A nomogram to predict 28-day mortality in neonates with sepsis: a retrospective study based on the MIMIC-III database
Highlight box
Key findings
• Jaundice, weight, serum calcium, base excess, corticosteroids and inotropes were correlated with mortality in neonates with sepsis.
What is known and what is new?
• Sepsis is the second-leading cause of death in neonates, early identification and diagnosis of severe sepsis can improve neonatal outcomes.
• The nomogram will facilitate rapid identification of severe neonatal sepsis and will help clinicians make the right treatment decisions.
What is the implication, and what should change now?
• There is no accurate and concise method to identify neonates with severe sepsis, and it is necessary to develop and validate a tool for early identification of critically ill neonates.
Introduction
Neonatal death pertains to the death of live-born infants within the initial 28 days of life. Prematurity, neonatal sepsis and intrapartum-related complications are the most prevalent causes of neonatal death (1). Sepsis should be defined as life-threatening organ dysfunction resulting from a dysregulated host response to infection (2) and is perceived as the second-leading cause of death in neonates (3). A recent study on the global occurrence of neonatal sepsis indicated the incidence of 2,824 per 100,000 live births with a mortality rate up to 17.6% (4). Milton et al. reported an all-cause mortality of 5.65 per 1,000 neonate-days for those with clinically suspected and laboratory-confirmed neonatal sepsis (5). Despite the declining global case fatality rates of severe sepsis and septic shock in children over the past 30 years, the morbidity of neonatal sepsis is still underestimated due to the lack of a diagnostic gold standard (6). Sepsis carries a significant economic burden in low- and middle-income nations, amounting to over $7 billion in annual healthcare costs in the United States (7,8). Neonatal sepsis generally presents with diverse manifestations and non-specific laboratory results, which can rapidly progress to severe illness. Furthermore, the World Health Organization (WHO) recently accentuated that prevention can decrease sepsis morbidity and mortality (9). As such, the early detection and diagnosis of severe sepsis are imperative.
Currently, there are several prognostic tools available for identifying critical neonatal sepsis (10,11), including the Pediatric Logistic Organ Dysfunction score (PELOD), the Updated PELOD-2 score, Pediatric Risk of Mortality III scales (PRISM-III), Pediatric Multiple Organ Dysfunction score (P-MODS) and neonatal sequential organ failure assessment score (nSOFA). The PELOD score and PELOD-2 score included 12 and 10 factors, respectively, comprising five different organ dysfunctions, whereas the PRISM-III scales evaluated as many as 17 variables (12-14). These tools have limited operability and can be cumbersome, leading to time wastage and thus, are not applicable to newborns. Additionally, PELOD (or PELOD-2) shares commonalities with the P-MODS score and neglect to take into account the unique physiological traits of neonates and perinatal-related factors. Although nSOFA is simple and convenient, it only includes respiration, circulation and blood system score (15). Bestati et al. (16) has demonstrated that the individual correlation of neurological and hepatic dysfunction with neonatal mortality is absent in the evaluation of the nSOFA, resulting in a potential shortcoming. More recently, the machine learning (ML) algorithms have been utilized to forecast neonatal mortality in order to develop more effective tools (17), their restricted interpretability may hinder their usefulness in evaluating the prognosis of neonatal sepsis (18).
An urgent need exists for a straightforward and effective tool to predict neonatal sepsis mortality. Among the statistical visual tools available, nomogram has demonstrated concise and efficient performance when compared to traditional methods. With the evolution of statistical theory, nomograms have increasingly gained recognition and attention. Nomograms have indicated excellent performance in predicting late-onset sepsis in preterm infants with thyroid dysfunction (19) and have been widely used to determine the prognosis of critically ill patients in recent years (20,21). Currently, there is no relevant research that utilizes the nomogram as an instrument to foretell the mortality rate of neonatal sepsis to our understanding.
This study had dual objectives: firstly, to establish a nomogram by extracting neonatal demographic and comprehensive clinical data from the Medical Information Mart for Intensive Care III (MIMIC-III v1.4) database. Secondly, to compare the performance of the established nomogram with the nSOFA in predicting the mortality of neonates with sepsis within a 28-day period. Ultimately, the goal of our study is to establish a robust model to enable early identification of newborns at critically septic stages, which may help doctors to make informed treatment decisions based on a theoretical basis. We present this article in accordance with the TRIPOD reporting checklist (available at https://tp.amegroups.com/article/view/10.21037/tp-23-150/rc).
Methods
Database and study population
This was a retrospective case-control study based on the MIMIC-III database. The MIMIC-III (v1.4) database is an open-access research database comprising information relating to the Intensive Care Unit (ICU) patients from the Beth Israel Deaconess Medical Center (Boston, MA, USA) between 2001 and 2012 (22). To extract data from the MIMIC-III database, we completed the National Institutes of Health’s Protecting Human Research Participants (certification No. 44980177) web-based course.
Identity information and comprehensive clinical data of patients were integrated into the MIMIC-III database, which includes a total of 7,870 neonates. Since neonates were not directly involved, this study did not receive approval from the Institutional Review Committee board of Xinhua Hospital, Shanghai Jiaotong University, School of Medicine, Shanghai, China. The study was conducted in accordance with the Declaration of Helsinki (as revised in 2013). The study was approved by the Massachusetts Institute of Technology (Cambridge, MA, USA) and Beth Israel Deaconess Medical Center (Boston, MA, USA), and individual consent for this retrospective analysis was waived.
Data extraction
Neonates who met the criteria for sepsis in the MIMIC-III database were included in this study. Sepsis was defined according to the Sepsis-3 criteria (2,23). Clinical data for the first clinical features of patients after admission were extracted from the MIMIC-III database using structured query language (SQL), including demographics, vital signs and laboratory tests. Comorbidities were identified using ICD-9 codes, including respiratory distress syndrome (RDS), jaundice, acute kidney injury (AKI) and others. AKI was diagnosed based on the Kidney Disease: Improving Global Outcomes (KDIGO) guidelines as an increase in serum creatinine (Scr) of 0.3 mg/dL or more (24). Data on medications, urine output and intravenous fluid volume within the first 24 h after ICU admission were extracted.
The primary outcome of this study was neonatal death, defined as death occurring within 28 d during hospitalization in the Neonatal Intensive Care Unit (NICU). In addition, the sequential organ failure assessment (SOFA) score of the neonates was extracted as a model. The nSOFA score uses categorical scores [total score ranging from 0 (best) to 15 (worst)] to objectively assess the condition of patients, with increasing scores indicating worse organ dysfunction (15,25), as follows: (I) receipt of mechanical ventilation and oxygen to maintain physiological peripheral saturation, with scores ranging from 0 to 8; (II) inotropic or vasoactive drug support, including the use of corticosteroids for presumed adrenal insufficiency or catecholamine-resistant shock, with scores ranging from 0 to 4; and (III) the presence and severity of thrombocytopenia based on the most recent platelet measure, with scores ranging from 0 to 3.
Missing data handling
Demographic information was complete, but missing data on laboratory tests and physiological items are common in the MIMIC-III database. However, if patients with incomplete data or incomplete variables are eliminated, a large bias would be produced. Therefore, in the study, patients or variables with more than 50% missing values were eliminated. Finally, the missing values in the database were replaced with the method “norm.predict” in Multivariate Imputation by Chained Equation (MICE), in which every variable is imputed conditional on all other variables (26).
Sample size and calculation
To precisely estimate the overall outcome risk or mean outcome value in our study, the required sample size was calculated as follows (27):
The anticipated outcome proportion () in our study was 0.05, and the absolute margin of error (δ) was generally recommended to be less than 0.05. According to Eq. [1], the sample size required for the study was at least 73 participants.
To acquire a small prediction error in the estimated outcome probabilities across all individuals, the required sample size was calculated as follows (27):
The mean absolute prediction error (MAPE) was no larger than 0.050. Moreover, 30 candidate predictor parameters (P) were extracted from the MIMIC-III database. According to Eq. [2], the sample size required for the study was at least 544 participants.
The study included neonates with sepsis who were admitted the NICU between 2001 and 2012, with an estimated sample size of 924.
Statistical analysis
Based on neonatal in-hospital death or survival as the dependent variable, we divided all neonates into two groups. Demographics, vital signs, laboratory tests, medications and comorbidities were defined as independent variables. According to the normality of distribution, continuous variables are presented as the mean ± SD for parametric variables or as the median and IQR for nonparametric variables. Categorical variables are expressed as numbers and percentages. For continuous variables, unpaired Student’s test and Wilcoxon rank sum tests were used to assess significant differences between the two groups. For all categorical variables, the significant difference between the two groups was compared using the χ2-test or Fisher’s exact test.
Figure 1 shows the process of data acquisition, with 924 neonates included in our study. Based on the groupings described previously, we compared significant differences among the independent variables. Moreover, according to the dependent and independent variables described previously, a univariate logit binomial general linear model (GLM) was performed. The independent variables with P<0.05 in univariate analysis were incorporated into the GLM for stepwise regression analysis to further identify risk factors and establish a model (Further information regarding the model can be found in the Appendix 1 Supplement methods). The least absolute shrinkage and selection operator (LASSO) method was also used to screen variables, which can avoid overfitting by imposing a penalty on the magnitude of the model coefficients (28), and another model was established. To avoid multicollinearity among the variables, we employed both Pearson and nonparametric Spearman correlation matrices (29), with specific details presented in Figure S1. Additionally, the variance inflation factor (VIF) value of 5 or higher indicates the presence of multicollinearity (30). We examined potential nonlinear relationships between candidate continuous variables and death using restricted cubic splines and the Box-Tidwell test. Discrimination was assessed by the receiver operating characteristic (ROC) curve and area under the ROC curve (AUC), while calibration curves were plotted to assess model accuracy. Decision curve analysis (DCA) was also conducted to quantify the clinical net benefit at different threshold probabilities (31). Internal validation of the models and nSOFA score was performed using 500 bootstrap resamples and we compared the performance of the three models by calculating sensitivity, specificity, accuracy, integrated discrimination improvement (IDI), and net reclassification improvement (NRI). A nomogram was used to create a visual representation of the optimal model. This visualization tool includes graphic scoring which calculates the probability of a clinical event (32).
The SOFA (Sequential Organ Failure Assessment) score was originally developed to assess the severity of organ dysfunction or failure and was not designed to predict the risk of death (33). In recent years, the SOFA score has been extensively used to evaluate patient mortality (34,35), and the modified SOFA score (Table S1) has also been used to assess the risk of death in newborns (15). The SOFA score was validated in this study and compared with our developed model in terms of discrimination, calibration and the clinical net benefit.
All statistical tests were two-sided, and P values ≤0.05 were considered to be statistically significant. We used R statistical software (V.4.1.1; https://www.R-project.org) and SPSS statistical software (SPSS Statistics 25.0) for statistical analyses.
Results
Baseline characteristics of the included neonates
A total of 1,977 neonates from the MIMIC-III database who met the Sepsis-3 criteria were included. A total of 1,053 neonates were excluded, including 863 patients with more than 50% missing variables and 190 patients with deficient creatinine, calcium and blood urea nitrogen levels. Table S2 provides comprehensive details on how the remaining missing values were handled. The selection procedure of the study population is shown in Figure 1. Ultimately, 924 patients were enrolled in our study, of whom 44 patients died and 880 patients were still alive after 28 days.
The comparisons of demographics and variables for the derivation dataset between patients who died and those who survived during hospitalization are shown in Table 1. The incidence of AKI was higher in the non-surviving group. Patients who died had lower serum bicarbonate, hematocrit, hemoglobin, RDW, calcium, bilirubin, base excess, weight, height, pO2/FiO2_mean and 24-hour urine levels. However, the levels of creatinine, chloride and pCO2 in patients who died within 28 days were significantly increased. Additionally, more patients received systemic corticosteroids and inotropes in the non-surviving group. The nSOFA score of the non-surviving group was higher than that of the surviving group, which was consistent with previous reports. There was no significant difference in other variables between the surviving and non-surviving groups.
Table 1
Characteristic | Overall (N=924) | Outcome | P | |
---|---|---|---|---|
Survival (N=880) | Death (N=44) | |||
Demography | ||||
Gender, male | 513 (55.5) | 486 (55.2) | 27 (61.4) | 0.520 |
Age, hours | 13.60 [8.10, 19.30] | 13.62 [8.00, 19.31] | 12.40 [9.93, 18.60] | 0.968 |
Weight, kg | 1.45 [1.04, 2.10] | 1.46 [1.07, 2.11] | 0.92 [0.69, 1.53] | <0.001 |
Height, cm | 40.33±5.78 | 40.54±5.66 | 36.08±6.41 | <0.001 |
Vital signs | ||||
Heartrate, bmp | 143.00±10.21 | 142.88±10.14 | 145.50±11.21 | 0.096 |
pO2/FiO2mean | 200.00 [154.17, 277.52] | 210.00 [159.09, 284.15] | 131.35 [95.25, 197.60] | <0.001 |
Laboratory tests | ||||
Hematocrit, % | 46.91±7.39 | 47.04±7.26 | 44.32±9.35 | 0.017 |
Hemoglobin, g/dL | 15.60±2.45 | 15.64±2.42 | 14.78±2.92 | 0.023 |
Platelet, K/µL | 249.33±84.03 | 250.36±83.97 | 228.82±83.58 | 0.097 |
RDW, % | 16.90 [16.20, 17.80] | 16.93 [16.22, 17.80] | 16.15 [15.50, 17.12] | 0.004 |
WBC, K/µL | 9.30 [6.40, 13.40] | 9.34 [6.46, 13.33] | 10.20 [5.45, 13.57] | 0.717 |
Neutrophils, K/µL | 30.69±17.30 | 30.74±17.32 | 29.63±16.99 | 0.677 |
Lymphocytes, K/µL | 56.80±18.54 | 56.73±18.50 | 58.14±19.56 | 0.624 |
Anion gap, mmol/L | 16.33±3.63 | 16.28±3.50 | 17.36±5.57 | 0.053 |
Bicarbonate, mmol/L | 20.94±3.16 | 20.99±3.10 | 19.91±4.09 | 0.027 |
Chloride, mmol/L | 106.67±5.05 | 106.58±5.04 | 108.61±4.98 | 0.009 |
Calcium, mg/dL | 9.68±1.39 | 9.76±1.33 | 8.08±1.56 | <0.001 |
Serum potassium, mEq/L | 4.89±1.05 | 4.90±1.05 | 4.86±0.99 | 0.859 |
Serum sodium, mEq/L | 139.02±4.66 | 138.92±4.62 | 141.07±4.91 | 0.003 |
Bilirubin, mg/dL | 4.93±1.95 | 5.03±1.92 | 3.02±1.35 | <0.001 |
Creatinine, mg/dL | 0.69±0.33 | 0.68±0.32 | 0.87±0.34 | <0.001 |
BUN, mg/dL | 17.00 [12.00, 26.00] | 17.70 [12.00, 26.00] | 18.00 [14.50, 24.25] | 0.414 |
pCO2, mmHg | 47.90±11.90 | 47.60±11.35 | 54.00±19.15 | <0.001 |
Base excess, mmol/L | −2.00 [−4.00, 0.00] | −2.10 [−3.98, −0.97] | −4.50 [−10.25, −2.00] | <0.001 |
Comorbidities | ||||
Jaundice | 798 (86.4) | 779 (88.5) | 19 (43.2) | <0.001 |
RDS | 703 (76.1) | 673 (76.5) | 30 (68.2) | 0.281 |
AKI (24 h) | 57 (6.2) | 47 (5.3) | 10 (22.7) | <0.001 |
Others | 381 (41.2) | 364 (41.4) | 17 (38.6) | 0.840 |
Score | ||||
nSOFA | 4.00 [2.00, 6.00] | 4.10 [2.10, 5.10] | 7.00 [5.00, 9.25] | <0.001 |
Treatment | ||||
Liquid intake, mL/d | 131.78±54.67 | 132.39±53.77 | 119.60±70.09 | 0.130 |
Urine out, mL/d | 86.93±47.55 | 89.16±47.13 | 42.18±31.38 | <0.001 |
Corticosteroid | 47 (5.1) | 32 (3.6) | 15 (34.1) | <0.001 |
Inotropes | 123 (13.3) | 95 (10.8) | 28 (63.6) | <0.001 |
Data are presented as the mean ± SD, median [IQR], or numbers and percentages. RDW, red blood cell distribution width; WBC, white blood cell; BUN, blood urea nitrogen; RDS, respiratory distress syndrome; AKI, acute kidney injury; nSOFA, neonatal sequential organ failure assessment.
Selected features
Univariable and stepwise regression analysis was used to assess variables associated with death (Table 2). As shown in Table 2, stepwise regression analysis screened 5 candidate predictors with P values <0.05.
Table 2
Variables | Univariate analysis | Multivariate analysis | |||
---|---|---|---|---|---|
OR (95% CI) | P | OR (95% CI) | P | ||
Demography | |||||
Gender, male | 1.30 (0.69, 2.40) | 0.430 | |||
Age, hours | 1.00 (0.97, 1.01) | 0.760 | |||
Weight, kg | 0.38 (0.22, 0.66) | 0.001 | 0.47 (0.28, 0.81) | 0.006 | |
Height, cm | 0.87 (0.82, 0.92) | 0.000 | |||
Vital signs | |||||
Heartrate, bmp | 1.00 (0.99, 1.10) | 0.096 | |||
pO2/FiO2mean | 0.97 (0.96, 0.99) | 0.000 | 0.98 (0.97, 0.99) | 0.000 | |
Laboratory tests | |||||
Hematocrit, % | 0.96 (0.93, 0.99) | 0.017 | |||
Hemoglobin, g/dL | 0.88 (0.79, 0.98) | 0.023 | |||
Platelet, K/µL | 1.00 (0.99, 1.01) | 0.096 | |||
RDW, % | 0.85 (0.72, 1.00) | 0.066 | |||
WBC, K/µL | 0.98 (0.94, 1.00) | 0.520 | |||
Neutrophils, K/µL | 1.00 (0.98, 1.02) | 0.680 | |||
Lymphocytes, K/µL | 1.00 (0.99, 1.01) | 0.620 | |||
Anion gap, mmol/L | 1.10 (1.00, 1.20) | 0.053 | |||
Bicarbonate, mmol/L | 0.90 (0.82, 0.99) | 0.025 | 0.91 (0.81, 0.98) | 0.027 | |
Chloride, mmol/L | 1.10 (1.00, 1.20) | 0.009 | |||
Calcium, mg/Dl | 0.52 (0.43, 0.62) | 0.000 | |||
Serum potassium, mEq/L | 0.97 (0.73, 1.30) | 0.860 | |||
Serum sodium, mEq/L | 1.10 (1.00, 1.20) | 0.003 | 1.09 (1.01, 1.16) | 0.018 | |
Bilirubin, mg/dL | 0.43 (0.34, 0.55) | 0.000 | |||
Creatinine, mg/dL | 3.70 (1.80, 7.60) | 0.000 | 4.38 (1.91, 10.05) | 0.001 | |
BUN, mg/dL | 1.00 (0.98, 1.01) | 0.600 | |||
pCO2, mmHg | 1.00 (0.91, 1.10) | 0.001 | |||
Base excess, mmol/L | 0.86 (0.82, 0.91) | 0.000 | |||
Comorbidities | |||||
Jaundice | 0.10 (0.05, 0.19) | 0.000 | |||
RDS | 0.66 (0.34, 1.30) | 0.210 | |||
AKI (24 h) | 5.20 (2.40, 11.00) | 0.000 | |||
Others | 0.89 (0.48, 1.70) | 0.720 | |||
Treatment | |||||
Liquid intake, mL/d | 0.99 (0.99, 1.00) | 0.130 | |||
Urine out, mL/d | 0.96 (0.95, 0.97) | 0.000 | |||
Corticosteroid | 14.00 (6.70, 28.00) | 0.000 | |||
Inotropes | 14.00 (7.50, 28.00) | 0.000 |
RDW, red blood cell distribution width; WBC, white blood cell; BUN, blood urea nitrogen; RDS, respiratory distress syndrome; AKI, acute kidney injury; nSOFA, neonatal sequential organ failure assessment.
Figure 2 shows the results of 32 variables that were incorporated into the Lasso regression algorithm from the derivation group. Lasso regression was performed with a λ of 0.021 (one standard error of the minimum λ), and only 12 variables remained in the model. To make the model include fewer variables, lasso regression was performed with a λ of 0.028, and a total of 6 variables entered the model, which may be the most important candidate predictors for developing the model, including corticosteroid use, inotropes, jaundice, weight, serum calcium and base excess, as shown in Table 3.
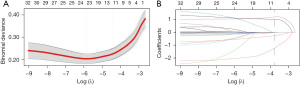
Table 3
Variables | LASSO selected predictors | |
---|---|---|
OR (95% CI) | P | |
Jaundice | 0.05 (0.02, 0.14) | 0.001 |
Corticosteroid | 3.81 (1.35, 10.72) | 0.011 |
Weight, kg | 0.22 (0.11, 0.43) | 0.001 |
Calcium, mg/dL | 0.53 (0.40, 0.69) | 0.001 |
Inotropes | 3.58 (1.50, 8.55) | 0.004 |
Base excess, mmol/L | 0.94 (0.88, 0.99) | 0.048 |
LASSO, the least absolute shrinkage and selection operator.
Model development
For the derivation dataset, candidate variables obtained by lasso regression that had a significant effect on inhospital death are shown in Table 3. In addition, candidate variables with statistically significant differences in stepwise regression analysis were included in the binary logistic regression, as shown in Table 2. Ultimately, the following 6 candidate factors, identified as statistically significant variables using the multivariate likelihood ratio (LR), were included in the Lasso regression algorithm: jaundice (OR 0.05, P=0.001), corticosteroid use (OR 3.81, P=0.011), weight (OR 0.22, P=0.001), serum calcium (OR 0.53, P=0.001), inotropes (OR 3.58, P=0.004) and base excess (OR 0.94, P=0.048). Correspondingly, the binary logistic regression model incorporated a total of 5 variables from the derivation dataset, which included weight (OR 0.47, P=0.006), pO2/FiO2_mean (OR 0.98, P=0.000), bicarbonate (OR 0.91, P=0.027), serum sodium (OR 1.09, P=0.018) and creatinine (OR 4.38, P=0.001). Ultimately, both the lasso and binary logistic regression models exhibited no nonlinearity relationships in the continuous variables, as depicted in Figure 3. A linear correlation was observed between nSOFA score and mortality. The candidate variables did not exhibit multicollinear relationships (Figure S1), and the VIFs are presented in Table 4. We developed two regression models, the lasso regression model and binary logistic regression model, using the candidate variables screened earlier.
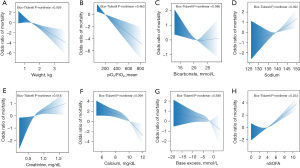
Table 4
Variables | VIF |
---|---|
Multivariate analysis | |
Bicarbonate, mmol/L | 1.011 |
Serum sodium, mEq/L | 1.066 |
Weight, kg | 1.058 |
pO2/FiO2mean | 1.003 |
Creatinine, mg/dL | 1.009 |
LASSO selected predictors | |
Jaundice | 1.332 |
Corticosteroid | 1.138 |
Weight, kg | 1.429 |
Calcium, mmol/L | 1.213 |
Inotropes | 1.208 |
Base excess, mmol/L | 1.195 |
VIFs, variance inflation factors; LASSO, the least absolute shrinkage and selection operator.
Validation and comparison of the models
The discrimination of the two models and the nSOFA score in the derivation dataset was assessed using the ROC curve. In the derivation dataset, the AUC values of three prediction models, namely the stepwise regression model, Lasso algorithm model, and nSOFA score, were determined as 0.784 (95% CI: 0.703–0.866), 0.924 (95% CI: 0.869–0.978), and 0.807 (95% CI: 0.728–0.866), respectively (Table S3). Following 500-times bootstrap resampling, the values changed to 0.763 (95% CI: 0.695–0.874), 0.912 (95% CI: 0.870–0.977), and 0.807 (95% CI: 0.732–0.883) (Figure 4). The discrimination of the LASSO algorithm model was better than that of the stepwise regression model and nSOFA score (P<0.05) (Figure 5). There was no statistically significant difference in the discrimination performance between the stepwise regression model and the nSOFA score (P=0.418) (Figure 5C). In addition, the sensitivity, specificity and accuracy of each model are presented in Table S3. The stepwise regression model and nSOFA score displayed high sensitivity and accuracy, but their specificity was lower. In contrast, the Lasso algorithm model demonstrated better sensitivity, specificity, and accuracy. Moreover, the calibration curve plot of the Lasso algorithm model and nSOFA score model roughly showed an ideal diagonal in the derivation dataset (Figure 4D,4F). Instead, the stepwise regression model exhibited a relatively low consistency among the models (Figure 4B). Decision curve analysis (DCA) was applied to evaluate the clinical net benefit across the entire range of threshold probabilities for each of the three models (Figure 5D). Results indicate that the Lasso algorithm model had the highest clinical and public health value.
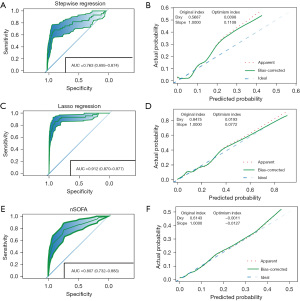
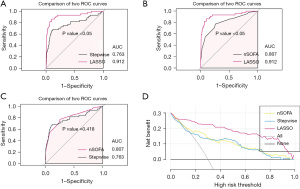
The performance of several models predicting mortality in neonatal populations with sepsis was compared (Table S4). The Lasso algorithm significantly enhances prediction performance compared to that of the stepwise regression and nSOFA score. The net reclassification improvement (NRI) of Lasso algorithm with respect to stepwise regression and nSOFA score are 52.95% and 53.64%, respectively. Moreover, Lasso algorithm improved model performance validated by integrated discrimination improvement (IDI) by 30.93% and 26.98% compared to stepwise regression and nSOFA score, respectively. Overall, the Lasso algorithm model outperforms both the stepwise regression model and nSOFA score in terms of performance.
Development of the best-performing prediction nomogram
The 6 factors had nonzero coefficients in the Lasso regression model based on the derivation groups (Table 3), which were integrated into the nomogram (R2 =0.526, C-index =0.912). A candidate predictor corresponds to a unique score and then sums these scores to obtain a total score, which corresponds to the total score axis and finally to the inhospital mortality axis (Figure 6). Physicians can make the corresponding treatment decisions according to the probability of death that is obtained.
Discussion
Sepsis is a major cause of neonatal death; the mortality of sepsis can be reduced by identifying critically ill patients early (3). Because the clinical signs at the early stages of neonatal sepsis are non-specific, there is currently no uniform standard for early identification of critically ill patients, resulting in delayed management of critically ill patients, which may negatively affect their clinical outcomes. Therefore, based on data derived from the MIMIC-III database, we selected independent risk factors for mortality of neonates with sepsis by using stepwise regression and LASSO regression analysis. Meanwhile, two mortality prediction models with independent predictors for neonates with sepsis were developed. The optimal model was selected by comparing the two prediction models with the nSOFA score model. Finally, a neonatal sepsis mortality prediction nomogram was developed based on the optimal model. The nomogram may facilitate the early identification of neonates with severe sepsis and may reduce mortality of critically ill patients.
In 2015, neonatal deaths accounted for approximately 45.1% of children who did not live to 5 years of age, with one of the leading causes being sepsis or meningitis (1). A study showed mortality ranging from 11% in the USA to 19% in India (3). Another recent study showed that the all-cause mortality of neonatal sepsis was 0.83 (0.37–2.00) per 1,000 neonate-days in low-income and middle-income countries (5). The mortality in our study was 5%, which was lower than that previously described. These differences in mortality may be attributed to better medical conditions in the United States and relatively advanced neonatal care.
Despite advances in neonatal intensive care technology, nonspecific clinical symptoms often lead to rapidly progress to severe illness. Therefore, we developed a mortality prediction model to provide a simpler tool for physicians to identify neonates with severe sepsis early that may help reduce the mortality of infection-related illnesses. Our results indicated that weight, pO2/FiO2, serum calcium, base excess and bicarbonate had protective effects on neonatal in-hospital death, while systemic corticosteroids, inotropes, and high serum creatinine levels were risk factors within the first 24 h after NICU admission. Previous studies have demonstrated that weight, pO2/FiO2, serum calcium, base excess and bicarbonate are protective factors associated with neonatal death (15,36-38). Firstly, the pO2/FiO2 is an available noninvasive marker for the identification of children with acute lung injury or ARDS (39). Secondly, serum calcium is crucial for various physiological processes, including signal transduction in cells, nerve transmission, coagulation cascade and muscle contraction (40). Neonatal sepsis frequently causes hypocalcemia due to the presence of inflammatory markers, including procalcitonin (PCT), tumor-necrosis factor α (TNF-α), interleukin-1β (IL-1β) (41). Further research demonstrates that Neonatal sepsis accompanied by hypocalcemia increases the risk for developing cardiovascular and renal dysfunction, as well as disseminated intravascular coagulation and epilepsy, ultimately resulting in higher mortality rates (42). Additionally, animal study supports the involvement of calcium ions in lethal coagulation during sepsis (43). Thirdly, hemodynamic instability during neonatal sepsis results in tissue hypoperfusion, causing metabolic acidosis and consequently, reduced levels of serum bicarbonate and BE. Bicarbonate effectively improves tissue perfusion, myocardial contractility, and cellular dysfunction in severe acidosis (44,45). Reduced levels of bicarbonate are known to correlate with decreased levels of BE. Our study did not utilize lactate to assess tissue hypoperfusion, as excessive lactate deficiency can lead to unreliable results. A study reveals that increase of negative value of BE values can better reflect hypoperfusion-induced metabolic acidosis in critically ill patients compared to lactate (46). These findings also offset certain limitations in our study. Moreover, low serum levels of both bicarbonate and BE are indicative of a higher mortality in critically ill patients (46-49). These studies support our conclusions while further enhancing our understanding of the association between these indicators and neonatal mortality.
The literature has presented an elevation of plasma bilirubin levels indicates strong liver conversion function, while lower concentration of cholestasis-related markers implies potential protective effect of bilirubin (50). Previous study has indicated that bilirubin accumulation may be beneficial for the survival of newborns and septic neonates who survived had higher plasma bilirubin levels (51). Stocker et al. believed that Bilirubin is a strong antioxidant whose ability to suppress the oxidation in vitro is more prominent than that of another powerful antioxidant-α-tocophero (52). Also, bilirubin can protect low-density lipoprotein from damage caused by oxidation (53). Meanwhile, for newborns suffering from sepsis, inhibiting GBS growth is possible by affecting substrate utilization when the plasma bilirubin concentration is below the diagnostic criteria for neonatal hyperbilirubinemia (54). These results demonstrated a protective effect of jaundice on neonatal sepsis. However, the mechanism by which bilirubin provides such protection against neonatal sepsis remains unclear and requires additional research.
Our study found that systematic corticosteroids and inotropes were associated with adverse outcomes in neonates, supported by the nSOFA score (15). The efficacy of corticosteroids can improve mortality in patients with sepsis remains controversial, however, the administration of corticosteroids was associated with more superinfection, hyperglycemia and hypernatremia (55). Additionally, corticosteroids are primarily prescribed to children with sepsis who suffer from fluid refractory and catecholamine resistant shock (56). Simultaneously, refractory septic shock in children was associated with high vaso-inotrope doses, indicating that neonates had more critical outcomes (57). The inotropes represented circulatory instability and it was a major cause of arrhythmia and leaded to some life-threatening complications such as central nervous bleeding and intestinal ischemia (58). Furthermore, high serum creatinine levels indicated worsening acute kidney injury associated with poor outcomes (59,60), which confirmed our conclusion.
Among the available sickness prediction scoring systems for assessing the severity of neonates, the Clinical Risk Index for Babies (CRIB) and CRIB-II scores primarily evaluate several parameters during the perinatal period and are applicable to newborns with gestational age <33 weeks (61). In addition, The Transport Risk Index of Physiologic Stability (TRIPS) and TRIPS-II scores evaluate temperature, respiratory status, blood pressure, response to noxious stimuli in infants (62,63). TRIPS and TRIPS-II scores are applied to rapidly assess the infants requiring transport to a NICU, and cannot accurately assess the severity of neonatal sepsis. The Score for Neonatal Acute Physiology (SNAP) consists of 27 items of vital signs, blood gas indicators, peripheral blood cells, and biochemistry indicators (64). The SNAP Perinatal Extension (SNAPPE), derived from SNAP, includes three supplementary indicators, that is, birth weight, small for gestational age (SGA), and Apgar score at 5 min. However, their applicability is limited by the complexity of the calculations and the multiple variables involved. The simplified SNAP-II score includes mean blood pressure, lowest body temperature, P(O2)/FiO2, pH, urine output, and multiple seizures, but its performance in evaluating the severity of neonatal diseases is not ideal (65). The SNAPPE-II score adds birth weight, SGA, and Apgar score at 5 min to the SNAP-II score. Previous studies compared the CRIB-II and SNAPPE-II for predicting mortality, and neither of them fully estimated the risk of death (66). Obviously, an accurate scoring system with fewer variables is more convenient for clinical application than relying on multiple variables. In our study, the nomogram incorporates only 6 easily ascertainable variables, while considering the impact of organ function and treatment measures on the progression of sepsis. These are factors that CRIB-II, SNAP-II, and SNAPPE-II scores, commonly used to assess the severity of neonates, have not considered.
The optimal SOFA cutoff to discriminate mortality was a score higher than 8 points, and the performance of the maximum SOFA score in discriminating in-hospital mortality was similar to the performance of the PRISM and PELOD-2 and better than the P-MODS score (10). The PRISM, PELOD-2, and P-MODS have been validated in the prognosis of sepsis in children (67) but have not been applied to neonates. Moreover, a SOFA score of 2 or higher has limited sensitivity in predicting in-hospital mortality risk (11). However, our findings indicate that nSOFA has relatively high sensitivity but low specificity. We noticed several relevant parallels of our study with studies on SOFA performance (Figure 3). As the SOFA score increased, the risk of death increased (10,11,15), and the SOFA performance (AUC, 0.81; 95% CI: 0.73–0.88) was similar to the conclusion of a previous study (AUC, 0.81; 95% CI: 0.76–0.85) (15), which proved that the performance of the nomogram may be better than that of the SOFA score.
As prognostic devices, nomograms have been widely used to predict the probability of clinical events. We developed a nomogram for predicting the risk of in-hospital mortality in neonates, which can enable physicians to quickly identify severe sepsis in neonates and make rapid clinical decisions. Moreover, the nomogram showed good predictive accuracy in predicting mortality in neonates due to severe sepsis, with an AUC of 0.912. However, there are some inevitable limitations in our study that need to be addressed. First, the study was a single-center retrospective study based on the MIMIC-III database, which made it impossible for external validation. Second, the model’s statistical power was affected by a small sample size, as there were fewer neonates in the non-surviving group. Although we utilized a recent technique for calculating sample size, the conventional method that meets Peduzzi’s criterion of an event per variable (EPV) of >10 shows that we cannot achieve adequate statistical power. In addition, the etiology of individual patient-level information were not available. This may limit our more accurate conclusion. Moreover, due to the late availability of etiological information, its role in accurate medication within 24 h of admission was relatively limited. Nonetheless, our findings provide novel insights into the prediction of mortality in neonates with sepsis, which has potentially important public health implications for improving survival of neonates with sepsis. Our study’s limitations should be acknowledged, that need to be addressed in future studies.
Conclusions
Jaundice, weight, serum calcium and base excess were associated with a reduced risk of mortality in neonates with sepsis, while corticosteroids and inotropes were related to an increased risk of mortality in neonates with sepsis. We developed a mortality prediction nomogram with a small number of routinely collected variables for neonates with sepsis, which can be applied to identify critically ill neonates early. The nomogram can be easily used in the NICU.
Acknowledgments
We thank all participants in the Xinhua Hospital, School of Medicine, Shanghai Jiaotong University, Shanghai, China. The authors gratefully acknowledge the editors and reviewers of Transnational Pediatrics for their invaluable feedback, particularly their insightful comments on statistical issues that improved the manuscript. Additionally, we appreciate their support in editing our manuscript.
Funding: This work was supported in part by grants from
Footnote
Reporting Checklist: The authors have completed the TRIPOD reporting checklist. Available at https://tp.amegroups.com/article/view/10.21037/tp-23-150/rc
Peer Review File: Available at https://tp.amegroups.com/article/view/10.21037/tp-23-150/prf
Conflicts of Interest: All authors have completed the ICMJE uniform disclosure form (available at https://tp.amegroups.com/article/view/10.21037/tp-23-150/coif). All authors report that this work was supported in part by grants from the National Natural Science Foundation of China (NSFC Project No. 82171948), and the Shanghai Municipal Health Commission Scientific Research Project (Project No. 20214Y0126). The sponsors of this study had no role in study design, data collection, data analysis, data interpretation, or writing of the report. The authors have no other conflicts of interest to declare.
Ethical Statement: The authors are accountable for all aspects of the work in ensuring that questions related to the accuracy or integrity of any part of the work are appropriately investigated and resolved. The study was conducted in accordance with the Declaration of Helsinki (as revised in 2013). The study was approved by the Massachusetts Institute of Technology (Cambridge, MA) and Beth Israel Deaconess Medical Center (Boston, MA), and individual consent for this retrospective analysis was waived.
Open Access Statement: This is an Open Access article distributed in accordance with the Creative Commons Attribution-NonCommercial-NoDerivs 4.0 International License (CC BY-NC-ND 4.0), which permits the non-commercial replication and distribution of the article with the strict proviso that no changes or edits are made and the original work is properly cited (including links to both the formal publication through the relevant DOI and the license). See: https://creativecommons.org/licenses/by-nc-nd/4.0/.
References
- Liu L, Oza S, Hogan D, et al. Global, regional, and national causes of under-5 mortality in 2000-15: an updated systematic analysis with implications for the Sustainable Development Goals. Lancet 2016;388:3027-35. [Crossref] [PubMed]
- Singer M, Deutschman CS, Seymour CW, et al. The Third International Consensus Definitions for Sepsis and Septic Shock (Sepsis-3). JAMA 2016;315:801-10. [Crossref] [PubMed]
- Fleischmann-Struzek C, Goldfarb DM, Schlattmann P, et al. The global burden of paediatric and neonatal sepsis: a systematic review. Lancet Respir Med 2018;6:223-30. [Crossref] [PubMed]
- Fleischmann C, Reichert F, Cassini A, et al. Global incidence and mortality of neonatal sepsis: a systematic review and meta-analysis. Arch Dis Child 2021; Epub ahead of print. [Crossref] [PubMed]
- Milton R, Gillespie D, Dyer C, et al. Neonatal sepsis and mortality in low-income and middle-income countries from a facility-based birth cohort: an international multisite prospective observational study. Lancet Glob Health 2022;10:e661-72. [Crossref] [PubMed]
- Tan B, Wong JJ, Sultana R, et al. Global Case-Fatality Rates in Pediatric Severe Sepsis and Septic Shock: A Systematic Review and Meta-analysis. JAMA Pediatr 2019;173:352-62. [Crossref] [PubMed]
- Ranjeva SL, Warf BC, Schiff SJ. Economic burden of neonatal sepsis in sub-Saharan Africa. BMJ Glob Health 2018;3:e000347. [Crossref] [PubMed]
- Carlton EF, Barbaro RP, Iwashyna TJ, et al. Cost of Pediatric Severe Sepsis Hospitalizations. JAMA Pediatr 2019;173:986-7. [Crossref] [PubMed]
- Board WHOE. EB140/12: Improving the prevention, diagnosis and clinical management of sepsis Published 2017. Available online: http://apps.who.int/gb/ebwha/pdf_files/EB140/B140_12-en.pdf.
- Matics TJ, Sanchez-Pinto LN. Adaptation and Validation of a Pediatric Sequential Organ Failure Assessment Score and Evaluation of the Sepsis-3 Definitions in Critically Ill Children. JAMA Pediatr 2017;171:e172352. [Crossref] [PubMed]
- Balamuth F, Scott HF, Weiss SL, et al. Validation of the Pediatric Sequential Organ Failure Assessment Score and Evaluation of Third International Consensus Definitions for Sepsis and Septic Shock Definitions in the Pediatric Emergency Department. JAMA Pediatr 2022;176:672-8. [Crossref] [PubMed]
- Leteurtre S, Martinot A, Duhamel A, et al. Validation of the paediatric logistic organ dysfunction (PELOD) score: prospective, observational, multicentre study. Lancet 2003;362:192-7. [Crossref] [PubMed]
- Leteurtre S, Duhamel A, Salleron J, et al. PELOD-2: an update of the PEdiatric logistic organ dysfunction score. Crit Care Med 2013;41:1761-73. [Crossref] [PubMed]
- Graciano AL, Balko JA, Rahn DS, et al. The Pediatric Multiple Organ Dysfunction Score (P-MODS): development and validation of an objective scale to measure the severity of multiple organ dysfunction in critically ill children. Crit Care Med 2005;33:1484-91. [Crossref] [PubMed]
- Fleiss N, Coggins SA, Lewis AN, et al. Evaluation of the Neonatal Sequential Organ Failure Assessment and Mortality Risk in Preterm Infants With Late-Onset Infection. JAMA Netw Open 2021;4:e2036518. [Crossref] [PubMed]
- Bestati N, Leteurtre S, Duhamel A, et al. Differences in organ dysfunctions between neonates and older children: a prospective, observational, multicenter study. Crit Care 2010;14:R202. [Crossref] [PubMed]
- Hsu JF, Chang YF, Cheng HJ, et al. Machine Learning Approaches to Predict In-Hospital Mortality among Neonates with Clinically Suspected Sepsis in the Neonatal Intensive Care Unit. J Pers Med 2021;11:695. [Crossref] [PubMed]
- Yoon CH, Torrance R, Scheinerman N. Machine learning in medicine: should the pursuit of enhanced interpretability be abandoned? J Med Ethics 2022;48:581-5. [Crossref] [PubMed]
- Huang Y, Yu X, Li W, et al. Development and validation of a nomogram for predicting late-onset sepsis in preterm infants on the basis of thyroid function and other risk factors: Mixed retrospective and prospective cohort study. J Adv Res 2020;24:43-51. [Crossref] [PubMed]
- Lu Z, Zhang J, Hong J, et al. Development of a Nomogram to Predict 28-Day Mortality of Patients With Sepsis-Induced Coagulopathy: An Analysis of the MIMIC-III Database. Front Med (Lausanne) 2021;8:661710. [Crossref] [PubMed]
- Hou N, Li M, He L, et al. Predicting 30-days mortality for MIMIC-III patients with sepsis-3: a machine learning approach using XGboost. J Transl Med 2020;18:462. [Crossref] [PubMed]
- Johnson AE, Pollard TJ, Shen L, et al. MIMIC-III, a freely accessible critical care database. Sci Data 2016;3:160035. [Crossref] [PubMed]
- Weiss SL, Peters MJ, Alhazzani W, et al. Surviving sepsis campaign international guidelines for the management of septic shock and sepsis-associated organ dysfunction in children. Intensive Care Med 2020;46:10-67. [Crossref] [PubMed]
- Kellum JA, Lameire N. Diagnosis, evaluation, and management of acute kidney injury: a KDIGO summary (Part 1). Crit Care 2013;17:204. [Crossref] [PubMed]
- Lambden S, Laterre PF, Levy MM, et al. The SOFA score-development, utility and challenges of accurate assessment in clinical trials. Crit Care 2019;23:374. [Crossref] [PubMed]
- van Buuren S, Groothuis-Oudshoorn K. mice: Multivariate Imputation by Chained Equations in R. J Stat Softw 2011;45:1-67. [Crossref]
- Riley RD, Ensor J, Snell KIE, et al. Calculating the sample size required for developing a clinical prediction model. BMJ 2020;368:m441. [Crossref] [PubMed]
- Sauerbrei W, Royston P, Binder H. Selection of important variables and determination of functional form for continuous predictors in multivariable model building. Stat Med 2007;26:5512-28. [Crossref] [PubMed]
- Fabio A, Li W, Strotmeyer S, et al. Racial segregation and county level intentional injury in Pennsylvania: analysis of hospital discharge data for 1997-1999. J Epidemiol Community Health 2004;58:346-51. [Crossref] [PubMed]
- Shen Y, Huang X, Zhang W. Platelet-to-lymphocyte ratio as a prognostic predictor of mortality for sepsis: interaction effect with disease severity-a retrospective study. BMJ Open 2019;9:e022896. [Crossref] [PubMed]
- Kerr KF, Brown MD, Zhu K, et al. Assessing the Clinical Impact of Risk Prediction Models With Decision Curves: Guidance for Correct Interpretation and Appropriate Use. J Clin Oncol 2016;34:2534-40. [Crossref] [PubMed]
- Iasonos A, Schrag D, Raj GV, et al. How to build and interpret a nomogram for cancer prognosis. J Clin Oncol 2008;26:1364-70. [Crossref] [PubMed]
- Vincent JL, de Mendonça A, Cantraine F, et al. Use of the SOFA score to assess the incidence of organ dysfunction/failure in intensive care units: results of a multicenter, prospective study. Working group on "sepsis-related problems" of the European Society of Intensive Care Medicine. Crit Care Med 1998;26:1793-800. [Crossref] [PubMed]
- Raith EP, Udy AA, Bailey M, et al. Prognostic Accuracy of the SOFA Score, SIRS Criteria, and qSOFA Score for In-Hospital Mortality Among Adults With Suspected Infection Admitted to the Intensive Care Unit. JAMA 2017;317:290-300. [Crossref] [PubMed]
- Elias A, Agbarieh R, Saliba W, et al. SOFA score and short-term mortality in acute decompensated heart failure. Sci Rep 2020;10:20802. [Crossref] [PubMed]
- Park HW, Park SY, Kim EA. Prediction of In-Hospital Mortality After 24 Hours in Very Low Birth Weight Infants. Pediatrics 2021;147:e2020004812. [Crossref] [PubMed]
- Zhang K, Zhang S, Cui W, et al. Development and Validation of a Sepsis Mortality Risk Score for Sepsis-3 Patients in Intensive Care Unit. Front Med (Lausanne) 2020;7:609769. [Crossref] [PubMed]
- Knutzen L, Svirko E, Impey L. The significance of base deficit in acidemic term neonates. Am J Obstet Gynecol 2015;213:373.e1-7. [Crossref] [PubMed]
- Khemani RG, Patel NR, Bart RD 3rd, et al. Comparison of the pulse oximetric saturation/fraction of inspired oxygen ratio and the PaO2/fraction of inspired oxygen ratio in children. Chest 2009;135:662-8. [Crossref] [PubMed]
- Jia X, Chen X, Gao C, et al. Functional cooperation between IK(Ca) and TRPC1 channels regulates serum-induced vascular smooth muscle cell proliferation via mediating Ca(2+) influx and ERK1/2 activation. Cell Prolif 2023;56:e13385. [Crossref] [PubMed]
- Kutílek Š, Vracovská M, Pečenková K, et al. Calcemia and Inflammatory Markers in Early-Onset Neonatal Infection. Acta Medica (Hradec Kralove) 2019;62:58-61. [Crossref] [PubMed]
- Liu Y, Chai Y, Rong Z, et al. Prognostic Value of Ionized Calcium Levels in Neonatal Sepsis. Ann Nutr Metab 2020;76:193-200. [Crossref] [PubMed]
- Zhang H, Zeng L, Xie M, et al. TMEM173 Drives Lethal Coagulation in Sepsis. Cell Host Microbe 2020;27:556-570.e6. [Crossref] [PubMed]
- Kraut JA, Madias NE. Lactic acidosis. N Engl J Med 2014;371:2309-19. [Crossref] [PubMed]
- Berend K, de Vries AP, Gans RO. Physiological approach to assessment of acid-base disturbances. N Engl J Med 2014;371:1434-45. [Crossref] [PubMed]
- Yuan J, Liu X, Liu Y, et al. Association between base excess and 28-day mortality in sepsis patients: A secondary analysis based on the MIMIC- IV database. Heliyon 2023;9:e15990. [Crossref] [PubMed]
- Couto-Alves A, Wright VJ, Perumal K, et al. A new scoring system derived from base excess and platelet count at presentation predicts mortality in paediatric meningococcal sepsis. Crit Care 2013;17:R68. [Crossref] [PubMed]
- Tan L, Xu Q, Li C, et al. Association Between the Admission Serum Bicarbonate and Short-Term and Long-Term Mortality in Acute Aortic Dissection Patients Admitted to the Intensive Care Unit. Int J Gen Med 2021;14:4183-95. [Crossref] [PubMed]
- Allen CJ, Wagenaar AE, Horkan DB, et al. Predictors of mortality in pediatric trauma: experiences of a level 1 trauma center and an assessment of the International Classification Injury Severity Score (ICISS). Pediatr Surg Int 2016;32:657-63. [Crossref] [PubMed]
- Jenniskens M, Langouche L, Vanwijngaerden YM, et al. Cholestatic liver (dys)function during sepsis and other critical illnesses. Intensive Care Med 2016;42:16-27. [Crossref] [PubMed]
- Khalil S, Shah D, Faridi MM, et al. Prevalence and outcome of hepatobiliary dysfunction in neonatal septicaemia. J Pediatr Gastroenterol Nutr 2012;54:218-22. [Crossref] [PubMed]
- Stocker R, Yamamoto Y, McDonagh AF, et al. Bilirubin is an antioxidant of possible physiological importance. Science 1987;235:1043-6. [Crossref] [PubMed]
- Tomaro ML, Batlle AM. Bilirubin: its role in cytoprotection against oxidative stress. Int J Biochem Cell Biol 2002;34:216-20. [Crossref] [PubMed]
- Hansen R, Gibson S, De Paiva Alves E, et al. Adaptive response of neonatal sepsis-derived Group B Streptococcus to bilirubin. Sci Rep 2018;8:6470. [Crossref] [PubMed]
- Chan ED, Chan MM, Chan MM, et al. Use of glucocorticoids in the critical care setting: Science and clinical evidence. Pharmacol Ther 2020;206:107428. [Crossref] [PubMed]
- Rhodes A, Evans LE, Alhazzani W, et al. Surviving Sepsis Campaign: International Guidelines for Management of Sepsis and Septic Shock: 2016. Intensive Care Med 2017;43:304-77. [Crossref] [PubMed]
- Morin L, Ray S, Wilson C, et al. Refractory septic shock in children: a European Society of Paediatric and Neonatal Intensive Care definition. Intensive Care Med 2016;42:1948-57. [Crossref] [PubMed]
- Annane D, Ouanes-Besbes L, de Backer D, et al. A global perspective on vasoactive agents in shock. Intensive Care Med 2018;44:833-46. [Crossref] [PubMed]
- Choi HJ, Kim I, Lee HJ, et al. Clinical characteristics of neonatal cholestasis in a tertiary hospital and the development of a novel prediction model for mortality. EBioMedicine 2022;77:103890. [Crossref] [PubMed]
- Shalaby MA, Sawan ZA, Nawawi E, et al. Incidence, risk factors, and outcome of neonatal acute kidney injury: a prospective cohort study. Pediatr Nephrol 2018;33:1617-24. [Crossref] [PubMed]
- Vardhelli V, Murki S, Tandur B, et al. Comparison of CRIB-II with SNAPPE-II for predicting survival and morbidities before hospital discharge in neonates with gestation ≤ 32 weeks: a prospective multicentric observational study. Eur J Pediatr 2022;181:2831-8. [Crossref] [PubMed]
- Lee SK, Zupancic JA, Pendray M, et al. Transport risk index of physiologic stability: a practical system for assessing infant transport care. J Pediatr 2001;139:220-6. [Crossref] [PubMed]
- Lee SK, Aziz K, Dunn M, et al. Transport Risk Index of Physiologic Stability, version II (TRIPS-II): a simple and practical neonatal illness severity score. Am J Perinatol 2013;30:395-400. [PubMed]
- Richardson DK, Gray JE, McCormick MC, et al. Score for Neonatal Acute Physiology: a physiologic severity index for neonatal intensive care. Pediatrics 1993;91:617-23. [Crossref] [PubMed]
- McLeod JS, Menon A, Matusko N, et al. Comparing mortality risk models in VLBW and preterm infants: systematic review and meta-analysis. J Perinatol 2020;40:695-703. [Crossref] [PubMed]
- Gagliardi L, Cavazza A, Brunelli A, et al. Assessing mortality risk in very low birthweight infants: a comparison of CRIB, CRIB-II, and SNAPPE-II. Arch Dis Child Fetal Neonatal Ed 2004;89:F419-22. [Crossref] [PubMed]
- Zhang L, Wu Y, Huang H, et al. Performance of PRISM III, PELOD-2, and P-MODS Scores in Two Pediatric Intensive Care Units in China. Front Pediatr 2021;9:626165. [Crossref] [PubMed]