Development and validation of a novel stemness-related prognostic model for neuroblastoma using integrated machine learning and bioinformatics analyses
Highlight box
Key findings
• The newly developed neuroblastoma (NB) stemness-related prognostic signature in this study could facilitate the prognostic prediction and immune responses.
What is known and what is new?
• NB tumors with poor prognosis harbor an undifferentiated stem cell population that is responsible for their highly aggressive nature.
• By utilizing the stemness index and machine learning methods, our study delved into the features of cancer stem cells in NB, further providing evidence of their essential role in the development and progression of the disease. For the first time, we constructed a novel mRNAsi-associated signature for NB that exhibited significant associations with prognosis, clinical characteristics, and tumor immune response in NB.
What is the implication, and what should change now?
• The identification of hub genes in this study significantly advances our understanding of NB stem cell maintenance and suggests that they may serve as promising therapeutic targets for inhibiting NB stemness characteristics.
Introduction
Cancers in children and young adults are relatively uncommon and have unique diagnostic features compared to those affecting older individuals (1). These malignancies often originate from immature cells that possess stem cell-like properties, potentially contributing to the observed clinical and biological variations (2). Neuroblastoma (NB) is currently the most prevalent extracranial solid tumor in childhood, with the vast majority of cases (90%) being identified in children under the age of 5 years (3). The clinical prognosis of NB is highly variable, with patients displaying a broad range of outcomes from complete remission to refractory disease that is challenging to treat (3). Despite intensive efforts to improve outcomes, current treatments still encounter high rates of resistance, recurrence, and progression in high-risk NB cases, with long-term survival still less than 40% (4). Previous studies on NB have identified several prognostic biomarkers, such as MYCN amplification, ALK mutations, and age at diagnosis, among others (5,6). While these markers are informative, their predictive power is often limited by NB’s extensive biological diversity, which stems from its complex genetic and epigenetic landscape. Therefore, finding a robust index to predict and evaluate clinical prognosis and therapeutic efficacy has become an urgent issue, with the goal of achieving accurate clinical interventions.
Many investigations have shown that in various types of tumors, a minute fraction of undifferentiated cells, resembling stem cells, have the capability to initiate the development of cancer. These cells have been designated as cancer stem cells (CSCs) (7). CSCs are believed to play a critical role in the growth, progression, recurrence, and resistance to therapy of solid malignancies (8). These uncommon and difficult-to-detect cells have the ability to spread at an early stage and conceal themselves in specific niches located in remote organs. This makes them capable of causing disease relapse even after successful treatment of the primary tumor (9). It has been demonstrated that NB tumors with poor prognosis harbor an undifferentiated stem cell population that is responsible for their highly aggressive nature (10-12). Pandian et al. successfully isolated highly aggressive cellular clones from metastatic NB tumors, and these clones were found to retain their plasticity and adaptive stemness despite exposure to different culture conditions, indicating their potential as NB CSCs (13). Drug resistance in treatment is a major challenge in the management of refractory cancers. It is widely accepted that CSCs play a significant role in the development of therapy resistance in tumors (14-16). During prolonged drug selection of NB cells, a subpopulation of cells with CSC characteristics becomes enriched (17). Despite the evidence that the CSC subpopulation in NB contributes to disease metastases and therapy resistance, the origin and characteristics of this cell subset are still not fully understood (13,18,19). The heterogeneity of CSC populations within NB tumors contributes to the variability in patient responses to treatment, necessitating the exploration of new biomarkers that can encompass this diversity. Currently, there are no established therapeutic modalities specifically targeting CSCs, scientists have adopted artificial intelligence (AI) to further examine the characteristics of CSCs within tumors, in order to obtain a more comprehensive understanding of these cells. In a study by Malta et al., a novel messenger RNA (mRNA) expression-based stemness index (mRNAsi) was generated by machine learning algorithm to quantify tumor stemness (20). By quantifying tumor stemness, mRNAsi may offer a more comprehensive biomarker for NB prognosis. Stemness attributes, reflective of a tumor’s ability to sustain growth, resist therapy, and metastasize, are particularly pertinent to the aggressive and often treatment-resistant nature of high-risk NB. Prognostic signatures based on mRNAsi have been successfully developed in various types of cancer, indicating the stemness of CSCs and predicting unfavorable outcomes (21-23). Despite this, few studies have sought to determine the predictive and prognostic value of mRNAsi-related genes in NB.
Our study proposes mRNAsi as a promising tool for prognostic assessment, with the potential to enhance the precision of clinical interventions in NB. We utilized the mRNAsi in a comprehensive bioinformatics analysis to identify genes associated with stemness, with the aim of discovering potential therapeutic targets and developing a prognostic signature for NB. The mRNAsi of NB patients was evaluated and a risk model was developed using the four hub genes that we identified. A comprehensive evaluation of prognosis, clinical characteristics, tumor microenvironment (TME), and response to immunotherapy was conducted across different subtypes. Our findings offer novel insights into CSC research in NB and have the potential to improve individualized treatments for NB patients. We present this article in accordance with the TRIPOD reporting checklist (available at https://tp.amegroups.com/article/view/10.21037/tp-23-582/rc).
Methods
Data acquisition
The microarray data were downloaded from the Gene Expression Omnibus (GEO) database (24). The gene expression profiles and clinical information of 498 NB patients were obtained from the dataset GSE49710, which is hosted on the GEO. Overall survival (OS) is defined as the duration from the date of diagnosis or initiation of treatment to either the date of death from any cause or the last follow-up. The probes were mapped to gene symbols using the platform GPL16876’s gene mapping information. We obtained the E-MTAB-8248 dataset (N=223) from the ArrayExpress database (https://www.ebi.ac.uk/biostudies/arrayexpress) to externally validate our signature. Table S1 provides a detailed illustration of patient information derived from two datasets. The study was conducted in accordance with the Declaration of Helsinki (as revised in 2013).
Calculation of stemness index
By using the one-class logistic regression (OCLR) machine learning (ML) algorithm, we calculated the mRNAsi of all 498 samples to represent their stemness values (20). To normalize the mRNAsi values to a range of 0 to 1, we employed a linear transformation technique, which involved subtracting the minimum value from each data point and then dividing the result by the range, defined as the maximum value minus the minimum value. Patients were stratified into two groups based on the median value of mRNAsi. The prognostic significance of mRNAsi was evaluated using Kaplan-Meier (KM) analysis for OS difference between the patient groups. Multivariate Cox regression was employed to validate the prognostic independence of mRNAsi.
Analysis of enriched gene sets
We employed gene set enrichment analysis (GSEA) to investigate the differences in functional pathways between the two mRNAsi groups. The dataset was obtained from the Molecular Signatures Database (MSigDB). The latest MSigDB consists of nine categories (C1–C8 and Hallmark) (25). The R package “clusterProfiler” was used for analysis (26).
Construction and verification of a four-gene signature
The 498 samples of GSE49710 were used for signature development and 223 samples of E-MTAB-8248 for model validation. The R package “limma” was used to identify differentially expressed genes (DEGs) (27). Cox analysis and least absolute shrinkage and selection operator (LASSO) regression analysis were used to further screen DEGs. Ultimately, the risk score for the prognostic gene signature was derived by multiplying the mRNA expression level with the regression coefficient assigned to each gene. Then, two patient risk groups were built according to the median score. The assessment of the predictive performance of the model was conducted by KM analysis, decision curve analysis (DCA), and area under the curve (AUC) of the receiver operating characteristic (ROC) curve. We adopted AUC threshold values of >0.7 for acceptable, >0.8 for excellent, and >0.9 for outstanding predictive performance.
Analysis of immune microenvironment and prediction of therapeutic sensitivity
To evaluate the degree of immune infiltration, four algorithms were used, including Estimation of STromal and Immune cells in MAlignant Tumours using Expression data (ESTIMATE) (28), Cell-type Identification By Estimating Relative Subsets Of RNA Transcripts (CIBERSORT) (4), Microenvironment Cell Populations-counter (MCPcounter) (29), and xCell (30). The activity of 14 cancer-related pathways was analyzed by the “PROGENy” R package (31). In order to assess the potential immunotherapy response, we used the Tumor Immune Dysfunction and Exclusion (TIDE, http://tide.dfci.harvard.edu/) (32) and immunophenoscore (IPS, https://tcia.at/tools/toolsMain) (33) algorithms. A high IPS score and low TIDE score may suggest a more favorable response to immunotherapy. From the database of Genomics of Drug Sensitivity in Cancer (GDSC, https://www.cancerrxgene.org/) (34), we obtained the anti-cancer drugs information. To determine the IC50 of drugs, we employed the “pRRophetic” R package (35).
Predictive nomogram development and validation
A nomogram plot incorporating the risk score of the four-gene model and the International Neuroblastoma Staging System (INSS) stage was built using the “rms” R package. The predictive ability of the nomogram was assessed by analyzing the ROC curve and the calibration plot. The global and individual Schoenfeld tests were used to estimate the time-varying covariance of the Cox proportional risk regression analysis hypothesis. We assumed that Schoenfeld residuals were time independent. A P value <0.05 indicated rejection of this assumption.
Statistical methods
R software (version 4.2.1; The R Foundation for Statistical Computing, Vienna, Austria) was employed for statistical analysis and data visualization. We used two-tailed unpaired Student’s t-test and Wilcoxon test to compare differences between two groups. The differences between rates were tested using either the chi-square test or Fisher’s exact test. To generate images, we used Adobe Illustrator (version 22.0; Adobe, San Jose, CA, USA). In this study, a two-sided P values less than 0.05 were considered as statistically significant.
Results
The relationship between clinical features and mRNAsi in NB
Following the computation with the OCLR algorithm, the mRNAsi values of 498 samples were subsequently arranged in ascending order. The correlation between clinical characteristics and mRNAsi was assessed and is visualized in Figure 1A. The analysis revealed that patients with high mRNAsi tended to exhibit clinical characteristics indicative of more aggressive disease, such as higher INSS stage and poor OS status. Notably, MYCN amplification, a well-established marker of poor prognosis in NB, was also more prevalent in this group. Additionally, these patients were more likely to be over 18 months at diagnosis, another factor associated with a less favorable outcome in NB. Statistically significant differences in mRNAsi were observed among patients with different clinical characteristics (Figure 1B-1D). Patients with INSS stage 4 were observed to have significantly higher mRNAsi levels compared to the other stages (P<0.0001), and those with MYCN amplification displayed a markedly higher mRNAsi than patients lacking amplification (P<0.0001). Furthermore, the mRNAsi levels of patients aged over 18 months at diagnosis were markedly higher than those diagnosed before 18 months (P<0.0001). In addition, patients with lower mRNAsi had significantly better survival outcomes than those with high mRNAsi (P<0.0001) (Figure 1E). The Cox regression indicated that mRNAsi could independently predict the OS of NB, in addition to INSS stage, MYCN, and age (Figure 1F).
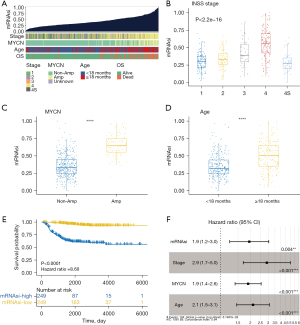
Significant survival discrepancies between different mRNAsi groups
According to the respective mRNAsi value of each patient, two groups were created: low mRNAsi and high mRNAsi. As indicated by the survival analysis, high mRNAsi patients had significantly shorter OS, regardless of their INSS stages (Figure 2A,2B), MYCN statuses (Figure 2C,2D), or ages (Figure 2E,2F). Among the 131 NB patients aged over 18 months who were also INSS stage 4, those with high mRNAsi also had a worse prognosis (P=0.0072) (Figure 2G). Similarly, in the subset of 47 patients with MYCN amplification, those categorized in the high mRNAsi group demonstrated a poorer survival outcome (P=0.047) (Figure 2H).
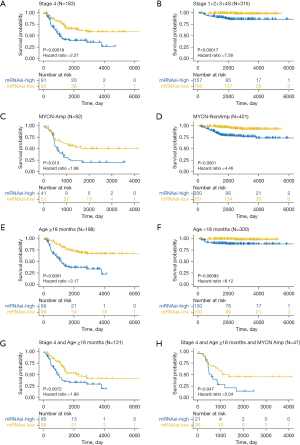
GSEA of DEGs
To investigate the function of DEGs between patients with low and high mRNAsi, we used MSigDB to conduct an enrichment analysis. The top two enrichments with the lowest P values in each of the nine categories (C1–C8 and Hallmark) are presented in Figure 3. In Figure S1A,S1B, the top 10 enrichment with the lowest P values of C5 [Gene Ontology (GO) gene sets] and H (Hallmark gene sets) were presented, respectively. The gene set on the left represents normalized enrichment score (NES) <0. As depicted in Figure 3, genes located on chr1p36 were found to be more enriched in the low mRNAsi group, suggesting a potential higher frequency of 1p36 deletion in patients with high mRNAsi. Loss of tumor suppressor genes at the 1p36 locus is crucial in the oncogenesis and progression of NB (36,37). According to GO enrichment analysis, the low mRNAsi group showed enrichment of gene sets associated with positive regulation of cell adhesion, whereas the high mRNAsi group exhibited enrichment of gene sets related to chromosome separation and nuclear division (Figure S1A). The Hallmark enrichment results showed that the gene sets related to MYC targets, DNA repair, G2/M checkpoint, and E2F targets were enriched in the high mRNAsi group (Figure S1B). These pathways related to cell division, DNA damage repair, and cell cycle are critical for the survival of CSCs.
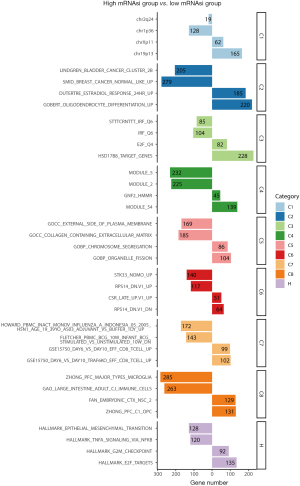
Construction of the four-gene signature
Analysis of gene expression differences revealed 20 DEGs, as shown in Figure 4A. Univariate Cox analysis demonstrated that all 20 genes were significantly associated with OS (Figure 4B). LASSO regression analysis identified seven variables as the most influential (Figure 4C,4D), and three collinear variables were excluded by multivariate Cox regression (Figure 5A). Finally, four genes (ERCC6L, DUXAP10, NCAN, DIRAS3) were included in the prediction signature, and the chordal graph displayed in Figure 5B demonstrates their close interrelation. Based on the coefficients of the four genes, the risk score was calculated by the following formula:
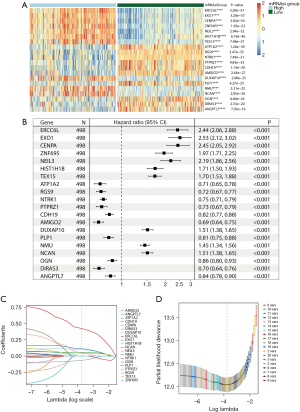
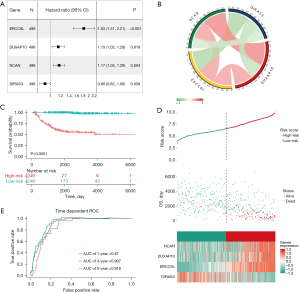
Internal and external verification of the four-gene signature
By using the median risk score (6.534) as a cutoff point, the 498 NB samples were categorized into two groups. The two groups showed significant differences in survival, with shorter OS in the high-risk score group (P<0.0001) (Figure 5C,5D). KCAN, DUXAP10, and ERCC6L were upregulated in the high-risk score group, whereas DIRAS3 was overexpressed in the low-risk score group. The ROC curve demonstrates the risk score’s predictive ability for OS, with high AUC values at different time points (Figure 5E).
The E-MTAB-8248 dataset was employed to evaluate the predictive ability of the risk score. The KM analysis revealed that, in line with the findings of the training set, the high-risk group demonstrated shorter OS (P<0.0001) (Figure S2A), highlighting significant survival differences between the two groups (Figure S2B).
Comparative analysis and correlation of the four-gene signature with other clinical indicators
The significant and positive association between high-risk scores and high mRNAsi indicated that the four-gene signature can effectively reflect the stemness of NB specimens (Figure 6A). The advanced INSS stage, MYCN amplification, and age above 18 months are established clinical factors that are highly linked with a dismal prognosis in NB (3). Figure 6B depicts the correlation between these features and the risk score for predicting the prognosis. High-risk scores were observed to be associated with clinical indicators such as INSS stage 4, MYCN amplification, age >18 months, and deceased OS status. Moreover, compared to the low mRNAsi group, all low-risk patients in the four-gene model were non-MYCN-amplified, indicating a higher accuracy of the gene signature, as illustrated in Figure 6C. It is worth mentioning that the predictive ability of the four-gene signature exceeded that of MYCN status, INSS stage, and mRNAsi. This was evident in the area under the ROC curve (Figure 6D) and the DCA curve for 5-year OS prediction (Figure 6E), both of which demonstrated the superior predictive ability of the model.
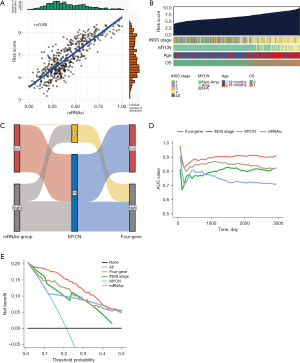
Immune infiltration and sensitivity to treatment
The low and high-risk score groups exhibited remarkable differences in immune infiltration, as calculated by the four-gene signature. Figure 7A and Table S2 provide an overview of the immune infiltration differences, as assessed by ESTIMATE, CIBERSORT, MCPcounter, and xCell. Patients identified as low risk by the ESTIMATE algorithm tend to have higher ImmuneScore, StromalScore, and ESTIMATEScore (P<0.0001). The results obtained from CIBERSORT showed that the low-risk score group had a significantly higher infiltration abundance of M2 macrophages compared to the high-risk score group (P<0.0001). The significant distinctions in the abundance of naïve B cells (P<0.01) and memory B cells (P<0.0001) also warrant attention. Naïve B cells have been reported to secrete cytokines that hinder the proliferation of cancer cells (38). Memory B cells have a significant impact on the tumor immune microenvironment by recognizing and binding to tumor-associated antigens. They initiate the production of antibodies and stimulate the immune system to attack cancer cells (38). The application of MCPcounter revealed substantial differences in 8 out of 10 cell types between the two groups (P<0.05). Among them, the significantly higher abundance of natural killer (NK) cells and cytotoxic lymphocytes in patients with low-risk score attracted our attention in particular (P<0.0001). Additionally, as per xCell, the low-risk group showed significantly higher infiltration abundance of CD4+ T cells, CD8+ T cells, and M2 macrophages (P<0.0001). Furthermore, both the TIDE score (Figure 7B) and the IPS (Figure 7C) were significantly different between the high and low-risk score groups, with the former being higher and the latter being lower in the high-risk group. These findings suggested a poor immunotherapy response in the high-risk score group. Specifically, low-risk score patients (65%) were more responsive to immunotherapy than high-risk patients (29%), as revealed by TIDE algorithms (P<0.001) (Figure S3).
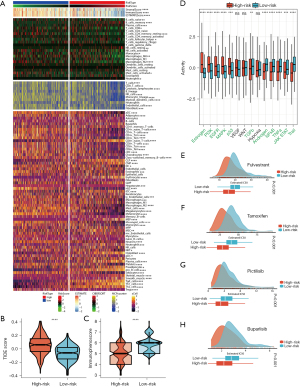
As depicted in Figure 7D, between the high- and low-risk score groups, significant differences in activity were observed in 11 out of the 14 tumor-related pathways. Specifically, the high-risk score group showed significantly higher activity in the estrogen, PI3K, EGFR, MAPK, and p53 pathways, compared to the low-risk score group. Conversely, the TGF-β, androgen, NF-κB, TNF-α, JAK-STAT, and Trail pathways exhibited higher activity in the low-risk group. Furthermore, a significant reduction in the IC50 of four anti-cancer drugs related to the estrogen and PI3K pathways was observed in the high-risk score group: fulvestrant (P<0.001) (Figure 7E), tamoxifen (P<0.001) (Figure 7F), pictilisib (P<0.001) (Figure 7G), and buparlisib (P<0.001) (Figure 7H). This indicates that these drugs are more effective for the high-risk group.
Nomogram development and validation
The nomogram was constructed to make the clinical application of the four-gene signature more feasible for prognosis prediction. The Schoenfeld tests indicated that the INSS stage and risk score did not violate the proportional risk hypothesis (P>0.05) (Figure S4). Hence, the nomogram was constructed by integrating the risk score and INSS stage (Figure 8A). The ROC curve (Figure 8B) and calibration curve (Figure 8C) demonstrate the nomogram’s predictive value. The AUC for 1-, 3-, and 5-year OS prediction in the ROC curves were 0.865, 0.915, and 0.926, respectively.
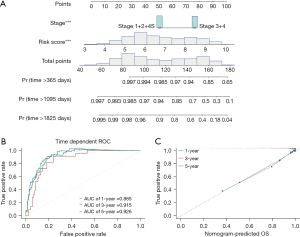
Discussion
By employing the OCLR machine-learning algorithm, we computed the mRNAsi for NB patients, which enabled us to uncover novel biological mechanisms associated with CSCs. We observed that higher mRNAsi scores were correlated with worse clinicopathological factors, such as advanced INSS stage, MYCN amplification, and older age. Our study revealed that high mRNAsi patients tended to have a worse prognosis, irrespective of their INSS stages, MYCN status, and age. Furthermore, mRNAsi was found to be an independent risk factor with strong predictive value for the survival of NB patients. These findings suggest that mRNAsi has potential as a promising prognostic indicator for NB.
Through GSEA, we observed that the low mRNAsi group had enriched genes associated with cell adhesion, whereas gene sets representing active cell division, such as chromosome segregation and nuclear division, were found to be more enriched in the high mRNAsi group.
CSCs differ from normal stem cells in their inability to regulate their mode of cell division, which results in perpetual cell division and consequent unchecked growth of tumors (39). Moreover, some CSCs have acquired specific genetic alterations that confer them with migratory abilities, enabling them to detach from adhesion with other tumor cells and further disperse and metastasize (40). Gene sets related to DNA repair and cell cycle were predominantly enriched in the high mRNAsi group. It is known that DNA repair pathways have a crucial role in maintaining genome integrity, but CSCs activate these pathways inappropriately to evade anticancer therapies (41). Stem cells are able to maintain their longevity by exiting the cell cycle for extended periods, which allows them to preserve their replicative potential and reduce DNA damage (42). Consequently, slow-cycling dormant CSCs can survive cytotoxic drugs and differentiate into highly proliferative cells that have the ability to regenerate tumors (43). Our study also demonstrated that high mRNAsi NB patients were more likely to have 1p36 deletion, which is a common segmental chromosomal loss in NB and is strongly linked to poor OS (44). In another study, it was shown that miR-200b, located at chromosome 1p36, is downregulated in hepatocellular carcinoma (HCC) and is correlated with the stemness of CSCs. Through the inhibition of ZEB1 expression, miR-200b can suppress the stemness of HCC CSCs (45).
Various prognostic signatures for NB have been reported in previous studies (12,46,47). However, to our knowledge, no previous study in NB has reported measuring stemness for prognostication. In our study, we first utilized bioinformatics methods to construct a gene prediction model for NB that is related to cancer stemness. By employing an ML approach, we identified potential hub biomarkers from mRNAsi phenotype-based DEGs. Among them, four genes (ERCC6L, DUXAP10, NCAN, and DIRAS3) were identified as promising cancer stemness-related biomarkers for NB. The four-gene signature remained an effective reflection of the NB stemness value while outperforming the mRNAsi subgrouping in terms of prognostic accuracy. These stemness-related hub genes are very likely to be crucial in NB progression and may hold promise as potential targets for treatment.
Drug resistance in treatment is a major challenge in the management of refractory cancers. It is widely accepted that CSCs play a significant role in the development of therapy resistance in tumors (14-16). During prolonged drug selection of NB cells, a subpopulation of cells with CSC characteristics becomes enriched (17). A recent study has shown that drug resistance induced by CSCs may be closely associated with immune evasion and the formation of a distinct tumor immune microenvironment (48). Therefore, targeting CSCs and enhancing the immune microenvironment represent promising strategies to overcome drug resistance and improve treatment efficacy. In recent research, mRNAsi was found to be higher in tumors with lower anti-tumor immunity, suggesting its strong association with the TME (22,23). We demonstrated through multiple algorithms that, in our stemness predictor gene model, low-risk score NB patients responded better to immunotherapy than did high-risk patients. Moreover, the distribution of immune cells between high- and low-risk score NB patients is significantly different. Typically, there is a higher infiltration abundance of CD4+ and CD8+ T-cells in the low-risk group, reflecting a better immune activity in low-risk score patients. Interestingly, we observed higher levels of M2 type macrophages in the low-risk score group, corroborating earlier research reports. In NB, M2 type macrophage has been reported to be linked with a favorable outcome, and more M2-like macrophages have been found in stage 1/2 than in stage 4S (49,50). By conducting single-cell analysis, Liu et al. observed that M2-like macrophages were the predominant immune cell type within the NB tumor immune microenvironment, with their abundance decreasing progressively from favorable stages to advanced stages (50). This finding suggests that M2-like macrophages are likely to have a critical function in inhibiting tumor growth in NB, which is somewhat contradictory to their tumor-promoting characteristics observed in other cancers (51,52). Additional investigations are required to elucidate the precise subtypes and phenotypes of M2-like macrophages present in NB. In sum, our four-gene signatures established based on the NB mRNAsi confirmed the close relationship between CSCs and TME, and demonstrated its potential to predict response to immunotherapy in NB patients. The identified four hub genes may serve as potential targets to aid in the immunotherapy of NB.
Despite advances in the selection of CSCs in NB, targeting these cells effectively remains challenging (53). Our study examined the activity of signaling pathways associated with mRNAsi-related genes, and identified several drugs targeted at certain pathways that suggested better efficacy in the high-risk score group.
Among the four pivotal genes, ERCC6L, also known as PICH, is a DNA helicase that has recently been identified. It has been shown to be involved in embryonic development, suggesting its important role in growth regulation. ERCC6L dysfunction can lead to DNA damage, affecting the cell cycle and division, and promoting cancer development (54,55). Its significant role in tumorigenesis and progression has been demonstrated in many studies (56-59). The long non-coding RNA (lncRNA) DUXAP10 is a pseudogene located on chromosome 14q11.2 and has been found to be upregulated in several types of cancer (60-62). Studies have demonstrated that upregulation of DUXAP10 is linked to advanced disease features and poor outcome, indicating its potential as a prognostic biomarker for predicting cancer outcomes (63-65). Belonging to the lectican family, neurocan (NCAN) is a secreted chondroitin sulfate proteoglycan that inhibits axon regeneration after nervous injury (66). Increased NCAN expression levels have been associated with poor OS in NB patients (67). Additionally, NCAN overexpression in NB cells has been shown to stimulate sphere formation and enhance malignancy. Knockdown of NCAN led to the suppression of potential stemness markers. Based on these results, Su et al. proposed that NCAN may serve as a constituent of the extracellular matrix, creating a specific microenvironment that promotes the growth of CSCs (67). DIRAS3, also known as ARHI, is commonly downregulated or lost in various types of cancer and acts as a tumor suppressor (68-70). Our research has indicated that DIRAS3 also exhibits a protective effect. The restoration of DIRAS3 expression results in inhibited malignant biological behavior in various types of cancer (71-73). Nevertheless, it remains unclear whether DIRAS3 can also suppress tumor growth in NB. To summarize, the mechanistic plausibility of our findings is supported by these genes’ influence on the progression and prognosis of many cancers, including NB. However, some of these genes have not been thoroughly investigated in the context of NB and may represent potential targets for suppressing NB stemness features.
Finally, we developed a prognostic nomogram that provides a user-friendly scoring system. In the clinical setting, it is customary to forecast the prognosis of NB patients by evaluating their clinicopathological features. However, the accuracy of these predictions is often suboptimal. Our nomogram demonstrated prediction accuracy superior to that held by prognostic indicators currently used in clinical practice (stage, MYCN status, age at diagnosis), as indicated by the calibration curves. By combining the four-gene signatures with staging system, this nomogram has the potential to serve as a precise and efficient tool to help evaluate the outcome of NB patients. Such a tool could prove invaluable in facilitating medical decision-making.
Several limitations should be considered in this study. Firstly, the retrospective nature of the analysis and the reliance on bioinformatics approaches are significant drawbacks. Thus, it would be advantageous to obtain prospective sequencing data for future investigations. Secondly, while the stemness index-related signature and the developed prognostic nomogram have shown impressive predictive capabilities for NB survival, it is essential to validate these findings through expanded research involving larger sample sizes, comprehensive data from a broader range of centers, and in-depth prospective studies. Thirdly, to ensure the model aligns with the needs and expectations of key stakeholders, including healthcare professionals and patients, comprehensive qualitative research is imperative. This will involve gathering perspectives on the prognostic model’s utility, challenges, and potential integration into clinical practice, particularly in the context of its deployment as an AI medical device. The nomogram is a two-dimensional graphic scoring method that might be difficult to assign a value to in continuous variables. Alternative methods for simplifying the use of the prediction tool in common practice include web-based applications and scoring tables. As computerized clinical decision support systems (CCDSS), the online application will simplify the predictive model for use in general practice or external validation by other hospitals. A prior systematic review discovered that CCDSS improved care processes such as screening and treatment while having a challenging influence on patient outcomes, healthcare costs, and patient safety (74).
Conclusions
By utilizing the stemness index and ML methods, our study evaluated the prognostic value of CSC features in NB, confirming their role in the development and progression of the disease. For the first time, we constructed a novel mRNAsi-associated signature for NB that exhibited significant associations with prognosis, clinical characteristics, and tumor immune response in NB. The identification of four influential genes for NB stemness in this study improves on prior approaches to NB prognostication and identifies promising therapeutic targets for inhibiting NB stemness characteristics.
Acknowledgments
We express our gratitude to the GEO and ArrayExpress networks for generously sharing the gene expression data utilized in this study.
Funding: This research was supported by
Footnote
Reporting Checklist: The authors have completed the TRIPOD reporting checklist. Available at https://tp.amegroups.com/article/view/10.21037/tp-23-582/rc
Peer Review File: Available at https://tp.amegroups.com/article/view/10.21037/tp-23-582/prf
Conflicts of Interest: All authors have completed the ICMJE uniform disclosure form (available at https://tp.amegroups.com/article/view/10.21037/tp-23-582/coif). H.D.J.H. has been supported in reviewing this manuscript through the National Institute for Health Research (NIHR) doctoral fellowship award, which had no role in the design or delivery of this study. T.H. is an employee of Philips and an editor for several scientific journals, outside the submitted work. The other authors have no conflicts of interest to declare.
Ethical Statement: The authors are accountable for all aspects of the work in ensuring that questions related to the accuracy or integrity of any part of the work are appropriately investigated and resolved. The study was conducted in accordance with the Declaration of Helsinki (as revised in 2013).
Open Access Statement: This is an Open Access article distributed in accordance with the Creative Commons Attribution-NonCommercial-NoDerivs 4.0 International License (CC BY-NC-ND 4.0), which permits the non-commercial replication and distribution of the article with the strict proviso that no changes or edits are made and the original work is properly cited (including links to both the formal publication through the relevant DOI and the license). See: https://creativecommons.org/licenses/by-nc-nd/4.0/.
References
- Kattner P, Strobel H, Khoshnevis N, et al. Compare and contrast: pediatric cancer versus adult malignancies. Cancer Metastasis Rev 2019;38:673-82. [Crossref] [PubMed]
- Marshall GM, Carter DR, Cheung BB, et al. The prenatal origins of cancer. Nat Rev Cancer 2014;14:277-89. [Crossref] [PubMed]
- Chung C, Boterberg T, Lucas J, et al. Neuroblastoma. Pediatr Blood Cancer 2021;68:e28473. [Crossref] [PubMed]
- Newman EA, Abdessalam S, Aldrink JH, et al. Update on neuroblastoma. J Pediatr Surg 2019;54:383-9. [Crossref] [PubMed]
- Tang J, Lu H, Yang Z, et al. Associations between WTAP gene polymorphisms and neuroblastoma susceptibility in Chinese children. Transl Pediatr 2021;10:146-52. [Crossref] [PubMed]
- Qiu B, Matthay KK. Advancing therapy for neuroblastoma. Nat Rev Clin Oncol 2022;19:515-33. [Crossref] [PubMed]
- Reya T, Morrison SJ, Clarke MF, et al. Stem cells, cancer, and cancer stem cells. Nature 2001;414:105-11. [Crossref] [PubMed]
- Ayob AZ, Ramasamy TS. Cancer stem cells as key drivers of tumour progression. J Biomed Sci 2018;25:20. [Crossref] [PubMed]
- Phan TG, Croucher PI. The dormant cancer cell life cycle. Nat Rev Cancer 2020;20:398-411. [Crossref] [PubMed]
- Singh SK, Clarke ID, Terasaki M, et al. Identification of a cancer stem cell in human brain tumors. Cancer Res 2003;63:5821-8.
- Buhagiar A, Ayers D. Chemoresistance, cancer stem cells, and miRNA influences: the case for neuroblastoma. Anal Cell Pathol (Amst) 2015;2015:150634. [Crossref] [PubMed]
- Tian X, Cao F, Li X, et al. Tumor dormancy is closely related to prognosis prediction and tumor immunity in neuroblastoma. Transl Pediatr 2023;12:445-61. [Crossref] [PubMed]
- Pandian V, Ramraj S, Khan FH, et al. Metastatic neuroblastoma cancer stem cells exhibit flexible plasticity and adaptive stemness signaling. Stem Cell Res Ther 2015;6:2. [Crossref] [PubMed]
- Anjomshoaa A, Nasri S, Humar B, et al. Slow proliferation as a biological feature of colorectal cancer metastasis. Br J Cancer 2009;101:822-8. [Crossref] [PubMed]
- Moore N, Lyle S. Quiescent, slow-cycling stem cell populations in cancer: a review of the evidence and discussion of significance. J Oncol 2011;2011:396076. [Crossref] [PubMed]
- Damen MPF, van Rheenen J, Scheele CLGJ. Targeting dormant tumor cells to prevent cancer recurrence. FEBS J 2021;288:6286-303. [Crossref] [PubMed]
- Zheng X, Naiditch J, Czurylo M, et al. Differential effect of long-term drug selection with doxorubicin and vorinostat on neuroblastoma cells with cancer stem cell characteristics. Cell Death Dis 2013;4:e740. [Crossref] [PubMed]
- Walton JD, Kattan DR, Thomas SK, et al. Characteristics of stem cells from human neuroblastoma cell lines and in tumors. Neoplasia 2004;6:838-45. [Crossref] [PubMed]
- Veschi V, Verona F, Thiele CJ. Cancer Stem Cells and Neuroblastoma: Characteristics and Therapeutic Targeting Options. Front Endocrinol (Lausanne) 2019;10:782. [Crossref] [PubMed]
- Malta TM, Sokolov A, Gentles AJ, et al. Machine Learning Identifies Stemness Features Associated with Oncogenic Dedifferentiation. Cell 2018;173:338-354.e15. [Crossref] [PubMed]
- Chen X, Zhang D, Jiang F, et al. Prognostic Prediction Using a Stemness Index-Related Signature in a Cohort of Gastric Cancer. Front Mol Biosci 2020;7:570702. [Crossref] [PubMed]
- Chen D, Liu J, Zang L, et al. Integrated Machine Learning and Bioinformatic Analyses Constructed a Novel Stemness-Related Classifier to Predict Prognosis and Immunotherapy Responses for Hepatocellular Carcinoma Patients. Int J Biol Sci 2022;18:360-73. [Crossref] [PubMed]
- Li N, Li Y, Zheng P, et al. Cancer Stemness-Based Prognostic Immune-Related Gene Signatures in Lung Adenocarcinoma and Lung Squamous Cell Carcinoma. Front Endocrinol (Lausanne) 2021;12:755805. [Crossref] [PubMed]
- Clough E, Barrett T. The Gene Expression Omnibus Database. Methods Mol Biol 2016;1418:93-110. [Crossref] [PubMed]
- Liberzon A, Birger C, Thorvaldsdóttir H, et al. The Molecular Signatures Database (MSigDB) hallmark gene set collection. Cell Syst 2015;1:417-25. [Crossref] [PubMed]
- Yu G, Wang LG, Han Y, et al. clusterProfiler: an R package for comparing biological themes among gene clusters. OMICS 2012;16:284-7. [Crossref] [PubMed]
- Ritchie ME, Phipson B, Wu D, et al. limma powers differential expression analyses for RNA-sequencing and microarray studies. Nucleic Acids Res 2015;43:e47. [Crossref] [PubMed]
- Yoshihara K, Shahmoradgoli M, Martínez E, et al. Inferring tumour purity and stromal and immune cell admixture from expression data. Nat Commun 2013;4:2612. [Crossref] [PubMed]
- Becht E, Giraldo NA, Lacroix L, et al. Estimating the population abundance of tissue-infiltrating immune and stromal cell populations using gene expression. Genome Biol 2016;17:218. [Crossref] [PubMed]
- Aran D, Hu Z, Butte AJ. xCell: digitally portraying the tissue cellular heterogeneity landscape. Genome Biol 2017;18:220. [Crossref] [PubMed]
- Schubert M, Klinger B, Klünemann M, et al. Perturbation-response genes reveal signaling footprints in cancer gene expression. Nat Commun 2018;9:20. [Crossref] [PubMed]
- Jiang P, Gu S, Pan D, et al. Signatures of T cell dysfunction and exclusion predict cancer immunotherapy response. Nat Med 2018;24:1550-8. [Crossref] [PubMed]
- Charoentong P, Finotello F, Angelova M, et al. Pan-cancer Immunogenomic Analyses Reveal Genotype-Immunophenotype Relationships and Predictors of Response to Checkpoint Blockade. Cell Rep 2017;18:248-62. [Crossref] [PubMed]
- Yang W, Soares J, Greninger P, et al. Genomics of Drug Sensitivity in Cancer (GDSC): a resource for therapeutic biomarker discovery in cancer cells. Nucleic Acids Res 2013;41:D955-61. [Crossref] [PubMed]
- Geeleher P, Cox NJ, Huang RS. Clinical drug response can be predicted using baseline gene expression levels and in vitro drug sensitivity in cell lines. Genome Biol 2014;15:R47. [Crossref] [PubMed]
- Laut AK, Dorneburg C, Fürstberger A, et al. CHD5 inhibits metastasis of neuroblastoma. Oncogene 2022;41:622-33. [Crossref] [PubMed]
- Liu Z, Zhang X, Xu M, et al. Loss of CASZ1 tumor suppressor linked to oncogenic subversion of neuroblastoma core regulatory circuitry. Cell Death Dis 2022;13:871. [Crossref] [PubMed]
- Downs-Canner SM, Meier J, Vincent BG, et al. B Cell Function in the Tumor Microenvironment. Annu Rev Immunol 2022;40:169-93. [Crossref] [PubMed]
- Pine SR, Liu W. Asymmetric cell division and template DNA co-segregation in cancer stem cells. Front Oncol 2014;4:226. [Crossref] [PubMed]
- Brabletz T, Jung A, Spaderna S, et al. Opinion: migrating cancer stem cells - an integrated concept of malignant tumour progression. Nat Rev Cancer 2005;5:744-9. [Crossref] [PubMed]
- Maugeri-Saccà M, Vigneri P, De Maria R. Cancer stem cells and chemosensitivity. Clin Cancer Res 2011;17:4942-7. [Crossref] [PubMed]
- Wilson A, Laurenti E, Oser G, et al. Hematopoietic stem cells reversibly switch from dormancy to self-renewal during homeostasis and repair. Cell 2008;135:1118-29. [Crossref] [PubMed]
- Chen J, Li Y, Yu TS, et al. A restricted cell population propagates glioblastoma growth after chemotherapy. Nature 2012;488:522-6. [Crossref] [PubMed]
- Yue ZX, Xing TY, Zhao W, et al. MYCN amplification plus 1p36 loss of heterozygosity predicts ultra high risk in bone marrow metastatic neuroblastoma. Cancer Med 2022;11:1837-49. [Crossref] [PubMed]
- Tsai SC, Lin CC, Shih TC, et al. The miR-200b-ZEB1 circuit regulates diverse stemness of human hepatocellular carcinoma. Mol Carcinog 2017;56:2035-47. [Crossref] [PubMed]
- Xia Y, Li X, Tian X, et al. Identification of a Five-Gene Signature Derived From MYCN Amplification and Establishment of a Nomogram for Predicting the Prognosis of Neuroblastoma. Front Mol Biosci 2021;8:769661. [Crossref] [PubMed]
- Gupta M, Kannappan S, Jain M, et al. Development and validation of a 21-gene prognostic signature in neuroblastoma. Sci Rep 2023;13:12526. [Crossref] [PubMed]
- Nallasamy P, Nimmakayala RK, Parte S, et al. Tumor microenvironment enriches the stemness features: the architectural event of therapy resistance and metastasis. Mol Cancer 2022;21:225. [Crossref] [PubMed]
- Stahl D, Knoll R, Gentles AJ, et al. Prognostic Gene Expression, Stemness and Immune Microenvironment in Pediatric Tumors. Cancers (Basel) 2021;13:854. [Crossref] [PubMed]
- Liu Q, Wang Z, Jiang Y, et al. Single-cell landscape analysis reveals distinct regression trajectories and novel prognostic biomarkers in primary neuroblastoma. Genes Dis 2022;9:1624-38. [Crossref] [PubMed]
- Chen Y, Zhang S, Wang Q, et al. Tumor-recruited M2 macrophages promote gastric and breast cancer metastasis via M2 macrophage-secreted CHI3L1 protein. J Hematol Oncol 2017;10:36. [Crossref] [PubMed]
- Yamaguchi T, Fushida S, Yamamoto Y, et al. Tumor-associated macrophages of the M2 phenotype contribute to progression in gastric cancer with peritoneal dissemination. Gastric Cancer 2016;19:1052-65. [Crossref] [PubMed]
- Farina AR, Cappabianca LA, Zelli V, et al. Mechanisms involved in selecting and maintaining neuroblastoma cancer stem cell populations, and perspectives for therapeutic targeting. World J Stem Cells 2021;13:685-736. [Crossref] [PubMed]
- Abbasi R, Ramroth H, Becher H, et al. Laryngeal cancer risk associated with smoking and alcohol consumption is modified by genetic polymorphisms in ERCC5, ERCC6 and RAD23B but not by polymorphisms in five other nucleotide excision repair genes. Int J Cancer 2009;125:1431-9. [Crossref] [PubMed]
- Hübner NC, Wang LH, Kaulich M, et al. Re-examination of siRNA specificity questions role of PICH and Tao1 in the spindle checkpoint and identifies Mad2 as a sensitive target for small RNAs. Chromosoma 2010;119:149-65. [Crossref] [PubMed]
- Zhang G, Ma J, Xiong J, et al. Upregulation of Excision Repair Cross-Complementation Group 6-Like (ERCC6L) Promotes Tumor Growth in Hepatocellular Carcinoma. Dig Dis Sci 2021;66:1097-109. [Crossref] [PubMed]
- Chen D, Liu Q, Cao G. ERCC6L promotes cell growth and metastasis in gastric cancer through activating NF-κB signaling. Aging (Albany NY) 2021;13:20218-28. [Crossref] [PubMed]
- Xie Y, Yu J, Wang F, et al. ERCC6L promotes cell growth and invasion in human colorectal cancer. Oncol Lett 2019;18:237-46. [Crossref] [PubMed]
- Huang X, Jiang L, Lu S, et al. Overexpression of ERCC6L correlates with poor prognosis and confers malignant phenotypes of lung adenocarcinoma. Oncol Rep 2022;48:131. [Crossref] [PubMed]
- Zhu Q, Liu J, Tang J, et al. Overexpression of long non-coding RNAs DUXAP9 and DUXAP10 is associated with prognosis in patients with hepatocellular carcinoma after hepatectomy. Int J Clin Exp Pathol 2018;11:1407-14.
- Wu B, Yang C, Fang Y, et al. Long noncoding RNA DUXAP10 promotes the stemness of glioma cells by recruiting HuR to enhance Sox12 mRNA stability. Environ Toxicol 2021;36:840-9. [Crossref] [PubMed]
- Lv XY, Ma L, Chen JF, et al. Knockdown of DUXAP10 inhibits proliferation and promotes apoptosis in bladder cancer cells via PI3K/Akt/mTOR signaling pathway. Int J Oncol 2018;52:288-94. [Crossref] [PubMed]
- Chen J, Wang XF, Qin YC, et al. Downregulation of long non-coding RNA DUXAP10 inhibits proliferation, migration, and invasion of renal cell carcinoma. Eur Rev Med Pharmacol Sci 2020;24:11041-51. [Crossref] [PubMed]
- Lian Y, Xu Y, Xiao C, et al. The pseudogene derived from long non-coding RNA DUXAP10 promotes colorectal cancer cell growth through epigenetically silencing of p21 and PTEN. Sci Rep 2017;7:7312. [Crossref] [PubMed]
- Lin HP, Wang Z, Yang C. LncRNA DUXAP10 Upregulation and the Hedgehog Pathway Activation Are Critically Involved in Chronic Cadmium Exposure-Induced Cancer Stem Cell-Like Property. Toxicol Sci 2021;184:33-45. [Crossref] [PubMed]
- Asher RA, Morgenstern DA, Fidler PS, et al. Neurocan is upregulated in injured brain and in cytokine-treated astrocytes. J Neurosci 2000;20:2427-38. [Crossref] [PubMed]
- Su Z, Kishida S, Tsubota S, et al. Neurocan, an extracellular chondroitin sulfate proteoglycan, stimulates neuroblastoma cells to promote malignant phenotypes. Oncotarget 2017;8:106296-310. [Crossref] [PubMed]
- Zhang S, Feng XL, Shi L, et al. Genome-wide analysis of DNA methylation in tongue squamous cell carcinoma. Oncol Rep 2013;29:1819-26. [Crossref] [PubMed]
- Zou CF, Jia L, Jin H, et al. Re-expression of ARHI (DIRAS3) induces autophagy in breast cancer cells and enhances the inhibitory effect of paclitaxel. BMC Cancer 2011;11:22. [Crossref] [PubMed]
- Li J, Cui G, Sun L, et al. ARHI overexpression induces epithelial ovarian cancer cell apoptosis and excessive autophagy. Int J Gynecol Cancer 2014;24:437-43. [Crossref] [PubMed]
- Sutton MN, Lu Z, Li YC, et al. DIRAS3 (ARHI) Blocks RAS/MAPK Signaling by Binding Directly to RAS and Disrupting RAS Clusters. Cell Rep 2019;29:3448-3459.e6. [Crossref] [PubMed]
- Zhao X, Li J, Zhuo J, et al. Reexpression of ARHI inhibits tumor growth and angiogenesis and impairs the mTOR/VEGF pathway in hepatocellular carcinoma. Biochem Biophys Res Commun 2010;403:417-21. [Crossref] [PubMed]
- Badgwell DB, Lu Z, Le K, et al. The tumor-suppressor gene ARHI (DIRAS3) suppresses ovarian cancer cell migration through inhibition of the Stat3 and FAK/Rho signaling pathways. Oncogene 2012;31:68-79. [Crossref] [PubMed]
- Souza NM, Sebaldt RJ, Mackay JA, et al. Computerized clinical decision support systems for primary preventive care: a decision-maker-researcher partnership systematic review of effects on process of care and patient outcomes. Implement Sci 2011;6:87. [Crossref] [PubMed]