Construction of a nomogram for early diagnosis of refractory Mycoplasma pneumoniae pneumonia in children
Highlight box
Key findings
• Fever duration of more than 10.5 days, pleural effusion, white blood cells >10.13×109/L, neutrophil count >6.43×109/L, C-reactive protein >29.45 mg/L, lactate dehydrogenase >370.50 U/L, neutrophil-to-lymphocyte ratio (NLR) >3.47, and serum uric acid (SUA) <170.5 µmol/mL was an early predictive model of refractory Mycoplasma pneumoniae pneumonia (RMPP).
What is known and what is new?
• Although nomograms for RMPP had been established in previous studies, the clinical specificity of them is not very high or they do not fully apply to primary doctors.
• We found that the SUA and NLR are a widely available and low-cost predictive index with good clinical value. SUA and NLR indicators were added to the nomogram, which could more comprehensively help clinicians to predict RMPP, especially in primary care hospitals.
What is the implication, and what should change now?
• The simple and easy-to-use nomogram can help clinicians, especially primary doctors, to make early diagnoses of RMPP.
IntroductionOther Section
Mycoplasma pneumoniae (MP) is an important pathogenic bacterium which can cause community-acquired pneumonia in children. MP pneumonia (MPP) is responsible for 10–40% of hospitalizations among children with community-acquired pneumonia (1). Most children with MPP have a mild condition and a self-limiting process. Refractory Mycoplasma pneumoniae pneumonia (RMPP) has a serious, rapid progression that can easily cause a variety of extra-pulmonary complications, leading to poor efficacy of conventional drugs; the condition is susceptible to delayed treatment, seriously threatening children’s physical and mental health and safety (2). Therefore, searching for early warning indicators of RMPP has important clinical implications. At present, there are many clinical studies on RMPP and the more recommended indicators are lactate dehydrogenase (LDH), C-reactive protein (CRP), ferritin (FER), and D-dimer (3-5). Shen et al. (3) reported that CRP, LDH, and D-dimer were predictive factors for RMPP, with an area under the receiver operating characteristic (ROC) curve (AUC) of the nomogram of 0.881. However, the clinical specificity of the above indicators is not high. In recent years, studies have also confirmed that cytokine imbalance can serve as a key factor in predicting RMPP. Studies have shown that interleukin (IL)-6, IL-10, IL-17, IL-8, interferon (IFN)-γ, tumor necrosis factor (TNF)-α, and CXCL10/IP-10 may be potential biomarkers of RMPP (6,7). The study found that the soluble B7-DC (sB7-DC) and IL-17 levels of children with RMPP were significantly increased. The sensitivity of IL-17 to RMPP prediction was 83.3% and the specificity was 62.9%. The sensitivity and specificity of sB7-DC to RMPP were 86.7% and 62.9%, respectively, and sB7-DC had a higher predictive value than IL-17 for RMPP (8). Zhu et al. (9) reported that the AUC of CCL2 in bronchoalveolar lavage fluid (BALF) predicting RMPP was 0.94, the cut-off value was 0.645 ng/mL, the sensitivity was 85%, and the specificity was 94%. However, the prediction role of these cytokines has not achieved a wide consensus, and these indicators are difficult to obtain clinically, especially in primary hospitals. Therefore, the discovery of a safe, accurate, efficient, easy to obtain, and quantifiable diagnostic tool to predict RMPP is an urgent need.
Serum uric acid (SUA) is an end product of purine metabolism in the body (10,11). Studies have shown that abnormal uric acid levels are a risk factor for cardiovascular, neurological, and metabolic diseases. However, studies of uric acid levels in respiratory tract infections are rare; there are few studies on the prediction of SUA levels in RMPP. Neutrophil-to-lymphocyte ratio (NLR) represents an essential cellular component of human host defense. Neutrophils (NEP) are the first cells in the line of defense against infection in the non-specific immune system. They induce and activate an inflammatory response, whereas lymphocytes play a role in adaptive immunity. It has been reported that the NLR could better predict the clinical outcomes of patients with systemic inflammation (12). The clinical significance of the NLR for the early diagnosis of RMPP is currently unclear. This study aimed to establish and validate a risk prediction model based on clinical manifestations, laboratory blood indicators, and radiological findings to help clinicians identify patients who are at high risk of RMPP. We present this article in accordance with the TRIPOD reporting checklist (available at https://tp.amegroups.com/article/view/10.21037/tp-24-16/rc).
MethodsOther Section
Participants and inclusion and exclusion criteria
This retrospective study was a single-center clinical data analysis. We retrospectively analyzed the medical records of 369 children with MPP admitted to Xi’an Children’s Hospital between January 2022 and January 2023. Patient-related information, including demographics, clinical and laboratory findings, and imaging data were extracted from the medical records. The inclusion criteria were as follows: (I) MPP: consistent with the clinical presentation and imaging changes of pneumonia; (II) cephalexin and penicillin antibiotics were not effective; (III) the diagnosis of MP infection was based on the positive serologic test results (MP IgM positive and antibody titer 1:160) combined with positive results for MP polymerase chain reaction (PCR) tests of nasopharyngeal secretions. General Mycoplasma pneumoniae pneumonia (GMPP): MPP was treated with macrolide antibiotics for 5–7 days and improved significantly. RMPP: MPP has been regularly treated with macrolide antibiotics for 7 days, with aggravated clinical signs, persistent fever, and worse pulmonary imaging symptoms. The exclusion criteria were as follows: congenital heart disease, genetic and metabolic diseases, neurological diseases, congenital bronchopulmonary dysplasia, immune deficiency, and long-term use of immunosuppressants, tuberculosis, asthma, and bronchial foreign bodies was excluded. All cases of MPP were reviewed by a final diagnosis committee composed of two specialist pediatricians (with experience in pediatric respiratory medicine) and a radiologist in cases of pneumonia. The cases were divided into GMPP and RMPP groups according to the diagnostic criteria. The study was conducted in accordance with the Declaration of Helsinki (as revised in 2013). This study was approved by the Medical Ethics Committee of Xi’an Children’s Hospital (reference number: 20230004) and informed consent was provided by the parents or legal guardians of the children.
Data collection
The study collected the case data of children, including general details (age, sex), clinical data (fever duration, fever peak, wheezing), laboratory examination results [white blood cells (WBCs), NEP, lymphocytes (LYM), platelets (PLT), erythrocyte sedimentation rate (ESR), procalcitonin (PCT), CRP, LDH, lymphocyte/leukocyte ratio, NLR, platelet/lymphocyte ratio], and imaging data (consolidation, lung atelectasis, pleural effusion). Values for the abovementioned laboratory indictors were determined using an automatic blood analyzer and automatic biochemical analyzer at Xi’an Children’s Hospital. The radiological reports were performed by a radiologist. All laboratory examinations were completed within 24 hours of hospital admission. Patients with incomplete data and information were excluded.
Statistical analysis
Variable selection and model construction
Statistical analysis was performed using the software SPSS 25.0 (IBM Corp., Armonk, NY, USA). Measurement data were non-normally distributed. Non-normally distributed continuous variables were summarized as medians and interquartile ranges (IQRs), and the rank-sum test was used to assess the significance of differences between groups. Categorical data were expressed as n (%), and a chi-square test was used for the comparison between groups. A single factor analysis was used to screen the indicators with significant differences. Those variables with P value of 0.2 and below in univariable analysis were entered into a binary logistic regression. The independent risk factors for RMPP were analyzed by binary logistic regression analysis and were considered statistically significant with P<0.05. ROC analysis was used to determine the cut-off value for each index and to evaluate the predictive value of the relevant indicators in the RMPP. Multiple linear regression analysis was used to find the correlation between the independent risk factors.
Model performance evaluation
Statistical analysis was carried out using R software (version 4.0.5; The R Foundation for Statistical Computing, Vienna, Austria) to create all of the graphics. A 2-sided α less than 0.05 was considered statistically significant. The performance of the prediction model was evaluated from discrimination ability, calibration ability, and clinical value. The discrimination ability was evaluated through ROC analysis with AUC, and the calibration plot accompanied with the Hosmer-Lemeshow test was applied to assess the calibration ability. A P value >0.05 in the model calibration test (Hosmer-Lemeshow test) indicated acceptable model calibration. The closer the AUCs were to 1.0, the higher the authenticity of the detection method. The higher the sensitivity, the higher the diagnostic accuracy, and the higher the specificity, the lower the misdiagnosis rate. The usual performance measurements for risk prediction models (discrimination and calibration) were unable to address the question of how beneficial the produced nomogram would be in clinical practice. As a result, decision curve analysis (DCA) was performed with the intention of developing a more clinically interpretable risk prediction model. The model was validated using a bootstrap method with 1,000 resamples to quantify any overfitting.
Statistical analyses were performed separately by two researchers to ensure the reliability of the findings.
ResultsOther Section
A total of 369 children with MPP were included after excluding 8 cases with incomplete data, including 86 cases of RMPP and 283 cases of GMPP 283.
Clinical characteristics of children with RMPP and GMPP
There were no statistically significant differences in sex and age between the children in the RMPP group and those in the GMPP group. Baseline data were comparable between both groups. The results showed that the fever peak and duration of the children in the RMPP group were higher and longer compared with the GMPP group. The wheezing symptom was not significantly different between the RMPP and GMPP groups, as shown in Table 1. There was a significant difference in the incidence of lobar pneumonia and pleural effusion in pulmonary imaging between the two groups, but there were no statistically significant differences in the incidence of atelectasis (Table 1). Laboratory tests showed that WBC, NEP, CRP, ESR, PCT, SUA, albumin (ALB), LDH, lymphocyte-to-white blood cell ratio (LWR) and NLR were statistically different between the two groups.
Table 1
Variables | GMPP (n=283) | RMPP (n=86) | Z/χ2 | P value |
---|---|---|---|---|
Baseline characteristics | ||||
Age (years) | 6.75 (5.160–8.16) | 6.91 (5.500–8.43) | −1.383 | 0.167 |
Sex, male | 157 (55.5) | 45 (52.3) | 0.264 | 0.607 |
Clinical characteristics | ||||
Fever peak (℃) | 39.6 (39.2–40) | 40.0 (40–40.525) | −7.339 | <0.001* |
Fever duration (days) | 9 (7–11) | 13.5 (12–17) | −10.168 | <0.001* |
Wheezing | 18 (6.4) | 7 (8.1) | 0.331 | 0.565 |
Pulmonary imaging characteristics | ||||
Lobar pneumonia | 247 (87.3) | 85 (98.8) | 9.767 | 0.002* |
Atelectasis | 8 (2.8) | 3 (3.5) | 0.100 | 0.752 |
Pleural effusion | 72 (25.4) | 67 (77.9) | 77.324 | <0.001* |
Laboratory characteristic | ||||
WBC (×109/L) | 7.88 (5.94–9.61) | 11.07 (7.92–13.35) | −6.473 | <0.001* |
NEP (×109/L) | 4.98 (3.56–6.48) | 7.55 (5.47–10.26) | −7.260 | <0.001* |
LYM (×109/L) | 1.90 (1.48–2.38) | 2.00 (1.50–2.62) | −1.004 | 0.315 |
PLT (×109/L) | 294.00 (229.00–351.00) | 265.50 (206.75–393) | −0.761 | 0.446 |
CRP (mg/L) | 11.25 (0.00–25.95) | 59.27 (24.71–118) | −9.515 | <0.001* |
ESR (mm/h) | 38.00 (30.00–60.00) | 50.50 (32.75–80.00) | −3.249 | 0.001* |
PCT (ng/mL) | 0.07 (0.00–1.40) | 0.22 (0.10–0.745) | −7.822 | <0.001* |
SUA (μmol/mL) | 203.00 (168.00–250.00) | 150.00 (124.50–184.50) | −6.854 | <0.001* |
ALB (g/L) | 37.90 (34.90–39.90) | 31.95 (28.55–35.23) | −9.304 | <0.001* |
LDH (U/L) | 268.00 (224.00–339.00) | 473.00 (389.75–622.00) | −10.340 | <0.001* |
LWR | 0.25 (0.19–0.31) | 0.19 (0.15–0.26) | −5.682 | <0.001* |
NLR | 2.56 (1.78–3.63) | 3.77 (2.67–5.26) | −5.987 | <0.001* |
PLR | 150.00 (118.50–195.10) | 140.60 (104.60–196.20) | −1.186 | 0.236 |
All continuous variables are non-normally distributed and presented as median (interquartile range). Categorical variables are presented as n (%). *, P<0.05, compared with children with GMPP and RMPP. GMPP, general Mycoplasma pneumoniae pneumonia; RMPP, refractory Mycoplasma pneumoniae pneumonia; WBC, white blood cell; NEP, neutrophil count; LYM, lymphocyte count; PLT, platelet count; CRP, C-reactive protein; ESR, erythrocyte sedimentation rate; PCT, procalcitonin; SUA, serum uric acid; ALB, albumin; LDH, lactate dehydrogenase; LWR, lymphocyte-to-white blood cell ratio; NLR, neutrophil-to-lymphocyte ratio; PLR, platelet-to-lymphocyte ratio.
Results of the RMPP binary logistic regression analysis
The above meaningful indicators were subjected to binary logistic regression analysis. Fever duration, pleural effusion, WBC, NEP, LDH, CRP, NLR, and SUA levels were identified as independent predictors of RMPP, as shown in Table 2.
Table 2
Factors | B | S.E. | Wald | P value | OR | 95% CI |
---|---|---|---|---|---|---|
Fever duration | 0.287 | 0.067 | 18.299 | <0.001 | 1.332 | 1.168–1.520 |
Fever peak | 0.811 | 0.433 | 3.498 | 0.061 | 2.249 | 0.962–5.260 |
Lobar pneumonia | 0.549 | 1.350 | 0.166 | 0.684 | 1.732 | 0.123–24.430 |
Pleural effusion | 1.060 | 0.435 | 5.944 | 0.015 | 2.885 | 1.231–6.764 |
WBC | −1.149 | 0.416 | 7.615 | 0.006 | 0.317 | 0.140–0.717 |
NEP | 1.695 | 0.567 | 8.927 | 0.003 | 5.447 | 1.792–16.562 |
ESR | −0.014 | 0.009 | 2.615 | 0.106 | 0.986 | 0.969–1.003 |
PCT | 0.202 | 0.196 | 1.060 | 0.303 | 1.224 | 0.833–1.797 |
CRP | 0.021 | 0.006 | 12.575 | <0.001 | 1.021 | 1.009–1.033 |
SUA | −0.012 | 0.005 | 5.417 | 0.020 | 0.989 | 0.979–0.998 |
ALB | 0.079 | 0.070 | 1.266 | 0.261 | 1.082 | 0.943–1.240 |
LDH | 0.007 | 0.002 | 17.099 | <0.001 | 1.007 | 1.004–1.011 |
LWR | −7.912 | 4.454 | 3.155 | 0.076 | 0.000 | 0.000–2.266 |
NLR | −0.910 | 0.328 | 7.700 | 0.006 | 0.403 | 0.212–0.765 |
Constant | −36.887 | 17.892 | 4.251 | 0.039 | 0.000 | – |
WBC, white blood cell; NEP, neutrophil count; ESR, erythrocyte sedimentation rate; PCT, procalcitonin; CRP, C-reactive protein; SUA, serum uric acid; ALB, albumin; LDH, lactate dehydrogenase; LWR, lymphocyte-to-white blood cell ratio; NLR, neutrophil-to-lymphocyte ratio; B, β value; S.E., standard error; OR, odds ratio; CI, confidence interval.
ROC curve analysis
The ROC curve results showed that fever duration, WBC, NEP, CRP, LDH, NLR, and SUA had good predictive value. The AUC values were 0.861, 0.730, 0.758, 0.837, 0.868, 0.713, and 0.744 and the best cutoff values were 10.50, 10.13, 6.43, 29.45, 370.50, 3.47, and 170.50, respectively. The best predictor of the above laboratory indicators was the LDH, with a sensitivity and specificity of 0.837 and 0.837, respectively. The newly discovered indicator was the SUA levels. When the cutoff values of SUA were 170.50, the sensitivity and specificity were 0.651 and 0.742, respectively (Table 3).
Table 3
Factors | AUC | P value | Cut off | Sensitivity | Specificity |
---|---|---|---|---|---|
Fever duration | 0.861 | <0.001 | 10.50 | 0.895 | 0.728 |
WBC | 0.730 | <0.001 | 10.13 | 0.616 | 0.809 |
NEP | 0.758 | <0.001 | 6.43 | 0.674 | 0.742 |
CRP | 0.837 | <0.001 | 29.45 | 0.733 | 0.774 |
LDH | 0.868 | <0.001 | 370.50 | 0.837 | 0.837 |
NLR | 0.713 | <0.001 | 3.47 | 0.605 | 0.731 |
SUA | 0.744 | <0.001 | 170.50 | 0.651 | 0.742 |
RMPP, refractory Mycoplasma pneumoniae pneumonia; WBC, white blood cell; NEP, neutrophil count; CRP, C-reactive protein; LDH, lactate dehydrogenase; NLR, neutrophil-to-lymphocyte ratio; SUA, serum uric acid.
Multiple linear regression analysis
A multivariate linear regression analysis was carried out for the independent risk factors obtained by logistic regression analysis. The fever duration was selected as the dependent variable, SUA, CRP, LDH, and NLR as the independent variables. Multivariate linear regression model analysis showed the R2 was 0.328, indicating that the four indicators of SUA, CRP, LDH, and NLR could explain 32.8% of the fever duration variability. The Durbin-Watson index of 1.77, which is used to detect the presence of autocorrelation in the residuals of a regression, indicated a slight non-independence (Table 4). However, there was little effect on the accuracy of the regression results. The results of multiple linear regression analysis showed a positive linear relationship between CRP, LDH, NLR, and fever duration, and a negative linear relationship between SUA and fever duration, as shown in Table 5.
Table 4
Model | Values |
---|---|
R | 0.572a |
R2 | 0.328 |
Adjusted R2 | 0.32 |
S.E. of the estimate | 3.835 |
Durbin-Watson | 1.77 |
a, predictors: (Constant), SUA, CRP, LDH, NLR. S.E., standard error. ; SUA, serum uric acid; CRP, C-reactive protein; LDH, lactate dehydrogenase; NLR, neutrophil-to-lymphocyte ratio
Table 5
Model | Coefficientsa | t | P value | Capacity | VIF | |
---|---|---|---|---|---|---|
Unstandardized coefficients (β) | Standardized coefficients (β) | |||||
SUA | −0.010 | −0.130 | −2.851 | 0.005 | 0.889 | 1.125 |
CRP | 0.022 | 0.203 | 4.047 | <0.001 | 0.731 | 1.369 |
LDH | 0.006 | 0.256 | 5.349 | <0.001 | 0.808 | 1.237 |
NLR | 0.587 | 0.241 | 5.098 | <0.001 | 0.830 | 1.205 |
a, dependent variable: fever duration. SUA, serum uric acid; CRP, C-reactive protein; LDH, lactate dehydrogenase; NLR, neutrophil-to-lymphocyte ratio; VIF, variance inflation factor.
Nomogram of the final model
The eight selected variables were applied to establish a logistic regression model and presented with a nomogram. These eight variables included fever duration, pleural effusion, WBC, NEP, CRP, LDH, NLR, and SUA. The final predictive model incorporating the eight factors is shown as a nomogram in Figure 1A. Total points based on the sum of the points for each predictor in this nomogram were associated with the risk of RMPP.
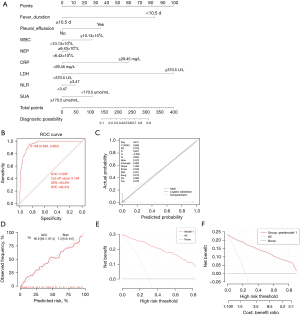
Performance of the nomogram
By internal bootstrap validation with 1,000 resamples, the mean AUC of the nomogram based on the development dataset was 0.956 [95% confidence interval (CI): 0.937–0.974], the sensitivity, and specificity of the nomogram model were 0.848, and 0.953, respectively (see Figure 1B), with good discrimination ability for predicting RMPP patients. Furthermore, the calibration plot and Hosmer-Lemeshow test (P=0.70) of the prediction model showed good consistency between the predicted probability and actual probability, as shown in Figure 1C,1D. The DCA showed that the nomogram model can bring clinical benefit to patients within a large threshold range (see Figure 1E,1F).
DiscussionOther Section
RMPP disease progresses rapidly, the symptoms are relatively intense, and the conventional treatment effect is poor. RMPP often causes various intrapulmonary complications, such as bronchial mucus plug formation, necrotizing pneumonia, bronchiolitis obliterans, and bronchiectasis. It can also cause extrapulmonary complications, involving multiple systems, such as myocarditis, nephritis, encephalitis, hepatitis, arthritis, Steven-Johnson syndrome, and others, and even lead to multi-system, multi-organ involvement and death (13,14). Therefore, actively exploring the early warning indicators of RMPP and initiating timely intervention can reduce the occurrence of RMPP complications and reduce the mortality rate. This study was aimed at developing a risk prediction nomogram for prediction of RMPP patients.
The results of this study showed no statistically significant differences between sex and age in the RMPP and GMPP groups. Compared with the GMPP group, the RMPP group had longer thermal duration, higher thermal peak, higher incidence of consolidation, and pleural effusion. This is consistent with the research results of Shen et al. (3), who uncovered significant differences in WBC, NEP, PCT, CRP, LDH, ALB, and SUA between the two groups. The logistic regression analysis found that the fever duration, pleural effusion, WBC, NEP, CRP, LDH, NLR, and SUA were independent predictors of RMPP. The fever duration and laboratory indicators (WBC, NEP, CRP, LDH, NLR, and SUA) were included to draw the ROC curves. The AUC values were 0.861, 0.730, 0.758, 0.837, 0.868, 0.713, and 0.744 and the best cutoff values were 10.50, 10.13, 6.43, 29.45, 370.50, 3.47, and 170.50 respectively. The best predictor of the above laboratory indicators was the LDH, with a sensitivity and specificity of 0.837 and 0.837, respectively. The newly discovered indicator was the SUA levels. When the cutoff value of SUA was 170.50, the sensitivity and specificity were 0.651 and 0.742, respectively.
The nomogram model can evaluate the prognosis intuitively, concisely, and accurately, which helps medical staff make better clinical decisions. In this study, we screened the predictive variables for RMPP through logistic regression analysis to build a nomogram. Finally, fever duration, pleural effusion, WBC, NEP, CRP, LDH, NLR, and SUA were entered into the predictive model. The results of this study show that the prediction model has good prediction effect and calibration degree, and the internal validation results confirm that the model has good performance. The DCA showed that the nomogram model can bring clinical benefit to patients within a large threshold range. Cheng et al. (15) used LASSO regression analysis to screen for risk factors for RMPP and found that high fever, ALB, LDH, and the neutrophil ratio were 4 independent risk factors; the AUC of the nomogram was greater than 0.8 in both the training and validation groups. Bi et al. (16) took six indicators, including age, fever days, CRP, alanine aminotransferase (ALT), LDH, and chest imaging score, and developed a predictive nomogram for RMPP with a sensitivity of 78.3% and a specificity of 86.2%. The AUC of the predictive scale was 0.871. The clinical predictive model included in this study showed an AUC of 0.956, which was significantly higher than the above two study models. The results of this study were similar to the model predictive power of the study conducted by Liu et al. (17). The results of their study showed that consolidation size/body surface area (BSA), pleural effusion, CRP, and LDH were significant indicators of RMPP development, and were subsequently used to develop a nomogram prediction tool. The AUC for the predictive nomogram was 0.955 in the training cohort and 0.916 in the validation cohort.
The results of this study showed that the sensitivity and specificity of LDH were 0.837 and 0.837, respectively, which were better predictors. LDH is a non-specific inflammatory marker, mainly involved in anaerobic glucose metabolism. LDH catalyzes the interconversion of lactate and pyruvate, and that of nicotinamide adenine dinucleotides and nicotinamide adenine dinucleotides. LDH is present in the cytoplasm of cells in all tissues, released to the extracellular matrix upon cell membrane damage or cell lysis, and is a surrogate marker for tissue damage. The results of many Chinese and international studies suggest that LDH and CRP have an important role in the early identification of RMPP and the utility time of glucocorticoid (18-21), but large sample studies are still needed to determine their value. Huang et al. showed that LDH greater than 339.0 U/L was an independent risk factor for predicting RMPP (5). Another result suggested that the best cut-off value of LDH for predicting RMPP was 384.5 U/L and the sensitivity and specificity were 78.1% and 80.8%, respectively, which is more consistent with the best cut-off value of this study (370.50 U/L) (22).
SUA is produced in the liver by xanthine oxidase oxidation, and it is the end-product of purine metabolism in the human body. It exists in the blood and is excreted with urine, and the kidneys play an important role in the regulation of uric acid level in the body, accounting for 60–70% of uric acid excretion (23). Therefore, renal function impairment or purine metabolism disorders cause changes in the body’s uric acid levels (24). Uric acid has a variety of biological effects in the human body, which can promote the inflammatory response, and can also serve as an antioxidant and endogenous free radical scavenger; it is also closely related to adult chronic obstructive pulmonary disease. In addition to chronic obstructive pulmonary disease, respiratory diseases are also closely associated with pulmonary interstitial fibrosis, asthma, obstructive sleep apnea, and hypoventilation syndrome, lung cancer, and pneumonia. Meanwhile, uric acid is also present in the occurrence and development of diabetes, and liver, kidney, cardiovascular, cerebrovascular, and other systemic diseases (25,26). The majority of patients in the intensive care unit usually experience a variety of pathogenic processes, such as ischemia–reperfusion injury, inflammation, and coagulopathy. Uric acid may be a factor involved in the aforementioned pathogenic processes and may be of potential value for assessing changes in clinical conditions and disease outcomes. The results of this study suggest that a decreased SUA level may be a predictor of RMPP. The AUC was 0.744; the best cutoff value was 170.5 µmol/mL; the sensitivity was 0.651; and the specificity was 0.742. Although SUA is not the best predictor, the SUA level is a widely available and low-cost predictive RMPP index, which has certain clinical value. In addition, the results of multiple linear regression analysis showed a positive linear relationship between CRP, LDH, and NLR and the duration of the fever, suggesting that CRP, LDH, and NLR increased with the delay of fever duration. There is a negative linear relationship between SUA and the duration of the fever, suggesting that the SUA level decreases with the extension of fever duration, and whether it is of great significance for the pathogenesis, treatment, and prognosis of RMPP needs further study.
The mechanism of the reduced SUA levels in RMPP patients is unknown. The study found that the concentration of uric acid in respiratory epithelial tissue fluid was high, and the uric acid of respiratory epithelial lining fluid played an antioxidant effect together with other endogenous antioxidants and ascorbic acid (27). Uric acid was found to act as an endogenous modulator of innate immunity, so it is tempting to speculate that low levels of SUA may exacerbate the cytokine storm during coronavirus disease 2019 (COVID-19) (28,29). Recent evidence suggests that acute and severe hypouricemia induced in healthy individuals causes endothelial cell dysfunction (30). Recently, researchers have focused on SUA levels in COVID-19, believing that hyperuricemia in COVID-19 patients may lead to acute kidney injury and adverse outcome (31). A most recent clinical study showed that SUA exhibits protective effects in the pediatric MPP process, with an anti-inflammatory effect (32). All the above suggest that reduced SUA level is a risk factor for disease, but the specific mechanism needs further study.
Limitations and strengths
This study showed that SUA level and NLR are a widely available and low-cost predictive index with good clinical value. SUA levels and NLR indicators were added to the nomogram, which could more comprehensively and fully help clinicians to predict RMPP, especially in primary care hospitals. Moreover, this study is retrospective, and there may have been bias in case selection, which requires further validation by large sample prospective studies.
ConclusionsOther Section
Fever duration of more than 10.5 days, pleural effusion, WBC >10.13×109/L, NEP >6.43×109/L, CRP >29.45 mg/L, LDH >370.50 U/L, NLR >3.47 and SUA <170.5 µmol/mL are early predictors of RMPP. This study shows that SUA and NLR are a widely available and cost-effective predictor with certain clinical value, and can provide a reference for clinicians, especially primary hospitals to diagnose RMPP. The developed nomogram, which has a satisfactory level of accuracy and good calibration, can be utilized to predict RMPP patients. The model has been found to be useful in clinical practice and is clinically interpretable. In addition, the study found that the SUA level decreased with the extension of the fever duration, and whether this is of great significance to the pathogenesis, treatment, and prognosis of RMPP needs to be further studied. However, as this study was conducted retrospectively, there may have been bias in case selection, thus further validation is required via large sample prospective studies.
AcknowledgmentsOther Section
We would like to express our gratitude to the physicians from Xi’an Children’s Hospital for their significant contribution to the data collection.
Funding: This study was supported by grants from
FootnoteOther Section
Reporting Checklist: The authors have completed the TRIPOD reporting checklist. Available at https://tp.amegroups.com/article/view/10.21037/tp-24-16/rc
Data Sharing Statement: Available at https://tp.amegroups.com/article/view/10.21037/tp-24-16/dss
Peer Review File: Available at https://tp.amegroups.com/article/view/10.21037/tp-24-16/prf
Conflicts of Interest: Both authors have completed the ICMJE uniform disclosure form (available at https://tp.amegroups.com/article/view/10.21037/tp-24-16/coif). The authors have no conflicts of interest to declare.
Ethical Statement: The authors are accountable for all aspects of the work in ensuring that questions related to the accuracy or integrity of any part of the work are appropriately investigated and resolved. The study was conducted in accordance with the Declaration of Helsinki (as revised in 2013). This study was approved by the Medical Ethics Committee of Xi’an Children’s Hospital (reference number: 20230004) and informed consent was provided by the parents or legal guardians of the children.
Open Access Statement: This is an Open Access article distributed in accordance with the Creative Commons Attribution-NonCommercial-NoDerivs 4.0 International License (CC BY-NC-ND 4.0), which permits the non-commercial replication and distribution of the article with the strict proviso that no changes or edits are made and the original work is properly cited (including links to both the formal publication through the relevant DOI and the license). See: https://creativecommons.org/licenses/by-nc-nd/4.0/.
ReferencesOther Section
- Jain S, Williams DJ, Arnold SR, et al. Community-acquired pneumonia requiring hospitalization among U.S. children. N Engl J Med 2015;372:835-45. [Crossref] [PubMed]
- Tong L, Huang S, Zheng C, et al. Refractory Mycoplasma pneumoniae Pneumonia in Children: Early Recognition and Management. J Clin Med 2022;11:2824. [Crossref] [PubMed]
- Shen F, Dong C, Zhang T, et al. Development of a Nomogram for Predicting Refractory Mycoplasma pneumoniae Pneumonia in Children. Front Pediatr 2022;10:813614. [Crossref] [PubMed]
- Wang S, Jiang Z, Li X, et al. Diagnostic value of serum LDH in children with refractory Mycoplasma pneumoniae pneumoniae: A systematic review and meta-analysis. Front Pediatr 2023;11:1094118. [Crossref] [PubMed]
- Huang X, Li D, Liu F, et al. Clinical significance of D-dimer levels in refractory Mycoplasma pneumoniae pneumonia. BMC Infect Dis 2021;21:14. [Crossref] [PubMed]
- Li M, Chen Y, Li H, et al. Serum CXCL10/IP-10 may be a potential biomarker for severe Mycoplasma pneumoniae pneumonia in children. BMC Infect Dis 2021;21:909. [Crossref] [PubMed]
- Fan H, Lu B, Yang D, et al. Distribution and Expression of IL-17 and Related Cytokines in Children with Mycoplasma pneumoniae Pneumonia. Jpn J Infect Dis 2019;72:387-93. [Crossref] [PubMed]
- Zhang WH, Zhou MP, Zou YY, et al. The predictive values of soluble B7-DC in children with refractory mycoplasma pneumoniae pneumonia. Transl Pediatr 2023;12:396-404. [Crossref] [PubMed]
- Zhu J, Liu X, Zhan X, et al. Predictive value of chemokines (CCL 2) in bronchoalveolar lavage fluid for refractory mycoplasma pneumonia in children. Ital J Pediatr 2023;49:125. [Crossref] [PubMed]
- Kajana V, Massaro G, Somaschini A, et al. Serum uric acid in patients with ST-segment elevation myocardial infarction: An innocent bystander or leading actor? Nutr Metab Cardiovasc Dis 2022;32:1583-9. [Crossref] [PubMed]
- Han R, Zhang Y, Jiang X. Relationship Between Four Non-Insulin-Based Indexes of Insulin Resistance and Serum Uric Acid in Patients with Type 2 Diabetes: A Cross-Sectional Study. Diabetes Metab Syndr Obes 2022;15:1461-71. [Crossref] [PubMed]
- Yilmaz H, Yalcin KS, Namuslu M, et al. Neutrophil-Lymphocyte Ratio (NLR) Could Be Better Predictor than C-reactive Protein (CRP) for Liver Fibrosis in Non-alcoholic Steatohepatitis (NASH). Ann Clin Lab Sci 2015;45:278-86.
- Kutty PK, Jain S, Taylor TH, et al. Mycoplasma pneumoniae Among Children Hospitalized With Community-acquired Pneumonia. Clin Infect Dis 2019;68:5-12. [Crossref] [PubMed]
- Poddighe D. Mycoplasma pneumoniae-related hepatitis in children. Microb Pathog 2020;139:103863. [Crossref] [PubMed]
- Cheng S, Lin J, Zheng X, et al. Development and validation of a simple-to-use nomogram for predicting refractory Mycoplasma pneumoniae pneumonia in children. Pediatr Pulmonol 2020;55:968-74. [Crossref] [PubMed]
- Bi Y, Zhu Y, Ma X, et al. Development of a scale for early prediction of refractory Mycoplasma pneumoniae pneumonia in hospitalized children. Sci Rep 2021;11:6595. [Crossref] [PubMed]
- Liu G, Wang G, Yang Z, et al. A Lung Ultrasound-Based Nomogram for the Prediction of Refractory Mycoplasma pneumoniae Pneumonia in Hospitalized Children. Infect Drug Resist 2022;15:6343-55. [Crossref] [PubMed]
- Wen J, Su Y, Sun H, et al. The combination of initial markers to predict refractory Mycoplasma pneumoniae pneumonia in Chinese children: a case control study. Respir Res 2021;22:89. [Crossref] [PubMed]
- Gong H, Sun B, Chen Y, et al. The risk factors of children acquiring refractory mycoplasma pneumoniae pneumonia: A meta-analysis. Medicine (Baltimore) 2021;100:e24894. [Crossref] [PubMed]
- Zhang J, Wang T, Li R, et al. Prediction of risk factors of bronchial mucus plugs in children with Mycoplasma pneumoniae pneumonia. BMC Infect Dis 2021;21:67. [Crossref] [PubMed]
- Zhao J, Ji X, Wang Y, et al. Clinical Role of Serum Interleukin-17A in the Prediction of Refractory Mycoplasma pneumoniae Pneumonia in Children. Infect Drug Resist 2020;13:835-43. [Crossref] [PubMed]
- Zheng XX, Lin JL, Dai JH. Value of lactate dehydrogenase in predicting refractory Mycoplasma pneumoniae pneumonia in children: an evaluation based on decision curve analysis and dose-response analysis. Zhongguo Dang Dai Er Ke Za Zhi 2020;22:112-7. [Crossref] [PubMed]
- Kim GH, Jun JB. Altered Serum Uric Acid Levels in Kidney Disorders. Life (Basel) 2022;12:1891. [Crossref] [PubMed]
- Yanai H, Adachi H, Hakoshima M, et al. Molecular Biological and Clinical Understanding of the Pathophysiology and Treatments of Hyperuricemia and Its Association with Metabolic Syndrome, Cardiovascular Diseases and Chronic Kidney Disease. Int J Mol Sci 2021;22:9221. [Crossref] [PubMed]
- Otani N, Ouchi M, Misawa K, et al. Hypouricemia and Urate Transporters. Biomedicines 2022;10:652. [Crossref] [PubMed]
- Allegrini S, Garcia-Gil M, Pesi R, et al. The Good, the Bad and the New about Uric Acid in Cancer. Cancers (Basel) 2022;14:4959. [Crossref] [PubMed]
- Lee HW, Choi SM, Lee J, et al. Serum Uric Acid Level as a Prognostic Marker in Patients With Acute Respiratory Distress Syndrome. J Intensive Care Med 2019;34:404-10. [Crossref] [PubMed]
- Luis-Rodríguez D, Donate-Correa J, Martín-Núñez E, et al. Serum urate is related to subclinical inflammation in asymptomatic hyperuricaemia. Rheumatology (Oxford) 2021;60:371-9. [Crossref] [PubMed]
- Ma Q, Honarpisheh M, Li C, et al. Soluble Uric Acid Is an Intrinsic Negative Regulator of Monocyte Activation in Monosodium Urate Crystal-Induced Tissue Inflammation. J Immunol 2020;205:789-800. [Crossref] [PubMed]
- De Becker B, Coremans C, Chaumont M, et al. Severe Hypouricemia Impairs Endothelium-Dependent Vasodilatation and Reduces Blood Pressure in Healthy Young Men: A Randomized, Placebo-Controlled, and Crossover Study. J Am Heart Assoc 2019;8:e013130. [Crossref] [PubMed]
- Chauhan K, Pattharanitima P, Piani F, et al. Prevalence and Outcomes Associated with Hyperuricemia in Hospitalized Patients with COVID-19. Am J Nephrol 2022;53:78-86. [Crossref] [PubMed]
- Pan C, Chen Y, Wang S, et al. The study of routine laboratory factors in children with mycoplasma pneumoniae pneumonia: serum uric acid may have anti-inflammatory effect. J Clin Lab Anal 2021;35:e24026. [Crossref] [PubMed]
(English Language Editor: J. Jones)