Clinical relevance of short-term glycemic variability in children and adolescents with type 1 diabetes: a narrative review
Introduction
Background
Type 1 diabetes (T1D) is a chronic autoimmune disease characterized by the destruction of pancreatic β-cells, leading to insulin deficiency and requiring lifelong insulin substitutive therapy (1). It affects millions of individuals worldwide, with a substantial portion being diagnosed during childhood and adolescence (2).
Managing T1D in youth poses unique challenges, primarily related to the developmental, emotional, and physiological changes that characterize growth and maturation. The primary objective of T1D management in pediatric age is to prevent associated morbidity and mortality by maintaining glucose levels as close as possible to the euglycemic range (3). The preventing role of tight glycemic control on long-term complications has been recognized for decades, since the Diabetes Control and Complications Trial (DCCT) revealed a direct correlation between the risk of diabetes-related complications—including retinopathy, nephropathy, neuropathy, and macrovascular disease—and glycated hemoglobin A1C (HbA1c) levels (4). HbA1c, the most widely adopted marker for assessing longitudinal glucose control in individuals with diabetes, is strongly influenced by the concentration of blood glucose over the preceding 8 to 12 weeks (5). International guidelines for children and adolescents with T1D set a target HbA1c below 7% to define satisfactory glucose control (5).
The widespread adoption of continuous glucose monitoring (CGM) systems in clinical practice has provided more detailed insights into daily glucose patterns of individuals with T1D than the sole HbA1c levels. Glycemic variability can be classified as short-term, consisting of daily oscillations in blood glucose levels—including both their amplitude and frequency—and long-term, referring to variations in longitudinal glucose control markers such as HbA1c. In recent years, short-term glycemic variability has garnered increasing interest among the scientific community, particularly regarding its potential implication as an independent risk factor for long-term complications.
Rationale and knowledge gap
Understanding glycemic variability in pediatric T1D is crucial for several reasons. Firstly, children and adolescents frequently experience glucose excursions due to factors such as growth spurts, varying physical activity levels, dietary habits, and hormonal changes associated with puberty. Achieving recommended targets of glycemic variability is challenging in this population, even with the use of advanced therapeutic tools such as second-generation automated insulin delivery systems (6,7). Secondly, the impact of glycemic variability on long-term outcomes, including the development of microvascular and macrovascular complications, remains unclear and is object of ongoing research and debate (8) (Figure 1).
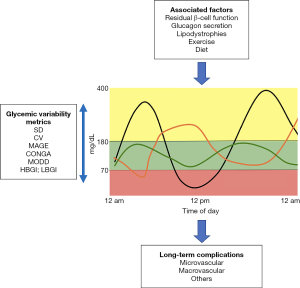
Objective
This narrative review aimed to provide a comprehensive overview of glycemic variability in pediatric individuals with T1D. By reviewing existing literature, we explored measurement methodologies, clinical factors contributing to glycemic variability in the pediatric population, and evidence regarding its association with long-term complications. We present this article in accordance with the Narrative Review reporting checklist (available at https://tp.amegroups.com/article/view/10.21037/tp-24-114/rc).
Methods
We performed a comprehensive search of published literature using the PubMed MEDLINE database from January 2010 to January 2024. We used the following combination of search terms: “glycemic variability”, “pediatric”, “type 1 diabetes”, and “children”. The literature search was conducted equally by all co-authors, and any disagreements were resolved by G.S.
Non-English language papers, case reports, and editorials were excluded. Particular emphasis was placed on randomized controlled studies, observational studies, systematic reviews, and meta-analyses.
Studies involving adult populations on specific topics with scarce pediatric data, such as measurement methods and long-term complications, were also considered. The search strategy is summarized in Table 1. A total of 71 papers (4 literature reviews, 3 guidelines, 10 randomized controlled trials, and 54 observational studies) were included in the review.
Table 1
Items | Specification |
---|---|
Date of search | 31 January 2024 |
Databases and other sources searched | PubMed MEDLINE |
Search terms used | “Glycemic variability”, “Pediatric”, “Type 1 diabetes”, “Children” |
Timeframe | January 2010–January 2024 |
Inclusion and exclusion criteria | Inclusion: meta-analyses, systematic reviews, randomized controlled studies, and observational studies |
Exclusion: non-English language papers, case reports, editorials | |
Selection process | All authors worked independently on the selection of papers. Disagreements between authors were resolved by discussion and consensus |
Glycemic variability assessment
In recent years, CGM systems have emerged as the standard for monitoring children and adolescents with T1D (5). These wearable devices offer real-time measurements of interstitial glucose levels, providing valuable insights into average daily glucose trends and time spent within, above, and below the target glycemic range, allowing the prompt identification of the duration and extent of hypo- and hyperglycemia.
Furthermore, CGM facilitate accurate retrospective analysis of daily glucose fluctuations, offering insights into the amplitude of sensor glucose peaks and valleys and their frequency. However, quantifying glucose variability remains challenging due to the asymmetry of the blood glucose measurement scale, where the hypoglycemic range is significantly narrower than the hyperglycemic range, rendering excursions in glucose levels within the hypoglycemic range clinically more significant (9).
Moreover, obtaining a reliable estimate of glucose variability necessitates CGM data over a sufficient time window. An analysis of CGM data from children and adolescents with T1D suggested a minimum interval of twelve days for assessing glucose variability, with no more than four hours between each glucose measurement (10). Conversely, Piona et al. found that a 4-week dataset better reflects glucose variability among intermittently scanned CGM users, while a 2-week period can provide acceptable accuracy in real-time CGM users (11).
Several CGM metrics quantifying glycemic variability have been developed over the years (Table 2). The standard deviation (SD) of sensor glucose is the most immediate metric, easily calculated by CGM systems alongside the mean sensor glucose. This indicator has demonstrated a good capability to assess the stability of glucose control due to the positive correlation between SD and HbA1c (12). However, its main limitation lies in the non-normal distribution of blood glucose values (13).
Table 2
Metric | Description | Advantages | Disadvantages |
---|---|---|---|
SD | Variation of sensor glucose | Easily calculated | Typical non-normal distribution of blood glucose values |
CV | SD/mean glucose ×100 | Easily calculated; reliable predictor of hypoglycemia risk; correlation with TIR and TITR | Disparity between within-day CV and total CV; low discriminant ratio |
MAGE | Calculated from excursions of sensor glucose exceeding 1 SD | Assessment of glycemic amplitude | Lack of information about frequency; complexity of calculation |
CONGA | Measurement of duration and degree of glucose fluctuations | Assessment of the frequency of glucose variations | Complexity of calculation |
MODD | Mean of absolute differences between glucose values measured at the same time of day on two consecutive days | Assessment of between-day glucose variability | Lack of information about within-day variability; complexity of calculation |
HBGI, LBGI | Risk indexes of hypo- and hyperglycemia, calculated on logarithmic basis | Provide useful information about hypo- and hyperglycemia risk | Overlap with other metrics |
ADRR | Evaluates glycemic variability and classifies risk of hypoglycemia and hyperglycemia | Can be calculated also in individuals on self-monitoring of blood glucose | Further validation is needed in youth with type 1 diabetes |
SD, standard deviation; CV, coefficient of variation; MAGE, mean amplitude of glycemic excursion; CONGA, continuous overlapping net glycemic action; MODD, mean of daily differences; HBGI, high blood glucose index; LBGI, low blood glucose index; ADRR, average daily risk range; TIR, time in range; TITR, time in tight range.
The coefficient of variation (CV) is the most commonly adopted indicator of glycemic variability in clinical practice and experimental studies. It is calculated by dividing SD by sensor mean glucose. The international consensus for CGM data interpretation defined CV as the primary indicator of glycemic variability due to its relative sensitivity to hypoglycemia compared to SD alone, and its ease of calculation (14). CV has been identified as a significant predictor of hypoglycemia, particularly of time spent with glucose <54%, compared to other CGM metrics (15-17). Furthermore, several studies have investigated the relationship between CV and other glucose control indicators (18). A cross sectional study on 195 individuals with T1D found a strong correlation between CV and time in range (TIR) 70–180 mg/dL (19), while a pediatric study on 854 CGM users identified CV as a predictor of longer time in tight range, a novel metric assessing the time spent in narrower range between 70 and 140 mg/dL (20). A subanalysis of data from five different phase 3 trials demonstrated a significant influence of within-day CV on HbA1c and daily mean glucose (21). Conversely, some studies have revealed an absent or only partial relationship between CV and HbA1c (16,18), while Castañeda et al. defined CV as a poor marker of hypoglycemia risk based on data from 10,404 advanced hybrid closed-loop users (22). Based on literature data, a target of 36% has been suggested to define stable glucose control (14). However, this target refers to the within-day CV, while most CGM platforms automatically calculate the total CV of the analyzed time frame. This disparity represents a limit to the interpretation of glycemic variability in clinical practice, as evidenced by a retrospective study on 104 subjects with T1D, where 21% exhibited a substantial discrepancy between within-day and total CV (23). Moreover, the threshold of 36% might be inadequate to ensure low hypoglycemia, as indicated by data from 1,002,946 intermittently-scanned CGM readers, showing that achieving the recommended target of <1% time below 54 mg/dL corresponds to a within-day CV below 33.5% (24). As an additional limit, a subanalysis of the Juvenile Diabetes Research Foundation dataset revealed that CV has the lowest discriminant ratio among all glycemic variability metrics, indicating poor ability to detect individual variation within a population (25).
The mean amplitude of glycemic excursion (MAGE) focuses on the width of glycemic variations and is calculated from excursions of sensor glucose exceeding 1 SD. Generally, a 2-day interval of CGM data is sufficient to extrapolate MAGE (26). Despite being a promising marker of glucose variability, its reliability is limited by the lack of information about the time taken by glucose values to fluctuate and excursions <1 SD (27).
Additional glucose variability metrics include the continuous overlapping net glycemic action (CONGA), which accounts for the frequency of glucose levels variations, the mean of daily differences (MODD), assessing between-day glucose variability, and indexes of the risk of hypo- and hyperglycemia (e.g., High Blood Glucose Index, Low Blood Glucose Index, Glycemia Risk Index) (28,29). While useful in characterizing different patterns of glucose variations, their complexity of calculation and overlap with other metrics limit their application in clinical practice (30). Additionally, there is currently no consensus on recommended targets. Another valuable metric for evaluating glycemic variability and classifying the risk of hypoglycemic and hyperglycemic episodes is the average daily risk range (ADRR), particularly for individuals conducting self-monitoring of blood glucose with a minimum of 3 measurements per day (31).
Associated factors
Glucose homeostasis is intricately regulated by hormonal pathways involving pancreatic islets, the liver, the gut, and the nervous system. In healthy individuals, these regulators respond to physiological conditions such as fasting, food intake, and physical activity to maintain stable glucose levels (9). In individuals with T1D, this complex homeostasis is seriously compromised due to insulin deficiency. Additionally, exogenous insulin therapy may itself induce unwanted glucose fluctuations, leading to patient exposure to hypoglycemia.
The poor or absent residual β-cell function typical of individuals with T1D results in marked glycemic variability compared to other types of diabetes. For instance, when comparing CGM data between youths with T1D and those with Wolfram syndrome—a genetic syndrome causing non-autoimmune diabetes—the former consistently exhibit worse glycemic variability metrics (32). Supporting the relationship between residual β-cell function and glucose variability, a longitudinal study on 78 newly diagnosed children with T1D found increased glycemic stability among individuals undergoing partial remission phase (33). Additionally, post-hypoglycemic hyperglycemia, a pattern strongly correlated with glycemic variability, has been identified as a reliable marker of partial remission phase (34).
However, β-cell secretion may not be the sole process involved in the disruption of glucose homeostasis for people with T1D. According to a secondary analysis on 28 participants of the Diabetes Research in Children Network study, impaired glucagon secretion in response to insulin-induced hypoglycemia may be associated to worse CV and CONGA values (35).
Glycemic variability can be also correlated to the concomitant presence of T1D and metabolic syndrome. This association, which has become unfortunately common due to the increasing trend of obesity in Western countries, adds the mechanism of insulin resistance to the already compromised glucose homeostasis in T1D. Notably, a multicenter cross-sectional study involving 207 adults showed higher CV levels in subjects with T1D associated to metabolic syndrome (36). However, adiposity seems to be positively associated with time spent in hyperglycemia but not with glycemic variability metrics in youths with T1D (37).
Skin issues related to diabetes management may hinder the achievement of satisfactory glucose control (38). Insulin-induced lipodystrophies are the most common dermatological complications in individuals with T1D. By altering the regular absorption of insulin in the subcutaneous tissue, these skin lesions have been identified as potential determinants of glycemic excursions (39). A correlation between the presence of lipodystrophy and higher CV and SD of sensor glucose was detected in a cohort of 212 children and adolescents with T1D (40). Similarly, Gupta et al. reported higher MAGE and CONGA among subjects injecting insulin at lipohypertrophy sites (41).
Younger children with T1D are well-known to experience high rates of glycemic excursions. Achieving recommended targets of CV and other glucose control indicators in this age group remains challenging, likely due to dietary habits and underlying hormonal factors affecting insulin sensitivity. To support this theory, an analysis of 4-week CGM data of 107 youths with T1D showed that pre-pubertal children have higher values of glucose SD and CV compared to pubertal and post-pubertal (42).
Physical activity is widely acknowledged as one of the main reasons for glycemic fluctuations in people with T1D, with the type and duration of exercise responsible for distinct glucose patterns. Aerobic activity, defined as prolonged exercise with low-to-moderate intensity, is associated with an increased risk of hypoglycemia (43). Conversely, anaerobic high-intensity exercise can be associated with acute hyperglycemia (44). Studies combining CGM and accelerometry data found that physical activity leads to a higher risk of nocturnal hypoglycemia, with an increase of 60–80% every 60 minutes of activity (45,46). Additionally, there was a 31% increased risk of hypoglycemia among adolescents with more than 30 minutes of moderate-to-vigorous physical activity (46). In contrast to this evidence, a real-world analysis on ten adolescents with T1D practicing their usual physical activity over a 14-day period, showed an inverse correlation between the total amount of moderate and vigorous physical activity and overall glucose variability metrics, including CV and SD (47), while Rebesco et al. did not detect any difference in CV, SD, and MAGE between physically active and sedentary days (48). Similar results emerged from a cohort of preschoolers, where moderate-to-vigorous physical activity appeared to negatively affect parental fear of hypoglycemia, without any significant correlation with glycemic variability (49). These data suggest an overall benefit of regular exercise on glycemic stability, with the advantages of a good level of fitness exceeding the disadvantages of the risk of hypo- and hyperglycemia during and after exercise. In support of this hypothesis, a study on nineteen adolescents with T1D revealed an inverse association between the level of aerobic fitness, measured by maximal aerobic capacity (VO2max), and MAGE (50).
Among lifestyle habits, even sleep characteristics seem to influence the daily glucose level variability. A cross-sectional study on 84 children with T1D found that poor sleep efficiency, longer sleep onset latency, and nocturnal wake duration are significantly correlated with higher overnight glycemic variability (51).
Nutrition plays a fundamental role in the management of T1D, with healthy and balanced diets being crucial for achieving optimal glucose control. It is well-established that the quality and the balance of macronutrients have a substantial impact on postprandial glycemic control and overall glucose variability. In a real-world study on 208 youth with T1D taking pictures of each meal over a 10-day observation period to allow an accurate evaluation of macronutrient composition, a higher glycemic variability assessed by CV and glucose SD was observed following meals with more carbohydrates in comparison to fat- and protein-based meals (52). Similar results were obtained from a cohort of preschoolers, with greater postprandial glucose variability recorded after meals with high carbohydrate content (53). Nevertheless, fats and proteins are also known to have an effect on postprandial glycemic variability, manifesting as late hyperglycemia 3–6 hours after the meal (54,55). Alongside the overall macronutrient intake, an accurate carbohydrate counting is crucial to improve glycemic control, especially among users of automated insulin delivery systems. Brazeau et al. demonstrated that inaccurate carbohydrate counting is a predictor of higher glycemic variability measured by MAGE and SD (56).
Gluten-free foods, known to have high glycemic index and low-fiber content, can exacerbate glycemic excursions in subjects with concomitant T1D and celiac disease. In support of this hypothesis, a pediatric case-control study found that gluten-free diet is associated with greater glycemic excursions in youths with T1D and celiac disease (57). Conversely, Mozzillo et al. reported similar glucose metrics between children with T1D and celiac disease and those with T1D only, except for individuals who were not strictly adhering to a gluten-free diet, who presented higher hyperglycemia levels (58).
Glycemic variability and long-term outcomes
In addition to the established relationship between glycemic variability and the risk of acute complications of diabetes, such as severe hypoglycemia (59), the role of glucose excursions as independent risk factor for long-term complications has garnered significant interest among researchers in recent years.
Long-term complications of T1D consist of microvascular damage, including retinopathy, neuropathy, and nephropathy, as well as macrovascular diseases. These complications usually develop insidiously, and clinical signs may occur several years after the onset of vascular damage (60). Hence, regular screening procedures and tailored therapeutic interventions aimed at achieving tight glycemic control become imperative, especially during pediatric age.
Since the DCCT and the Epidemiology of Diabetes and Interventions and Complications (EDIC) study (4,61), HbA1c has been recognized as a predictor of long-term complications and has been considered the gold standard for assessing glycemic control (5). However, subsequent data have suggested that HbA1c and average glucose levels may not be the sole determinants of diabetes-related long-term complications (62).
In recent years, the relationship between glycemic variability and the occurrence of complications has been widely investigated, with controversial findings. In a systematic review of the literature, the role of glycemic variability emerged as irrelevant for the development of complications in T1D populations (63). A post-hoc analysis of DCCT data, based on capillary fingerstick glucose measurements, showed no association between within-day glycemic variability and the occurrence of microvascular complications (64).
Conversely, several studies, mainly on adult populations, have suggested a role of glucose excursions as independent risk factors for complications. Nerve excitability scores were inversely correlated with CONGA levels in a cohort of individuals with T1D (65). In a study on 37 adults with T1D, an association between glycemic variability metrics, including low blood glucose index and CONGA, and early structural damage of neuroretina was identified (66). Shen et al. detected an increased risk of distal symmetrical polyneuropathy among subjects with higher CV and MAGE (67). Cardiovascular autonomic neuropathy, a common and often misdiagnosed phenotype of diabetic neuropathy, has been associated with higher CV, SD, and MAGE in a case-control study (68). A link between glycemic variability and macrovascular disease has been also proposed, since an association between glucose SD and coronary artery calcium, a sign of subclinical atherosclerosis, was observed in cohort of men with T1D (69).
Limited studies are available regarding the long-term outcomes of glycemic variability during pediatric age. However, it is feasible that early consequences of glucose variability start during the first years of disease, regardless of the appearance of clinical manifestations. This hypothesis is supported by a cross-sectional study on 25 children with T1D, which found a relationship between greater glycemic excursions and oxidative stress, as measured by urinary excretion of 8-iso-prostanglandin F2-alpha (70). Oxysterol species, other biomarkers of oxidative stress, have been also found to be strongly correlated with glucose SD and MAGE (71). Moreover, findings from an in vitro study suggest that fluctuations in glucose levels may exert a more relevant influence than hyperglycemia alone on the activation of several genes associated with the development of microvascular complications (72).
Regarding long-term glucose variability, a retrospective analysis of electronic records of 1,195 children with T1D revealed an association between higher SD of HbA1c and microalbuminuria (73). In a cohort of 267 youths, lipoprotein-associated phospholipase A2, a marker of early vascular damage, was significantly associated with CV calculated from CGM data of a 4-week period (74). Additionally, masked hypertension, a cardiovascular risk factor defined as hypertension detected by continuous blood pressure monitoring with normal office blood pressure, has been detected more frequently in children and adolescents with T1D showing higher glucose SD (75).
Furthermore, long-term outcomes of high glucose variability are not limited to micro- and macrovascular damage, but a potential involvement of other organs, such as thyroid and periodontal tissue, has been described (76,77).
Cognitive disfunctions are closely related to suboptimal glycemic control in people with diabetes. A magnetic resonance spectroscopy investigation on human and murine models showed that brain glucose levels are strongly influenced by glycemic excursions (78). A metanalysis analyzing data from nineteen pediatric studies found that extreme blood glucose values are associated with poorer cognition and memory performance (79). Sleep quality has been also found to be negatively influenced by high glycemic variability during adolescence (80).
Lastly, metabolic control is fundamental for appropriate anthropometric development in children with T1D. Glycemic variability is likely involved in the linear growth process, as shown by a longitudinal study revealing an inverse correlation between height SD and glucose CV in 144 prepubertal children (81).
Strengths and limitations
The primary strength of this narrative review lies in its extensive coverage of studies on CGM data in pediatric populations with T1D, including real-world data. However, a notable limitation of this review is the current scarcity of long-term studies available to assess the impact of CGM-derived glycemic control indicators on the risk of chronic diabetes complications. Moreover, data availability is further constrained in pediatric populations due to the lower incidence of complications in this age group.
Conclusions
The widespread use of CGM systems has facilitated the characterization and quantification of glycemic fluctuations, thereby enabling the incorporation of glycemic variability as a useful therapeutic target in the routine clinical management of youths with T1D. This population is characterized by greater glycemic excursions related to behavioral and hormonal factors. While several studies suggest a potential role of glycemic variability as an independent risk factor for diabetes-related complications, additional long-term data are awaited to corroborate these findings. In this context, the increasing use of automated insulin delivery systems for the management of pediatric T1D represents a valuable treatment strategy to minimize the risk of glucose excursion in this population.
Acknowledgments
Funding: None.
Footnote
Reporting Checklist: The authors have completed the Narrative Review reporting checklist. Available at https://tp.amegroups.com/article/view/10.21037/tp-24-114/rc
Peer Review File: Available at https://tp.amegroups.com/article/view/10.21037/tp-24-114/prf
Conflicts of Interest: All authors have completed the ICMJE uniform disclosure form (available at https://tp.amegroups.com/article/view/10.21037/tp-24-114/coif). The authors have no conflicts of interest to declare.
Ethical Statement: The authors are accountable for all aspects of the work in ensuring that questions related to the accuracy or integrity of any part of the work are appropriately investigated and resolved.
Open Access Statement: This is an Open Access article distributed in accordance with the Creative Commons Attribution-NonCommercial-NoDerivs 4.0 International License (CC BY-NC-ND 4.0), which permits the non-commercial replication and distribution of the article with the strict proviso that no changes or edits are made and the original work is properly cited (including links to both the formal publication through the relevant DOI and the license). See: https://creativecommons.org/licenses/by-nc-nd/4.0/.
References
- Atkinson MA, Eisenbarth GS, Michels AW. Type 1 diabetes. Lancet 2014;383:69-82. [Crossref] [PubMed]
- Maahs DM, West NA, Lawrence JM, et al. Epidemiology of type 1 diabetes. Endocrinol Metab Clin North Am 2010;39:481-97. [Crossref] [PubMed]
- Lind M, Svensson AM, Kosiborod M, et al. Glycemic control and excess mortality in type 1 diabetes. N Engl J Med 2014;371:1972-82. [Crossref] [PubMed]
- Nathan DM, Genuth S, et al. The effect of intensive treatment of diabetes on the development and progression of long-term complications in insulin-dependent diabetes mellitus. N Engl J Med 1993;329:977-86. [Crossref] [PubMed]
- de Bock M, Codner E, Craig ME, et al. ISPAD Clinical Practice Consensus Guidelines 2022: Glycemic targets and glucose monitoring for children, adolescents, and young people with diabetes. Pediatr Diabetes 2022;23:1270-6. [Crossref] [PubMed]
- Lombardo F, Passanisi S, Alibrandi A, et al. MiniMed 780G Six-Month Use in Children and Adolescents with Type 1 Diabetes: Clinical Targets and Predictors of Optimal Glucose Control. Diabetes Technol Ther 2023;25:404-13. [Crossref] [PubMed]
- Arrieta A, Battelino T, Scaramuzza AE, et al. Comparison of MiniMed 780G system performance in users aged younger and older than 15 years: Evidence from 12 870 real-world users. Diabetes Obes Metab 2022;24:1370-9. [Crossref] [PubMed]
- Piona C, Ventrici C, Marcovecchio L, et al. Long-term complications of type 1 diabetes: what do we know and what do we need to understand? Minerva Pediatr (Torino) 2021;73:504-22. [Crossref] [PubMed]
- Kovatchev B. Glycemic Variability: Risk Factors, Assessment, and Control. J Diabetes Sci Technol 2019;13:627-35. [Crossref] [PubMed]
- Neylon OM, Baghurst PA, Cameron FJ. The Minimum Duration of Sensor Data From Which Glycemic Variability Can Be Consistently Assessed. J Diabetes Sci Technol 2014;8:273-6. [Crossref] [PubMed]
- Piona C, Marigliano M, Mozzillo E, et al. Long-term glycemic control and glucose variability assessed with continuous glucose monitoring in a pediatric population with type 1 diabetes: Determination of optimal sampling duration. Pediatr Diabetes 2020;21:1485-92. [Crossref] [PubMed]
- Piona C, Marigliano M, Mozzillo E, et al. Relationships between HbA1c and continuous glucose monitoring metrics of glycaemic control and glucose variability in a large cohort of children and adolescents with type 1 diabetes. Diabetes Res Clin Pract 2021;177:108933. [Crossref] [PubMed]
- Mo Y, Lu J, Zhou J. Glycemic variability: Measurement, target, impact on complications of diabetes and does it really matter? J Diabetes Investig 2024;15:5-14. [Crossref] [PubMed]
- Battelino T, Danne T, Bergenstal RM, et al. Clinical Targets for Continuous Glucose Monitoring Data Interpretation: Recommendations From the International Consensus on Time in Range. Diabetes Care 2019;42:1593-603. [Crossref] [PubMed]
- Gómez AM, Henao DC, Imitola Madero A, et al. Defining High Glycemic Variability in Type 1 Diabetes: Comparison of Multiple Indexes to Identify Patients at Risk of Hypoglycemia. Diabetes Technol Ther 2019;21:430-9. [Crossref] [PubMed]
- El-Laboudi AH, Godsland IF, Johnston DG, et al. Measures of Glycemic Variability in Type 1 Diabetes and the Effect of Real-Time Continuous Glucose Monitoring. Diabetes Technol Ther 2016;18:806-12. [Crossref] [PubMed]
- Piona C, Marigliano M, Mozzillo E, et al. High Glycemic Variability Is Associated with Worse Continuous Glucose Monitoring Metrics in Children and Adolescents with Type 1 Diabetes. Horm Res Paediatr 2021;94:369-73. [Crossref] [PubMed]
- Lazar S, Ionita I, Reurean-Pintilei D, et al. To What Extent Is Hb(A1c) Associated with Glycemic Variability in Patients with Type 1 Diabetes? A Retrospective, Noninterventional Study. J Clin Med 2024;13:450. [Crossref] [PubMed]
- Díaz-Soto G, Bahíllo-Curieses MP, Jimenez R, et al. The relationship between glycosylated hemoglobin, time-in-range and glycemic variability in type 1 diabetes patients under flash glucose monitoring. Endocrinol Diabetes Nutr (Engl Ed) 2021;68:465-71. [Crossref] [PubMed]
- Passanisi S, Piona C, Salzano G, et al. Aiming for the Best Glycemic Control Beyond Time in Range: Time in Tight Range as a New Continuous Glucose Monitoring Metric in Children and Adolescents with Type 1 Diabetes Using Different Treatment Modalities. Diabetes Technol Ther 2024;26:161-6. [Crossref] [PubMed]
- Luo J, Qu Y, Zhang Q, et al. Relationship of Glucose Variability With Glycated Hemoglobin and Daily Mean Glucose: A Post Hoc Analysis of Data From 5 Phase 3 Studies. J Diabetes Sci Technol 2018;12:325-32. [Crossref] [PubMed]
- Castañeda J, Arrieta A, van den Heuvel T, et al. The significance of coefficient of variation as a measure of hypoglycaemia risk and glycaemic control in real world users of the automated insulin delivery MiniMed 780G system. Diabetes Obes Metab 2023;25:2545-52. [Crossref] [PubMed]
- Julla JB, Jacquemier P, Fagherazzi G, et al. Is the Consensual Threshold for Defining High Glucose Variability Implementable in Clinical Practice? Diabetes Care 2021;44:1722-5. [Crossref] [PubMed]
- Riveline JP, Wojtusciszyn A, Guerci B, et al. Real world hypoglycaemia related to glucose variability and Flash glucose scan frequency assessed from global FreeStyle Libre data. Diabetes Obes Metab 2022;24:2102-7. [Crossref] [PubMed]
- Moscardó V, Herrero P, Reddy M, et al. Assessment of Glucose Control Metrics by Discriminant Ratio. Diabetes Technol Ther 2020;22:719-26. [Crossref] [PubMed]
- Vergès B, Pignol E, Rouland A, et al. Glycemic Variability Assessment with a 14-Day Continuous Glucose Monitoring System: When and How Long to Measure MAGE (Mean Amplitude of Glucose Excursion) for Optimal Reliability? J Diabetes Sci Technol 2022;16:982-7. [Crossref] [PubMed]
- Kovatchev BP. Metrics for glycaemic control - from HbA(1c) to continuous glucose monitoring. Nat Rev Endocrinol 2017;13:425-36. [Crossref] [PubMed]
- Klonoff DC, Wang J, Rodbard D, et al. A Glycemia Risk Index (GRI) of Hypoglycemia and Hyperglycemia for Continuous Glucose Monitoring Validated by Clinician Ratings. J Diabetes Sci Technol 2023;17:1226-42. [Crossref] [PubMed]
- Piona C, Marigliano M, Roncarà C, et al. Glycemia Risk Index as a Novel Metric to Evaluate the Safety of Glycemic Control in Children and Adolescents with Type 1 Diabetes: An Observational, Multicenter, Real-Life Cohort Study. Diabetes Technol Ther 2023;25:507-12. [Crossref] [PubMed]
- Joshi A, Mitra A, Anjum N, et al. Patterns of Glycemic Variability During a Diabetes Self-Management Educational Program. Med Sci (Basel) 2019;7:52. [Crossref] [PubMed]
- Kovatchev BP, Otto E, Cox D, et al. Evaluation of a new measure of blood glucose variability in diabetes. Diabetes Care 2006;29:2433-8. [Crossref] [PubMed]
- Zmyslowska A, Fendler W, Szadkowska A, et al. Glycemic variability in patients with Wolfram syndrome is lower than in type 1 diabetes. Acta Diabetol 2015;52:1057-62. [Crossref] [PubMed]
- Pollé OG, Delfosse A, Martin M, et al. Glycemic Variability Patterns Strongly Correlate With Partial Remission Status in Children With Newly Diagnosed Type 1 Diabetes. Diabetes Care 2022;45:2360-8. [Crossref] [PubMed]
- Harvengt AA, Polle OG, Martin M, et al. Post-Hypoglycemic hyperglycemia are highly relevant markers for stratification of glycemic variability and partial remission status of pediatric patients with new-onset type 1 diabetes. PLoS One 2023;18:e0294982. [Crossref] [PubMed]
- Alghothani N, Dungan KM. The effect of glycemic variability on counterregulatory hormone responses to hypoglycemia in young children and adolescents with type 1 diabetes. Diabetes Technol Ther 2011;13:1085-9. [Crossref] [PubMed]
- Guo K, Zhang L, Ye J, et al. Metabolic syndrome associated with higher glycemic variability in type 1 diabetes: A multicenter cross-sectional study in china. Front Endocrinol (Lausanne) 2022;13:972785. [Crossref] [PubMed]
- Lipsky LM, Gee B, Liu A, et al. Glycemic control and variability in association with body mass index and body composition over 18months in youth with type 1 diabetes. Diabetes Res Clin Pract 2016;120:97-103. [Crossref] [PubMed]
- Passanisi S, Salzano G, Lombardo F. Skin Involvement in Paediatric Patients with Type 1 Diabetes. Curr Diabetes Rev 2022;18:e030921196145. [Crossref] [PubMed]
- Famulla S, Hövelmann U, Fischer A, et al. Insulin Injection Into Lipohypertrophic Tissue: Blunted and More Variable Insulin Absorption and Action and Impaired Postprandial Glucose Control. Diabetes Care 2016;39:1486-92. [Crossref] [PubMed]
- Lombardo F, Bombaci B, Alibrandi A, et al. The Impact of Insulin-Induced Lipodystrophy on Glycemic Variability in Pediatric Patients with Type 1 Diabetes. Children (Basel) 2022;9:1087. [Crossref] [PubMed]
- Gupta SS, Gupta KS, Gathe SS, et al. Clinical Implications of Lipohypertrophy Among People with Type 1 Diabetes in India. Diabetes Technol Ther 2018;20:483-91. [Crossref] [PubMed]
- Zhu J, Volkening LK, Laffel LM. Distinct Patterns of Daily Glucose Variability by Pubertal Status in Youth With Type 1 Diabetes. Diabetes Care 2020;43:22-8. [Crossref] [PubMed]
- Shetty VB, Fournier PA, Davey RJ, et al. Effect of Exercise Intensity on Glucose Requirements to Maintain Euglycemia During Exercise in Type 1 Diabetes. J Clin Endocrinol Metab 2016;101:972-80. [Crossref] [PubMed]
- Adolfsson P, Taplin CE, Zaharieva DP, et al. ISPAD Clinical Practice Consensus Guidelines 2022: Exercise in children and adolescents with diabetes. Pediatr Diabetes 2022;23:1341-72. [Crossref] [PubMed]
- Bachmann S, Hess M, Martin-Diener E, et al. Nocturnal Hypoglycemia and Physical Activity in Children With Diabetes: New Insights by Continuous Glucose Monitoring and Accelerometry. Diabetes Care 2016;39:e95-6. [Crossref] [PubMed]
- Metcalf KM, Singhvi A, Tsalikian E, et al. Effects of moderate-to-vigorous intensity physical activity on overnight and next-day hypoglycemia in active adolescents with type 1 diabetes. Diabetes Care 2014;37:1272-8. [Crossref] [PubMed]
- Jaggers JR, King KM, McKay T, et al. Association between Intensity Levels of Physical Activity and Glucose Variability among Children and Adolescents with Type 1 Diabetes. Int J Environ Res Public Health 2023;20:1623. [Crossref] [PubMed]
- Rebesco DB, França SN, Lima VA, et al. Different amounts of moderate to vigorous physical activity and change in glycemic variability in adolescents with type 1 diabetes: is there dose-response relationship? Arch Endocrinol Metab 2020;64:312-8. [Crossref] [PubMed]
- Patton SR, Monzon AD, Noser AE, et al. Physical Activity, Glycemic Variability, and Parental Hypoglycemia Fear in Preschoolers With Type 1 Diabetes. Pediatr Exerc Sci 2022;34:135-40. [Crossref] [PubMed]
- Singhvi A, Tansey MJ, Janz K, et al. Aerobic fitness and glycemic variability in adolescents with type 1 diabetes. Endocr Pract 2014;20:566-70. [Crossref] [PubMed]
- İpar N, Boran P, Barış HE, et al. Associations between sleep characteristics and glycemic variability in youth with type 1 diabetes. Sleep Med 2023;109:132-42. [Crossref] [PubMed]
- Patton SR, Bergford S, Sherr JL, et al. Postprandial Glucose Variability Following Typical Meals in Youth Living with Type 1 Diabetes. Nutrients 2024;16:162. [Crossref] [PubMed]
- Monzon AD, Smith LB, Powers SW, et al. The Association Between Glycemic Variability and Macronutrients in Young Children with T1D. J Pediatr Psychol 2020;45:749-58. [Crossref] [PubMed]
- Paterson MA, Smart CEM, Howley P, et al. High-protein meals require 30% additional insulin to prevent delayed postprandial hyperglycaemia. Diabet Med 2020;37:1185-91. [Crossref] [PubMed]
- Smith TA, Blowes AA, King BR, et al. Families' reports of problematic foods, management strategies and continuous glucose monitoring in type 1 diabetes: A cross-sectional study. Nutr Diet 2021;78:449-57. [Crossref] [PubMed]
- Brazeau AS, Mircescu H, Desjardins K, et al. Carbohydrate counting accuracy and blood glucose variability in adults with type 1 diabetes. Diabetes Res Clin Pract 2013;99:19-23. [Crossref] [PubMed]
- Pham-Short A, Donaghue KC, Ambler G, et al. Greater postprandial glucose excursions and inadequate nutrient intake in youth with type 1 diabetes and celiac disease. Sci Rep 2017;7:45286. [Crossref] [PubMed]
- Mozzillo E, Marigliano M, Cuccurullo I, et al. Maintaining the gluten-free diet: The key to improve glycemic metrics in youths with type 1 diabetes and celiac disease. Diabetes Res Clin Pract 2024;207:111074. [Crossref] [PubMed]
- Cox DJ, Kovatchev BP, Julian DM, et al. Frequency of severe hypoglycemia in insulin-dependent diabetes mellitus can be predicted from self-monitoring blood glucose data. J Clin Endocrinol Metab 1994;79:1659-62. [Crossref] [PubMed]
- Bjornstad P, Dart A, Donaghue KC, et al. ISPAD Clinical Practice Consensus Guidelines 2022: Microvascular and macrovascular complications in children and adolescents with diabetes. Pediatr Diabetes 2022;23:1432-50. [Crossref] [PubMed]
- Sustained effect of intensive treatment of type 1 diabetes mellitus on development and progression of diabetic nephropathy: the Epidemiology of Diabetes Interventions and Complications (EDIC) study. JAMA 2003;290:2159-67. [Crossref] [PubMed]
- Šoupal J, Škrha J Jr, Fajmon M, et al. Glycemic variability is higher in type 1 diabetes patients with microvascular complications irrespective of glycemic control. Diabetes Technol Ther 2014;16:198-203. [Crossref] [PubMed]
- Smith-Palmer J, Brändle M, Trevisan R, et al. Assessment of the association between glycemic variability and diabetes-related complications in type 1 and type 2 diabetes. Diabetes Res Clin Pract 2014;105:273-84. [Crossref] [PubMed]
- Lachin JM, Bebu I, Bergenstal RM, et al. Association of Glycemic Variability in Type 1 Diabetes With Progression of Microvascular Outcomes in the Diabetes Control and Complications Trial. Diabetes Care 2017;40:777-83. [Crossref] [PubMed]
- Issar T, Tummanapalli SS, Kwai NCG, et al. Associations between acute glucose control and peripheral nerve structure and function in type 1 diabetes. Diabet Med 2020;37:1553-60. [Crossref] [PubMed]
- Picconi F, Parravano M, Ylli D, et al. Retinal neurodegeneration in patients with type 1 diabetes mellitus: the role of glycemic variability. Acta Diabetol 2017;54:489-97. [Crossref] [PubMed]
- Shen Z, Jiang H, Huang R, et al. Association of glycemic variability and hypoglycemia with distal symmetrical polyneuropathy in adults with type 1 diabetes. Sci Rep 2021;11:22875. [Crossref] [PubMed]
- Naaman SH, Mizrak HI, Lind N, et al. Cardiovascular Autonomic Neuropathy Is Associated With Increased Glucose Variability in People With Type 1 Diabetes. Diabetes Care 2022;45:2461-5. [Crossref] [PubMed]
- Snell-Bergeon JK, Roman R, Rodbard D, et al. Glycaemic variability is associated with coronary artery calcium in men with Type 1 diabetes: the Coronary Artery Calcification in Type 1 Diabetes study. Diabet Med 2010;27:1436-42. [Crossref] [PubMed]
- Colomo N, López-Siguero JP, Leiva I, et al. Relationship between glucose control, glycemic variability, and oxidative stress in children with type 1 diabetes. Endocrinol Diabetes Nutr (Engl Ed) 2019;66:540-9. [Crossref] [PubMed]
- Ünlütürk U, Bahçecioğlu AB, Samadi A, et al. Glycemic variability leads to higher levels of auto-oxidized oxysterol species in patients with type 1 diabetes mellitus. J Endocrinol Invest 2023;46:2547-54. [Crossref] [PubMed]
- Kuricová K, Pácal L, Šoupal J, et al. Effect of glucose variability on pathways associated with glucotoxicity in diabetes: Evaluation of a novel in vitro experimental approach. Diabetes Res Clin Pract 2016;114:1-8. [Crossref] [PubMed]
- Raman S, Dai H, DeLurgio SA, et al. High hemoglobin A1c variability is associated with early risk of microalbuminuria in children with T1D. Pediatr Diabetes 2016;17:398-406. [Crossref] [PubMed]
- Morandi A, Piona C, Corradi M, et al. Risk factors for pre-clinical atherosclerosis in adolescents with type 1 diabetes. Diabetes Res Clin Pract 2023;198:110618. [Crossref] [PubMed]
- Homhuan W, Poomthavorn P, Paksi W, et al. Masked hypertension and its associations with glycemic variability metrics in children and adolescents with type 1 diabetes. Pediatr Nephrol 2021;36:379-86. [Crossref] [PubMed]
- Dicembrini I, Barbato L, Serni L, et al. Glucose variability and periodontal disease in type 1 diabetes: a cross-sectional study-The "PAROdontopatia e DIAbete" (PARODIA) project. Acta Diabetol 2021;58:1367-71. [Crossref] [PubMed]
- Bellastella G, Maiorino MI, Scappaticcio L, et al. TSH oscillations in young patients with type 1 diabetes may be due to glycemic variability. J Endocrinol Invest 2018;41:389-93. [Crossref] [PubMed]
- Hwang JJ, Jiang L, Sanchez Rangel E, et al. Glycemic Variability and Brain Glucose Levels in Type 1 Diabetes. Diabetes 2019;68:163-71. [Crossref] [PubMed]
- He J, Ryder AG, Li S, et al. Glycemic extremes are related to cognitive dysfunction in children with type 1 diabetes: A meta-analysis. J Diabetes Investig 2018;9:1342-53. [Crossref] [PubMed]
- Griggs S, Redeker NS, Jeon S, et al. Daily variations in sleep and glucose in adolescents with type 1 diabetes. Pediatr Diabetes 2020;21:1493-501. [Crossref] [PubMed]
- Blasetti A, Castorani V, Polidori N, et al. Role of glucose variability on linear growth in children with type 1 diabetes. Endocr Connect 2023;12:e220370. [Crossref] [PubMed]