Bioinformatic analysis of immune-related transcriptome affected by IFIT1 gene in childhood systemic lupus erythematosus
Highlight box
Key findings
• IFIT1-affected transcriptome is involved in immune cell infiltration and tertiary lymphoid structure formation in childhood SLE.
What is known and what is new?
• The interferon-related immune pathway involving IFIT1 gene is associated with the pathogenesis of SLE.
• IFIT1 gene changes the transcriptional features, which affects immune cell infiltration and tertiary lymphoid structure formation in child SLE.
What is the implication, and what should change now?
• This study provides a comprehensive overview of IFIT1-regulated genes in childhood SLE, and functional annotation requires experimental validation.
Introduction
Systemic lupus erythematosus (SLE) is defined as a chronic multisystem autoimmune/inflammatory disease presenting heterogeneous clinical features ranging from mild cutaneous symptoms to multiple organ failure (1,2). Childhood SLE shows great disease activity, a high incidence of irreversible organ lesions, and a more aggressive clinical course, which may be profoundly due to infection and disease recurrence (3-6). This autoimmune disease is characterized by abnormal inflammatory responses due to genetic, epigenetic, immunoregulatory, ethnic, hormonal, and environmental factors (7-11). Immunological abnormalities are increasingly implicated in the pathogenesis of SLE (12,13). Researchers have identified that a subset of immune cells is associated with the development of SLE, including T helper (Th)17 memory cells, naïve CD4+ T cells, B cells, macrophages, and neutrophils (14-18).
The pathophysiology of SLE includes environmental factors that trigger the activation of the innate and adaptive immune systems (19). It has been reported that SLE may be related to type I interferon-induced hypomethylation in naïve CD4+ T cells, suggesting a genetic mechanism for type I interferon hyperresponsiveness in lupus T cells (20). In SLE, type I interferon is a primary pathogenic cause, and high systemic type I interferon activity results in a propensity for severe manifestations such as lupus nephritis (21). Recent evidence has well established the association between high type I interferon activity and clinical manifestations of lupus nephritis and specific autoantibodies, including anti-ribonucleoprotein, anti-Ro, anti-Sm, and anti-double-stranded antibodies (22,23). To diminish chronic inflammation and end-organ injuries, type I interferon has been described as a potential target (24).
Interferon-induced protein with tetratricopeptide repeats 1 (IFIT1) is an antiviral protein that recognizes 5’-triphosphate RNA of microbial structures during the process of antiviral innate immunity (25). Viral infection enhances the specific abundance of the IFIT1 protein, which is antagonized by the IFIT complex through sequestering specific viral nucleic acids (25). Interferon-induced IFIT1 is strongly expressed in cutaneous lupus erythematosus skin (26). Furthermore, the interferon-related immune pathway involving IFIT1 has been related to the pathogenesis of SLE (27). The IFIT1 gene may play roles in SLE by inducing the activation of Rho protein by interacting with Rho/Rac guanine nucleotide exchange factor (28). The IFIT1 gene is described as a potential candidate target of SLE for therapeutic intervention (28). However, the expression profile of IFIT1 gene has not been investigated in childhood SLE.
Compared to adult SLE, there is a great challenge to manage childhood SLE. It is needed to delineate IFIT1-mediated immunopathogenesis, which may provide new insights to the diagnostic therapy. Here, this study aimed to investigate the diagnostic potential of IFIT1 and differentially expressed genes (DEGs) affected by IFIT1 in childhood SLE. Particularly, it is unclear whether IFIT1 mediates immune cell infiltration. The current study then assessed immune cell infiltration, immune checkpoints and tertiary lymphoid structures in childhood SLE. We present this article in accordance with the TRIPOD reporting checklist (available at https://tp.amegroups.com/article/view/10.21037/tp-23-365/rc).
Methods
Data collection and normalization
Dataset GSE11909 (Platform: GPL96) was downloaded from the Gene Expression Omnibus (GEO) (https://www.ncbi.nlm.nih.gov/gds). The dataset provides the transcriptional profiles of peripheral blood mononuclear cells (PBMCs) from 103 cases and 12 healthy individuals. The clinical and demographic information has been listed in the study of Chaussabel et al. (29). Data normalization was performed using the R package “Limma”. The study was conducted in accordance with the Declaration of Helsinki (as revised in 2013).
Principal component analysis and IFIT1 expression analysis
Principal component analysis (PCA) was implemented using the R packages “FactoMineR” and “FactoExtra”. PCA plots were computed, and the IFIT1 expression level was presented. The transcription level of the IFIT1 gene is shown in the violin plot using the function ViolinPlot from the R package.
Receiver operating characteristics (ROC) analysis of the diagnostic potential of the IFIT1 gene
The ROC curve is commonly used in clinical settings to assess the performance of classifiers. In the case of an area under the curve (AUC) >0.5, the closer the AUC is to 1, the better the diagnosis. The ROC assessment has low accuracy when the AUC is between 0.5 and 0.7, some accuracy when the AUC is between 0.7 and 0.9, and high accuracy when the AUC is above 0.9. Here, ROC analysis was performed to differentiate between IFIT1-high and -low subjects. The diagnostic performance of IFIT1 gene expression for SLE was analyzed by calculating AUC. The ROCs were plotted using the R package “pROC”.
Analysis of differentially expressed genes (DEGs) between the case and control groups
To screen the DEGs between the case and control groups, the R packages “Limma” and “DESeq2” were used to analyze gene expression data. The cutoff criteria were log2(fold change) more than 1 and corrected P values less than 0.05. Childhood SLE patients were grouped into two groups: higher-than-median (high group) and low-than-median (low group) IFIT1 gene expression groups. DEGs between the high group and low group were identified with log2(fold change) more than 1 and corrected P values less than 0.01. A Venn diagram was plotted using the R package “VennDiagram”. The distribution of IFIT1-affected dysregulated DEGs was presented using a 4-quadrant plot, and the expression profile was illustrated using a heatmap. Functional analysis of IFIT1-affected dysregulated DEGs, including biological process (BP) and Kyoto Encyclopedia of genes and genomes (KEGG) pathway analyses, was performed using the R package “clusterprofiler”.
IFIT1-based least absolute shrinkage and selection operator (LASSO) model
The R package “LASSO” was used to select the effective marker genes (AUC >0.85) for diagnosis from the gene set of IFIT1-affected dysregulated DEGs. Regression coefficients were narrowed toward zero. The optimal lambda was selected according to the minimum cross-validation error in 10-fold cross-validation. The expression profile of the selected marker genes was presented in the box plot using the R package “ggplot2”. The semantic similarity among gene ontology terms and marker genes was measured using the R package “GOSemSim”.
Immune infiltration analysis
Analysis of immune infiltration was performed using the R package “CIBERSORT”. Immune cell infiltration was analyzed by machine learning and deconvolution algorithms to assess the relationship between gene expression and immune cell infiltration. The infiltration abundance of immune cells was plotted using the R package heatmap. The R package PlotCor was used to plot correlation scatter plots, which aimed to indicate the correlation between the abundance of infiltrating immune cells and IFIT1 expression.
Results
mRNA expression of IFIT1 gene in children with SLE
PCA was performed to reduce dimensionality, compress data, extract feature data, and visualize the distribution of the subjects. Figure 1A shows that the transcriptome data were able to stratify childhood patients with SLE. The childhood patients with SLE showed high mRNA levels of the IFIT1 gene compared to the controls. The violin plot also showed higher mRNA levels of the IFIT1 gene in the case group than in the control group (Figure 1B). Considering the high expression of the IFIT1 gene in childhood patients with SLE, we estimated the predictive performance of the IFIT1 gene. Figure 1C indicates that IFIT1 showed the potential to serve as a marker for childhood SLE, which was evidenced by the results from the ROC curve with an AUC value of 0.947.
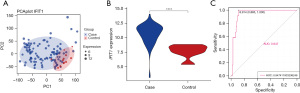
DEGs in childhood SLE patients compared to healthy individuals
In this study, we obtained 826 upregulated DEGs and 4,111 downregulated DEGs in the case group compared to the control group (Figure 2A up). A total of 208 upregulated DEGs and 214 downregulated DEGs were identified in the high group compared to the low group (Figure 2A down). The Venn diagram shows the intersections of DEGs (Figure 2B). A total of 249 genes were recognized as DEGs among the 4 groups. Here, we defined the 249 DEGs as the dysregulated genes affected by IFIT1. Next, IFIT1-affected dysregulated DEGs were classified based on the expression level of the IFIT1 gene. Figure 2C shows that the intersections of upregulated DEGs between the case and high groups were defined as the positive-functional genes, as well as the intersections of downregulated DEGs in the case group and upregulated DEGs in the high group. The negative-functional genes were the intersections of downregulated DEGs between the case and high groups, as well as the intersections of upregulated DEGs in the case group and downregulated genes in the high group. Figure 2D indicates the mRNA expression signature of IFIT1-affected dysregulated DEGs. These IFIT1-affected dysregulated DEGs were involved in regulating the defense response to virus, type I interferon signaling pathway, and cellular response to type I interferon (Figure 3A) and mediating signaling pathways related to hepatitis C, proteasome, and RIG-I-like receptor (Figure 3B).
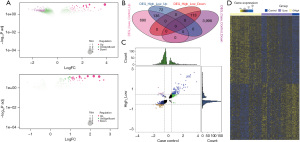
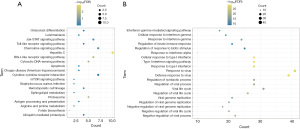
Clinical diagnostic potential of the LASSO model
LASSO regression is a common data mining method in machine learning. LASSO performs variable screening and complexity adjustment while fitting a generalized linear model, which can better solve the problem of multicollinearity in regression analysis. Marker genes were selected from the IFIT1-affected dysregulated DEGs (AUC value >0.85). As a result, 7 marker genes were obtained, including IFIT1, LGALS9, RPS10L, RP3-334F4.1, IFI44, RP6-11O7.2, and RPL7P52. Lambda plots are shown in Figure 4A, demonstrating the predictive performance of the model. The LASSO model plot is shown in Figure 4B, which indicates the confidence level of the model under the corresponding log(lambda). The ROC curves of the LASSO model are shown in Figure 4C, suggesting that the IFIT1-based model showed good diagnostic efficacy for childhood SLE patients (AUC value =0.9950). In addition, the diagnostic efficacy was further proven (AUC value =0.9474), as shown in Figure 4D. Box plots were drawn to demonstrate the transcriptional profile of marker genes. Figure 4E indicates that IFI44 mRNA was highly expressed in the case group compared to the control group. The semantic similarity among gene ontology terms and marker genes was analyzed. Figure 4F shows that IFI44, IFIT1, and LGALS9 were significantly associated with biological processes of SLE.
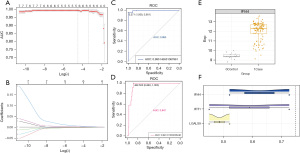
Immune cell infiltration was affected by IFIT1
A large number of immune cells are present in the microenvironment of different diseases, including lymphocytes, dendritic cells, monocytes/macrophages, granulocytes, and mastocytes. Immune cells play an important role in the development of SLE. Figure 5A suggests that the level of immune cell infiltration was low in IFIT1 high expression samples. Figure 5B shows that IFIT1 expression was significantly and positively correlated with the infiltration abundance of cells such as activated mast cells. The results in Figure 5C show that the marker genes of the IFIT1-based model were significantly related to immune cell abundance. Immune checkpoints are a set of molecules expressed on immune cells that regulate the degree of immune activation. Figure 5D shows that IFIT1-based model genes were significantly correlated with some immune checkpoint-related genes and tertiary lymphoid structural marker genes.
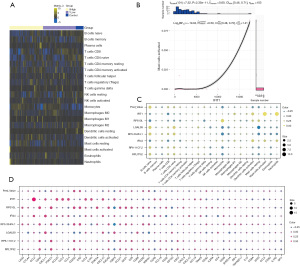
Discussion
SLE is a complex and multifactorial disease that manifests as significant immunological abnormalities (1,2). Its etiologies may be related to hormonal, genetic and environmental factors (30). IFIT1 was identified as an interferon-induced gene that plays roles in SLE by interacting with Rho/Rac guanine nucleotide exchange factor (28). In the current study, we revealed that IFIT1 altered the transcriptional signatures in childhood SLE. IFIT1-affected genes were related to immune cell infiltration, immune checkpoints, and tertiary lymphoid structure formation.
As an interferon-induced gene, the expression profile of the IFIT1 gene has been related to the diverse immune phenotype of SLE, and the IFIT1 gene was overexpressed in SLE patients (28). It has been reported that IFIT genes may be involved in immune microenvironment of cardiovascular disease (31). However, the exact mechanisms have not been reported. The expression level of IFIT1 in childhood SLE remains uninvestigated. Our results consistently showed that IFIT1 mRNA expression was higher in childhood SLE patients than in controls. Additionally, Mähönen et al. found that IFIT1 and IFIT2 were strongly upregulated in keratinocytes in cutaneous lupus erythematosus (26). A study pointed out that the expression level of the IFIT1 gene may be positively correlated with the SLE activity index (32). IFIT1 overexpression was considered to be related to renal affection of SLE patients, which appears to be the molecular basis for the diverse immune phenotype of SLE (32). Considering the high expression of the IFIT1 gene in SLE, we assessed the diagnostic potential of IFIT1 for SLE patients. ROC analysis further confirmed the diagnostic performance of IFIT1 for childhood SLE patients. These results indicated that IFIT1 could be detected for the diagnosis of childhood SLE.
RNA sequencing data uncovered the distinct transcriptome architectures of SLE and showed specific marker genes related to autoimmunity (33,34). Next, we studied whether IFIT1 is implicated in the regulation of the transcriptome in patients with SLE. Compared to healthy individuals, 249 DEGs were defined as dysregulated genes affected by IFIT1. Functional analysis showed the enrichment of biological processes for the 249 DEGs, including defense response to virus, type I interferon signaling pathway, cellular response to type I interferon, and mediating signaling pathways related to hepatitis C, proteasome, RIG-I-like receptor. Type I interferon is a primary pathogenic factor of SLE, and a high level of type I interferon may result in the activation of immature myeloid dendritic cells, autoreactive T cells, B cells and cytotoxic CD8+ T cells (35). Its increase in circulation contributes to the presence of lupus nephritis, arthritis, mucocutaneous, and autoantibodies (23). Hence, we speculated that IFIT1 may participate in mediating inflammation in SLE through affecting immune gene expression and immune cell composition.
Among these 249 DEGs, 7 marker genes showed predictive potential for childhood systemic lupus erythematosus, including IFIT1, LGALS9, RPS10L, RP3-334F4.1, IFI44, RP6-11O7.2, and RPL7P52. The 7 marker genes were strongly related to immune cell infiltration, which has been consistently reported in previous studies (36-39). IFIT1 was correlated with CD8 T cells, activated mast cells and neutrophils. LGALS9 was related to naïve B cells and monocytes. RPS10L was predicted to be linked to naïve B cells, naïve CD4 T cells, and resting mast cells. RP3-334F4.1 may be associated with naïve B cells, naïve CD T cells, monocytes, and resting mast cells. IFI44 was correlated with naïve B cells, CD8 T cells, and activated dendritic cells. RP6-11O7.2 may mediate the function of naïve B cells, naïve CD4 T cells, and monocytes. RPL7P52 may be involved in the cellular function of naïve B cells, naïve CD4 T cells, and monocytes. In particular, the 7 marker genes were significantly correlated with a number of immune checkpoint-related genes and tertiary lymphoid structural marker genes, such as CCL (40), IGSF (41), and CXCL (42). For example, IFIT1 was positively related to CCL2, CCL20, CCL3, CCL4, CCL8, and IL1R1. RP6-11O7.2 and CD40 were positively related to PDCD1. These findings suggested that IFIT1 could be targeted to relieve inflammation in childhood SLE.
However, our study still showed limitations in some aspects, such as participants should be enrolled for the validation of the prediction model. Besides, the predictive genes or genes-related to childhood SLE should be experimentally confirmed, and their biological or clinical functions should be further studied. These mentioned limitations will be our future study focus.
Conclusions
This comprehensive study provides a detailed comparative overview of the DEGs regulated by IFIT1 in childhood SLE. Functional annotation revealed the important roles of these genes in mediating the defense response to virus, type I interferon signaling pathway, cellular response to type I interferon, and regulating signaling pathways related to hepatitis C, proteasome, RIG-I-like receptor. The identified predictive genes (IFIT1, RPS10L, IFI44, RP3-334F4.1, IGALS9 and RP6-11O7.2) might be responsible for the distribution landscape of immune cell infiltration. There were obvious correlations between the 7 marker genes and immune checkpoint genes, as well as signature genes related to tertiary lymphoid structure formation in childhood SLE.
Acknowledgments
Funding: This work was funded by
Footnote
Reporting Checklist: The authors have completed the TRIPOD reporting checklist. Available at https://tp.amegroups.com/article/view/10.21037/tp-23-365/rc
Peer Review File: Available at https://tp.amegroups.com/article/view/10.21037/tp-23-365/prf
Conflicts of Interest: All authors have completed the ICMJE uniform disclosure form (available at https://tp.amegroups.com/article/view/10.21037/tp-23-365/coif). The authors have no conflicts of interest to declare.
Ethical Statement: The authors are accountable for all aspects of the work in ensuring that questions related to the accuracy or integrity of any part of the work are appropriately investigated and resolved. The study was conducted in accordance with the Declaration of Helsinki (as revised in 2013).
Open Access Statement: This is an Open Access article distributed in accordance with the Creative Commons Attribution-NonCommercial-NoDerivs 4.0 International License (CC BY-NC-ND 4.0), which permits the non-commercial replication and distribution of the article with the strict proviso that no changes or edits are made and the original work is properly cited (including links to both the formal publication through the relevant DOI and the license). See: https://creativecommons.org/licenses/by-nc-nd/4.0/.
References
- Metry AM, Al Salmi I, Al Balushi F, et al. Systemic Lupus Erythematosus: Symptoms and Signs at Initial Presentations. Antiinflamm Antiallergy Agents Med Chem 2019;18:142-50. [Crossref] [PubMed]
- Murimi-Worstell IB, Lin DH, Nab H, et al. Association between organ damage and mortality in systemic lupus erythematosus: a systematic review and meta-analysis. BMJ Open 2020;10:e031850. [Crossref] [PubMed]
- Ferreira JCOA, Trindade VC, Espada G, et al. Epidemiology and management practices for childhood-onset systemic lupus erythematosus patients: a survey in Latin America. Clin Rheumatol 2018;37:3299-307. [Crossref] [PubMed]
- Silva CA. Childhood-onset systemic lupus erythematosus: early disease manifestations that the paediatrician must know. Expert Rev Clin Immunol 2016;12:907-10.
- Hiraki LT, Feldman CH, Marty FM, et al. Serious Infection Rates Among Children With Systemic Lupus Erythematosus Enrolled in Medicaid. Arthritis Care Res (Hoboken) 2017;69:1620-6. [Crossref] [PubMed]
- Silva MF, Ferriani MP, Terreri MT, et al. A Multicenter Study of Invasive Fungal Infections in Patients with Childhood-onset Systemic Lupus Erythematosus. J Rheumatol 2015;42:2296-303. [Crossref] [PubMed]
- Bertsias G, Cervera R, Boumpas DT. Systemic lupus erythematosus: pathogenesis and clinical features. EULAR Textbook on Rheumatic Diseases 2012;5:476-505.
- Catalina MD, Owen KA, Labonte AC, et al. The pathogenesis of systemic lupus erythematosus: Harnessing big data to understand the molecular basis of lupus. J Autoimmun 2020;110:102359. [Crossref] [PubMed]
- Mistry P, Nakabo S, O'Neil L, et al. Transcriptomic, epigenetic, and functional analyses implicate neutrophil diversity in the pathogenesis of systemic lupus erythematosus. Proc Natl Acad Sci U S A 2019;116:25222-8. [Crossref] [PubMed]
- Huang Y, Yang DD, Li XY, et al. ZBP1 is a significant pyroptosis regulator for systemic lupus erythematosus. Ann Transl Med 2021;9:1773. [Crossref] [PubMed]
- Zhan Y, Cheng L, Wu B, et al. Interleukin (IL)-1 family cytokines could differentiate primary immune thrombocytopenia from systemic lupus erythematosus-associated thrombocytopenia. Ann Transl Med 2021;9:222. [Crossref] [PubMed]
- Yap DYH, Chan TM. B Cell Abnormalities in Systemic Lupus Erythematosus and Lupus Nephritis-Role in Pathogenesis and Effect of Immunosuppressive Treatments. Int J Mol Sci 2019;20:6231. [Crossref] [PubMed]
- Pan L, Lu MP, Wang JH, et al. Immunological pathogenesis and treatment of systemic lupus erythematosus. World J Pediatr 2020;16:19-30. [Crossref] [PubMed]
- Shan J, Jin H, Xu Y T. Cell Metabolism: A New Perspective on Th17/Treg Cell Imbalance in Systemic Lupus Erythematosus. Front Immunol 2020;11:1027. [Crossref] [PubMed]
- Kato R, Sumitomo S, Tsuchida Y, et al. CD4(+)CD25(+)LAG3(+) T Cells With a Feature of Th17 Cells Associated With Systemic Lupus Erythematosus Disease Activity. Front Immunol 2019;10:1619. [Crossref] [PubMed]
- Ma K, Du W, Wang X, et al. Multiple Functions of B Cells in the Pathogenesis of Systemic Lupus Erythematosus. Int J Mol Sci 2019;20:6021.
- Ma C, Xia Y, Yang Q, et al. The contribution of macrophages to systemic lupus erythematosus. Clin Immunol 2019;207:1-9. [Crossref] [PubMed]
- O'Neil LJ, Kaplan MJ, Carmona-Rivera C. The Role of Neutrophils and Neutrophil Extracellular Traps in Vascular Damage in Systemic Lupus Erythematosus. J Clin Med 2019;8:1325. [Crossref] [PubMed]
- Bolouri N, Akhtari M, Farhadi E, et al. Role of the innate and adaptive immune responses in the pathogenesis of systemic lupus erythematosus. Inflamm Res 2022;71:537-54. [Crossref] [PubMed]
- Coit P, Jeffries M, Altorok N, et al. Genome-wide DNA methylation study suggests epigenetic accessibility and transcriptional poising of interferon-regulated genes in naïve CD4+ T cells from lupus patients. J Autoimmun 2013;43:78-84. [Crossref] [PubMed]
- Iwamoto T, Dorschner JM, Selvaraj S, et al. High Systemic Type I Interferon Activity Is Associated With Active Class III/IV Lupus Nephritis. J Rheumatol 2022;49:388-97. [Crossref] [PubMed]
- Muskardin TLW, Niewold TB. Type I interferon in rheumatic diseases. Nat Rev Rheumatol 2018;14:214-28. [Crossref] [PubMed]
- Oke V, Gunnarsson I, Dorschner J, et al. High levels of circulating interferons type I, type II and type III associate with distinct clinical features of active systemic lupus erythematosus. Arthritis Res Ther 2019;21:107. [Crossref] [PubMed]
- Postal M, Vivaldo JF, Fernandez-Ruiz R, et al. Type I interferon in the pathogenesis of systemic lupus erythematosus. Curr Opin Immunol 2020;67:87-94. [Crossref] [PubMed]
- Pichlmair A, Lassnig C, Eberle CA, et al. IFIT1 is an antiviral protein that recognizes 5'-triphosphate RNA. Nat Immunol 2011;12:624-30. [Crossref] [PubMed]
- Mähönen K, Hau A, Bondet V, et al. Activation of NLRP3 Inflammasome in the Skin of Patients with Systemic and Cutaneous Lupus Erythematosus. Acta Derm Venereol 2022;102:adv00708.
- Siddiqi KZ, Wilhelm TR, Ulff-Møller CJ, et al. Cluster of highly expressed interferon-stimulated genes associate more with African ancestry than disease activity in patients with systemic lupus erythematosus. A systematic review of cross-sectional studies. Transl Res 2021;238:63-75. [Crossref] [PubMed]
- Ye S, Pang H, Gu YY, et al. Protein interaction for an interferon-inducible systemic lupus associated gene, IFIT1. Rheumatology (Oxford) 2003;42:1155-63. [Crossref] [PubMed]
- Chaussabel D, Quinn C, Shen J, et al. A modular analysis framework for blood genomics studies: application to systemic lupus erythematosus. Immunity 2008;29:150-64. [Crossref] [PubMed]
- Durán-Barragán S, Bátiz-Andrade JP, Valenzuela-Marrufo R, et al. Influence of the environment, gender, and hormones on systemic lupus erythematosus: A narrative review. Revista Colombiana de Reumatología 2021;28:177-90.
- Peng Y, Liu Z, Fu G, et al. Identification microenvironment immune features and key genes in elderly stroke patients. Medicine (Baltimore) 2023;102:e33108. [Crossref] [PubMed]
- Mervat SAN, Shereen MS, Noha HS, et al. Interferon-inducible systemic lupus associated gene, IFIT1 in systemic lupus erythematosus patients. Medical Journal of Cairo University 2008;76:777-83.
- Zheng M, Hu Z, Zhou W, et al. Single-cell transcriptome reveals immunopathological cell composition of skin lesions in subacute cutaneous lupus erythematosus. Clin Immunol 2022;245:109172. [Crossref] [PubMed]
- Panousis NI, Bertsias GK, Ongen H, et al. Combined genetic and transcriptome analysis of patients with SLE: distinct, targetable signatures for susceptibility and severity. Ann Rheum Dis 2019;78:1079-89. [Crossref] [PubMed]
- Banchereau J, Pascual V. Type I interferon in systemic lupus erythematosus and other autoimmune diseases. Immunity 2006;25:383-92. [Crossref] [PubMed]
- Dulken BW, Buckley MT, Navarro Negredo P, et al. Single-cell analysis reveals T cell infiltration in old neurogenic niches. Nature 2019;571:205-10. [Crossref] [PubMed]
- Holderried TAW, de Vos L, Bawden EG, et al. Molecular and immune correlates of TIM-3 (HAVCR2) and galectin 9 (LGALS9) mRNA expression and DNA methylation in melanoma. Clin Epigenetics 2019;11:161. [Crossref] [PubMed]
- Zhang Y, Hua X, Shi H, et al. Systematic analyses of the role of prognostic and immunological EIF3A, a reader protein, in clear cell renal cell carcinoma. Cancer Cell Int 2021;21:680. [Crossref] [PubMed]
- Pan H, Wang X, Huang W, et al. Interferon-Induced Protein 44 Correlated With Immune Infiltration Serves as a Potential Prognostic Indicator in Head and Neck Squamous Cell Carcinoma. Front Oncol 2020;10:557157. [Crossref] [PubMed]
- Letourneur D, Danlos FX, Marabelle A. Chemokine biology on immune checkpoint-targeted therapies. Eur J Cancer 2020;137:260-71. [Crossref] [PubMed]
- Singh S, Long JP, Tchakarov A, et al. Tertiary lymphoid structure signatures are associated with immune checkpoint inhibitor related acute interstitial nephritis. JCI Insight 2022;e165108. [Crossref] [PubMed]
- Weinstein AM, Giraldo NA, Petitprez F, et al. Association of IL-36γ with tertiary lymphoid structures and inflammatory immune infiltrates in human colorectal cancer. Cancer Immunol Immunother 2019;68:109-20. [Crossref] [PubMed]