Distinguishing diffuse large B-cell lymphoma from Hodgkin’s lymphoma in children using an enhanced computed tomography radiomics approach
Highlight box
Key findings
• Pathologic subtypes of pediatric lymphoma patients can be distinguished on enhanced computed tomography (CT) images using radiomics. This study sought to distinguish between two types of lymphomas that are similar on pathologic examination; that is, diffuse large B-cell lymphoma (DLBCL) and Hodgkin’s lymphoma (HL).
What is known, and what is new?
• Even on pathologic examination, it remains difficult to distinguish between DLBCL and HL in some cases, and accurate identification is costly. Lymphoma can be diagnosed by conventional imaging using a radiomics approach; however, to date, studies have largely focused on adult patients.
• This study confirmed that radiomics can also be used to identify DLBCL and HL, and well performing models can be established for pediatric patients.
What is the implication, and what should change now?
• Enhanced CT-based radiomics can be used to identify pathologic subtypes of pediatric lymphoma and provide additional imaging information in cases in which subtypes are difficult to identify.
Introduction
Lymphoma is one of the most common malignancies in children and adolescents (1-3). Lymphomas are characterized by complex pathological subtypes and different therapeutic principles (4-7). In clinical practice, the early and accurate diagnosis of subtypes of lymphoma is needed to adopt appropriate therapeutic strategies. Currently, pathologic biopsy is the only way to definitively diagnose the pathologic subtypes of lymphoma; however, even in pathologic biopsies, distinguishing between certain lymphoma subtypes is notoriously challenging, and in some cases, it is very difficult to differentiate between subtypes based on morphology alone, as is the case for Hodgkin’s lymphoma (HL) and diffuse large B-cell lymphoma (DLBCL).
For example, mesenchymal DLBCL cells may mimic HL cells and Reed-Sternberg (R-S) cells by presenting a multi-nucleated or macro-nucleated morphology (8). In immuno-morphology, nodular lymphocyte predominant Hodgkin’s lymphoma (NLPHL) may be similar to diffuse T-cell/histiocyte-rich large B-cell lymphoma (THRLBCL)/DLBCL, and there is a type of B-cell lymphoma in the World Health Organization’s classification of B-cell lymphomas (9) that cannot be classified and that has intermediate features of both DLBCL and HL, which suggests a biological overlap between these two subtypes. Further, a previous study has shown that a proportion of HLs have a tendency to transform to DLBCL (10), which raises the cost of diagnosing lymphoma subtypes by biopsy.
Radiological examination plays an important role in the early diagnosis, accurate staging, and efficacy evaluation of lymphoma (11-15). A previous study showed that traditional imaging and functional imaging also play a role in the diagnosis of lymphoma and identification of pathological subtypes (16); however, radiologists are still unable to differentiate between HL and DLBCL by comparing images. In recent years, radiomics has developed rapidly. This high-throughput, quantitative analysis method extracts features from a large number of images (17). Previous research studies have applied radiomics in the diagnosis, pathological staging, and prognosis prediction of patients with lymphoma (18-20); however, these studies have largely focused on adult patients, and there is a lack of research on how to distinguish between DLBCL and HL. Therefore, this study aimed to develop a model to differentiate between HL and DLBCL in pediatric patients using radiomics approach based on enhanced computed tomography (CT) images. We present this article in accordance with the TRIPOD reporting checklist (available at https://tp.amegroups.com/article/view/10.21037/tp-23-586/rc).
Methods
The study was conducted in accordance with the Declaration of Helsinki (as revised in 2013). The study was approved by the Ethics Committee of the Children’s Hospital of Chongqing Medical University (No. 386[2023], data 2023.8.20) and individual consent for this retrospective analysis was waived.
Patients
From November 2012 to February 2023, the clinical and imaging data of patients pathologically confirmed by the Children’s Hospital of Chongqing Medical University to have HL and DLBCL were collected. In each patient, multiple enlarged lymph nodes (≤4) were selected to be delineated. A total of 139 lymphomas were included in the analysis. To be eligible for inclusion in this study, the patients had to meet the following inclusion criteria: (I) have HL or DLBCL confirmed by core needle biopsy or excisional biopsy; (II) have undergone enhanced CT scanning and have clear images; (III) have not undergone any radiotherapy, chemotherapy, or other treatments prior to the enhanced CT scanning; and (IV) have an enlarged lymph node with a long axis ≥2 cm. Patients were excluded from the study if they met any of the following exclusion criteria: (I) had poor quality images or incomplete clinical and imaging data; (II) had undergone radiotherapy, chemotherapy, or other treatments prior to enhanced CT scanning; and/or (III) had lymphomas in the whole body with a long axis <2 cm.
In total, 50 HL patients with a total of 106 enlarged lymph nodes and 16 DLBCL patients with a total of 33 enlarged lymph nodes were included in the study. Of the patients, 50 were male and 16 were female. The median age of the patients was 8.09±3.52 years (range, 2–16 years).
CT image acquisition
Transverse-axis scanning was performed using a GE LightSpeed VCT 64-row spiral CT machine, or a Philips Brilliance iCT 256-row spiral CT machine (Philips, Amsterdam, Netherlands). Children who were unable to cooperate quietly were sedated using oral 10% chloral hydrate (0.5 mL/kg), or intramuscular phenobarbital sodium injection (5 mg/kg). The following scanning parameters were set: tube voltage: 80–110 kV; tube current adjustment: automatic, layer thickness: 5.0 mm; and layer spacing: 5 mm. Enhancement scans were performed with iohexol (350 mgI/mL, 2 mL/kg body mass) injected by regimentation through a forearm vein at a flow rate of 0.5–3.5 mL/s, and enhanced CT images were obtained at 50–60 s after contrast agent injection.
Image segmentation
The Digital Imaging and Communications in Medicine (DICOM)-format images of the patients’ enhanced CT imaging data were imported into ITK-SNAP post-processing software (www.itksnap.org), and the region of interest (ROI) was manually sketched layer by layer and synthesized into the volume of interest. Obvious enhanced vascular shadows and necrotic foci in the lesion were avoided as much as possible. The images were preprocessed in the uAI Portal (Version: 20230515) developed by Shanghai Lianyin Intelligent Medical Technology Co. Pre-processing, such as gray-scale discretization, image normalization, and image re-sampling, was employed to ensure that feature extraction was not affected by the different machines.
Data pre-processing
The data set was grouped into a test set (25%) and a training set (75%) by completely randomized grouping. It was evident that the two types of lymphomas in the training set were class imbalanced with the DLBCL׃HL ratio approaching 1׃4. This study used a multiple down-sampling method to address these effects by sampling 46 HLs randomly in the training set, and the HL to DLBCL ratio changed to 2׃1; thus, balancing the ratio of the two types of sample distributions. An experiment was performed to ensure that all the HL samples were taken (Figure 1). Put-back sampling was performed until all 84 HLs were sampled. The experiment was repeated more than 10,000 times to determine the minimum number of draws to use the full HL samples at the 95% confidence interval (CI). Ultimately, we conducted 12 experiments that used sampling with replacement, and also constructed 12 predictive models for DLBCL and HL and tested their performance using an independent testing set.
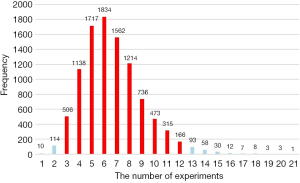
Feature extraction, feature selection, and classification methods
The uAI Portal (version 20230515) was used to automatically extract features. Among the 66 patients with a total of 139 lymphomas, 30 patients with a total of 59 lymphomas were randomly selected for the secondary ROI outlining work, and the radiomics features were then extracted to perform the intraclass correlation coefficient (ICC) calculations. An ICC value >0.75 was considered reproducible and robust. It also illustrated that the extracted features were not affected by the subjective observer factors. The Spearman rank correlation coefficient, maximum relevance and minimum redundancy, and least absolute shrinkage and selection operator algorithms were used sequentially in the training set to determine the feature combinations that were most relevant to predicting HL and DLBCL.
Due to the multiple down-sampling performed previously, the training set was split into 12 subsets. A quadratic discriminant analysis (QDA) and logistic regression (LR) analysis (the intercept, Wald’s Z, and P values are shown in Tables S1-S3) were chosen to build the sub-models (for detailed results, see Tables S1-S3). The LR model was optimized for parameters, such as C (1.0), penalty (L2), and tolerance (0.0001), while the QDA model was optimized for parameters, such as regularization (1.0) and tolerance (0.0001). The small sample size of each subset could not represent the final performance of the model, thus all the sub-models were ultimately ensembled. As mentioned above, 12 completely different sub-models were established. We assumed that these 12 sub-models had the same weight of 1, so the final composite model simply took the average of the predicted probabilities of the 12 sub-models, and the classification threshold was further determined to obtain its evaluation index.
Statistical analysis
SPSS 25.0 software was used to analyze the data. The chi-square test or Fisher’s exact test was used for the categorical variables, and the independent samples t-test was used for the continuous variables. A P value <0.05 was considered statistically significant. Confusion matrices were established, receiver operating characteristic (ROC) curves were applied, and areas under the curve (AUCs), sensitivity, and specificity were calculated to assess the discriminatory ability of the models. The Delong test was used to analyze differences in model efficacy, the Hosmer-Lemeshow test was used to examine the goodness-of-fit of the models, and the Brier score was used for the comprehensive evaluation.
Results
Patient characteristics
Details of the characteristics of the patients are set out in Table 1. Notably, weight loss and lactate dehydrogenase (LDH) were significant (with P values <0.05). The univariate and multivariate LR analyses in the training set revealed that LDH served as an independent predictor of HL and DLBCL in several experiments (Figure 2). Therefore, LDH was included as an available clinical feature in the analysis, and comprehensive models were constructed that also included the radiomic features using the same modeling methods described above.
Table 1
Characteristics | HL (n=50) | DLBCL (n=16) | P value |
---|---|---|---|
Gender | >0.99 | ||
Female | 12 (24.0) | 4 (25.0) | |
Male | 38 (76.0) | 12 (75.0) | |
Body part | >0.99 | ||
Head | 1 (2.0) | 2 (12.5) | |
Neck | 31 (62.0) | 4 (25.0) | |
Abdomen | 8 (16.0) | 9 (56.3) | |
Mediastinum | 9 (18.0) | 0 | |
Armpit | 1 (2.0) | 0 | |
Hilar | 0 | 1 (6.3) | |
Weight loss | 0.043 | ||
<10% | 23 (46.0) | 12 (75.0) | |
≥10% | 27 (54.0) | 4 (25.0) | |
Involve other part | 0.75 | ||
Yes | 29 (58.0) | 10 (62.5) | |
No | 21 (42.0) | 6 (37.5) | |
Involvement stage | 0.80 | ||
I | 0 | 1 (6.2) | |
II | 16 (32.0) | 6 (37.5) | |
III | 19 (38.0) | 7 (43.8) | |
IV | 15 (30.0) | 2 (12.5) | |
LDH class | 0.004 | ||
Abnormal | 8 (16.0) | 9 (56.2) | |
Normal | 42 (84.0) | 7 (43.8) | |
LDH (U/L) | 259.5 [228–287.225] | 323 [235–560.975] | 0.08 |
Age (years) | 8 [5–10] | 8.5 [6–10.5] | 0.41 |
Data are presented as n (%) or median [interquartile range]. DLBCL, diffuse large B-cell lymphoma; HL, Hodgkin’s lymphoma; LDH, lactate dehydrogenase.
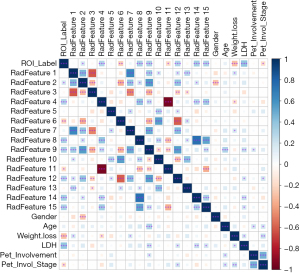
Feature selection results
In total, 2,264 radiomics features were extracted, including 104 original image features, and 2,160 filtered image features. Among them, the texture features included 21 gray-level co-occurrence matrix (GLCM) features, 14 gray-level dependence matrix (GLDM) features, 16 gray-level run length matrix (GLRLM) features, 18 gray-level size zone matrix (GLSZM) features and 5 neighborhood gray-tone difference matrix (NGTDM) features. Among the selected radiomics features in the 12 training data sets, 15 features had a frequency of occurrence >2 (Figure 3), of which 9 showed statistically significant differences between DLBCL and HL based on the t-test results.
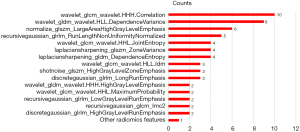
Model building and evaluating
Differentiation models for lymphoma subtypes based on radiomic features, and radiomic features + LDH were developed. The training set was divided into 12 subsets for training and validation as described above, and the following four models were then ensembled: Radiomics_LR, Radiomics_QDA, Radiomics + LDH_LR, and Radiomics + LDH_QDA. Using the four model test sets, the results were as follows: Radiomics_LR: AUC =0.814 (95% CI: 0.628–0.999), sensitivity: 0.800, and specificity: 0.864; Radiomics + LDH_LR: AUC =0.768 (95% CI: 0.580–0.956); sensitivity: 0.500; and specificity: 0.955; Radiomics_QDA: AUC =0.841 (95% CI: 0.691–0.991); sensitivity: 0.900; and specificity: 0.773; Radiomics + LDH_QDA: AUC =0.845 (95% CI: 0.695–0.996); sensitivity: 0.900; and specificity: 0.773 (Table 2). The ROC curve of each model test set and the confusion matrix are shown in Figure 4. The results of the Delong, Hosmer-Lemeshow, and Brier score tests are shown in Tables 3,4. To further accurately compare the discriminative efficacy and goodness-of-fit of the four models (Figure 5), calibration curves and decision curves were depicted.
Table 2
Model name | Cut off | AUC (95% CI) | Sensitivity | Specificity | Accuracy | Precision | F1-score |
---|---|---|---|---|---|---|---|
Radiomics_LR | 0.357 | 0.814 (0.628–0.999) | 0.800 | 0.864 | 0.844 | 0.727 | 0.762 |
Radiomics + LDH_LR | 0.504 | 0.768 (0.580–0.956) | 0.500 | 0.955 | 0.813 | 0.833 | 0.625 |
Radiomics_QDA | 0.242 | 0.841 (0.691–0.991) | 0.900 | 0.773 | 0.813 | 0.643 | 0.750 |
Radiomics + LDH_QDA | 0.220 | 0.845 (0.695–0.996) | 0.900 | 0.773 | 0.813 | 0.643 | 0.750 |
AUC, area under curve; CI, confidence interval; LR, logistic regression; LDH, lactate dehydrogenase; QDA, quadratic discriminant analysis.
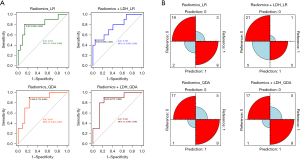
Table 3
Model name | Radiomics_LR | Radiomics + LDH_LR | Radiomics_QDA | Radiomics + LDH_QDA |
---|---|---|---|---|
Radiomics_LR | 1.000 | 0.420 | 0.567 | 0.516 |
Radiomics + LDH_LR | 0.420 | 1.000 | 0.321 | 0.274 |
Radiomics_QDA | 0.567 | 0.321 | 1.000 | 0.732 |
Radiomics + LDH_QDA | 0.516 | 0.274 | 0.732 | 1.000 |
LR, logistic regression; LDH, lactate dehydrogenase; QDA, quadratic discriminant analysis.
Table 4
Model name | Hosmer-Lemeshow test | Brier score |
---|---|---|
Radiomics_LR | 0.647 | 0.001 |
Radiomics + LDH_LR | 0.225 | 0.016 |
Radiomics_QDA | 0.325 | 0.016 |
Radiomics + LDH_QDA | 0.390 | 0.016 |
LR, logistic regression; LDH, lactate dehydrogenase; QDA, quadratic discriminant analysis.
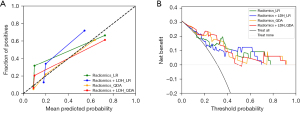
Testing for patients with lymphoma
In the previous section, we evaluated the established models with lymphomas. It should be noted that multiple lymphomas in this research might have come from the same patient. To understand the ability of each model to predict the type of lymphoma in a patient, each patient in the test set was validated. Figure 6 shows the confusion matrix.
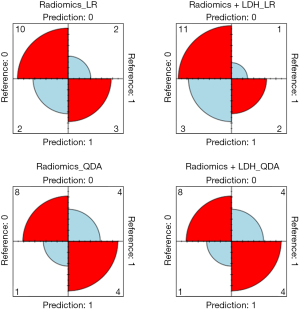
Discussion
DLBCL is a type of non-HL, and the treatment options for DLBCL are very different to those for HL. Thus, the accurate diagnosis and differentiation of DLBCL and HL have important clinical value. Enhanced CT is the most common imaging examination for patients before lymphoma treatment. On CT images, patients with DLBCL tend to show enlarged lymph nodes in the neck and mediastinum compared to patients with HL. Patients with DLBCL are also more likely to show signs of vascular compression, airway compression, and bowel obstruction (14).
CT plays an important role in the diagnosis and staging of tumors; however, it is still difficult to distinguish between DLBCL and HL on enhanced CT, as both appear as mildly enhanced masses. Previous research has shown that functional imaging methods, including functional magnetic resonance imaging (fMRI), and positron emission tomography (PET)-CT can also be used to identify lymphoma subtypes. Using PET-CT findings, Barber et al. (21) confirmed that the standard uptake values (SUVs) of patients with NLPHL were higher than those of patients with THRLBCL. Cottereau et al. (22) demonstrated that iron deposition was more likely to be detected in advanced HL and DLBCL than follicular lymphoma (FL) on whole-body diffusion-weighted MRI. However, the above examination methods are time consuming, expensive, and not easily accessible.
Pathologic biopsy remains the most accurate method for diagnosing lymphoma subtypes, but it has limitations. Notably, there is a biological overlap between DLBCL and HL, and thus it remains difficult to distinguish between the two subtypes in some cases. Pathologic biopsy is currently the only way to definitively diagnose lymphoma; however, biopsy is invasive, and thus very harmful to pediatric patients. Additionally, it is difficult to resect tumors located in the mediastinum, and it is not possible to take samples from every suspected lymph node.
Previous studies have shown that radiomics methods can be widely applied to differentiate lymphomas from other tumor lesions in different parts of the body. Radiomics models in the head and neck (23), oropharynx (24), intracranium (25), liver (26), and stomach (27) have demonstrated good discriminatory ability. Wu et al. (28) showed that radiomics can also recognize the different pathologies of lymphomas, and enhanced T1-weighted images in 3.0 T MRI can distinguish between DLBCL and FL based on three texture analyses of the gray-level run-length matrix with AUCs of 0.800, 0.798, and 0.798, respectively. de Jesus et al. (29) extracted features in co-aligned [18F]fluorodeoxyglucose (FDG) PET and CT images to distinguish DLBCL from FL. The performance of the model constructed based on radiomics features was compared with that of a LR model based on the SUVmax. The radiomics model had an AUC of 0.86 with an accuracy of 80%, and the SUV-based LR model had an AUC of 0.79 with an accuracy of 70%. The results showed that a texture analysis can reveal microscopic features of tumors that are difficult to see with the naked eye, and using this method, it is possible to distinguish between different subtypes of lymphoma. The above studies clearly illustrate the potential of radiomics in the study of lymphoma diseases, but the ability to accurately differentiate between DLBCL and HL in augmented CT-based radiomics models had not previously been examined. Thus, this study sought to examine whether radiomics could reveal the histological differences between DLBCL and HL.
In this study, a total of 2,264 radiomics features were extracted from 12 subsets, and it was discovered that LDH could serve as a clinical indicator in the analysis. Two classifiers (i.e., LR and QDA) were applied to model the radiomics features and clinical features. The Radiomics + LDH_QDA model had the highest AUC value in the results, but based on the DeLong test results, the AUC value of that model was not significantly better than those of the other three models. Similarly, in terms of the performance of the four models on the calibration curves, the Hosmer-Lemeshow test and the Brier scores revealed that the radiomics models built on LR classifiers performed better (P=0.64, Brier score =0.001) than the other three models. The radiomics model based on the LR classifier performed the best, and had the highest specificity, accuracy, precision, and F1-score, and a sensitivity that did not fall below 0.8. Finally, the confusion matrix indicated that the Radiomics_LR model based on enhanced CT was better able to distinguish DLBCL from HL.
Unlike previous radiomics studies on lymphoma, after adding LDH to build a comprehensive model of radiomics and clinical characteristics, the discriminative power of the model did not improve significantly. The correlation analysis showed that while there was a significant correlation between LDH and ROI_Label, there was not a high correlation between LDH and the other 15 most frequently occurring radiomics features. The reason for this result could be the small sample size; however, it should be noted that DLBCL is rare in pediatric lymphoma patients (30).
In the test for lymphoma patients, we obtained different results. In terms of the confusion matrix, LR had a higher predictive efficacy for HL (LR vs. QDA: 83.3% vs. 66.7%), and QDA had a higher predictive efficacy for DLBCL (LR vs. QDA: 60.0% vs. 80.0%), suggesting that LR and QDA have their own strengths in identifying the two types of patients with DLBCL and HL. While it is rare in pediatric patients, the incidence of DLBCL increases with age (6). Modeling with the QDA classifier in DLBCL patients in older children and adolescents in future studies may yield better results.
This study had some limitations. First, the sample size of the obtained imaging data was small. Small data sets often show signs of overfitting. In this study, the LR model showed slight overfitting, but the QDA model did not show overfitting. Second, the data used in this study were all collected from a single hospital, and thus external validation was lacking. Third, it was impossible to realize the precise correspondence between the pathological results and imaging manifestations. Despite the adoption of strict lymph node selection criteria, it was impossible to sample all enlarged lymph nodes during the biopsy of lymphoma patients, resulting in a lack of pathological confirmation of some lymphomas. Although the performance of the models in this study is not sufficient for clinical application, this study confirmed that CT-based radiomics can be used to identify different subtypes of childhood lymphoma. In the future, multicenter, multimodal, and prospective related studies can be conducted, and studies of imaging genomics can be carried out to increase the sample size or the amounts of features to establish a model with higher predictive efficacy.
Conclusions
In summary, radiomics modeling based on enhanced CT can be used to identify DLBCL and HL. The Radiomics_LR model had the strongest discriminatory ability. Radiomics addresses the shortcomings of traditional imaging methods and provides more imaging information in cases in which subtypes are difficult to identify in the clinic.
Acknowledgments
Funding: This study was supported by funding from
Footnote
Reporting Checklist: The authors have completed the TRIPOD reporting checklist. Available at https://tp.amegroups.com/article/view/10.21037/tp-23-586/rc
Data Sharing Statement: Available at https://tp.amegroups.com/article/view/10.21037/tp-23-586/dss
Peer Review File: Available at https://tp.amegroups.com/article/view/10.21037/tp-23-586/prf
Conflicts of Interest: All authors have completed the ICMJE uniform disclosure form (available at https://tp.amegroups.com/article/view/10.21037/tp-23-586/coif). F.W. is a current employee of Shanghai United Image Intelligent Medical Technology Co., Ltd. The other authors have no conflicts of interest to declare.
Ethical Statement: The authors are accountable for all aspects of the work in ensuring that questions related to the accuracy or integrity of any part of the work are appropriately investigated and resolved. The study was conducted in accordance with the Declaration of Helsinki (as revised in 2013). The study was approved by the Ethics Committee of the Children’s Hospital of Chongqing Medical University (No. 386[2023], data 2023.8.20) and individual consent for this retrospective analysis was waived.
Open Access Statement: This is an Open Access article distributed in accordance with the Creative Commons Attribution-NonCommercial-NoDerivs 4.0 International License (CC BY-NC-ND 4.0), which permits the non-commercial replication and distribution of the article with the strict proviso that no changes or edits are made and the original work is properly cited (including links to both the formal publication through the relevant DOI and the license). See: https://creativecommons.org/licenses/by-nc-nd/4.0/.
References
- Connors JM, Cozen W, Steidl C, et al. Hodgkin lymphoma. Nat Rev Dis Primers 2020;6:61. Erratum in: Nat Rev Dis Primers 2021;7:79. [Crossref] [PubMed]
- El-Mallawany NK, Alexander S, Fluchel M, et al. Children's Oncology Group's 2023 blueprint for research: Non-Hodgkin lymphoma. Pediatr Blood Cancer 2023;70:e30565. [Crossref] [PubMed]
- Miller KD, Fidler-Benaoudia M, Keegan TH, et al. Cancer statistics for adolescents and young adults, 2020. CA Cancer J Clin 2020;70:443-59. [Crossref] [PubMed]
- Flerlage JE, Hiniker SM, Armenian S, et al. Pediatric Hodgkin Lymphoma, Version 3.2021. J Natl Compr Canc Netw 2021;19:733-54. [Crossref] [PubMed]
- Belsky JA, Hochberg J, Giulino-Roth L. Diagnosis and management of Hodgkin lymphoma in children, adolescents, and young adults. Best Pract Res Clin Haematol 2023;36:101445. [Crossref] [PubMed]
- Metzger ML, Mauz-Körholz C. Epidemiology, outcome, targeted agents and immunotherapy in adolescent and young adult non-Hodgkin and Hodgkin lymphoma. Br J Haematol 2019;185:1142-57. [Crossref] [PubMed]
- Derebas J, Panuciak K, Margas M, et al. The New Treatment Methods for Non-Hodgkin Lymphoma in Pediatric Patients. Cancers (Basel) 2022;14:1569. [Crossref] [PubMed]
- Li S, Young KH, Medeiros LJ. Diffuse large B-cell lymphoma. Pathology 2018;50:74-87. [Crossref] [PubMed]
- Alaggio R, Amador C, Anagnostopoulos I, et al. The 5th edition of the World Health Organization Classification of Haematolymphoid Tumours: Lymphoid Neoplasms. Leukemia 2022;36:1720-48. Erratum in: Leukemia 2023;37:1944-51.
- Kenderian SS, Habermann TM, Macon WR, et al. Large B-cell transformation in nodular lymphocyte-predominant Hodgkin lymphoma: 40-year experience from a single institution. Blood 2016;127:1960-6. [Crossref] [PubMed]
- Kwok HM, Ng FH, Chau CM, et al. Multimodality imaging of extra-nodal lymphoma in the head and neck. Clin Radiol 2022;77:e549-59. [Crossref] [PubMed]
- Spijkers S, Littooij AS, Kwee TC, et al. Whole-body MRI versus an FDG-PET/CT-based reference standard for staging of paediatric Hodgkin lymphoma: a prospective multicentre study. Eur Radiol 2021;31:1494-504. [Crossref] [PubMed]
- Ko KWS, Bhatia KS, Ai QYH, et al. Imaging of head and neck mucosa-associated lymphoid tissue lymphoma (MALToma). Cancer Imaging 2021;21:10. [Crossref] [PubMed]
- McCarten KM, Nadel HR, Shulkin BL, et al. Imaging for diagnosis, staging and response assessment of Hodgkin lymphoma and non-Hodgkin lymphoma. Pediatr Radiol 2019;49:1545-64. [Crossref] [PubMed]
- Morakote W, Baratto L, Ramasamy SK, et al. Comparison of diffusion-weighted MRI and [18F]FDG PET/MRI for treatment monitoring in pediatric Hodgkin and non-Hodgkin lymphoma. Eur Radiol 2024;34:643-53. [Crossref] [PubMed]
- Ando T, Kato H, Matsuo M. Different CT imaging findings between histological subtypes in patients with primary thyroid lymphoma. Radiol Med 2022;127:191-8. [Crossref] [PubMed]
- Gillies RJ, Kinahan PE, Hricak H. Radiomics: Images Are More than Pictures, They Are Data. Radiology 2016;278:563-77. [Crossref] [PubMed]
- Zhao YT, Chen SY, Liu X, et al. Risk stratification and prognostic value of multi-modal MRI-based radiomics for extranodal nasal-type NK/T-cell lymphoma. BMC Cancer 2023;23:88. [Crossref] [PubMed]
- Enke JS, Moltz JH, D'Anastasi M, et al. Radiomics Features of the Spleen as Surrogates for CT-Based Lymphoma Diagnosis and Subtype Differentiation. Cancers (Basel) 2022;14:713. [Crossref] [PubMed]
- Dong C, Zheng YM, Li J, et al. A CT-based radiomics nomogram for differentiation of squamous cell carcinoma and non-Hodgkin's lymphoma of the palatine tonsil. Eur Radiol 2022;32:243-53. [Crossref] [PubMed]
- Barber NA, Loberiza FR Jr, Perry AM, et al. Does functional imaging distinguish nodular lymphocyte-predominant hodgkin lymphoma from T-cell/histiocyte-rich large B-cell lymphoma? Clin Lymphoma Myeloma Leuk 2013;13:392-7. [Crossref] [PubMed]
- Cottereau AS, Mulé S, Lin C, et al. Whole-Body Diffusion-weighted MR Imaging of Iron Deposits in Hodgkin, Follicular, and Diffuse Large B-Cell Lymphoma. Radiology 2018;286:560-7. [Crossref] [PubMed]
- Seidler M, Forghani B, Reinhold C, et al. Dual-Energy CT Texture Analysis With Machine Learning for the Evaluation and Characterization of Cervical Lymphadenopathy. Comput Struct Biotechnol J 2019;17:1009-15. [Crossref] [PubMed]
- Bae S, Choi YS, Sohn B, et al. Squamous Cell Carcinoma and Lymphoma of the Oropharynx: Differentiation Using a Radiomics Approach. Yonsei Med J 2020;61:895-900. [Crossref] [PubMed]
- Yun J, Park JE, Lee H, et al. Radiomic features and multilayer perceptron network classifier: a robust MRI classification strategy for distinguishing glioblastoma from primary central nervous system lymphoma. Sci Rep 2019;9:5746. [Crossref] [PubMed]
- Xu H, Zou X, Zhao Y, et al. Differentiation of Intrahepatic Cholangiocarcinoma and Hepatic Lymphoma Based on Radiomics and Machine Learning in Contrast-Enhanced Computer Tomography. Technol Cancer Res Treat 2021;20:15330338211039125. [Crossref] [PubMed]
- Feng B, Huang L, Liu Y, et al. A Transfer Learning Radiomics Nomogram for Preoperative Prediction of Borrmann Type IV Gastric Cancer From Primary Gastric Lymphoma. Front Oncol 2021;11:802205. [Crossref] [PubMed]
- Wu X, Sikiö M, Pertovaara H, et al. Differentiation of Diffuse Large B-cell Lymphoma From Follicular Lymphoma Using Texture Analysis on Conventional MR Images at 3.0 Tesla. Acad Radiol 2016;23:696-703. [Crossref] [PubMed]
- de Jesus FM, Yin Y, Mantzorou-Kyriaki E, et al. Machine learning in the differentiation of follicular lymphoma from diffuse large B-cell lymphoma with radiomic [18F]FDG PET/CT features. Eur J Nucl Med Mol Imaging 2022;49:1535-43. [Crossref] [PubMed]
- Mason EF, Kovach AE. Update on Pediatric and Young Adult Mature Lymphomas. Clin Lab Med 2021;41:359-87. [Crossref] [PubMed]
(English Language Editor: L. Huleatt)