The use of probabilistic graphical models in pediatric sepsis: a feasibility and scoping review
Highlight box
Key findings
• Performance of probabilistic graphical model (PGM) is relatively inferior to other machine learning (ML) methods on the same dataset.
• Considering their qualities in explainability and transparency, PGM can be useful in pediatric sepsis applications.
What is known and what is new?
• ML demonstrates potential to in pediatric sepsis applications, with certain advantages.
• PGM provides advantages of interactive representation, transparent reasoning, and missing data handling. There is, however, a lack of in-depth discussion of these aspects in comparison to other ML methods in the current literature.
What is the implication, and what should change now?
• PGM is a potential candidate for pediatric sepsis applications, demonstrating efficacy, robustness, explainability, reliability, and trustworthiness.
• More granular data should be collected to facilitate the extraction and application of multiple definitions of sepsis to enhance the predictive ability of ML models.
Introduction
It is estimated that more than 50 million cases of sepsis are detected each year, and more than 40% of these cases occur in children under the age of five (1,2). Incidence of sepsis in newborns ranged from 1 to 22 per 1,000 births, with a mortality rate of 5–16% worldwide (1,3,4). Approximately 77% of infants with sepsis require intensive care, while 16% of term infants with sepsis die from this condition (4). Sepsis continues to be the primary cause of severe morbidity and mortality for children in pediatric intensive care units (PICUs), with several challenges to address (2,5,6). One of the most pressing challenges is the absence of an objective, robust and universal sepsis definition, while the existence of disparate variations in clinical presentation and variables (based on clinical, biochemistry and microbiology findings) complicates the diagnosis process (2,7). Moreover, adult-based sepsis definitions often do not apply to children, since physiological and laboratory parameter cutoffs need to be age-appropriate (8). The diagnostic challenge is further compounded by the fact that results of microbiological cultures are often unavailable at the time of sepsis evaluation (9).
In recent years, researchers have utilized machine learning (ML) and electronic health records (EHRs) in an attempt to address these challenges. ML is an interdisciplinary field combining knowledge from mathematics, statistics, and data analytics. It offers a wide range of established methods, including supervised learning (e.g., regression, classification) and unsupervised learning (e.g., clustering). Both supervised and unsupervised learning can be used to search for predictive patterns in health data to distinguish between patients with and without diseases. Using these methods, researchers have developed several models to diagnose sepsis at the early stages with high accuracy, often without the need for microbiology results (10-13). As a result, they have the potential to contribute towards patient care, reducing patient hospital stay and their medical costs (14-16). Amongst the ML methods, probabilistic graphical models (PGMs), a set of methodologies that uses graphs and probability theory, is one of the most robust approaches available (17,18). PGM constructs a complex network of knowledge and incorporates different information for inference and prediction. Informative graphical representation, intuitive uncertainty handling with conditional probability distributions and joint probability factors, and transparent explanation capabilities make PGM an attractive option in the field of medicine (19). Gupta et al. (20) developed a risk prediction model for coronary artery disease (CAD) using PGM with an area under the curve of 0.93±0.06 and demonstrated the possibility of personalized CAD diagnosis and therapy selection. The authors also emphasized the strength of PGM and its efficacy in handling data with uncertainty or missing information. Other investigators have exhibited that PGM could capture the underlying distribution of the dataset and generated the synthetic data effectively with transparency (21). Even though their applications in pediatric sepsis are currently limited, past studies suggest that PGM has the potential to improve several aspects of pediatric sepsis applications (19-21).
In this scoping review, we aim to (I) evaluate the feasibility of PGM in pediatric sepsis application and (II) describe how pediatric sepsis definitions are used in the ML literature. The result of this review will allow us to evaluate potential research opportunities to use PGM for various applications in pediatric sepsis as well as provide our perspective on the use of pediatric sepsis definition for these studies. We present this article in accordance with the PRISMA-ScR reporting checklist (available at https://tp.amegroups.com/article/view/10.21037/tp-23-25/rc) (22).
Methods
The following medical databases (January 2000 to May 2023) were used for this review: PubMed, Scopus, Cumulative Index to Nursing and Allied Health Literature (CINAHL+), and Web of Science.
The keywords used for the searches included “sepsis”, “neonates”, “infants”, “pediatric”, “machine learning” and “probabilistic graphical model”. Similar keywords were found by using the Thesaurus dictionary, and the keyword list was refined through several iterative rounds of preliminary searches. At each round, the abstracts and titles of the top search were screened to extract more keywords. The process was repeated until there was no new word to add, and the search results were sufficiently comprehensible. The same processes were applied to the Mesh terms in the PubMed database and subheadings in CINAHL+ and Web of Science. In Scopus, searches were conducted using only keywords. In PubMed, CINAHL+, and Web of Science, searches were conducted using keywords, Mesh terms, and subheadings.
The search results were combined and imported into Covidence (Australia), a licensed literature review web application for screening and review (23). The screening included title/abstract, full-text screening and was completed by two authors (T.M.N., S.W.L. or Y.C.K.H.). They screened the publications independently, and conflicts were resolved by discussion or by involving a third author. Table 1 lists our inclusion criteria. Data charting was performed using a predetermined Microsoft Excel (United States) template and iteratively reviewed. Extracted data included: title, authors, publication year, objectives, patient characteristics, study design, data source, sepsis definition, sepsis incidence rate, ML variables, ML methodology, performance metrics, and results.
Table 1
Inclusion criteria |
(I) Year: 2000–2022 |
(II) Journal articles |
(III) Study contains the predefined keywords |
(IV) Study in English |
(V) Pediatric study |
(VI) Study conducted on humans |
(VII) Real data use |
There are a number of metrics that can be used to measure the performance of ML models, depending on their characteristics. In supervised learning for classification task, sensitivity (SEN), specificity (SPE), accuracy (ACC), area under the receiver-operating curve (AUROC), positive predictive value (PPV), and negative predictive value (NPV) are often popular choices. These measurements evaluate how reliable the model is by calculating the proportion of correctly and incorrectly predicted cases on the given dataset. Their equations are derived from the confusion matrix, which comprises the true positive (TP), true negative (TN), false positive (FP), and false negative (FN). AUROC is calculated by measuring the true positive rate against the false negative rate. An AUROC nearer to 1.0 represents a higher capability to distinguish between positive and negative cases. The choice of appropriate metrics depends on the nature of the problem and the dataset. In the event of an imbalanced dataset, the use of ACC, AUROC, or any single metric alone is not recommended because it does not accurately reflect the model’s predictive ability. A combination of them with additional F-score, G-mean, area under precision-recall curve (AUPRC), and various other metrics that provide different views on the predicted positives and negatives should be used instead. The most commonly used evaluation metrics for regression tasks in supervised learning are R squares or adjusted R squares, mean square errors (MSE) or root mean square errors (RMSE), and mean absolute errors (MAE). These metrics measure the fit between prediction values and ground truth values. As for unsupervised learning, performance evaluation is less straightforward as it often requires the evaluation of both the results and the unsupervised algorithms employed. Essentially, it seeks to determine whether the number of clusters discovered is optimal and reliable, as well as validate whether members within a cluster and between clusters are similar. Common metrics include the Davies-Bouldin Index, Calinski-Harabasz Index, and Silhouette Coefficient (24).
The capabilities of ML extend far beyond those of conventional statistical methods. They are, however, difficult to interpret because of their mathematical complexity. A common question that arises when using such a model is why a particular result is reached. One way to approach this question is by examining the features involved in the learning process and the extent to which they contribute to the final result, as seen in some explainable artificial intelligent (XAI) tools. In particular, Shapley additive explanations (SHAP) and local interpretable model-agnostic explanations (LIME) have been gaining popularity due to their model-agnostic nature and user-friendly interfaces that work on several ML models. Other approaches of XAI can be referred to (25).
In this review, we present the performance comparison in two approaches: (I) an overall qualitative comparison between PGM and other ML methods, and (II) a deeper comparison based on studies using both PGM and other ML methods on the same dataset. Performance was assessed using AUROC, SEN, SPE, NPV, and PPV, as these metrics have been reported commonly across several studies. In addition to the performance comparison, an analysis of pediatric sepsis definitions was also conducted from selected publications.
Results
Our search identified 3,244 studies, with 2,483 remaining after duplicate removal. Following title/abstract screening, 1,968 irrelevant studies were filtered. An additional 443 were excluded after the full-text screening, leaving 72 studies that met our inclusion criteria (Figure 1, Table S1). Among them, 59 studies (81.9%) were published after 2018 with most studies published in 2021 (n=19, 26.4%). There was a total of 57 retrospective studies (79.2%) conducted using EHR, eight literature reviews, one randomized controlled clinical trial, three observational cohort studies, a derivation-validation, a case-control and a prospective study. We analyzed the use of different pediatric sepsis definitions and classified them into four major categories: (I) positive cultures (n=19, 26.4%), (II) systemic inflammatory response syndrome (SIRS) with suspected or proven infections (IPCSS, 2005, n=11, 15.3%), (III) dysregulated infection response with organ dysfunction criteria (Adapted Sepsis-3, 2016, n=7, 9.7%), and (IV) general infections, including bacteremia, bacterial, viral, or fungal infections, (n=3, 4.2%) (Table 2). The remaining definitions vary from international classification of disease codes, clinician reviews, to the time of antibiotic administration (26-33). We observed an increase in Sepsis-3 use (n=1 before 2020, n=6 after 2020), even though positive cultures continue to be the most widely used definition (n=8 before 2020, n=11 after 2020). Most studies utilized only one definition to identify the sepsis cohort, and some did not provide a rationale as to why a particular definition was chosen (n=11, 15.3%). Number of study participants ranged from 15 to 35,074, with a number of studies focusing on infants (n=25, 34.7%). The incidence of sepsis ranged from 1.2–81%. Among the 25 studies focusing on infants, the common sepsis definitions used were positive cultures (n=14, 56%), bacterial sepsis (n=3, 12%), time of antibiotic administration (n=3, 12%). The study objectives in this group of studies mostly focused on identifying early and late onset sepsis or distinguishing between sepsis and other signs of infection such as SIRS.
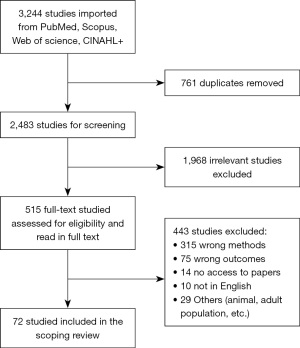
Table 2
Definitions | Description |
---|---|
IPSCC [2005] | SIRS and presence of suspected or proven infections |
Positive cultures | Positive cultures of blood, CSF, etc. |
Adapted Sepsis-3 [2016] | Dysregulated host response to infection and dysfunctional organs measured by age-based pSOFA |
General infections | |
Bacteremia | Blood stream infections with positive cultures |
Bacterial infection | Bacterial infection with or without positive cultures |
Viral infection | Viral infection with or without positive cultures |
Fungal infection | Invasive fungal infection with or without positive cultures |
In this review, asymptomatic patients with culture positive are not considered to have sepsis. CSF, cerebrospinal fluid; IPSCC, international pediatric sepsis consensus conference; SIRS, systemic inflammatory response syndrome; pSOFA, pediatric sequential organ failure assessment.
The application of ML models in pediatric sepsis is limited to early prediction, risk calculation, and biomarker identification, while studies on sepsis treatment and progression remain relatively scarce. Among the most common ML approaches used were logistic regression (LR, n=27), random forest (RF, n=24), and neural network (NN, n=18). In addition, other tree-based models, including classification and regression tree (CART, n=6), decision tree (DT, n=5), gradient boosted decision tree (GBDT, n=8), extra trees (ET, n=3), bagged trees (n=1), are also frequently used. Commonly chosen performance metrics were AUROC (n=49, 68.1%), and SEN/SPE (n=29, 40.3%) (Table S1). PGM models were used in 13 studies (18.1%, Table 3). PGM methods used included naïve Bayes (NB, n=9), tree augmented naïve Bayes (TAN, n=2), hidden Markov model (HMM, n=2), and Markov chain (n=1). Overall, the performance of PGM (AUROC: 0.59–0.91, SEN: 0–0.84, SPE: 0.18–0.99, NPV: 0.31–0.98, PPV: 0.28–0.80) vary on different settings and datasets. When comparing PGM and other MLs on the same dataset, PGM showed a relatively inferior performance to other MLs in most cases (Tables 3,4). Furthermore, the studies that used both PGM and other MLs examined and compared only the quantitatively measurable aspects of the methods (e.g., AUROC, SEN, SPE), whereas other attributes, such as explainability and visualization, were not examined. Otherwise, only three studies (34-36) utilized XAI tools, such as SHAP or LIME, to enhance the interpretation of the ML process/models.
Table 3
Authors, year (number of patients) | Objectives | ML methods | PGM methods | Performance metrics |
Other ML results | PGM results | PGM and ML methods performance comparison |
---|---|---|---|---|---|---|---|
Mani et al., 2014 (N=299) | To develop non-invasive predictive models for late-onset neonatal sepsis from off-the-shelf medical data and EHR | SVM, AODE, K-NN, DT, CART, RF, LR, LBR | NB, TAN | SEN, SPE, AUC, PPV, NPV |
SEN (0.75–0.88), SPE (0.18–0.36), PPV (0.68–0.71), NPV (0.24–0.38), AUC (0.54–0.65) |
SEN (0.75–0.84), SPE (0.18–0.32), PPV (0.7), NPV (0.31–0.32), AUC (0.59–0.64) |
PGM (NB, TAN) yielded comparable results with other ML methods in all performance metrics |
Stanculescu et al., 2014 (N=36) | To use Autoregressive HMM to model the distribution of the physiological events to detect early neonatal sepsis | Autoregressive HMM | AUC, EER | AUC (0.72 to 0.8), EER (0.27–0.34) | PGM (autoregressive HMM) yielded high AUC (0.72–0.8) in all reported models | ||
Gomez et al., 2019 (N=79) | To develop a minimally invasive and cost-effective tool, based on HRV monitoring and ML algorithms, to predict sepsis risk in neonates within the first 48 hours of life | RF, LR, SVM, AdaBoost, Bagged Trees, Classification Tree, K-NN | NB | SEN, SPE, PPV, NPV, AUC |
SEN (0.57–0.94), SPE (0.72–0.95), PPV (0.67–0.95), NPV (0.62–0.94), AUC (0.64–0.94) |
SEN (0.43), SPE (0.9), PPV (0.8), NPV (0.6), AUC (0.67) |
PGM (NB) yielded comparable SPE, PPV, NPV with other ML methods. However, the yielded NPV, SEN and AUC were lower |
Masino et al., 2019 (N=1,188) | To develop a model using readily available EHR data capable of recognizing infant sepsis at least 4 hours prior to clinical recognition | LR, RF, SVM, K-NN, Gaussian process, AdaBoost, GBDT | NB | AUC, SPE, NPV, PPV | SPE (0.6–0.74), PPV (0.39–0.53), NPV (0.9–0.93), AUC (0.79–0.87) |
SPE (0.73), PPV (0.52), NPV (0.92), AUC (0.84) | PGM (NB) yielded comparable results with other ML methods in all performance metrics |
Honore et al., 2020 (N=3,501) | To explore the use of traditional and contemporary HMM for sequential physiological data analysis and sepsis prediction in preterm infants | GMM-HMM, Flow-HMM, DFlow-HMM | ACC | ACC (0.69–0.75) | PGM (HMMs) yielded relatively high ACC (0.69–0.75) | ||
Song et al., 2020 | To examine the feasibility of a prediction model by using noninvasive vital sign data and machine learning technology | LR, RF, DT, ET, GBDT, AdaBoost, Bagging Classifier, multilayer perceptron | Gaussian NB | AUC, ACC, F1, PPV, NPV |
ACC (0.81–0.87), AUC (0.77–0.86), F1 (0.13–0.52), PPV (0.28–0.53), NPV (0.87–0.96) |
ACC (0.69), AUC (0.82), F1 (0.42), PPV (0.28), NPV (0.96) | PGM (GNB) yielded comparable results with other ML methods in most performance metrics. The yielded ACC, PPV were lower |
Cabrera-Quiros et al., 2021 (N=64) | To predict late-onset sepsis in preterm infants, based on multiple patient monitoring signals 24 hours before onset | LR, nearest mean classifier | NB | SEN, SPE, PPV | SEN (0.68–0.82), SPE (0.75–0.8), PPV (0.73–0.82) |
SEN (0.68±0.09), SPE (0.74±0.15), PPV (0.73±0.13) | PGM (NB) yielded comparable performance compared to other ML methods |
Ying et al., 2021 (N=364) | To develop an optimal gene model for the diagnosis of pediatric sepsis using statistics and machine learning approaches | ET, RF, SVM, GBDT, NN | NB | AUC | AUC (0.8–0.94) | AUC (0.8–0.91) | PGM (NB) yielded comparable AUC with other ML |
Kausch et al., 2021 (N=1,425) | To use Markov chain modeling to describe disease dynamics over time by describing how children transition between illness states | Markov chain | Markov chain | AUC | AUC (0.750, 95% CI: 0.708–0.809) | PGM (Markov chain) yielded relatively high AUC (0.75) | |
Chen et al. 2023 (N=677) | To develop and validate a predictive model for postoperative sepsis within seven using ML | SVM, RF, GBM, AdaBoost, MLP |
GNB | AUC, SEN, SPE, F1 | AUC (0.71–0.73), SEN (0.26–0.65), SPE (0.71–0.94), F1 (0.37–0.58) |
AUC (0.724), SEN (0), SPE (1), F1 (0) | PGM (GNB) did not perform well in this study compared to other MLs |
Mercurio et al. 2023 (N=35,074) | To identify sepsis risk factors among children presenting to a pediatric emergency department | SVM, LR, CART | NB | SEN, SPE, PPV, AUC, F1 |
AUC (0.77–0.81), SEN (0.7–0.93), SPE (0.7–0.92), PPV (0.02–0.05), F1 (0.04–0.1) |
AUC (0.65), SEN (0.37), SPE (0.94), PPV (0.04), F1 (0.07) | PGM (NB) yielded lower performance compared to other ML methods |
Nguyen et al. 2023 (N=3,014) | To explore the utility of PGM in pediatric sepsis in the pediatric intensive care unit | TAN | ACC, SEN, SPE, AUC, PPV, NPV | ACC (0.77–0.97), SEN (0.2–0.48), SPE (0.89–0.99), AUC (0.74–0.89), PPV (0.24–0.53), NPV (0.79–0.98) |
PGM (TAN) yielded high SPE, AUC, and NPV but low SEN and PPV | ||
Honore et al. 2023 (N=325) | To investigate the predictive value of ML-assisted analysis of non-invasive, high frequency monitoring data and demographic factors to detect neonatal sepsis | NB | AUC, LR+, LR− | AUC (0.69–0.81), LR+ (1.7–3.5), LR− (0.2–0.5) |
PGM (NB) yielded relatively high AUC |
PGM, probabilistic graphical model; ML, machine learning; EHR, electronic health records; SVM, support vector machine; AODE, averaged one dependence estimators; K-NN, K-Nearest Neighbor; DT, decision tree; CART, classification and regression tree; RF, random forest; LBR, Lazy Bayesian rules; NB, naïve Bayes; TAN, tree augmented naïve Bayes; SEN, sensitivity; SPE, specificity; AUC, area under the curve; PPV, positive predicted value; NPV, negative predicted value; HMM, hidden Markov model; EER, equal error rate; HRV, heart rate variability; LR, logistic regression; GMM-HMM, Gaussian mixture model-hidden Markov model; Flow-HMM, flow-based hidden Markov model; DFlow-HMM, discriminate flow-based hidden Markov model; ET, extra trees; GBDT, Gradient Boosting Decision Tree; ACC, accuracy; CI, confidence interval.
Table 4
Publication | Methods used | AUROC | SEN | SPE | NPV | PPV |
---|---|---|---|---|---|---|
Mani et al., 2014 | RF | 0.57–0.65 | 0.82–0.94 | 0.18–0.47 | 0.28–0.73 | 0.55–0.70 |
SVM | 0.61–0.68 | 0.79–0.88 | 0.18–0.26 | 0.27–0.59 | 0.51–0.69 | |
KNN | 0.54–0.62 | 0.83–0.86 | 0.18–0.29 | 0.30–0.55 | 0.52–0.70 | |
CART | 0.65–0.77 | 0.75–0.81 | 0.18–0.30 | 0.23–0.51 | 0.51–0.68 | |
LR | 0.61 | 0.86–0.87 | 0.18–0.33 | 0.35–0.57 | 0.52–0.72 | |
LBR | 0.58–0.62 | 0.86–0.85 | 0.18–0.33 | 0.36–0.52 | 0.52–0.72 | |
AODE | 0.53–0.61 | 0.85–0.88 | 0.18–0.36 | 0.38–0.54 | 0.52–0.73 | |
NB* | 0.64–0.78* | 0.83–0.95* | 0.18–0.47* | 0.31–0.76* | 0.55–0.72* | |
TAN* | 0.53–0.59* | 0.84* | 0.18–0.32* | 0.32–0.52* | 0.50–0.72* | |
Gomez et al., 2019 | Adaboost | 0.943 | 0.944 | 0.944 | 0.942 | 0.945 |
Bagged trees | 0.88 | 0.901 | 0.858 | 0.896 | 0.866 | |
RF | 0.84 | 0.861 | 0.818 | 0.853 | 0.827 | |
LR | 0.787 | 0.771 | 0.804 | 0.777 | 0.8 | |
SVM | 0.755 | 0.641 | 0.868 | 0.705 | 0.831 | |
DT | 0.751 | 0.816 | 0.687 | 0.788 | 0.726 | |
KNN | 0.64 | 0.565 | 0.715 | 0.62 | 0.667 | |
NB* | 0.666* | 0.431* | 0.901* | 0.61* | 0.814* | |
Masino et al., 2019 | Adaboost | 0.83–0.85 | 0.8 | 0.72 | 0.92 | 0.51 |
GB | 0.8–0.87 | 0.8 | 0.74 | 0.92 | 0.53 | |
GP | 0.75–0.79 | 0.8 | 0.6 | 0.9 | 0.44 | |
KNN | 0.73–0.79 | 0.8 | 0.55 | 0.9 | 0.39 | |
LR | 0.83–0.85 | 0.8 | 0.74 | 0.93 | 0.52 | |
RF | 0.82–0.86 | 0.8 | 0.74 | 0.92 | 0.53 | |
SVM | 0.82–0.86 | 0.8 | 0.72 | 0.92 | 0.51 | |
NB* | 0.81–0.84* | 0.8* | 0.73* | 0.92* | 0.52* | |
Song et al., 2020 | LR | 0.86 | – | – | 0.94–0.96 | 0.4–0.5 |
DT | 0.6–0.84 | – | – | 0.84–0.95 | 0.39–0.57 | |
AdaBoost | 0.81–0.83 | – | – | 0.91–0.94 | 0.41–0.53 | |
ET | 0.80 | – | – | 0.81–0.88 | 0.53–0.68 | |
Bagging | 0.77–0.81 | – | – | 0.83–0.88 | 0.45–0.59 | |
RF | 0.81–0.82 | – | – | 0.83–0.88 | 0.51–0.66 | |
GB | 0.86–0.87 | – | – | 0.92–0.94 | 0.45–0.56 | |
GNB* | 0.81–0.82* | –* | –* | 0.95–0.96* | 0.28–0.38* | |
Cabrera-Quiros et al., 2021 | LR | 0.79 | 0.78 | 0.8 | – | 0.82 |
NN | 0.7 | 0.67 | 0.74 | – | 0.73 | |
NB* | 0.71* | 0.68* | 0.74* | – | 0.73* | |
Mercurio et al., 2023 | RF | 0.81 | 0.93 | 0.84 | – | 0.04 |
CART | 0.77 | 0.85 | 0.7 | – | 0.02 | |
LR | 0.82 | 0.76 | 0.88 | – | 0.04 | |
SVM | 0.81 | 0.7 | 0.92 | – | 0.05 | |
GNB* | 0.65* | 0.37* | 0.94* | –* | 0.04* | |
Chen et al., 2023 | LR | 0.726 | 0.541 | 0.786 | – | – |
SVM | 0.71 | 0.648 | 0.706 | – | – | |
RF | 0.731 | 0.621 | 0.761 | – | – | |
GBM | 0.716 | 0.257 | 0.944 | – | – | |
AdaBoost | 0.723 | 0.258 | 0.937 | – | – | |
MLP | 0.718 | 0.343 | 0.877 | – | – | |
GNB* | 0.724* | 0* | 1* | –* | –* |
In this table, PGM methods are marked with “*”. Metrics presented in the table (AUROC, SEN, SPE, NPV, PPV) were those reported in the respective studies. We excluded Stanculescu et al. (2014), Honore et al. (2020), Ying et al. (2021), Kausch et al. (2021), Nguyen et al. (2023), and Honore et al. (2023) from this table because they only use single ML model or reported only AUROC. PGM, probabilistic graphical model; ML, machine learning; AUROC, area under the receiver-operating curve; SPE, specificity; SEN, sensitivity; NPV, negative predicted value; PPV, positive predicted value; RF, random forest; SVM, support vector machine; KNN, K-Nearest Neighbour; LR, logistic regression; LBR, Lazy Bayesian rules; AODE, averaged one dependence estimators; NB, naïve Bayes; TAN, tree augmented naïve Bayes; DT, decision tree; GB, Gradient Boosting; GP, Gaussian Process; ET, extra trees; NN, neural network; CART, classification and regression tree.
Discussion
In this review of ML-based pediatric sepsis literature, we found that most studies used retrospective EHR data and incorporated multiple ML methods to develop predictive models. LR, RF, and NN were the most frequently used ML methods, while positive cultures and IPSCC were the most widely used sepsis definitions. Our findings suggests that PGM performs relatively inferior compared to other ML techniques in most cases. In this section, we review the general ML approaches and assess the potential of PGM in pediatric sepsis.
ML approaches in pediatric sepsis
We observed that in pediatric sepsis, LR, RF, and NN were the most common ML methods, followed by the tree-based models. Similar to linear regression (fitting of a regression line to the data), the LR concept uses the sigmoid function in order to fit an S-curve to the data and determine the probability of the outcome. The RF consists of several small decision trees that work together as an ensemble, where the final decision is determined by a majority vote. Finally, a neural network is a structure of interconnected nodes nested in several layers, where each node is associated with a weight and an activation function. Secondly, the ML studies follow a similar structure for data processing, feature selection, model fitting, testing, and sensitivity analysis. Data is usually divided into training and testing subsets for model learning and validation. To reduce biases during the learning process, k cross-validations can be performed on the whole dataset or the training set. Model fitting is often preceded by feature selection methods such as Elastic Nets, RF, and Lasso when a number of variables are involved (37). Metrics of performance are carefully considered in ML tasks, and sensitivity analyses are usually required for studying the behavior of models under different conditions.
The application of ML models in pediatric sepsis is limited to early prediction, risk calculation, and biomarker identification, while studies on sepsis treatment and progression remain relatively scarce. It is expected that these topics should be explored further, especially in the area of sepsis management. Furthermore, research based on physiological markers, laboratories, heart rate variability, and genes are promising areas for future endeavors (38). Likewise, image and text data should be examined in pediatric sepsis in the same manner as they have been examined in adult sepsis (13,39,40). Finally, while traditional methods like LR and RF continue to be the popular choices in pediatric sepsis, a growing number of studies are incorporating deep learning into diagnostic models of sepsis (15,31,40,41). Despite the remarkable performance compared to traditional models, deep learning still falls short in several areas. To start, a large sample size is required for training. As for its models, they may have been termed ‘black boxes’, raising questions about whether the produced predictions can be trusted. Nevertheless, we cannot deny the growing potential and achievements of deep learning methods. Therefore, it is necessary to obtain additional evidence and validation before drawing any definitive conclusions about this controversial issue (42).
Even though most ML studies seek to improve performance, their biggest drawback perhaps lies in the lack of transparency in reasoning and interpretation. An “ideal” ML study in medicine must demonstrate trustworthiness, explainability, usability, reliability, transparency, and fairness (43). The literature has shown that although several ML approaches excel in terms of usability, performance, and proof-of-concept, not many are proficient in trustworthiness, transparency or explainability. Unfortunately, it is these areas that are crucial for ML studies to be accepted by the medical community (14). This problem applies not only to the entire field of medicine, but also to pediatric sepsis applications. We propose that future pediatric sepsis ML studies should consider methods that demonstrate not only efficacy and robustness, but also explainability, reliability, and trustworthiness A further layer of explainability can be achieved by using additional XAI tools, such as SHAP or LIME, to enhance interpretation of the model results. This is a realistic attempt to bridge the gap between theoretical ML frameworks and practical applications. In this paper, we assess PGM as an approach among the ML methods that can potentially meet these requirements.
PGM in pediatric sepsis
A total of 13 PGM studies were included in our review (nine NB, three HMM, and one TAN) (33,44-55). Comparing these studies with other ML methods, we observed the following points. As anticipated, the utilization of PGM in pediatric sepsis remains low. A low number of PGM studies may lead to the premature perception that PGM is an unreliable tool; however, this may not be the case as it has been shown to be effective in some precedent studies (42,43). In general, the performance of PGM, with NB being the most used model, appeared relatively less efficient compared to other models. NB may not be the most suitable prediction model for complicated medical conditions, such as pediatric sepsis. As its name suggests, the NB model is based on the naïve assumption that all variables are independent of each other (56). While NB is known to produce remarkable results when dealing with data with less associated variables and outcomes, it is not suitable for describing data with complex relationships. Pediatric sepsis is an excellent example of having such data, where the associations between variables such as history and physical examination findings, vital signs, and laboratory markers are highly correlated. As a result, NB performance was relatively inferior, whereas other models showed better results because they were able to describe the data more accurately. We observed the same situation in other diseases (e.g., adult sepsis, cancer, or cardiovascular) that NB performance is inferior to its PGM relative methods, such as Bayesian network (BN), dynamic Bayesian network (DBN) or HMM (38-40,57).
A comparison of the characteristics of PGM and other ML methods is presented in Table 5. Among the popular methods that are often used in the literature for PGM, we selected BN, NB, TAN, HMM, DBN, and influence diagram (ID). Other ML methods that we chose from our review include LR, RF, support vector machine (SVM), NN, extreme gradient boosting (XGBoost), and DT. We observed that all methods exhibit different strengths in different areas. For instance, several of the other ML methods shown in Table 5 are capable of performing both classification and regression, while PGM can only perform classification. The NN and the SVM excel on several criteria; however, they require longer training time, hardware dependence, and additional aids for visualization and interpretation. Additionally, certain limitations highlighted in some areas can be overcome through alternative solutions and additional assistance, such as pre-data processing, visualization aids, and XAI. For instance, continuous data and time series data can be broken down into categorical and sliding-window data to use with methods that do not natively support them. The main disadvantage of all methods is that they are sensitive to outliers, imbalanced data, and easily prone to overfitting when the model settings are not probably figured. The complexity of the model, the training time, and the interpretability of the results are also subjected to trade-offs. It is likely that highly complex models will require a larger amount of training time and be more challenging to interpret.
Table 5
Characteristics | PGM method | Other ML methods | ||||||||||||
---|---|---|---|---|---|---|---|---|---|---|---|---|---|---|
TAN | NB | HMM | ID | BN | DBN | LR | RF | SVM | NN | XGBoost | DT | |||
Data handling | ||||||||||||||
Handling small data size | * | * | * | * | * | * | * | |||||||
Handling big dataset | * | * | * | * | * | * | * | * | ||||||
Handling missing data | * | * | * | * | * | * | * | * | * | * | ||||
Handling imbalance data | ||||||||||||||
Handling noisy data | * | * | * | * | * | * | * | * | ||||||
Handling outliers | * | * | * | * | ||||||||||
Usage on continuous data | * | * | * | |||||||||||
Usage on category data | * | * | * | * | * | * | * | * | * | |||||
Usage on time-series data | * | * | * | * | ||||||||||
Variable selection | * | * | * | * | * | |||||||||
Presentation | ||||||||||||||
Visualization | * | * | * | * | * | * | * | * | * | |||||
Capability | ||||||||||||||
Classification | * | * | * | * | * | * | * | * | * | * | * | |||
Regression | * | * | * | * | * | |||||||||
Causal inference | * | * | * | * | * | * | ||||||||
Support decision-making | * | * | * | * | ||||||||||
Natural language processing | * | * | * | * | * | * | * | * | * | * | ||||
Image processing | * | * | * | * | * | * | * | * | * | * | ||||
Interpretation | ||||||||||||||
Explainable method | * | * | * | * | * | * | * | * | * | * | ||||
Computational requirement | ||||||||||||||
Require hardware dependency | * | * | * | |||||||||||
Require more training time | * | * | * | |||||||||||
Prone to overfitting | * | * | * | * | * | * | * | * | * | * | * | * |
An asterisk “*” indicates that the respective characteristic is available. It is important to note that each method exhibits different strengths in different areas. In this table, the characteristics described are not meant to be exhaustive. Additionally, certain limitations highlighted in some areas can be overcome through alternative solutions. It has been demonstrated that some of the characteristics of data handling, visualization, and explainability can be overcome through the use of additional assistance, such as pre-data processing, visualization aids, and explainable artificial intelligence (XAI). For instance, continuous data and time series data can be broken down into categorical and sliding-window data to use with methods that do not natively support them. PGM, probabilistic graphical model; ML, machine learning; TAN, tree augmented naïve Bayes; NB, naïve Bayes; HMM, hidden Markov model; ID, influence diagram; BN, Bayesian network; DBN, dynamic Bayesian network; LR, logistic regression; RF, random forest; SVM, support vector machine; NN, neural network; XGBoost, extreme gradient boosting; DT, decision tree.
In comparison with other ML methods, PGM has certain advantages and is particularly appealing with an interactive graphical representation, a wide range of methods, transparent reasoning, the ability for causal inference and handling missing data (Table 5). While there are presentation elements for other MLs (e.g., LR, SVM, NN), these are often confined to two or three dimensions requiring additional visualization aid (saliency maps, activation maximization). PGM, in contrast, is able to present high-dimensional data in the form of a compact and friendlier network, in which variables and their relationships are represented as nodes and edges. This representation allows clinicians to conduct causal inference and prediction tasks simultaneously, allowing them to diagnose as well as investigate the interactions between variables. As a result, this can facilitate the study of sepsis recognition, the calculation of sepsis risk, and the identification of sepsis biomarkers. Additionally, PGM has an extensive body of established methods that can be applied to a wide variety of data types (e.g., text, images, tabular data, and time series data), and can be tailored to meet various requirements (e.g., prediction, inference, and decision making) (18). In this way, PGM is capable of processing clinical images, physician notes, and the creation of monitoring and decision-support tools together with the causal inference ability. Figure 2 illustrates several PGM methods for sepsis applications, including TAN, NB, Markov models, and ID for assessing sepsis, monitoring the disease’s progression, and making clinical decisions. Finally, PGM provides transparency in reasoning, which is one of the most desirable characteristics for medical applications (43). At any point in time, the mechanism by which it produces its predictions can be explained using probability and graph theory; therefore there is no need to apply additional XAI tools. Considering the transparency requirement of the medical application and the future direction for pediatric sepsis research, we recommend that PGM be considered as a potential tool in pediatric sepsis diagnosis application among other ML methods.
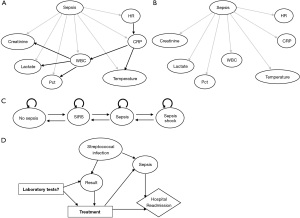
While PGMs have many benefits, the following drawbacks should be considered prior to selecting them as the prediction model for sepsis diagnosis. PGM does not support variable selection or regression. Despite the fact that it is capable of handling missing data, it may be necessary to perform pre-data processing in order to remove outliers and convert continuous variables into categorical variables. Furthermore, PGM faces the same limitations as other ML methods when dealing with imbalance data, which will require additional treatment before the model can be trained. Conventional PGMs also have the disadvantage of requiring expertise to select the variables and define their relationships during the model construction phase. While these models can leverage domain knowledge from experts, they are prone to bias and are difficult to modify. Moreover, it is impractical and time-consuming for experts to construct networks containing many variables, as is typically the case with sepsis diagnosis. Today, PGM structure is primarily extracted directly from data. However, these data-driven models, much like other ML data-driven models, are often data-specific and may suffer from poor generalization to another dataset. Moreover, even though explainability and transparency give PGM more advantages from other methods in ML, these qualities may have contributed to some degradation of its performance as well (58).
Pediatric sepsis definitions used in ML research
Sepsis definition plays a significant role in determining the study cohort and has a direct impact on ML model performance to detect actual cases of sepsis. In this subsection, we evaluate the use of pediatric sepsis definitions in ML studies.
Since the publication of the revised adult sepsis definition in 2016 and the launch of the Surviving Sepsis Campaign in 2020, an increasing number of studies have incorporated dysfunctional organ criteria as a means to detect sepsis in children (59,60). Several reasons may account for the preference for positive cultures in these studies, including the fact that infants exhibit non-specific clinical symptoms of sepsis. Moreover, it is possible that the selection of sepsis definitions in ML-based studies may be constrained by the information contained in the dataset, with the absence of certain variables preventing researchers from considering certain definitions. For instance, Sepsis-3 cannot be used if there is insufficient data to measure the organ dysfunction criteria. More importantly, we observed that most studies utilized only one definition to identify the sepsis cohort, and some failed to provide a rationale as to why a particular definition was chosen (34,50,61).
Table 2 shows the categories of the pediatric sepsis definition we analysed from the ML studies. These categories overlapped with one another, and a patient with sepsis might fall into either of them, as demonstrated by the Sepsis Prevalence, Outcomes, and Therapies (SPROUT) study involving 128 PICUs from 26 countries (Figure 3) (6). This indicates that pediatric sepsis cannot be reliably identified through a single definition and that utilizing more than one definition could increase the likelihood to identify sepsis cases. As for ML models, given that no single definition is capable of identifying sepsis effectively, a model trained on a singular definition may not be sufficiently generalizable. In contrast, a model trained on multiple definitions will be exposed to different characteristics of sepsis, thereby increasing its ability to recognize one later on. We hypothesize that, with a proper structure and set up, ML methods may have the capability to learn the characteristics of these subgroups. However, this approach needs to be tested and validated in future studies.
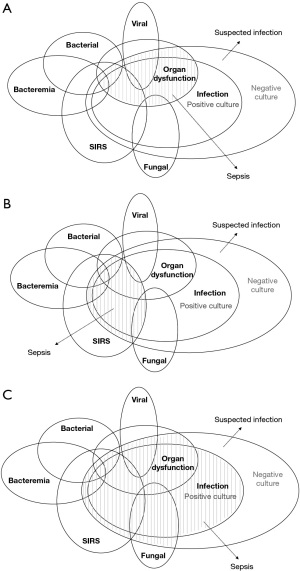
Recommendation
In view of the PGM’s inherent qualities and potential for clinical use, we recommend that researchers consider using PGM methods in future studies of pediatric sepsis. Additionally, we recommend that ML studies include more than one definition of sepsis in order to enhance their predictive capabilities. In the event that a dataset does not contain enough information to extract more than one definition, researchers may consider combining several datasets. More granular data should also be collected in future original studies on sepsis to facilitate the extraction of multiple sepsis definitions. Finally, it would be desirable to conduct studies that could compare single definition-learned model with the multiple definitions-learned model in order to validate our hypothesis that combining two or more sepsis definitions will improve performance of ML methods.
Study limitations
There are several limitations to this scoping review. Our review included only articles published in PubMed, Scopus, Web of Science, and CINAHL+. Therefore, it is possible that we have missed some publications in other databases. Moreover, given the pace of ML advancement, our results could be quickly complemented by new studies. For evaluation of ML models, it is recommended to use a number of different metrics, including AUROC, AUPRC, F1-score, G-mean, and more. Ideally, our performance comparison should have been conducted using these metrics. However, not all of the metrics mentioned above were reported in the selected studies. Comparing models in this manner may not represent all aspects of their performance accurately. Finally, we did not assess the quality of the selected studies, which could have provided readers with a broader perspective and evaluation. However, the quality assessment of included studies is not considered mandatory in the PRISMA-SCr guidelines (23).
Conclusions
This scoping review summarizes ML and PGM approaches in pediatric sepsis over the past two decades and discusses how sepsis definitions were applied in these studies. The performance of PGM was relatively inferior to other ML methods. However, with the advantages of explainability and transparency, PGM can be considered as a viable tool for future pediatric sepsis studies and application.
Acknowledgments
Funding: This work was supported by National Research Medical Council, Singapore (grant number MOH-TA19nov-0001).
Footnote
Reporting Checklist: The authors have completed the PRISMA-ScR reporting checklist. Available at https://tp.amegroups.com/article/view/10.21037/tp-23-25/rc
Peer Review File: Available at https://tp.amegroups.com/article/view/10.21037/tp-23-25/prf
Conflicts of Interest: All authors have completed the ICMJE uniform disclosure form (available at https://tp.amegroups.com/article/view/10.21037/tp-23-25/coif). All authors report this study has received payment from National Research Medical Council, Singapore. J.H.L. is serving as a Deputy Editor-in-Chief of Translational Pediatrics from July 2022 to June 2024. The authors have no other conflicts of interest to declare.
Ethical Statement: The authors are accountable for all aspects of the work in ensuring that questions related to the accuracy or integrity of any part of the work are appropriately investigated and resolved.
Open Access Statement: This is an Open Access article distributed in accordance with the Creative Commons Attribution-NonCommercial-NoDerivs 4.0 International License (CC BY-NC-ND 4.0), which permits the non-commercial replication and distribution of the article with the strict proviso that no changes or edits are made and the original work is properly cited (including links to both the formal publication through the relevant DOI and the license). See: https://creativecommons.org/licenses/by-nc-nd/4.0/.
References
- Fleischmann-Struzek C, Goldfarb DM, Schlattmann P, et al. The global burden of paediatric and neonatal sepsis: a systematic review. Lancet Respir Med 2018;6:223-30. [Crossref] [PubMed]
- Molloy EJ, Wynn JL, Bliss J, et al. Neonatal sepsis: need for consensus definition, collaboration and core outcomes. Pediatr Res 2020;88:2-4. [Crossref] [PubMed]
- Tan B, Wong JJ, Sultana R, et al. Global Case-Fatality Rates in Pediatric Severe Sepsis and Septic Shock: A Systematic Review and Meta-analysis. JAMA Pediatr 2019;173:352-62. [Crossref] [PubMed]
- Santos RP, Tristram D. A practical guide to the diagnosis, treatment, and prevention of neonatal infections. Pediatr Clin North Am 2015;62:491-508. [Crossref] [PubMed]
- Weiss SL, Fitzgerald JC, Pappachan J, et al. Global epidemiology of pediatric severe sepsis: the sepsis prevalence, outcomes, and therapies study. Am J Respir Crit Care Med 2015;191:1147-57. [Crossref] [PubMed]
- Schlapbach LJ, Straney L, Alexander J, et al. Mortality related to invasive infections, sepsis, and septic shock in critically ill children in Australia and New Zealand, 2002-13: a multicentre retrospective cohort study. Lancet Infect Dis 2015;15:46-54. [Crossref] [PubMed]
- Schlapbach LJ, Kissoon N. Defining Pediatric Sepsis. JAMA Pediatr 2018;172:312-4. [Crossref] [PubMed]
- Matics TJ, Sanchez-Pinto LN. Adaptation and Validation of a Pediatric Sequential Organ Failure Assessment Score and Evaluation of the Sepsis-3 Definitions in Critically Ill Children. JAMA Pediatr 2017;171:e172352. [Crossref] [PubMed]
- Gupta S, Sakhuja A, Kumar G, et al. Culture-Negative Severe Sepsis: Nationwide Trends and Outcomes. Chest 2016;150:1251-9. [Crossref] [PubMed]
- Moor M, Rieck B, Horn M, et al. Early Prediction of Sepsis in the ICU Using Machine Learning: A Systematic Review. Front Med (Lausanne) 2021;8:607952. [Crossref] [PubMed]
- Singh YV, Singh P, Khan S, et al. A Machine Learning Model for Early Prediction and Detection of Sepsis in Intensive Care Unit Patients. J Healthc Eng 2022;2022:9263391. [Crossref] [PubMed]
- Fan Y, Han Q, Li J, et al. Revealing potential diagnostic gene biomarkers of septic shock based on machine learning analysis. BMC Infect Dis 2022;22:65. [Crossref] [PubMed]
- Yan MY, Gustad LT, Nytrø Ø. Sepsis prediction, early detection, and identification using clinical text for machine learning: a systematic review. J Am Med Inform Assoc 2022;29:559-75. [Crossref] [PubMed]
- Ben-Israel D, Jacobs WB, Casha S, et al. The impact of machine learning on patient care: A systematic review. Artif Intell Med 2020;103:101785. [Crossref] [PubMed]
- Sendak MP, Ratliff W, Sarro D, et al. Real-World Integration of a Sepsis Deep Learning Technology Into Routine Clinical Care: Implementation Study. JMIR Med Inform 2020;8:e15182. [Crossref] [PubMed]
- Komorowski M. Clinical management of sepsis can be improved by artificial intelligence: yes. Intensive Care Med 2020;46:375-7. [Crossref] [PubMed]
- Koller D, Friedman N. Probabilistic graphical models: principles and techniques. Cambridge, MA: MIT Press; 2009. 1231 p. (Adaptive computation and machine learning).
- Sucar LE. Relational Probabilistic Graphical Models. In: Probabilistic Graphical Models [Internet]. London: Springer London; 2015 [cited 2021 Apr 15]. p. 219-35. (Advances in Computer Vision and Pattern Recognition).
- Javorník M, Dostál O, Roček A. Probabilistic Modelling and Decision Support in Personalized Medicine. In: Kriksciuniene D, Sakalauskas V, editors. Intelligent Systems for Sustainable Person-Centered Healthcare. Cham: Springer International Publishing; 2022. p. 211-23.
- Gupta A, Slater JJ, Boyne D, et al. Probabilistic Graphical Modeling for Estimating Risk of Coronary Artery Disease: Applications of a Flexible Machine-Learning Method. Med Decis Making 2019;39:1032-44. [Crossref] [PubMed]
- de Benedetti J, Oues N, Wang Z, et al. Practical Lessons from Generating Synthetic Healthcare Data with Bayesian Networks. In: Koprinska I, Kamp M, Appice A, et al., editors. ECML PKDD 2020 Workshops [Internet]. Cham: Springer International Publishing; 2020. p. 38-47.
- Tricco AC, Lillie E, Zarin W, et al. PRISMA Extension for Scoping Reviews (PRISMA-ScR): Checklist and Explanation. Ann Intern Med 2018;169:467-73. [Crossref] [PubMed]
- Covidence [Internet]. Available online: https://www.covidence.org/organizations/
- Palacio-Niño JO, Berzal F. Evaluation Metrics for Unsupervised Learning Algorithms [Internet]. arXiv; 2019 [cited 2023 Sep 7]. Available online: http://arxiv.org/abs/1905.05667
- Chaddad A, Peng J, Xu J, et al. Survey of Explainable AI Techniques in Healthcare. Sensors (Basel) 2023;23:634. [Crossref] [PubMed]
- Matikolaie FS, Tadj C. Machine Learning-Based Cry Diagnostic System for Identifying Septic Newborns. J Voice 2022;S0892-1997(21)00452-5.
- Leon C, Carrault G, Pladys P, et al. Early Detection of Late Onset Sepsis in Premature Infants Using Visibility Graph Analysis of Heart Rate Variability. IEEE J Biomed Health Inform 2021;25:1006-17. [Crossref] [PubMed]
- Hsu JF, Chang YF, Cheng HJ, et al. Machine Learning Approaches to Predict In-Hospital Mortality among Neonates with Clinically Suspected Sepsis in the Neonatal Intensive Care Unit. J Pers Med 2021;11:695. [Crossref] [PubMed]
- Huang B, Wang R, Masino AJ, et al. Aiding clinical assessment of neonatal sepsis using hematological analyzer data with machine learning techniques. Int J Lab Hematol 2021;43:1341-56. [Crossref] [PubMed]
- Cifra CL, Westlund E, Ten Eyck P, et al. An estimate of missed pediatric sepsis in the emergency department. Diagnosis (Berl) 2021;8:193-8. [Crossref] [PubMed]
- Kamaleswaran R, Akbilgic O, Hallman MA, et al. Applying Artificial Intelligence to Identify Physiomarkers Predicting Severe Sepsis in the PICU. Pediatr Crit Care Med 2018;19:e495-503. [Crossref] [PubMed]
- Back JS, Jin Y, Jin T, et al. Development and Validation of an Automated Sepsis Risk Assessment System. Res Nurs Health 2016;39:317-27. [Crossref] [PubMed]
- Stanculescu I, Williams CK, Freer Y. Autoregressive hidden Markov models for the early detection of neonatal sepsis. IEEE J Biomed Health Inform 2014;18:1560-70. [Crossref] [PubMed]
- Shaw P, Pachpor K, Sankaranarayanan S, Explainable AI. Enabled Infant Mortality Prediction Based on Neonatal Sepsis. Comput Syst Sci Eng 2023;44:311-25.
- Peng Z, Varisco G, Long X, et al. A Continuous Late-Onset Sepsis Prediction Algorithm for Preterm Infants Using Multi-Channel Physiological Signals From a Patient Monitor. IEEE J Biomed Health Inform 2023;27:550-61. [Crossref] [PubMed]
- Fan B, Klatt J, Moor MM, et al. Prediction of recovery from multiple organ dysfunction syndrome in pediatric sepsis patients. Bioinformatics 2022;38:i101-8. [Crossref] [PubMed]
- Saeys Y, Inza I, Larrañaga P. A review of feature selection techniques in bioinformatics. Bioinformatics 2007;23:2507-17. [Crossref] [PubMed]
- Yang AP, Liu J, Yue LH, et al. Neutrophil CD64 combined with PCT, CRP and WBC improves the sensitivity for the early diagnosis of neonatal sepsis. Clin Chem Lab Med 2016;54:345-51. [Crossref] [PubMed]
- Braley SE, Groner TR, Fernandez MU, et al. Overview of diagnostic imaging in sepsis. New Horiz 1993;1:214-30.
- Goh KH, Wang L, Yeow AYK, et al. Artificial intelligence in sepsis early prediction and diagnosis using unstructured data in healthcare. Nat Commun 2021;12:711. [Crossref] [PubMed]
- Alvi RH. Rahman MdH, Khan AAS, Rahman RM. Deep learning approach on tabular data to predict early-onset neonatal sepsis. J Inf Telecommun 2021;5:226-46.
- Lee H, Kim S. Black-Box Classifier Interpretation Using Decision Tree and Fuzzy Logic-Based Classifier Implementation. Int J Fuzzy Log Intell Syst 2016;16:27-35.
- MI in Healthcare Workshop Working Group, Cutillo CM, Sharma KR, et al. Machine intelligence in healthcare—perspectives on trustworthiness, explainability, usability, and transparency. Npj Digit Med 2020;3:47.
- Mani S, Ozdas A, Aliferis C, et al. Medical decision support using machine learning for early detection of late-onset neonatal sepsis. J Am Med Inform Assoc 2014;21:326-36. [Crossref] [PubMed]
- Gomez R, Garcia N, Collantes G, et al. Development of a Non-Invasive Procedure to Early Detect Neonatal Sepsis using HRV Monitoring and Machine Learning Algorithms. In: 2019 IEEE 32nd International Symposium on Computer-Based Medical Systems (CBMS) [Internet]. Cordoba, Spain: IEEE; 2019. p. 132-7.
- Masino AJ, Harris MC, Forsyth D, et al. Machine learning models for early sepsis recognition in the neonatal intensive care unit using readily available electronic health record data. PLoS One 2019;14:e0212665. [Crossref] [PubMed]
- Honore A, Liu D, Forsberg D, et al. Hidden Markov Models for Sepsis Detection in Preterm Infants. In: ICASSP 2020 - 2020 IEEE International Conference on Acoustics, Speech and Signal Processing (ICASSP). Barcelona, Spain: IEEE; 2020. p. 1130–4.
- Song W, Jung SY, Baek H, et al. A Predictive Model Based on Machine Learning for the Early Detection of Late-Onset Neonatal Sepsis: Development and Observational Study. JMIR Med Inform 2020;8:e15965. [Crossref] [PubMed]
- Cabrera-Quiros L, Kommers D, Wolvers MK, et al. Prediction of Late-Onset Sepsis in Preterm Infants Using Monitoring Signals and Machine Learning. Crit Care Explor 2021;3:e0302. [Crossref] [PubMed]
- Ying J, Wang Q, Xu T, et al. Diagnostic potential of a gradient boosting-based model for detecting pediatric sepsis. Genomics 2021;113:874-83. [Crossref] [PubMed]
- Kausch SL, Lobo JM, Spaeder MC, et al. Dynamic Transitions of Pediatric Sepsis: A Markov Chain Analysis. Front Pediatr 2021;9:743544. [Crossref] [PubMed]
- Nguyen TM, Poh KL, Chong SL, et al. Effective diagnosis of sepsis in critically ill children using probabilistic graphical model. Transl Pediatr 2023;12:538-51. [Crossref] [PubMed]
- Chen C, Chen B, Yang J, et al. Development and validation of a practical machine learning model to predict sepsis after liver transplantation. Ann Med 2023;55:624-33. [Crossref] [PubMed]
- Mercurio L, Pou S, Duffy S, et al. Risk Factors for Pediatric Sepsis in the Emergency Department: A Machine Learning Pilot Study. Pediatr Emerg Care 2023;39:e48-56. [Crossref] [PubMed]
- Honoré A, Forsberg D, Adolphson K, et al. Vital sign-based detection of sepsis in neonates using machine learning. Acta Paediatr 2023;112:686-96. [Crossref] [PubMed]
- Padmanaban H. Comparative Analysis of Naive Bayes and Tree Augmented Naive Bayes Models [Internet] [Master of Science]. [San Jose, CA, USA]: San Jose State University; 2014 [cited 2022 Mar 9]. Available online: https://scholarworks.sjsu.edu/etd_projects/356
- Liu S, See KC, Ngiam KY, et al. Reinforcement Learning for Clinical Decision Support in Critical Care: Comprehensive Review. J Med Internet Res 2020;22:e18477. [Crossref] [PubMed]
- Wanner J, Herm LV, Heinrich K, et al. The effect of transparency and trust on intelligent system acceptance: Evidence from a user-based study. Electron Mark 2022;32:2079-102.
- Evans L, Rhodes A, Alhazzani W, et al. Surviving Sepsis Campaign: International Guidelines for Management of Sepsis and Septic Shock 2021. Crit Care Med 2021;49:e1063-143. [Crossref] [PubMed]
- Singer M, Deutschman CS, Seymour CW, et al. The Third International Consensus Definitions for Sepsis and Septic Shock (Sepsis-3). JAMA 2016;315:801-10. [Crossref] [PubMed]
- Banerjee S, Mohammed A, Wong HR, et al. Machine Learning Identifies Complicated Sepsis Course and Subsequent Mortality Based on 20 Genes in Peripheral Blood Immune Cells at 24 H Post-ICU Admission. Front Immunol 2021;12:592303. [Crossref] [PubMed]