Identifying immunodeficiency status in children with pulmonary tuberculosis: using radiomics approach based on un-enhanced chest computed tomography
Highlight box
Key findings
• Radiomics maybe become a new application to identify the immunodeficiency status in children with pulmonary tuberculosis (PTB).
What is known and what is new?
• Children with primary immunodeficiency disease are at a high risk of Mycobacterium tuberculosis infection. And there are differences in PTB between primary immunocompromised and immunocompetent patients in pulmonary imaging.
• This study identifying immunodeficiency status in children with PTB with radiomics using a classification model established by logistic regression.
What is the implication, and what should change now?
• Radiomics would help clinicians to screen the patients of primary immunodeficiency in children with PTB, which will benefit children in high-burden tuberculosis endemic areas. We can apply radiomics to analyze the imaging of children with PTB and screen children at high risk of primary immunodeficiency improving the treatment planning.
Introduction
In 2021, the World Health Organization reported that approximately two billion people were infected with Mycobacterium tuberculosis (Mtb) and more than 10 million had onset with tuberculosis (TB), resulting in 1.6 million deaths. Children accounted for 11% of TB incidence and 16% of TB mortality worldwide and China ranked third amongst 30 high-burden countries for TB (1). Primary immunodeficiency diseases (PIDs), also known as inborn errors of immunity (IEI), are a group of diseases closely associated with genetic abnormalities. The etiology of PIDs is not fully understood, but more than 400 pathogenic genes have been identified (2,3). Children with PIDs are particularly vulnerable to Mtb infection due to congenital immune cell deficiency or dysfunction (4,5). Analogously, newborns and infants with PIDs are at a high risk of BCGosis when receive routine Bacillus Calmette-Guerin (BCG) vaccination in areas where TB is endemic (6,7).
However, diagnosis of PIDs requires genetic test, which is expensive, time-consuming and not comprehensive enough. In developing countries, especially in poor regions, genetic test is usually not possible due to some technological or funding reasons. Therefore, PIDs are often underestimated in children. There were no accurate statistical data of the incidence about PIDs in pulmonary tuberculosis (PTB) at present.
Under the masking of the active PTB manifestations, mycobacterial infection could be the first clinical manifestation with or without PIDs (8). Compared to no-PIDs, the symptoms and signs are not specific in the patients with PIDs, such as the presence of fever, cough and sputum production. Besides, PTB with PIDs are more likely to have other severe complications and sequelae, which can lead to poor healing and even significantly higher mortality (9,10). Immune function evaluation and immune-associated therapy is necessary except for anti-TB therapy for patients of PTB with PIDs. Therefore, identification of the presence or absence of PIDs in children with PTB is crucial for their clinical treatment strategies (8).
Chest computed tomography (CT) plays an irreplaceable role in lung disease, and the pulmonary manifestations can be used to evaluate the patient’s condition for PIDs and PTB (11). Some studies have shown that there are differences in PTB between immunocompromised and immunocompetent patients, such as the distribution and pattern of lesions (12-14).
Radiomics is a high-throughput method for extracting a large number of imaging features, transforming traditional radiological images into high-dimensional data that can be objectively and quantitatively analyzed. This approach provides more objective information beyond visual evaluations and can establish predictive models through artificial intelligence methods to assist clinical decision-making (15,16). Radiomics is an emerging technology that has been widely used in oncology research (17,18). In recent years, researchers have applied radiomics to non-tumor fields, such as the differential diagnosis and prediction of pulmonary diseases like COVID-19 and childhood infectious pneumonia (19-21). However, the potential value of radiomics in identifying immunodeficiency status in children with PTB remains unclear.
This study aimed to use radiomics analysis based on un-enhanced chest CT to identify immunodeficiency status in children with PTB, in order to detect immune function status early and provide support for clinical decision-making. The results of this study will help to personalize treatment for children with PTB. We present this article in accordance with the TRIPOD reporting checklist (available at https://tp.amegroups.com/article/view/10.21037/tp-23-309/rc).
Methods
Patients
This study was conducted in accordance with the Declaration of Helsinki (as revised in 2013). This retrospective study was approved by the Institutional Review Board of Children’s Hospital of Chongqing Medical University for using the data (Approval number: 20230171), and the individual written informed consent for this retrospective analysis was waived. We retrospectively enrolled the children with the tested immunity status for PTB who visited our hospital from January 2015 to December 2022, and the chest CT data before treatment of all the children were collected. The patient recruitment procedure is shown as Figure 1.
Experiment grouping
We randomly divided the included patients into the training set (129 cases, no-PIDs: PIDs =75:54) and the test set (44 cases, no-PIDs: PIDs =26:18) at a ratio of 3:1. Due to the limited sample size, we conducted model training with 5-fold cross-validation in the training set and internal validation. Ultimately, the optimal model was selected and tested independently in the test sets of 44 cases.
CT examination
All patients had an un-enhanced chest CT scan before treatment. The CT scan was performed using a 64-detector CT scanner (GE Lightspeed VCT 64, Philips Medical Systems, Amsterdam, the Netherlands) or a 128-detector CT scanner (Brilliance ICT, Philips Medical Systems). The CT acquisition parameters were as follows: tube voltage of 100 Kv, the tube current being automatically regulated, pitch of 0.984:1, slice thickness of 5.0 mm, and slice interval of 5.0 mm. The scans ranged from the apex to the bottom of the lung.
Image segmentation
For CT image segmentation, we utilized Digital Imaging and Communications in Medicine (DICOM) images obtained from the picture archiving and communication system (PACS). The region of interest (ROI) for pulmonary lesions was manually delineated using ITK-SNAP software version 3.4.0, by a pediatric radiologist with 5 years of experience. The ROI for each slice, encompassing the entire lesion, was meticulously marked along the edge of the lesion in an axial lung window of un-enhanced CT image. Subsequently, the segmentation was validated by a senior radiologist with 12 years of experience (Figure 2). As the ROI was manually delineated, there were certain subjective differences in the interpretation of the lesion. Therefore, to ensure the availability of radiomics features, we randomly selected 51 patients (no-PIDs: PIDs =31:20) to re-delineate the ROI, and calculated the intraclass correlation coefficients (ICCs) of all radiomics features extracted from the twice-delineated ROI in the 51 patients (22).
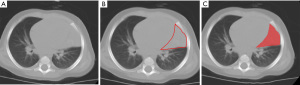
Radiomics feature extraction and selection
Radiomics feature extraction was achieved through the uAI research platform (uRP) (United Imaging, China). Filters provided by the uRP platforms included log, mean, normalize, binomialblurimage, boxmean, boxsigmaimage, curvatureflow, laplaciansharpening, discretegaussian, recursivegaussian, shotnoise, specklenoise, additivegaussiannoise and wavelet.
For all radiomics features extracted, Z-score normalization method was performed to eliminate the dimensional influence between the different features (23-25). For the selection of features, the variance threshold method was first used to eliminate the features with variance below 0.80. Then, the SelectkBest method was used to calculate the P-value and score of each feature. The top 100 selected features were imported into the Least absolute shrinkage and selection operator (Lasso) algorithm to select the most important features for identify the immune function of patients with TB. The hyperparameters including the alpha value, were determined automatically by 5-fold cross-validation, sought and determine the optimal alpha value was automatically.
Radiomics model construction
Using the Logistic regression algorithm, we constructed the radiomics model to identify the immune function status of patients with TB by analyzing the linear combination relationship of the selected features and the weighting coefficient. These features were then used to reflect the immune function status of the patients. The diagnostic performance of the radiomics model was quantified by calculating the area under the receiver operating characteristic curve (AUC) in both the training and test sets.
Radiomics model evaluation and validation
The discriminative efficacy of the model was assessed using the receiver operating characteristic (ROC) curves and calibration curves in the training and test sets. The clinical application value of the model was evaluated through decision curve analysis (DCA) in the training and test sets. DCA was used to assess the patient benefit impact of radiomics models, thereby assisting clinicians in making treatment decisions. The calibration curve was used to evaluate the predictive performance of the radiomics model. The calibration curve reflected how well the model distinguished primary immune deficiency consistent with the actual occurrence. The calibration curve that was closer to the diagonal indicated that the model’s ability to identify PID with PTB was closer to the true values and with a higher accuracy.
Statistical analysis
All statistical analyses were performed in software R (version 4.1.1). A P value less than 0.05 indicated statistical significance. In machine learning, the sensitivity and specificity of the radiomics features were calculated, and the ROC curve was analyzed and the AUC was calculated, which proved the discriminatory ability of the radiomics model. The performance of the classifier was evaluated using the following indicators in this study, including sensitivity, specificity, accuracy, precision, and F1 score.
Results
Patient characteristics
A total of 72 patients with PIDs and 101 patients with no-PIDs satisfied the inclusion criteria and were included in the study. There were 29 male patients and 43 female patients in the PIDs groups, the mean age was 2.85±3.09 years, and an age range of 3 months to 14 years. The no-PIDs groups included 46 male patients and 55 female patients with a mean age of 3.96±4.85 years and an age range of 4 months to 13 years.
Feature extraction and selection
For each ROI, 2,264 radiomics features were extracted, including 104 original radiomics features and 2,160 filtered radiomics features, which could be divided into three categories: first order [18], shape [14], and texture [72] features. Texture features included 21 gray level co-occurrence matrix feature (GLCM), 14 gray level dependence matrix feature (GLDM), 16 gray level run length matrix feature (GLRLM), 16 gray level size zone matrix features (GLSZM) and 5 neighborhood gray tone difference matrix feature (NGTDM).
After ICC analysis, 188 features with ICC values below 0.85 were discarded, accounting for 8.30% of the total features, and 2,076 features were remaining. The ICCs distribution of the 2,264 features is shown in Figure 3. Features with an ICCs greater than 0.85 were selected and entered into the subsequent analysis.
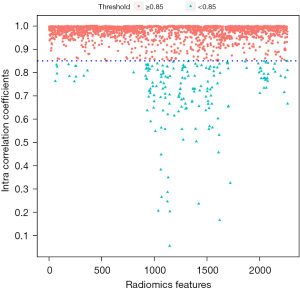
For identifying the immune function status of children with PTB, we finally selected three first-order features, four GLCM features, one GLSZM feature, and one GLRLM feature. These features are shown in Table 1.
Table 1
Filter | Type | Radiomics features | Coefficients |
---|---|---|---|
Laplaciansharpening | glcm | Differencevariance | 0.644 |
Recursivegaussian | firstorder | Range | 0.322 |
Laplaciansharpening | glcm | Clustertendency | 0.220 |
Shotnoise | glrlm | Shortrunlowgraylevelemphasis | 0.201 |
Discretegaussian | firstorder | Range | −0.240 |
Discretegaussian | glcm | Differencevariance | −0.267 |
Laplaciansharpening | glszm | Graylevelvariance | −0.278 |
Laplaciansharpening | glcm | Contrast | −0.296 |
Boxsigmaimage | firstorder | Totalenergy | −0.408 |
Model building and evaluating
A logical regression model was used to classify the immune function status of children with PTB, and the classification threshold of the model was set to 0.5. After dimensional reduction, nine optimal features, including three original first-order feature and four GLCM and one GLRLM, were identified and selected to construct the radiomics models. In the training set the AUC of the constructed prediction model was 0.837, sensitivity was 0.783, specificity was 0.780, and accuracy was 0.719. In the validation set the AUC was 0.847, sensitivity was 0.834, specificity was 0.720, and accuracy was 0.680. And in the test set the AUC was 0.746, sensitivity was 0.722, specificity was 0.692, and accuracy was 0.813. The results are shown in detail in Table 2. Besides, the ROC curves and the nomogram of the models are shown as Figure 4 and Figure 5, respectively.
Table 2
Fold | AUC | Sensitivity | Specificity | Accuracy | F1-score | Precision | |||||||||||
---|---|---|---|---|---|---|---|---|---|---|---|---|---|---|---|---|---|
Train | Validation | Train | Validation | Train | Validation | Train | Validation | Train | Validation | Train | Validation | ||||||
Fold_1 | 0.828 (0.748–0.908) | 0.818 (0.654–0.983) | 0.791 | 0.818 | 0.783 | 0.733 | 0.786 | 0.769 | 0.756 | 0.750 | 0.723 | 0.692 | |||||
Fold_2 | 0.813 (0.729–0.897) | 0.915 (0.782–1.000) | 0.698 | 1.000 | 0.800 | 0.800 | 0.757 | 0.885 | 0.706 | 0.880 | 0.714 | 0.786 | |||||
Fold_3 | 0.866 (0.794–0.937) | 0.648 (0.423–0.874) | 0.837 | 0.727 | 0.800 | 0.667 | 0.816 | 0.692 | 0.791 | 0.667 | 0.750 | 0.615 | |||||
Fold_4 | 0.864 (0.794–0.933) | 0.642 (0.409–0.876) | 0.837 | 0.727 | 0.767 | 0.667 | 0.796 | 0.692 | 0.774 | 0.667 | 0.720 | 0.615 | |||||
Fold_5 | 0.817 (0.734–0.900) | 0.847 (0.691–1.000) | 0.750 | 0.900 | 0.750 | 0.733 | 0.750 | 0.800 | 0.717 | 0.783 | 0.688 | 0.692 | |||||
Mean | 0.838 (0.760–0.915) | 0.774 (0.592–0.945) | 0.783 | 0.834 | 0.780 | 0.720 | 0.781 | 0.768 | 0.749 | 0.749 | 0.719 | 0.68 | |||||
Test | 0.746 | 0.722 | 0.692 | 0.705 | 0.823 | 0.813 |
PIDs, primary immunodeficiency diseases; PTB, pulmonary tuberculosis; AUC, area under the ROC curve; ROC, the receiver operating characteristic.
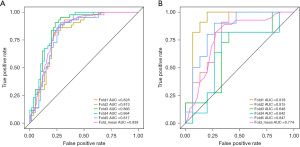
The results of the logistic regression are shown in Table 3, and the column of Coefficients represents the weight coefficients of each feature. The P value of the Hosmer-Lemeshow test (HL test) for the logistic regression model was 0.1247, which prove that there is no significant difference between the predicted value and the true value, and further indicating that the degree of fitting model is well. Only two features, the discretegaussian_firstorder_range and discretegaussian_glcm_difference variance, had P values greater than 0.05 and were not used as independent predictors. The various characteristics were significantly correlated with the immune function status of the child with PTB (P<0.05) (Table 4).
Table 3
Features | Coefficients | Stand error | Z value | P value |
---|---|---|---|---|
Intercept | −20.345 | 8.711 | −2.340 | 0.020 |
Boxsigmaimage_firstorder_totalenergy | −2.282 | 0.805 | −2.830 | 0.005 |
Laplaciansharpening_glcm_clustertendency | 2.702 | 1.023 | 2.640 | 0.008 |
Laplaciansharpening_glcm_contrast | −7.092 | 2.208 | −3.210 | 0.001 |
Laplaciansharpening_glcm_differencevariance | 6.541 | 2.207 | 2.960 | 0.003 |
Laplaciansharpening_glszm_graylevelvariance | −3.493 | 1.258 | −2.780 | 0.006 |
Discretegaussian_firstorder_range | −4.806 | 3.060 | −1.570 | 0.116 |
Discretegaussian_glcm_difference variance | −0.088 | 0.112 | −0.780 | 0.436 |
Recursivegaussian_firstorder_range | 0.020 | 0.010 | 2.020 | 0.043 |
Shotnoise_glrlm_shortrunlowgraylevelemphasis | 11.146 | 4.086 | 2.730 | 0.006 |
Table 4
Features | r | P value |
---|---|---|
Boxsigmaimage_firstorder_total energy | −0.351 | <0.001 |
Discretegaussian_firstorder_range | −0.329 | <0.001 |
Recursivegaussian_firstorder_range | −0.302 | 0.001 |
Laplaciansharpening_glcm_cluster tendency | −0.342 | <0.001 |
Laplaciansharpening_glcm_contrast | −0.312 | <0.001 |
Laplaciansharpening_glcm_difference variance | −0.315 | <0.001 |
Discretegaussian_glcm_difference variance | −0.345 | <0.001 |
Laplaciansharpening_glszm_gray level variance | −0.388 | <0.001 |
Shotnoise_glrlm_short run low gray level emphasis | 0.316 | <0.001 |
Clinical value analysis
The calibration curves indicated a good consistency of the model compared with the true value. There was no significant difference between probabilities from the predicted model and the actual scenario. DCA demonstrated that the model can generate net benefit at different risk thresholds, indicating there is clinical value and would benefit the patients (Figure 6).
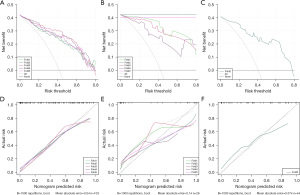
Discussion
As far as we know, no previous studies have utilized radiomics to analyze the immune status of children with TB with either PIDs or no-PIDs. Our study, however, has revealed that a radiomics model can effectively Identify the primary immune function in these children. Notably, this model creatively employs CT images to assess immune function, and is based on routine CT scans that are easily applicable in clinical practice. Therefore, this radiomics model has promising potential as a tool to distinguish between the presence or absence of PIDs in children diagnosed with PTB.
Following Mtb infection, the human body produces an anti-TB immune response that is mainly characterized by cellular immunity and tissue necrosis caused by allergic injury. This often leads to the onset of TB and lung involvement (26). The body’s anti-TB response relies heavily on the complete IL-12/23-IFN-γ pathway, which requires the participation of phagocytes, T lymphocytes, NK cells, and other immune cells, as well as various cytokines (4,27). The immune status of patients, both congenital and acquired, is closely linked to this process (12,27,28). Considered that children with PIDs are particularly susceptible to multiple pathogens, including Mtb, and that pulmonary complications significantly impact prognosis, it is important to raise awareness of PIDs in these patients (29-31).
Thoracic imaging, particularly CT, is critical for both clinical diagnosis and treatment evaluation in children infected with Mtb (11,32). Typical pulmonary imaging findings associated with TB include consolidation, necrotic cavities, centrilobular nodules, calcifications, and caseous necrosis (11,32). However, due to the imbalanced residual immune levels in children with PIDs, the immune response to Mtb can vary, resulting in differences in the location, nature, morphology, and distribution range of the corresponding lesions (13,32,33). Moreover, these lesions can overlap or even mask one another, leading to complex pulmonary imaging findings (34). Since radiologists are influenced by individual factors, the non-specific morphological parameters in chest CT images of Mtb-infected children are often difficult to identify by conventional imaging features, making it a challenge to effectively assess the immune function status of children with PTB.
The field of radioimmunology has demonstrated the potential ability using machine Learning methods to capture useful information and to improve the accuracy of clinical differential diagnosis (20). In this study, we constructed a predicted model and screened the characteristic values of 2,264 radiomics features by Lasso dimensionality reduction method, and finally screened out nine features, including three first-order features, four GLCM feature, a GLSZM feature and a GLRLM feature. These features, which were significantly different between PIDs and no-PIDs in children with TB, are important radiomics features in the two kinds of immune states, but the exact clinical interpretation remains uncertain. The first-order features related to the gray-level frequency within the ROI were obtained from the voxel intensity histogram, reflecting the overall information of the intensity histogram. Cluster tendency, contrast and difference variance belong to the GLCM group, and these features usually capture texture variation to quantify the spatial relationship of image voxels according to previous researches (35).
In previous researches of cancer, the texture features were proven to reflect the image heterogeneity of the tumor (13,36), and thus indicated the genetic heterogeneity and invasiveness of the tumor. In our study, although the extent, shape, or grayscale of infectious lesions may be consistent, there may be differences in microstructure of lesion due to differences in immune responses. Therefore, we speculate that CT image heterogeneity of intrapulmonary lesions varies between PIDs and no-PIDs in children with PTB.
In this study, the radiomics model constructed has a balanced sensitivity (0.783) and specificity (0.780). The performance of the current model was moderate in the test set which the sensitivity was 0.722 and the specificity was 0.692. Besides, the value of F1-score in the test set was expected which indicated a high accuracy of the model. Considering that the sample size of the current applied is small, and the sample size will be expanded to continue the research later. Although the diagnostic accuracy of this radiomics model is not high enough, the model can effectively identify the immune function status of children with PTB though conventional CT images, which is not easily possible by radiologists. At the same time, in order to increase the reproducibility of the study, ICCs was used to evaluate the consistency of radiomics features, which effectively improved the consistency of features within and between groups, and reduced the impact of subjective differences due to the delineator discriminated the CT image (22).
Moreover, this study not only used the ROC curve, but also used the calibration curve to evaluate the discriminant performance of model. This study also used a DCA that can estimate whether patients benefit or not to evaluate the clinical application value of the model, which increases the credibility of the model.
However, there are some limitations with this study. This is a retrospective study with an inevitable selective bias. It is a small sample size and a single center study. In order to improve the effectiveness of the model and the credibility of the study, a large sample and multicenter study will be followed. Moreover, this study did not include the clinical indicators, such as the clinical test indicator for assessing immune function status. Subsequently, the clinical indicators will be combined to further improve the efficacy of the radiomics model.
Conclusions
Radiomics is a cutting-edge, non-invasive tool for identifying immune function status. By combining radiomics features to construct models, it has demonstrated a strong ability to identify pulmonary lesions caused by Mtb infection in children with the presence or absence of PIDs, and has shown promise in evaluating the immune function status of children with PTB. These findings have the potential to enhance clinical applications and drive the advancement of precision medicine. As radiomics continues to evolve, it is expected to become a valuable diagnostic supportive tool for PIDs, particularly in high-burden TB-endemic regions where genetic testing for PIDs is limited.
Acknowledgments
Funding: This study was supported by
Footnote
Reporting Checklist: The authors have completed the TRIPOD reporting checklist. Available at https://tp.amegroups.com/article/view/10.21037/tp-23-309/rc
Data Sharing Statement: Available at https://tp.amegroups.com/article/view/10.21037/tp-23-309/dss
Peer Review File: Available at https://tp.amegroups.com/article/view/10.21037/tp-23-309/prf
Conflicts of Interest: All authors have completed the ICMJE uniform disclosure form (available at https://tp.amegroups.com/article/view/10.21037/tp-23-309/coif). F.W. is an employee of Shanghai United Imaging Intelligence Co., Ltd, a for-profit company, during the conduct of the study. The other authors have no conflicts of interest to declare.
Ethical Statement: The authors are accountable for all aspects of the work in ensuring that questions related to the accuracy or integrity of any part of the work are appropriately investigated and resolved. This study was conducted in accordance with the Declaration of Helsinki (as revised in 2013). This retrospective study was approved by the Institutional Review Board of Children’s Hospital of Chongqing Medical University for using the data (Approval number: 20230171), and the individual written informed consent for this retrospective analysis was waived.
Open Access Statement: This is an Open Access article distributed in accordance with the Creative Commons Attribution-NonCommercial-NoDerivs 4.0 International License (CC BY-NC-ND 4.0), which permits the non-commercial replication and distribution of the article with the strict proviso that no changes or edits are made and the original work is properly cited (including links to both the formal publication through the relevant DOI and the license). See: https://creativecommons.org/licenses/by-nc-nd/4.0/.
References
- Global Tuberculosis Report 2022[website]. Geneva: World Health Organization. 2022. Available online: https://www.who.int/publications/i/item/9789240061729
- Bousfiha A, Moundir A, Tangye SG, et al. The 2022 Update of IUIS Phenotypical Classification for Human Inborn Errors of Immunity. J Clin Immunol 2022;42:1508-20. [Crossref] [PubMed]
- Singh A, Jindal AK, Joshi V, et al. An updated review on phenocopies of primary immunodeficiency diseases. Genes Dis 2020;7:12-25. [Crossref] [PubMed]
- Boisson-Dupuis S, Bustamante J, El-Baghdadi J, et al. Inherited and acquired immunodeficiencies underlying tuberculosis in childhood. Immunol Rev 2015;264:103-20. [Crossref] [PubMed]
- Ulusoy E, Karaca NE, Aksu G, et al. Frequency of Mycobacterium bovis and mycobacteria in primary immunodeficiencies. Turk Pediatri Ars 2017;52:138-44. [Crossref] [PubMed]
- Glanzmann B, Uren C, de Villiers N, et al. Primary immunodeficiency diseases in a tuberculosis endemic region: challenges and opportunities. Genes Immun 2019;20:447-54. [Crossref] [PubMed]
- Fekrvand S, Yazdani R, Olbrich P, et al. Primary Immunodeficiency Diseases and Bacillus Calmette-Guérin (BCG)-Vaccine-Derived Complications: A Systematic Review. J Allergy Clin Immunol Pract 2020;8:1371-86. [Crossref] [PubMed]
- Ying W, Sun J, Liu D, et al. Clinical characteristics and immunogenetics of BCGosis/BCGitis in Chinese children: a 6 year follow-up study. PLoS One 2014;9:e94485. [Crossref] [PubMed]
- Yazdani R, Abolhassani H, Asgardoon MH, et al. Infectious and Noninfectious Pulmonary Complications in Patients With Primary Immunodeficiency Disorders. J Investig Allergol Clin Immunol 2017;27:213-24. [Crossref] [PubMed]
- Reisi M, Azizi G, Kiaee F, et al. Evaluation of pulmonary complications in patients with primary immunodeficiency disorders. Eur Ann Allergy Clin Immunol 2017;49:122-8.
- Dos Santos TCS, Setúbal S, Dos Santos AASMD, et al. Radiological aspects in computed tomography as determinants in the diagnosis of pulmonary tuberculosis in immunocompetent infants. Radiol Bras 2019;52:71-7. [Crossref] [PubMed]
- Mathur M, Badhan RK, Kumari S, et al. Radiological Manifestations of Pulmonary Tuberculosis - A Comparative Study between Immunocompromised and Immunocompetent Patients. J Clin Diagn Res 2017;11:TC06-9. [Crossref] [PubMed]
- Yao Q, Zhou QH, Shen QL, et al. Imaging characteristics of pulmonary BCG/TB infection in patients with chronic granulomatous disease. Sci Rep 2022;12:11765. [Crossref] [PubMed]
- Nacaroğlu HT, Bahçeci Erdem S, Gülez N, et al. Tuberculosis masked by immunodeficiency: a review of two cases diagnosed with chronic granulomatous disease. Tuberk Toraks 2017;65:56-9.
- Lambin P, Leijenaar RTH, Deist TM, et al. Radiomics: the bridge between medical imaging and personalized medicine. Nat Rev Clin Oncol 2017;14:749-62. [Crossref] [PubMed]
- Avanzo M, Wei L, Stancanello J, et al. Machine and deep learning methods for radiomics. Med Phys 2020;47:e185-202. [Crossref] [PubMed]
- Liu Z, Wang S, Dong D, et al. The Applications of Radiomics in Precision Diagnosis and Treatment of Oncology: Opportunities and Challenges. Theranostics 2019;9:1303-22. [Crossref] [PubMed]
- Lambin P, Rios-Velazquez E, Leijenaar R, et al. Radiomics: extracting more information from medical images using advanced feature analysis. Eur J Cancer 2012;48:441-6. [Crossref] [PubMed]
- Yang Y, Lure FYM, Miao H, et al. Using artificial intelligence to assist radiologists in distinguishing COVID-19 from other pulmonary infections. J Xray Sci Technol 2021;29:1-17. [Crossref] [PubMed]
- Wang B, Li M, Ma H, et al. Computed tomography-based predictive nomogram for differentiating primary progressive pulmonary tuberculosis from community-acquired pneumonia in children. BMC Med Imaging 2019;19:63. [Crossref] [PubMed]
- Chen X, Li W, Wang F, et al. Early recognition of necrotizing pneumonia in children based on non-contrast-enhanced computed tomography radiomics signatures. Transl Pediatr 2021;10:1542-51. [Crossref] [PubMed]
- Fornacon-Wood I, Mistry H, Ackermann CJ, et al. Reliability and prognostic value of radiomic features are highly dependent on choice of feature extraction platform. Eur Radiol 2020;30:6241-50. [Crossref] [PubMed]
- Lee H, Troschel FM, Tajmir S, et al. Pixel-Level Deep Segmentation: Artificial Intelligence Quantifies Muscle on Computed Tomography for Body Morphometric Analysis. J Digit Imaging 2017;30:487-98. [Crossref] [PubMed]
- Masoudi S, Harmon SA, Mehralivand S, et al. Quick guide on radiology image pre-processing for deep learning applications in prostate cancer research. J Med Imaging (Bellingham) 2021;8:010901. [Crossref] [PubMed]
- Huo Y, Tang Y, Chen Y, et al. Stochastic tissue window normalization of deep learning on computed tomography. J Med Imaging (Bellingham) 2019;6:044005. [Crossref] [PubMed]
- Jaganath D, Beaudry J, Salazar-Austin N. Tuberculosis in Children. Infect Dis Clin North Am 2022;36:49-71. [Crossref] [PubMed]
- Lee WI, Huang JL, Yeh KW, et al. Immune defects in active mycobacterial diseases in patients with primary immunodeficiency diseases (PIDs). J Formos Med Assoc 2011;110:750-8. [Crossref] [PubMed]
- Venturini E, Turkova A, Chiappini E, et al. Tuberculosis and HIV co-infection in children. BMC Infect Dis 2014;14:S5. [Crossref] [PubMed]
- Kawai T, Watanabe N, Yokoyama M, et al. Interstitial lung disease with multiple microgranulomas in chronic granulomatous disease. J Clin Immunol 2014;34:933-40. [Crossref] [PubMed]
- Jesenak M, Banovcin P, Jesenakova B, et al. Pulmonary manifestations of primary immunodeficiency disorders in children. Front Pediatr 2014;2:77. [Crossref] [PubMed]
- Membrila-Mondragón J, Staines-Boone AT, Sánchez-Sánchez LM, et al. Pulmonary complications in pediatric patients with primary immunodeficiency. Gac Med Mex 2015;151:157-63.
- Buonsenso D, Pata D, Visconti E, et al. Chest CT Scan for the Diagnosis of Pediatric Pulmonary TB: Radiological Findings and Its Diagnostic Significance. Front Pediatr 2021;9:583197. [Crossref] [PubMed]
- Doğru D, Kiper N, Ozçelik U, et al. Tuberculosis in children with congenital immunodeficiency syndromes. Tuberk Toraks 2010;58:59-63.
- Krishnan VP, Taur P, Pandrowala A, et al. X-Linked Hyper IgM Syndrome Presenting with Recurrent Tuberculosis-a Case Report. J Clin Immunol 2020;40:531-3. [Crossref] [PubMed]
- Rios Velazquez E, Parmar C, Liu Y, et al. Somatic Mutations Drive Distinct Imaging Phenotypes in Lung Cancer. Cancer Res 2017;77:3922-30. [Crossref] [PubMed]
- Bi WL, Hosny A, Schabath MB, et al. Artificial intelligence in cancer imaging: Clinical challenges and applications. CA Cancer J Clin 2019;69:127-57. [Crossref] [PubMed]