Artificial intelligence-powered early identification of refractory constipation in children
Highlight box
Key findings
• A machine-learning model for early detection of refractory constipation among children was built based on features of the colonic anatomy.
What is known and what is new?
• Patients with constipation, often presenting colonic redundancy and dilatation, are diagnosed based on symptoms that can lead to prolonged illness and low quality of life.
• Characteristics of colonic anatomy are described in children with constipation.
What is the implication, and what should change now?
• We described the characteristics of colonic anatomy in children with different types of constipation and developed a supervised machine learning model for the early identification of refractory constipation, which may improve the management of the disease.
Introduction
Constipation is a common condition among adults and children (1). The proportion of constipation in pediatric gastroenterology outpatient clinics is reported to be approximately 25% (2). Among them, only a few are of an organic etiology including Hirschsprung’s disease, anorectal abnormalities, hypothyroidism, spina bifida, etc. (3). More than 95% of constipations are considered to be functional (4). Currently, the approaches to diagnosing functional constipation are based on the symptoms (5). A series of Rome criteria has been launched and modified for the diagnosis of functional constipation, and Rome IV is the current gold standard of diagnostic tools (6,7).
According to the Rome IV criteria, children with functional constipation can be treated with rectal or oral disimpaction at first when fecal impaction is presented followed by maintenance therapy to prevent reaccumulation of feces using a variety of agents such as polyethylene glycol (PEG) or lactulose (7). Education is considered essential, along with medical therapy, and maintaining a regular intake of fiber and fluids is also beneficial (8).
For most functional constipated children, clinical symptoms can be improved after conventional therapies. Despite optimal conventional treatments, up to one-third of the children remain unresponsive for more than three months, at which point they are considered to have refractory constipation according to ESPGHAN and NASPGHAN (3). Functional constipation has a significant impact on the quality of life of both children and parents (9). According to clinical investigations of Bongers et al. (2), a delay in treatment is a risk factor for the long-term poor clinical outcome of children with functional constipation and also affects their quality of life.
However, for children with refractory constipation who do not respond to treatment, the situation may be substantially worse (10). This prolonged state of constipation and fecal loading can contribute to the development of colonic dilation (11). Studies indicate that dilated segments exhibit a reduced number of high-amplitude propagating contractions and disruptions in the postprandial cyclic propagating motor pattern, causing dysfunction in colonic motility (12-14). Consequently, the dilated colon tends to reabsorb more water, resulting in the formation of hard stools and stretching of the colonic lumen (15).
In adults, a redundant colon has been proven to be associated with refractory constipation (16). A >6.5 cm colonic width is commonly recognized as an indicator for the redundant colon and thus suggests the presence of refractory constipation (17). However, absolute cut-off values for redundant colon have not been established for children, mainly because colonic diameters and length in children vary a lot depending on age and body size. To address this issue, standardized colon size (SCS) was first proposed to normalize the colonic diameters and length despite age and body size (18). By calculating the various colonic diameters and lengths to the second lumbar vertebral body (L2), Koppen et al. found that SCS ratios for the length of the rectosigmoid and the descending colon and the SCS ratio for sigmoid colon diameter were significantly larger in children with refractory constipation compared to a previously described normative population (19). Likewise, other attempts have been made to discover the relevance between the colonic diameters and length to the refractory constipation (12,20). It is believed that more attention and more intensive healthcare should be addressed for children with refractory constipation (1). However, no standard has been set up for the early identification of functional constipation and refractory constipation.
As one of the key techniques of artificial intelligence (AI), machine learning (ML) has been widely applied to disease prognosis, diagnosis, and treatment recently (21). ML allows predictions and classification of data from unidentified sources via learning patterns from data using algorithms (22). Numerous machine-learning models have been reported to aid the diagnosis of gastrointestinal diseases. For instance, a support vector machine (SVM) was used to classify pediatric inflammatory bowel disease according to a set of endoscopic and histological data with an accuracy of 82.7% (23). By extracting features from the electrogastrogram, an artificial neural network (ANN) enabled early abnormalities detection in the digestive system (24). The gradient-boosted regression trees were utilized for predicting fecal biomarkers associated with constipation (25). However, so far, the ML model for the early identification of refractory constipation has not yet been reported.
Hence, in this study, we aim to utilize the ML model for the early identification of functional constipation and refractory constipation in children. The early identification of refractory constipation may guide the management of the patient population including suggestion of further examination or more radical treatment. Also, for doctors and parents, more attention and more intensive healthcare should be paid. We present this article in accordance with the TRIPOD reporting checklist (available at https://tp.amegroups.com/article/view/10.21037/tp-23-497/rc).
Methods
Study design
This was a retrospective, single-center study among children aged 1–14 years old undergoing barium enema (BE) between January 2016 and February 2020 at the Department of Gastroenterology, Children’s Hospital of Nanjing Medical University (Nanjing, Jiangsu, China). The study was conducted in accordance with the Declaration of Helsinki (as revised in 2013). The study was approved by the Institutional Board of the Hospital of Nanjing Medical University (No. 202008054-1). All patients’ legal guardians provided their written consent to perform BE and agreed to share their demographics and clinical features.
Study participants
Children documented were 1–14 years old when taking BE. Children with organic constipation (e.g., Hirschsprung’s disease, anorectal abnormalities, hypothyroidism, spina bifida) or a lack of rectoanal inhibitory reflex were excluded. If BE data were incomplete or measurements could not be performed, children were also not eligible.
Study groups
Due to the retrospective nature of the study, children were grouped based on the patient’s medical records.
They were divided into three groups:
Patients with functional constipation
Children who met the Rome IV diagnostic criteria of functional constipation, and symptoms gradually improved after receiving conventional therapy.
Patients with refractory constipation
Children who met the Rome IV diagnostic criteria of functional constipation, but showed no response to optimal conventional treatments for more than three months as specified in the ESPGHAN-NASPGHAN functional constipation guidelines.
Controls
Children attended the clinic with symptoms of abdominal pain and bloating, after thorough examination by different tests, including BE, these children were last diagnosed with functional abdominal pain. These children were considered as the non-constipation group.
BE
The diagnostic testing of contrast enema was used to exclude anatomical abnormalities such as Hirschsprung’s disease, an abnormally narrow, funnel-shaped rectum, and also a way to evaluate the morphology of the colon. The legal guardians of each participant provided written informed consent for taking BE. All patients underwent BE with bowel preparation before the X-ray examination. During BE, children were placed in the supine position with the assistance of their parents to ensure that they did not move during the procedure. Then, the anus was catheterized with a suitable caliber tube for inserting barium contrast (composed of barium sulfate and normal saline at a 1:5 ratio) into the rectum by using a syringe, The colon was examined in anterior, posterior, and lateral projections which allowed the pediatric radiologist to capture radiographic pictures and analyze the colonic anatomy. Measurements were performed by an experienced pediatric radiologist and cross-referenced by a pediatrician.
Data collection
The ratio of SCS allows operators to standardize the colonic diameters and length despite age and body size. The size of the colon was measured together by the radiologist and pediatrician from the same radiographic images. Parameters including the diameters of the sigmoid colon, descending colon, transverse colon, ascending colon, rectum, and the length of rectosigmoid were measured, and the width of the second lumbar vertebral body (L2) was also measured. In addition, the SCS was calculated by the ratio between the above diameters to the width of L2, respectively.
Statistical analysis
The statistical analyses were carried out using IBM’s Statistical Package for Social Science (SPSS, version 24). The Kolmogorov-Smirnov test was used to ensure that the data were distributed evenly. If the data passed the normality test, the results from the three groups were presented as mean ± standard deviation (SD). The data were analyzed by one-way analysis of variance (one-way ANOVA) followed by the Tukey test. If the preliminary test for normality is not significant, the data were expressed as median values with interquartile ranges (IQRs). The Mann-Whitney U test was used to compare the results between the two genders. The Kruskal-Wallis H test was applied to compare colonic segmental measurements among various age groups. The statistical significance level for the P value was set at 0.05.
ML model development
For visual identification, all of the data were loaded into a Microsoft Excel sheet and plotted. The colon length dataset was fed into Python’s Scikit-Learn library (https://scikit-learn.org/stable/), which was used to create a supervised ML script. To minimize the dimensionality of the data, the characteristic features were initially selected based on the ANOVA F values. The control, functional constipation, and refractory constipation groups were then classified using a SVM. The dataset was divided into a training set and a test set randomly, with the training set being used to train the supervised learning model and the test set being used to evaluate the model’s classification accuracy. The number of selected features in ANOVA F value selection, kernel type, C value, and gamma value in SVM were among the parameters optimized using a grid search. In addition, k-fold cross-validation (k-fold value of three) was used to examine the model’s overall accuracy more satisfactorily. The confusion matrix and decision boundaries were then calculated using the model with the best performance parameters.
Results
Clinical characteristics of patient population
The workflow for the patient recruiting process is shown in Figure 1, and the clinical features are summarized in Table 1. A total of 293 patients underwent BE between January 2016 and February 2020. Eighty-eight patients were excluded due to organic disease (n=43), incomplete BE (n=30), or insufficient images (n=15). Based on the criteria, the remaining 205 participants were categorized into three groups: 65 patients in the control group (45%, n=29 male), 77 in the functional constipation group (47%, n=36 male), and 63 in the refractory constipation group (49%, n=31 male). No significant difference in gender (P=0.87) or age (P=0.71) were observed among the three groups. The duration of constipation has a statistical difference between the functional group [median, 14 months; 95% confidence interval (CI): 14.1 to 18.7] and the refractory constipation group (median, 22 months; 95% CI: 17.84 to 23.68) (P=0.039). Recto-anal inhibitory reflex (RAIR) was present in all patients in the functional and refractory constipation groups.
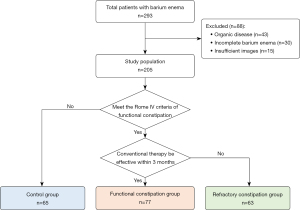
Table 1
Characteristics | Ctrl (n=65) | FC (n=77) | RC (n=63) | P |
---|---|---|---|---|
Gender | 0.87 | |||
Male | 29 [45] | 36 [47] | 31 [49] | |
Female | 36 [55] | 41 [53] | 32 [51] | |
Age (years) | 3 [2–6] (3.58–5.31) | 4 [3–5] (3.63–4.68) | 4 [2–5] (3.46–4.80) | 0.71 |
Constipation duration (months) | – | 14 [7.5–24.5] (14.1–18.7) | 22 [10–28] (17.84–23.68) | 0.039 |
RAIR present (n=140) | – | 77 | 63 | – |
Data are expressed as n [%], median [IQR] (95% CI) or number. Ctrl, control group; FC, functional constipation group; RC, refractory constipation group; RAIR, rectoanal inhibitory reflex; IQR, interquartile range; CI, confidence interval.
Colon size ratios analysis
As mentioned in the methods, the SCS ratios of various colon segments were measured and calculated. First, we analyzed the differences in the above SCS ratios between genders and among ages. The differences in the above SCS ratios in males and females are shown in Table S1 and no significant differences were observed (all P>0.05). The differences in the SCS ratios by age were analyzed in Table S2 and illustrated in Figure S1 with no significant differences found (all P>0.05). These results verified that the SCS ratios do not vary with sex or age, and thus can be used to standardize colon sizes.
The SCS ratios in the three groups were compared and the results are shown in Table 2. The differences in SCS ratios of all colon segments among the three groups are statistically significant (all P<0.01). Then pairwise comparison was performed for each group.
Table 2
Colon segments | Ctrl (n=65) | FC (n=77) | RC (n=63) | P |
---|---|---|---|---|
Rectum | 1.41±0.18 (1.37–1.46) | 1.63±0.19 (1.59–1.68) | 1.82±0.19 (1.77–1.86) | <0.001 |
Sigmoid colon | 1.12±0.10 (1.10–1.15) | 1.17±0.17 (1.13–1.21) | 1.48±0.20 (1.42–1.53) | <0.001 |
Descending colon | 1.04±0.09 (1.02–1.06) | 1.04±0.12 (1.01–1.06) | 1.14±0.12 (1.11–1.17) | <0.001 |
Transverse colon | 1.10±0.17 (1.06–1.14) | 1.20±0.16 (1.17–1.24) | 1.30±0.27 (1.24–1.38) | <0.001 |
Ascending colon | 1.13±0.11 (1.10–1.16) | 1.21±0.15 (1.17–1.26) | 1.29±0.13 (1.25–1.33) | <0.001 |
Rectosigmoid | 10.1±1.71 (9.64–10.5) | 13.7±2.57 (13.1–14.3) | 15.4±2.63 (14.7–16.0) | <0.001 |
Data are expressed as mean ± SD (95% CI). SCS, standardized colon size; Ctrl, control group; FC, functional constipation group; RC, refractory constipation group; SD, standard deviation; CI, confidence interval.
As shown in Figure 2 and Table 3, an incremental trend of severity can be observed by the development of constipation. Between the control group and functional constipation group, the SCS ratios of the rectum, transverse colon, ascending colon, and rectosigmoid were found to be significantly different (P<0.001; P=0.004; P=0.03; P<0.001), while that of the sigmoid colon and the descending colon were not (P=0.21; P=0.99). Between the functional constipation group and refractory constipation group, significant differences exist in SCS ratios of all of the colon segments (P≤0.001) except the ascending colon (P=0.32). And between the control group and the refractory constipation group, significant differences can be observed in the SCS ratios of all of the colon segments (P<0.001).
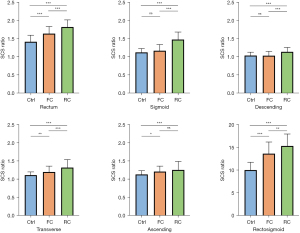
Table 3
Colon segments | Ctrl-FC | FC-RC | Ctrl-RC |
---|---|---|---|
Rectum | <0.001*** | <0.001*** | <0.001*** |
Sigmoid colon | 0.21 | <0.001*** | <0.001*** |
Descending colon | 0.99 | <0.001*** | <0.001*** |
Transverse colon | 0.004** | <0.001*** | <0.001*** |
Ascending colon | 0.03* | 0.32 | <0.001*** |
Rectosigmoid | <0.001*** | 0.001** | <0.001*** |
*, P<0.05; **, P<0.01; ***, P<0.001. SCS, standardized colon size; Ctrl, control group; FC, functional constipation group; RC, refractory constipation group.
ML model of constipation classification
In our study, the SVM acted as our model to classify patients from control, functional constipation, and refractory constipation groups. To reduce the dimensionality of the dataset, ANOVA F values were applied to select input features for SVM analysis. Input features included gender, age, and SCS ratios of the above six colon segments. After selecting, gender, age, and the SCS ratio of ascending colon were ruled out. The SCS ratios of the sigmoid colon, descending colon, transverse colon, rectum, and rectosigmoid were accepted. For the best classification, two different kernel types, linear kernel, and radial basis function (RBF) kernel were compared. The better kernel was determined by considering all the combinations of the following parameters through grid search: C values of 0.001, 0.01, 0.1, 1, 10, 100, 1,000, and 10,000 and gamma values of 0.001, 0.01, 0.1, 1, 10, and 100. The k-fold cross-validation (70% of the data for training, 30% for testing) was performed to evaluate model accuracy and prevent overfitting. The maximum accuracy was achieved by the SVM model with a linear kernel, a C value of 100, and a gamma value of 0.1 (81% accuracy, 95% CI: 79.17% to 83.19%). The corresponding confusion matrix is shown in Figure 3, and the decision boundaries of selected features are shown in Figure S2.
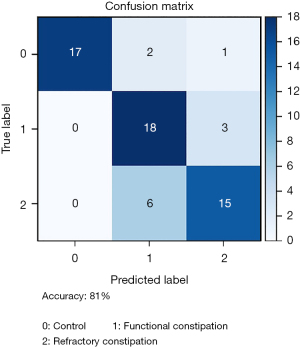
Since a linear SVM model was fitted in this research, it is possible to access the feature coefficients. Feature coefficients can be viewed as the weights of each feature in the fitted model. The absolute size of the feature coefficient can be used to determine the individual feature importance of the classification model. The feature coefficients of the above-fitted model are displayed in Figure 4. The ascending order of the feature coefficients based on the absolute size was the SCS ratios of rectosigmoid, transverse colon, rectum, sigmoid colon, and descending colon. The above results suggest the SCS ratios of the descending colon showed the highest importance in the classification of control/functional constipation/refractory constipation based on the dataset we collected. On the contrary, the SCS ratios of rectosigmoid exhibited the smallest feature coefficient, which indicated the SCS ratios of rectosigmoid contributed the least to the fitted classification model.
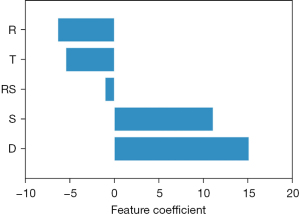
Discussion
In children diagnosed with functional constipation, almost one-third subsequently develop refractory constipation. These children undergo conventional treatment for a long time until it becomes evident that the treatment is ineffective. Early identification of refractory constipation might lead to timely interventions, improving clinical outcomes. In this study, we characterized the SCS ratios of colon segments in 205 children, and developed a supervised machine-learning model for earlier identification of refractory constipation in children. With an accuracy of 81%, we demonstrated that this model can effectively distinguish refractory constipation from functional constipation and non-constipation in children. The application of the ML model may assist clinicians in identifying patients with refractory constipation and supporting clinical decision-making, resulting in further examinations and more suitable regimens. Additionally, since the ML model can help identify patients with functional constipation, it can potentially increase patients’ confidence in the treatment, which is considered to be an important factor in the treatment of constipation, especially in children. To the best of our knowledge, this is the first to systematically characterize the length and SCS ratios of colon segments in children with refractory constipation, normal functional constipation, and non-constipation. Meanwhile, this is also the first study utilizing the ML model to classify these three populations.
It has been frequently reported that patients with constipation had a high frequency of colonic redundancy and dilatation (26-30). The reference values of rectosigmoid and sigmoid diameters in adults have long been investigated for the acquired megacolon diagnosis (11). However, there is no clear definition for colonic redundancy and dilatation in children, mainly due to variations in colon segments dimensions based on age and body size. To address this problem, Koppen et al. introduced the concept of SCS ratio to standardize colonic size for children. By performing a retrospective chart review of 119 children aged 0–5 years who had undergone air contrast enemas for intussusception, they obtained the reference data for normal colonic size in young children (18). Moreover, they also found that the diameter ratios of the rectum and the ascending colon increase with age, while that of the sigmoid, descending, and transverse colon segments did not. Later, Koppen et al. and Noviello et al. investigated the SCS ratios of children with refractory constipation and compared the results to the normative reference values as mentioned above (19,20), respectively. Koppen et al. performed a retrospective study on 30 children aged 0–18 years with refractory constipation who had undergone contrast enemas. They found that SCS ratios for the length of the rectosigmoid and the descending colon and the SCS ratio for sigmoid colon diameter were significantly larger in children with refractory constipation compared to the previously described normative population (19). On the other hand, Noviello et al. studied 57 patients aged 0–14 years with chronic refractory constipation and reported that none of the measurements was statistically significant except for the SCS ratio of the length of the rectosigmoid colon (20).
Similar to previous studies, the results in our study confirm the difference in the SCS ratios of colon segments between the non-constipation and refractory constipation children. However, some of our findings differ from those previously reported. One difference is that most of the SCS ratio values of colon segments of the non-constipation population in our study were slightly smaller than that in Koppen’s. It may be due to the different control populations and imaging modalities: patients with intussusception and air contrast were used by Koppen et al. (18), while patients without organic changes or constipation and BE were selected in our study. During pneumatic reduction of intussusception, the excessive pressure in the colon may lead to the expansion of its parts, thus exaggerating the width of the colon segments. Therefore, the normative values of our study may be more generalizable and representative. Second, different from the reference value provided by Koppen’s, according to our data, the SCS ratios do not increase along with age in the non-constipation group or the overall population. It is possibly due to the wide age range of the participants in our study, which may weaken the statistical difference, as 0–5 years chosen in the previous study is a period of rapid growth of children. Also, whether air contrast would increase the difference remains unknown. Third, a previous study reported that SCS ratios of some of the colon segments were significantly different between the refractory constipation group and the normal group, while we found it true for all the six parameters we measured. It comes back to the discussion about the reference data they used, in which the pressure of air contrast might enlarge the values of the normal group. As the data for the two groups were measured and proceeded in the same study, we, therefore, consider our results to be more reliable. Fourth, besides the refractory constipation group, the functional constipation group was considered for the first time. We hypothesize the state of the colon segments of functional constipation to be intermediate between non-constipation and refractory constipation. It is confirmed by the incremental trend of severity observed in three groups, which has not been studied before. However, whether the state of the colon segments can be restored to normal levels when the functional constipation children recover remains unknown. Further investigation is required. Last but not least, unlike previous studies focusing on the comparison and correlation of every single variable, we established a ML model and evaluated all the variables comprehensively. According to ANOVA F values, age, and gender were determined to be independent of classification, which is consistent with the statistical analysis. The ascending colon was considered to have little effect on classification, which hasn’t been mentioned in the previous studies. We identified that the SCS ratios of the descending colon, sigmoid colon, and rectum contributed the most to classification, which significantly corroborated with Koppen’s work. This is probably because, in refractory constipation, the actual dysfunctional region is immediately above the rectum, as reported previously (28-30). Currently, it is unclear if colonic dilation is the cause of dysmotility or vice versa, or if they have a cyclic causality (31-33). There may be other factors that influence the phenomenon (34,35). Further research may be necessary to investigate the definition of colon dilatation and redundancy, which is crucial for further treatment.
In recent years, the utilization of ML algorithms has become widespread for developing disease prediction models (36). Compared with conventional statistical methods, ML excels in accurately predicting outcomes across diverse datasets through high-level computing, constructing algorithms for automated data-driven predictions or classifications (37). Linear regression assumes a linear relationship between the input features and the target variable, which may not capture the underlying patterns in our dataset adequately (38). For image extraction tasks, convolutional neural networks (CNNs) are highly effective and widely used in ANNs designed specifically for image recognition and processing (39). In light of these advancements, we have made attempts to train a CNN model, but the limited sample size raised concerns about potential overfitting in the results. Human extraction of colonic diameters and standardization was chosen by the essential role of human expertise in accurately identifying and interpreting complex anatomical features, especially in children where anatomical structures can vary widely. In the future, we would extend our analysis to include other medical datasets for image extraction. In this study, the model establishment process involved randomly dividing all patients into a training set and a test set at a ratio of 7:3. The training set was used to establish the model, and the model underwent validation on the test set subsequently. ANOVA F was used to determine the characteristic features. SVM was used to model the data in the training set. Additionally, three-fold cross-validation was conducted to assess model stability and determine whether the model was over-fitted. In our specific case, the decision to use ML model was driven by the complex and non-linear relationships inherent in our data. Among various ML techniques, SVM stands out for its notable accuracy and efficiency, particularly in small datasets medical applications (40). SVM is a versatile algorithm that can effectively model non-linear relationships through the use of kernel functions. This flexibility allows SVM to capture intricate patterns and dependencies in the data, making it more suitable for our particular problem (41). Furthermore, research literature consistently highlights the remarkable accuracy of the SVM model in the medical diagnosis (42). However, there are some limitations to this study. First of all, we recognized the fact that acquiring normative data in healthy children was of great challenge in clinical studies, especially involving radiation exposure. Therefore, we selected children without a diagnosis of constipation and were diagnosed with functional abdominal pain. However, it is unclear whether the children are an accurate representative group of the healthy population, which has also been challenging in prior studies (43,44). Furthermore, there are possible measurement errors caused by the overlapping colon segments in images. To minimize the measurement error, we used only one two-dimensional image to obtain all colon size data and measured the rectosigmoid length in both supine and prone positions. Moreover, our study developed a new model rather than obtaining a specific parameter cutoff, which is not intuitive. Further development of integration of the model into the imaging system, including automatic measurements, would be more useful. Finally, while our study stands as the largest of its kind, it is important to acknowledge its limitations, including a small sample size and a single-center design. Additionally, the absence of external validation is a notable constraint. We plan to extend our analysis to include other medical datasets for comprehensive validation, thereby enhancing the robustness and generalizability of our model.
Conclusions
In this retrospective study, we described the characteristics of colonic anatomy in children with non-constipation, constipation, and refractory constipation. By building a supervised ML model, the three groups could be distinguished with 81% accuracy. For the future, we aim to use this model with additional medical datasets to further validate its effectiveness and expand its capabilities. The application of the ML model provides practical implications, which are significant for earlier identification and guiding management in children with refractory constipation. This enables clinicians to tailor management strategies, offering suggestion for further examinations or more radical treatment.
Acknowledgments
Funding: This study was supported by
Footnote
Reporting Checklist: The authors have completed the TRIPOD reporting checklist. Available at https://tp.amegroups.com/article/view/10.21037/tp-23-497/rc
Data Sharing Statement: Available at https://tp.amegroups.com/article/view/10.21037/tp-23-497/dss
Peer Review File: Available at https://tp.amegroups.com/article/view/10.21037/tp-23-497/prf
Conflicts of Interest: All authors have completed the ICMJE uniform disclosure form (available at https://tp.amegroups.com/article/view/10.21037/tp-23-497/coif). The authors have no conflicts of interest to declare.
Ethical Statement: The authors are accountable for all aspects of the work in ensuring that questions related to the accuracy or integrity of any part of the work are appropriately investigated and resolved. The study was conducted in accordance with the Declaration of Helsinki (as revised in 2013). The study was approved by the Institutional Board of the Hospital of Nanjing Medical University (No. 202008054-1). All patients’ legal guardians provided their written consent to perform BE and agreed to share their demographics and clinical features.
Open Access Statement: This is an Open Access article distributed in accordance with the Creative Commons Attribution-NonCommercial-NoDerivs 4.0 International License (CC BY-NC-ND 4.0), which permits the non-commercial replication and distribution of the article with the strict proviso that no changes or edits are made and the original work is properly cited (including links to both the formal publication through the relevant DOI and the license). See: https://creativecommons.org/licenses/by-nc-nd/4.0/.
References
- Vriesman MH, Koppen IJN, Camilleri M, et al. Management of functional constipation in children and adults. Nat Rev Gastroenterol Hepatol 2020;17:21-39. [Crossref] [PubMed]
- Bongers ME, van Wijk MP, Reitsma JB, et al. Long-term prognosis for childhood constipation: clinical outcomes in adulthood. Pediatrics 2010;126:e156-62. [Crossref] [PubMed]
- Tabbers MM, DiLorenzo C, Berger MY, et al. Evaluation and treatment of functional constipation in infants and children: evidence-based recommendations from ESPGHAN and NASPGHAN. J Pediatr Gastroenterol Nutr 2014;58:258-74. [Crossref] [PubMed]
- Loening-Baucke V. Chronic constipation in children. Gastroenterology 1993;105:1557-64. [Crossref] [PubMed]
- Mearin F, Lacy BE, Chang L, et al. Bowel Disorders. Gastroenterology 2016;S0016-5085(16)00222-5.
- Benninga MA, Faure C, Hyman PE, et al. Childhood Functional Gastrointestinal Disorders: Neonate/Toddler. Gastroenterology 2016;S0016-5085(16)00182-7.
- Hyams JS, Di Lorenzo C, Saps M, et al. Functional Disorders: Children and Adolescents. Gastroenterology 2016; Epub ahead of print. [Crossref]
- Nurko S, Zimmerman LA. Evaluation and treatment of constipation in children and adolescents. Am Fam Physician 2014;90:82-90.
- Belsey J, Greenfield S, Candy D, et al. Systematic review: impact of constipation on quality of life in adults and children. Aliment Pharmacol Ther 2010;31:938-49. [Crossref] [PubMed]
- Koppen IJ, Kuizenga-Wessel S, Lu PL, et al. Surgical decision-making in the management of children with intractable functional constipation: What are we doing and are we doing it right? J Pediatr Surg 2016;51:1607-12. [Crossref] [PubMed]
- Yik YI, Cook DJ, Veysey DM, et al. How common is colonic elongation in children with slow-transit constipation or anorectal retention? J Pediatr Surg 2012;47:1414-20. [Crossref] [PubMed]
- Cuda T, Gunnarsson R, de Costa A. Symptoms and diagnostic criteria of acquired Megacolon - a systematic literature review. BMC Gastroenterol 2018;18:25. [Crossref] [PubMed]
- Scott SM, Simrén M, Farmer AD, et al. Chronic constipation in adults: Contemporary perspectives and clinical challenges. 1: Epidemiology, diagnosis, clinical associations, pathophysiology and investigation. Neurogastroenterol Motil 2021;33:e14050. [Crossref] [PubMed]
- Dinning PG, Zarate N, Hunt LM, et al. Pancolonic spatiotemporal mapping reveals regional deficiencies in, and disorganization of colonic propagating pressure waves in severe constipation. Neurogastroenterol Motil 2010;22:e340-9. [Crossref] [PubMed]
- von der Ohe MR, Camilleri M, Carryer PW. A patient with localized megacolon and intractable constipation: evidence for impairment of colonic muscle tone. Am J Gastroenterol 1994;89:1867-70.
- Camilleri M, Brandler J. Refractory Constipation: How to Evaluate and Treat. Gastroenterol Clin North Am 2020;49:623-42. [Crossref] [PubMed]
- Preston DM, Lennard-Jones JE, Thomas BM. Towards a radiologic definition of idiopathic megacolon. Gastrointest Radiol 1985;10:167-9. [Crossref] [PubMed]
- Koppen IJ, Yacob D, Di Lorenzo C, et al. Assessing colonic anatomy normal values based on air contrast enemas in children younger than 6 years. Pediatr Radiol 2017;47:306-12. [Crossref] [PubMed]
- Koppen IJN, Thompson BP, Ambeba EJ, et al. Segmental colonic dilation is associated with premature termination of high-amplitude propagating contractions in children with intractable functional constipation. Neurogastroenterol Motil 2017;29:1-9. [Crossref] [PubMed]
- Noviello C, Nobile S, Romano M, et al. Functional constipation or redundancy of the colon? Indian J Gastroenterol 2020;39:147-52. [Crossref] [PubMed]
- Rajkomar A, Dean J, Kohane I. Machine Learning in Medicine. N Engl J Med 2019;380:1347-58. [Crossref] [PubMed]
- Jordan MI, Mitchell TM. Machine learning: Trends, perspectives, and prospects. Science 2015;349:255-60. [Crossref] [PubMed]
- Mossotto E, Ashton JJ, Coelho T, et al. Classification of Paediatric Inflammatory Bowel Disease using Machine Learning. Sci Rep 2017;7:2427. [Crossref] [PubMed]
- Amri MF, Yuliani AR, Simbolon AI, et al. Toward Early Abnormalities Detection on Digestive System: Multi-Features Electrogastrogram (EGG) Signal Classification based on Machine Learning. 2021 International Conference on Radar, Antenna, Microwave, Electronics, and Telecommunications (ICRAMET); 23-24 November; Bandung, Indonesia.IEEE; 2021:185-90.
- Chen Y, Wu T, Lu W, et al. Predicting the Role of the Human Gut Microbiome in Constipation Using Machine-Learning Methods: A Meta-Analysis. Microorganisms 2021;9:2149. [Crossref] [PubMed]
- Raahave D. Dolichocolon revisited: An inborn anatomic variant with redundancies causing constipation and volvulus. World J Gastrointest Surg 2018;10:6-12. [Crossref] [PubMed]
- van den Berg MM, Hogan M, Caniano DA, et al. Colonic manometry as predictor of cecostomy success in children with defecation disorders. J Pediatr Surg 2006;41:730-6; discussion 730-6. [Crossref] [PubMed]
- Radwan AB, El-Debeiky MS, Abdel-Hay S. Contrast enema as a guide for senna-based laxatives in managing overflow retentive stool incontinence in pediatrics. Pediatr Surg Int 2015;31:765-71. [Crossref] [PubMed]
- Levitt MA, Mathis KL, Pemberton JH. Surgical treatment for constipation in children and adults. Best Pract Res Clin Gastroenterol 2011;25:167-79. [Crossref] [PubMed]
- Raahave D, Loud FB, Christensen E, et al. Colectomy for refractory constipation. Scand J Gastroenterol 2010;45:592-602. [Crossref] [PubMed]
- Steffen RM. Colonic diversion for intractable constipation in children: colonic manometry helps guide clinical decisions. Clin Pediatr (Phila) 2002;41:449-50. [Crossref] [PubMed]
- Christison-Lagay ER, Rodriguez L, Kurtz M, et al. Antegrade colonic enemas and intestinal diversion are highly effective in the management of children with intractable constipation. J Pediatr Surg 2010;45:213-9; discussion 219. [Crossref] [PubMed]
- Rodriguez L, Nurko S, Flores A. Factors associated with successful decrease and discontinuation of antegrade continence enemas (ACE) in children with defecation disorders: a study evaluating the effect of ACE on colon motility. Neurogastroenterol Motil 2013;25:140-e81. [Crossref] [PubMed]
- Heredia DJ, Dickson EJ, Bayguinov PO, et al. Colonic elongation inhibits pellet propulsion and migrating motor complexes in the murine large bowel. J Physiol 2010;588:2919-34. [Crossref] [PubMed]
- Bolia R, Kumar Bhat N. Redundant colon and refractory constipation in children. Indian J Gastroenterol 2020;39:521-2. [Crossref] [PubMed]
- Shin D, Lee KJ, Adeluwa T, et al. Machine Learning-Based Predictive Modeling of Postpartum Depression. J Clin Med 2020;9:2899. [Crossref] [PubMed]
- Zhu H, Wang G, Zheng J, et al. Preoperative prediction for lymph node metastasis in early gastric cancer by interpretable machine learning models: A multicenter study. Surgery 2022;171:1543-51. [Crossref] [PubMed]
- Lee MR, Sankar V, Hammer A, et al. Using Machine Learning to Classify Individuals With Alcohol Use Disorder Based on Treatment Seeking Status. EClinicalMedicine 2019;12:70-8. [Crossref] [PubMed]
- Liu Z, Sun L, Zhang Q. High Similarity Image Recognition and Classification Algorithm Based on Convolutional Neural Network. Comput Intell Neurosci 2022;2022:2836486. [Crossref] [PubMed]
- May M. Eight ways machine learning is assisting medicine. Nat Med 2021;27:2-3. [Crossref] [PubMed]
- Li DC, Liu CW. Extending attribute information for small data set classification. IEEE Trans Knowl Data Eng 2010;24:452-64.
- Conforti D, Guido R. Kernel based support vector machine via semidefinite programming: Application to medical diagnosis. Computers & Operations Research 2010;37:1389-94.
- Singh SJ, Gibbons NJ, Vincent MV, et al. Use of pelvic ultrasound in the diagnosis of megarectum in children with constipation. J Pediatr Surg 2005;40:1941-4. [Crossref] [PubMed]
- Chiarioni G, de Roberto G, Mazzocchi A, et al. Manometric assessment of idiopathic megarectum in constipated children. World J Gastroenterol 2005;11:6027-30. [Crossref] [PubMed]