with arithmetic means (UPGMA). Although the two groups were not completely separated by PCoA and there was no statistical difference in PERMANOVA test (all P>0.05), LEfSe analysis revealed significant differences in some bacterial genera between the two groups.
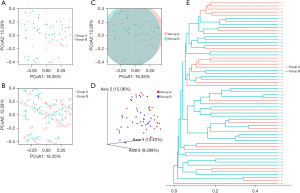
As shown in Figure 4, Staphylococcales (order), Lactobacillales (order), Enterococcaceae (family), Staphylococcaceae (family), Enterococcus (genus) and Staphylococcus (genus) were enriched in the CS group, and Coriobacteriia (class), Peptostreptococcales_Tissierellales (order), Coriobacteriales (order), Peptostreptococcaceae (family), Eggerthellaceae (family), Ruminococcus_gnavus_group (genus), Eubacterium_hallii_group (genus), and Romboutsia (genus) were enriched in non-CS group (all P<0.05).
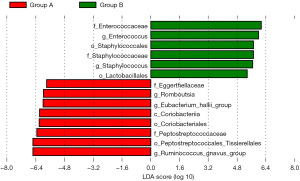
The Venn chart showed that the CS group had fewer OTUs than the non-CS group (1,664 vs. 2,041), with 1325 OTUs distributed between the two groups (Figure 5A). Then, we used the abundance data of the genera with the top 50 average abundance to observe the enriched bacterial genera between the two groups (Figure 5B). Figure S3 showed the enrichment distribution of other classification levels (e.g., phylum, class, order, family) between the two groups. In addition, Figure 5C showed the taxological hierarchy of the sample communities from phylum to genus, which further confirmed the results of Figure 4.
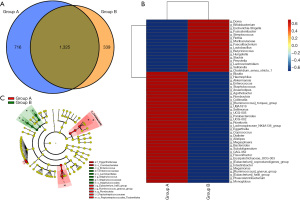
We use PICRUSt2 and KEGG database to predict gut microbiota functional abundance (Figure S4). Figure S5 showed the functional composition at different KEGG classification levels. For example, at the level of Class 1-KEGG classification, the main enrichment function was Metabolism, followed by Genetic information processing, Environmental information processing and Cellular processes. Then, we used STAMP to conduct differential analysis of KEGG function. And we found there were six metabolic pathways including oligomerization domain (NOD)-like receptor signaling pathway, apoptosis, hypertrophic cardiomyopathy (HCM), endocytosis, polycyclic aromatic hydrocarbon (PAH) degradation, Penicillin and cephalosporin biosynthesis significantly differed between the two groups (Figure 6). Furthermore, PAH degradation and Penicillin and cephalosporin biosynthesis metabolic pathways were more abundant in CS group than in non-CS group.
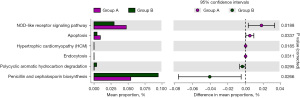
Discussion
Gut microbiota is associated with a variety of metabolic diseases, and its diversity has also been shown to be associated with bone metabolism-related diseases (13,18). Our study is the first population study to assess the association between gut microbiota diversity and CS using 16S rRNA high-throughput gene sequencing. In this study, we found that the CS group have significantly lower levels of family, genus, and species than non-CS group. In comparation with the non-CS group, the CS group had more abundant Staphylococcales and Lactobacillales at the order level, Enterococcaceae and Staphylococcaceae at the family level, Enterococcus and Staphylococcus at the genus level. In addition, functional prediction revealed pathways involving PAH degradation, and penicillin and cephalosporin biosynthesis were more abundant in CS group than in non-CS group.
Previous studies have reported that changes in gut microbiota were associated with bone development outcomes (20,21). Similar results were found in this study, where alpha diversity analyses showed gut microbiota diversity significantly differed between the two groups. Alpha diversity revealed that significant changes in the structure of gut microbiome were found in patients with CS at a wide range of phylogenetic levels. However, another clinical study showed a decreasing trend of alpha diversity in patients with osteoporosis compared with the normal BMD control (22), which is inverse to our results. These discrepancies might potentially be due to the different numbers of included patients, population information (age, gender compositions), living habits including diet and exercise, and medicine use including antibiotics and anti-osteoporosis drugs in these clinical studies. In this study, we found that Bacteroides (genus) was significantly enriched in the non-CS group, while Firmicutes (phylum) had no difference between the two groups. Previous studies have found that the ratio of Firmicutes/Bacteroidetes was negatively correlated with the loss of bone, which was consistent with our results (11,13). Then, Bifidobacterium occupied the largest proportion at the order, family and genus level, and the enrichment degree of CS group was higher than that of non-CS group. Li et al. (11) found that BMD increased with the increase of Bifidobacterium abundance, while Xu et al. (13) found that the BMD of hip decreased with the increase of Bifidobacterium abundance, which may be attributed to the inconsistency of bone development and density in different parts. Furthermore, Actinobacteria occupied the largest proportion at the class level, and the enrichment level of CS group was higher than that of non-CS group. That is, bone density increased with the increase of Actinobacteria abundance, which was consistent with the findings of Li et al. (11) and Ma et al. (12).
LEfSe analysis revealed Staphylococcales (order), Staphylococcaceae (family), and Staphylococcus (genus) were significantly enriched in the CS group. Staphylococcus, a gram-positive coccus, often clustered into grape clusters, most of which are non-pathogenic bacteria, while a few can cause diseases. Roshan et al. (23) reported a case of high IgE syndrome (HIES) patient, whose CT results revealed the CS accompanied by scalp abscess in the right parietal region. Staphylococcus aureus could be cultured with the concentrated fluid of the scalp abscess, which suggested that staphylococcus was associated with cranial overgrowth. Moreover, Lactobacillales was also observed significant abundant in the CS group. Previous study has reported that the beneficial bacteria (probiotics) could increase BMD, and the Lactobacillus play an important role (24), which was similar with our results. In addition, we also found Enterococcus was enriched in the CS group. Enterococcus is a symbiotic Gram-positive bacterium that exists in the oral cavity and gastrointestinal tract. Enterococcus faecalis, one of common Enterococcus, is mainly found in the root canal of patients with refractory apical periodontitis, accompanied by inflammation and bone regeneration dysfunction (25). Wang et al. (26) found that diet with Enterococcus faecium can change the gut microbiota of broilers and increase the relative abundance of short-chain fatty acid (SCFA) producing bacteria, subsequently promoting intestinal phosphorus absorption and metabolic activity of bone formation. However, Park et al. (27) found that Enterococcus faecalis would reduce the expression of Runx2, osteoblast, β-catenin, osteocalcin and type I collagen, inhibit the differentiation of osteoblasts, and increase the induction of chemokines, subsequently leading to the recruitment of inflammatory immune cells, which was contrary to our results. We hypothesized that this might be due to the different parts of bone development, since this study mainly focused on refractory apical periodontitis. Certainly, wo only found the difference in the genus level of Enterococcus.
Extensive data across the field of gut microbiota have demonstrated that gut microbiota affects host bone metabolism mainly through metabolic, endocrine and immune pathways (28). In this study, we observed that six metabolic pathways significantly differed between the two groups, especially pathways involving PAH degradation, and penicillin and cephalosporin biosynthesis were more abundant in CS group than in non-CS group. Based on the National Health and Nutrition Examination Survey (NHANES) database, some population studies observed that urine PAH metabolites were significantly associated with an increased level of N-terminal peptides, speculating that PAHs exposure induced bone resorption and an imbalance of bone turnover (29,30). This finding was consistent with the PAHs degradation observed in the CS group. For the Penicillin and cephalosporin biosynthesis, we hypothesized that it might be drug residues from the treatment (31).
Our study has the following advantages. First, this is the first study to evaluate the relationship between gut microbiota diversity and CS population through high-throughput gene sequencing. Second, this study demonstrated the gut microbiota distribution of CS subjects and found possible dominant bacteria. Third, this study elaborated the potential pathological mechanism of CS from the perspective of gut microbiota, providing the clue for the etiology study of CS. Fourth, it is difficult to detect the CS, and this study provided a reference for the early diagnosis of this disease.
Certainly, we had to admit that there were some shortcomings in this study. First, this study was only a case-control study, which was unable to dynamically observe the changes of gut microbiota in different pathological stages of CS, resulting in poor causality. Future studies can focus on cohort or longitudinal studies of this disease. Second, although we tried our best to guarantee that participants had similar eating habits in the two groups, differences in specific diets may still interfere with the diversity and abundance of gut microbiota. Third, 16S rRNA sequencing analysis may also have technical limitations such as sequencing abundance, which may not distinguish certain rare species. Fourth, our non-CS group were the patients treated with minor and minor head injuries for the first time, but traumatic brain injury has been reported to be associated with intestinal dysfunction. Future studies should try to select appropriate healthy controls to avoid the possible bias. Despite these limitations, our study provides a detailed introduction to the gut microbiota of CS subjects and may facilitate the early diagnosis and treatment of CS.
Conclusions
Taken together, the presented data indicate that the composition and abundance of gut microbiota differed between CS group and non-CS group. Several taxa and specific functional pathways with significantly altered abundance have been identified in CS patients. These findings can provide epidemiologic evidence to demonstrate the underlying microbiota associated mechanism in the CS pathology, and develop microbiota modification therapy for the treatment and early prevention of CS.
Acknowledgments
Funding: This work was supported by funding from
Footnote
Reporting Checklist: The authors have completed the STROBE reporting checklist. Available at https://tp.amegroups.com/article/view/10.21037/tp-23-76/rc
Data Sharing Statement: Available at https://tp.amegroups.com/article/view/10.21037/tp-23-76/dss
Peer Review File: Available at https://tp.amegroups.com/article/view/10.21037/tp-23-76/prf
Conflicts of Interest: All authors have completed the ICMJE uniform disclosure form (available at https://tp.amegroups.com/article/view/10.21037/tp-23-76/coif). The authors have no conflicts of interest to declare.
Ethical Statement: The authors are accountable for all aspects of the work in ensuring that questions related to the accuracy or integrity of any part of the work are appropriately investigated and resolved. The study was conducted in accordance with the Declaration of Helsinki (as revised in 2013). The study was approved by the Ethics Committee of Children’s Hospital of Nanjing Medical University (No. 201908226-1), and informed consent was taken by participants’ parents or legal guardians.
Open Access Statement: This is an Open Access article distributed in accordance with the Creative Commons Attribution-NonCommercial-NoDerivs 4.0 International License (CC BY-NC-ND 4.0), which permits the non-commercial replication and distribution of the article with the strict proviso that no changes or edits are made and the original work is properly cited (including links to both the formal publication through the relevant DOI and the license). See: https://creativecommons.org/licenses/by-nc-nd/4.0/.
References
- Xu C, Xu J, Zhang X, et al. Serum nickel is associated with craniosynostosis risk: Evidence from humans and mice. Environ Int 2021;146:106289. [Crossref] [PubMed]
- Twigg SR, Wilkie AO. A Genetic-Pathophysiological Framework for Craniosynostosis. Am J Hum Genet 2015;97:359-77. [Crossref] [PubMed]
- Osborn AJ, Roberts RM, Dorstyn DS, et al. Sagittal Synostosis and Its Association With Cognitive, Behavioral, and Psychological Functioning: A Meta-analysis. JAMA Netw Open 2021;4:e2121937. [Crossref] [PubMed]
- Dempsey RF, Monson LA, Maricevich RS, et al. Nonsyndromic Craniosynostosis. Clin Plast Surg 2019;46:123-39. [Crossref] [PubMed]
- Mathijssen IMJWorking Group Guideline Craniosynostosis. Updated Guideline on Treatment and Management of Craniosynostosis. J Craniofac Surg 2021;32:371-450. [Crossref] [PubMed]
- Azoury SC, Reddy S, Shukla V, et al. Fibroblast Growth Factor Receptor 2 (FGFR2) Mutation Related Syndromic Craniosynostosis. Int J Biol Sci 2017;13:1479-88. [Crossref] [PubMed]
- Alderman BW, Bradley CM, Greene C, et al. Increased risk of craniosynostosis with maternal cigarette smoking during pregnancy. Teratology 1994;50:13-8. [Crossref] [PubMed]
- Källén K. Maternal smoking and craniosynostosis. Teratology 1999;60:146-50. [Crossref] [PubMed]
- Wu X, Gu Y. Signaling Mechanisms Underlying Genetic Pathophysiology of Craniosynostosis. Int J Biol Sci 2019;15:298-311. [Crossref] [PubMed]
- Timberlake AT, Furey CG, Choi J, et al. De novo mutations in inhibitors of Wnt, BMP, and Ras/ERK signaling pathways in non-syndromic midline craniosynostosis. Proc Natl Acad Sci U S A 2017;114:E7341-7. [Crossref] [PubMed]
- Li C, Huang Q, Yang R, et al. Gut microbiota composition and bone mineral loss-epidemiologic evidence from individuals in Wuhan, China. Osteoporos Int 2019;30:1003-13. [Crossref] [PubMed]
- Ma S, Qin J, Hao Y, et al. Association of gut microbiota composition and function with an aged rat model of senile osteoporosis using 16S rRNA and metagenomic sequencing analysis. Aging (Albany NY) 2020;12:10795-808. [Crossref] [PubMed]
- Xu Z, Xie Z, Sun J, et al. Gut Microbiome Reveals Specific Dysbiosis in Primary Osteoporosis. Front Cell Infect Microbiol 2020;10:160. [Crossref] [PubMed]
- Ley RE, Hamady M, Lozupone C, et al. Evolution of mammals and their gut microbes. Science 2008;320:1647-51. [Crossref] [PubMed]
- Wastyk HC, Fragiadakis GK, Perelman D, et al. Gut-microbiota-targeted diets modulate human immune status. Cell 2021;184:4137-4153.e14. [Crossref] [PubMed]
- Turnbaugh PJ, Ley RE, Hamady M, et al. The human microbiome project. Nature 2007;449:804-10. [Crossref] [PubMed]
- Nagpal R, Yadav H, Marotta F. Gut microbiota: the next-gen frontier in preventive and therapeutic medicine? Front Med (Lausanne) 2014;1:15. [Crossref] [PubMed]
- Weaver CM. Diet, gut microbiome, and bone health. Curr Osteoporos Rep 2015;13:125-30. [Crossref] [PubMed]
- Biobank. UK. Biomarker assay quality procedures: approaches used to minimise systematic and random errors (and the wider epidemiological implications): version 1.2. Available online: https://biobank.ctsu.ox.ac.uk/crystal/crystal/docs/biomarker_issues.pdf
- Ibáñez L, Rouleau M, Wakkach A, et al. Gut microbiome and bone. Joint Bone Spine 2019;86:43-7.
- Yan J, Charles JF. Gut Microbiome and Bone: to Build, Destroy, or Both? Curr Osteoporos Rep 2017;15:376-84. [Crossref] [PubMed]
- Wang J, Wang Y, Gao W, et al. Diversity analysis of gut microbiota in osteoporosis and osteopenia patients. PeerJ 2017;5:e3450. [Crossref] [PubMed]
- Roshan AS, Janaki C, Parveen B, et al. Rare association of hyper IgE syndrome with cervical rib and natal teeth. Indian J Dermatol 2009;54:372-4. [Crossref] [PubMed]
- Villa CR, Ward WE, Comelli EM. Gut microbiota-bone axis. Crit Rev Food Sci Nutr 2017;57:1664-72. [Crossref] [PubMed]
- Zhu X, Wang Q, Zhang C, et al. Prevalence, phenotype, and genotype of Enterococcus faecalis isolated from saliva and root canals in patients with persistent apical periodontitis. J Endod 2010;36:1950-5. [Crossref] [PubMed]
- Wang W, Cai H, Zhang A, et al. Enterococcus faecium Modulates the Gut Microbiota of Broilers and Enhances Phosphorus Absorption and Utilization. Animals (Basel) 2020;10:1232.
- Park OJ, Kim J, Yang J, et al. Enterococcus faecalis Inhibits Osteoblast Differentiation and Induces Chemokine Expression. J Endod 2015;41:1480-5. [Crossref] [PubMed]
- Hernandez CJ, Guss JD, Luna M, et al. Links Between the Microbiome and Bone. J Bone Miner Res 2016;31:1638-46. [Crossref] [PubMed]
- Chen YY, Kao TW, Wang CC, et al. Association between polycyclic aromatic hydrocarbons exposure and bone turnover in adults. Eur J Endocrinol 2020;182:333-41. [Crossref] [PubMed]
- Guo J, Huang Y, Bian S, et al. Associations of urinary polycyclic aromatic hydrocarbons with bone mass density and osteoporosis in U.S. adults, NHANES 2005-2010. Environ Pollut 2018;240:209-18. [Crossref] [PubMed]
- Thabit AK, Fatani DF, Bamakhrama MS, et al. Antibiotic penetration into bone and joints: An updated review. Int J Infect Dis 2019;81:128-36. [Crossref] [PubMed]