Identification and analysis of inflammation-related biomarkers in tetralogy of Fallot
Highlight box
Key findings
• This study investigated the 5 inflammation-related biomarkers in tetralogy of Fallot (TOF) based on bioinformatics methods.
What is known and what is new?
• The inflammatory response plays an important role in the development of TOF, and pro-inflammatory factors can be re-synthesised in the myocardium of TOF patients.
• Our study identified inflammation-related biomarkers in TOF based on bioinformatics, providing a new direction and theoretical basis for TOF research.
What is the implication, and what should change now?
• Biomarkers would help clinicians to screen the patients of primary immunodeficiency with TOF, which are potential methods to better guide treatment. Although this study explored the biomarkers in depth, further clinical validation is still necessary.
Introduction
Tetralogy of Fallot (TOF) is the most common cyanotic congenital heart disease (CHD) diagnosed in liveborn children (1). TOF accounts for approximately 7% to 10% of all CHD cases and has a prevalence of approximately 3 in 10,000 neonates (2). The development of TOF is characterized by four main cardiac morphological defects: pulmonary stenosis, ventricular septal defect, right ventricular hypertrophy, and overriding aorta and is often accompanied by right ventricular outflow tract obstruction (3). TOF can be caused by both genetic and environmental factors (4). The genetic factors include single or multiple mutations (e.g., GATA4, JAG1, TBX5, etc.), chromosome number or structure abnormalities (e.g., 1p21.1 copy number variation, 22q11 microdeletion, 15q11.2 microdeletion, etc.), and epigenetic modifications based on gene methylation (e.g., methylation of LINE1, NKX2-5, ZFPM2, etc.) (5-9). The environmental factors include physical factors (such as ionizing radiation and noise pollution), chemical factors (such as long-term exposure to organic solvents, cocaine, etc.), biological factors (such as viral infection in early pregnancy), and psychosocial factors (such as stress and anxiety of pregnant women) (10,11). Besides, maternal diabetes may also be an important factor in inducing TOF. However, so far, the specific pathogenesis of TOF has not been fully clarified.
Systemic chronic inflammation and immune activation are closely associated with the progression and development of various cardiovascular diseases (12). CHD is a cardiovascular malformation resulting from abnormal cardiovascular development during the fetal period. A range of hemodynamic factors can trigger the immune system in patients with severe CHD. This activation can result in cardiac damage, hypoxia, edema, and hypoperfusion. Subsequently, these conditions can lead to the development of chronic inflammation as well as complications associated with CHD. Conversely, systemic inflammation with a remote injury can induce a local myocarditis response mediated by cardiac macrophages (13). Previous study has shown that in cyanotic children with CHD, myocarditis triggers the activation of inflammation-related pathways, such as IL-6-JAK-STAT and NF-κB pathways and the increase of IL-6, C-myc, and SOCS3 expression levels (14). Pro-inflammatory cytokines (TNF-α, IL-1-β, IL-6, IL-10) are synthesized in the myocardium of TOF patients, associated with the activation of NF-κB and p38-MAPK pathways (15). Therefore, inflammation-related genes may play an important role in the development of TOF, but its specific inflammation-related biomarkers and mechanisms of action still need to be further explored.
In this study, based on the Gene Expression Omnibus (GEO) database, a comprehensive bioinformatics analysis approach was used to identified inflammation-related genes in TOF. Moreover, a lncRNA-miRNA-mRNA regulatory network was established. The differences in the abundance of immune cell infiltration between TOF and controls were analyzed, and the correlation between biomarkers and infiltrating immune cells was also explored. This research contributes to a further understanding of TOF, providing a theoretical basis for the in-depth study of TOF. We present this article in accordance with the TRIPOD reporting checklist (available at https://tp.amegroups.com/article/view/10.21037/tp-24-8/rc).
Methods
Data extraction
TOF-related datasets (GSE36761 and GSE35776) were downloaded from the GEO database (https://www.ncbi.nlm.nih.gov/). The GSE36761 was applied as the training set, including 22 TOF samples and 8 control samples (16), and they were from Germany. The GSE35776 contained 16 TOF samples and 8 control samples, which was used as a validation set and were from USA (17). In total, 200 inflammation-related genes (IRGs) were downloaded from the ‘Hallnark-inflammatory response’ gene set from the Molecular Signatures Database (MSigDB, http://www.gsea-msigdb.org/gsea/msigdb/). The study was conducted in accordance with the Declaration of Helsinki (as revised in 2013).
Identification and function enrichment analysis of differential expressed IRGs (DE-IRGs) between TOF and control groups
Firstly, the differentially expressed genes (DEGs) between TOF and control groups in the training set were identified via the “DESeq2” package (version 1.26.0) with adj P<0.05 and |log2 fold change (FC)| >1.5 (18). The DE-IRGs were gained through taking the intersection of the DEGs between TOF and control groups and IRGs via Venn tool. Afterwards, the biological functional enrichment analysis of DE-IRGs was carried out via the “clusterProfiler” R package (version 4.0.2), based on Gene Ontology (GO) and Kyoto Encyclopedia of Genes and Genomes (KEGG) (adj P<0.05 and count >1) (19).
Screening of the biomarkers
Based on the DE-IRGs, we performed the least absolute shrinkage and selection operator (LASSO) and random forest (RF) to further identified the characteristic genes, respectively. Then, the biomarkers related to IRGs in TOF were identified by taking the intersection of characteristic genes screened by the two machine learning algorithms, which the diagnostic value for TOF was further assessed using receiver operating characteristic (ROC) curves in the GSE36761 and GSE35776. Additionally, the nomogram containing the biomarkers was established via lrm function of “RMS” R package (version 6.2-0) to predict TOF patient survival. The corresponding calibration curves were plotted to assess the reliability of the nomogram.
Immune analysis
To explore the relationship between biomarkers and immune microenvironment. The single sample gene set enrichment analysis (ssGSEA) algorithm was utilized to assess the infiltrating abundance of 28 immune cells in TOF and control groups (20). The differences between TOF and control groups were compared by Wilcox. test. The relevance between biomarkers and differential immune cells was evaluated via Spearman algorithm.
Gene set enrichment analysis (GSEA) of the biomarkers
To explore enrichment pathways of the biomarkers in TOF, we performed GSEA via “ClusterProfiler” R package (version 4.0.2) (adj P<0.05) (19). The correlation between the biomarkers and all genes of all samples (TOF samples and control samples) was calculated via Pearson correlation analysis, and ranked according to the correlation.
Construction of competing endogenous RNA (ceRNA) network
To better understand the mechanisms for the biomarkers in TOF, we created the ceRNA network. The ceRNAs are a class of RNA molecules that can competitively regulate each other’s expression by sharing miRNA regulatory elements. The miRNAs regulating the biomarkers were predicted via miRWalk database (http://mirwalk.umm.uni-heidelberg.de/) and starbase (https://starbase.sysu.edu.cn/). The predicted miRNAs of the two databases were intersected to obtain the final miRNAs. The mirnet database (https://www.mirnet.ca/miRNet/home.xhtml) and starbase database were utilized to predict the lncRNAs with regulatory interactions with miRNAs. The lncRNAs finally were got via taking the intersection of predicted lncRNAs of the two databases. Finally, the Cytoscape software (version 3.8.2) was utilized to visualize the lncRNA-miRNA-mRNA network (21).
Construction of transcription factor (TF)-mRNA network
TFs play a crucial role in organisms as another substance that can regulate gene expression. TFs control chromatin and transcription by recognising specific DNA sequences to form a complex system that directs the expression of genomic. We created a TF-mRNA network to better understand the mechanism underlying the biomarkers in TOF. The TFs of the biomarkers were predicted through NetworkAnalyst (http://www.networkanalyst.ca/faces/home.xhtml). The filter conditions were intensity signal <500 and score <1.
Single nucleotide polymorphism (SNP) analysis
The miRNASNP database (bioinfo.life.hust.edu.cn/miRNASNP/#!) was adopted to predicted the SNPs in the seed regions of the miRNAs, and the SNP locations in the seed region of the miRNAs affected biomarkers were screened (22). Afterwards, the miRNA-SNP-biomarker network was visualized via Cytoscape software (version 3.8.2) (21).
Drug prediction of the biomarkers
Each biomarker was used as the key word to search drugs interacting with biomarkers in the Genecards database (http://www.genecards.org). Then, Cytoscape software (version 3.8.2) was utilized to visualize the biomarker-drug network.
Expression analysis of the biomarkers
To further demonstrate the responsibility of our results, the expression levels of the biomarkers between TOF and control groups were compared in the GSE36761 and the GSE35776 via Wilcox test (P<0.05).
Statistical analysis
Statistical analysis was carried out through R software (version 4.1.1, https://www.r-project.org/). Differences between groups were analyzed via the Wilcox test. P<0.05 represented a significant difference.
Results
Acquisition and enrichment analysis of DE-IRGs
There were 971 DEGs between TOF and control groups, containing 358 up-regulated genes and 613 down-regulated genes (Figure 1A,1B). The DEGs were significantly enriched to produce 428 GO entries [321 biological progress (BP), 38 cellular component (CC), 69 molecular function (MF)] and 11 KEGG pathways, among which the signaling pathways related to inflammatory included complement and coagulation cascades, neuroactive ligand-receptor interaction, staphylococcus aureus infection, etc. (table available at https://cdn.amegroups.cn/static/public/tp-24-8-1.xlsx, Tables S1,S2). Then, 29 DE-IRGs were obtained, including 5 up-regulated genes (F3, NMUR1, CCL17, ICAM4, and RGS1) and 24 down-regulated genes (AQP9, CCL2, CCR7, CD14, CSF3R, CXCL6, ADGRE1, FFAR2, FPR1, HAS2, IL10, IL15RA, LIF, MARCO, MEFV, OSMR, PROK2, PTGER2, SELE, SERPINE1, SLAMF1, SLC7A1, SLC7A2, and TIMP1) (Figure 1C-1E). Subsequently, the DE-IRGs were significantly enriched 225 GO entries (table available at https://cdn.amegroups.cn/static/public/tp-24-8-2.xlsx), including leukocyte migration, cell chemotaxis, leukocyte chemotaxis, and so on (Figure 1F). Meanwhile, a total of 13 KEGG signaling pathways were obtained (Table S3), including iral protein interaction with cytokine and cytokine receptor, cytokine-cytokine receptor interaction, JAK-STAT signaling pathway, etc. (Figure 1G).
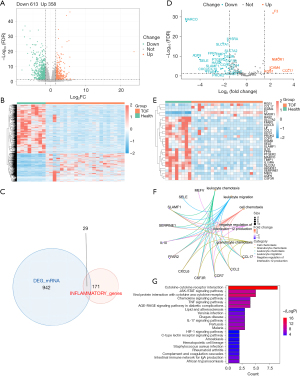
Acquisition of the biomarkers
In the LASSO algorithm, five characteristic genes were obtained, namely MARCO, CXCL6, F3, SLC7A2, and SLC7A1 (Figure 2A). For the RF algorithm, the accuracy of the model was optimal when the number of characteristic genes was 8 (Figure 2B). Therefore, we obtained eight characteristic genes through RF algorithm, namely CXCL6, SLC7A1, F3, MARCO, IL15RA, SLC7A2, FRP1, and CD14 (Figure 2C). Subsequently, five biomarkers were gained, namely MARCO, CXCL6, F3, SLC7A2, and SLC7A1 (Figure 2D). The area under curve (AUC) values of diagnostic model based on the training set and validation set were 1 and 0.97, respectively (Figure 2E,2F). These results suggested that the diagnostic model had good efficacy for TOF. Additionally, the nomogram containing the five biomarkers was generated to predict the probability of TOF disease occurrence (Figure 2G). The corresponding calibration curves suggested that the predicted performance for nomogram was close to the ideal model, indicating that the nomogram had wonderful predictive accuracy for the TOF (Figure 2H).
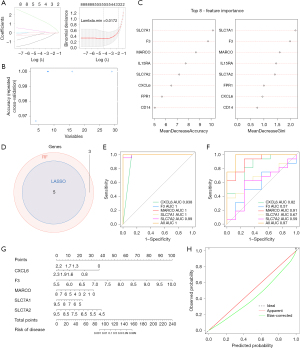
Immune analysis between TOF and control groups
The immune scores of 28 immune cells in TOF and control groups were demonstrated via the heatmap (Figure 3A). There were 18 immune cells with a significantly different level between TOF and control groups, such as activated B cells, neutrophil, type1 T helper cell, etc. (Figure 3B). The result of correlation analysis suggested that CXCL6 was significantly positively correlated with all differential immune cells (Figure 3C). The MARCO, SLC7A2, and SLC7A1 were markedly positively correlated with most differential immune cells, such as activated dendritic cells, central memory CD4 T cell, type1 T helper cell, etc., while F3 was significantly negatively correlated with most differential immune cells, such as activated dendritic cells, central memory CD4 T cell, type1 T helper cell, etc. (Figure 3C).
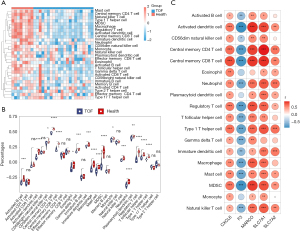
Enrichment analysis of the biomarkers
To further understand the impact of the biomarkers on TOF, we performed GSEA enrichment analysis. The top 10 GO items and KEGG pathways enriched were demonstrated. Of the GO results, MARCO, CXCL6, SLC7A1, and SLC7A2 were negatively related to RNA splicing, via transesterification reactions, mRNA splicing, via spliceosome, and RNA splicing, via transesterification reactions with bulged adenosine as nucleophile (Figure 4A-4D). And these genes were positively related to response to molecule of bacterial origin, activation of immune response, and adaptive immune response (Figure 4A-4D). However, the correlation of F3 with these pathways was the opposite (Figure 4E).
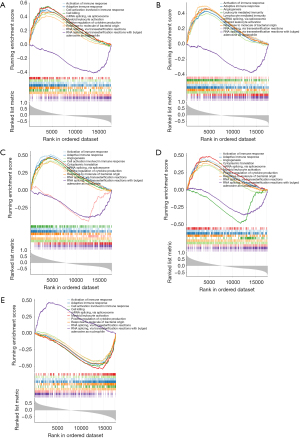
Of the KEGG results, MARCO, CXCL6, SLC7A1, and SLC7A2 were positively relevant to pathogenic Escherichia coli infection and tuberculosis (Figure 5A-5D), while the correlation of F3 with two pathways was the opposite (Figure 5E). MARCO, CXCL6, F3, and SLC7A1 were related to phagosome, complement and coagulation cascades, osteoclast differentiation, herpes simplex virus 1 infection, and salmonella infection (Figure 5A-5C,5E). Additionally, MARCO, CXCL6, F3, and SLC7A2 were related to cytokine-cytokine receptor interaction (Figure 5A,5B,5D,5E).
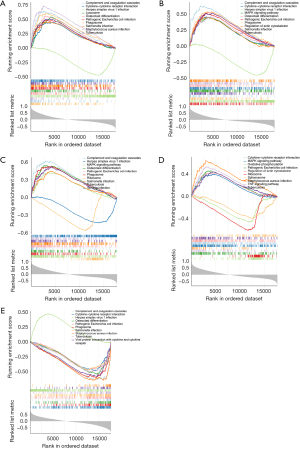
The lncRNA-miRNA-mRNA networks
Based on the miRWalk and starbase databases, a total of 5 miRNAs were predicted at the same time (Figure 6A). Based on the mirnet and starbase databases, 622 and 228 lncRNAs were predicted, respectively. Afterwards, the lncRNAs predicted via the two databases were intersected to gain 228 lncRNAs (Figure 6B). Finally, the lncRNA-miRNA-mRNA network was created, containing 4 mRNAs, 4 miRNAs, 228 lncRNAs, and 300 lncRNA-miRNA-mRNA pairs (Figure 6C). For instance, the regulatory relationships included ERICH3-AS1-hsa-miR-381-3p-F3, NPPA-AS1-hsa-miR-520d-3p-CXCL6, LINC01128-hsa-miR-214-3p-F3, LINC01128-hsa-miR-195-5p-SLC7A2, TNFRSF14-AS1-hsa-miR-195-5p-SLC7A2 etc.
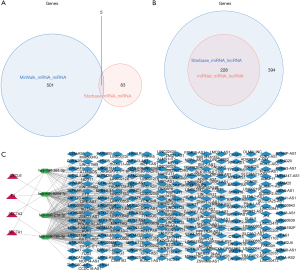
TFs-mRNA network
In this study, 10 TFs related to TFs were predicted, such as SMC3, NR2F2, MXD3, etc. (Figure 7). Besides, 9 TFs were predicted for SLC7A1, such as CHD1, EED, MXD4, etc. (Figure 7). The TF ARID4B could regulate F3 and SLC7A1. SP3 was a common TF for both SLC7A2 and CXCL6 (Figure 7). Finally, the TF-mRNA network was established, containing 4 mRNAs, 31 TFs, and 33 edges, for instance, ELF1-CXCL6, CBX8-SLC7A2, ZNF423-SLC7A1, ZNF71-F3 (Figure 7).
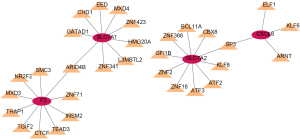
The miRNA-SNP-mRNA network
Potential miRNAs targeting biomarkers were analyzed via using miRNASNP to determine how SNP variants in the miRNA seed region affect the binding of the 3' untranslated regions (UTR) region of biomarker genes. Figure 8 shows the miRNA-SNP-mRNA network. A total of nine SNP locations were identified, which might play an important role in binding to biomarkers (Table 1).
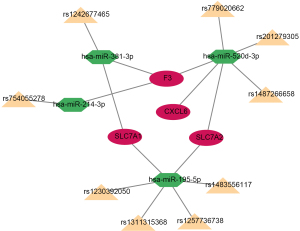
Table 1
Target gene | miRNA | SNP |
---|---|---|
F3 | hsa-miR-214-3p | rs754055278 |
SLC7A1 | hsa-miR-381-3p | rs1242677465 |
F3 | hsa-miR-381-3p | rs1242677465 |
SLC7A1 | hsa-miR-195-5p | rs1230392050 |
SLC7A1 | hsa-miR-195-5p | rs1257736738 |
SLC7A1 | hsa-miR-195-5p | rs1483556117 |
SLC7A1 | hsa-miR-195-5p | rs1311315368 |
SLC7A2 | hsa-miR-195-5p | rs1230392050 |
SLC7A2 | hsa-miR-195-5p | rs1257736738 |
SLC7A2 | hsa-miR-195-5p | rs1483556117 |
SLC7A2 | hsa-miR-195-5p | rs1311315368 |
CXCL6 | hsa-miR-520d-3p | rs779020662 |
CXCL6 | hsa-miR-520d-3p | rs201279305 |
CXCL6 | hsa-miR-520d-3p | rs201279305 |
CXCL6 | hsa-miR-520d-3p | rs1487266658 |
SLC7A2 | hsa-miR-520d-3p | rs779020662 |
SLC7A2 | hsa-miR-520d-3p | rs201279305 |
SLC7A2 | hsa-miR-520d-3p | rs201279305 |
SLC7A2 | hsa-miR-520d-3p | rs1487266658 |
F3 | hsa-miR-520d-3p | rs779020662 |
F3 | hsa-miR-520d-3p | rs201279305 |
F3 | hsa-miR-520d-3p | rs201279305 |
F3 | hsa-miR-520d-3p | rs1487266658 |
SNPs, single nucleotide polymorphisms.
Drug prediction
We constructed a biomarker-drug interaction network by the GeneCards. The results showed that a total of 3 drugs were predicted for CXCL6, namely serine, calcium, and oxygen (Figure 9). And 8 drugs were predicted for F3, such as simvastatin, tisotumab vedotin, dabigatran, etc. The silicon dioxide and titanium dioxide were the target drugs for MARCO. We found that the ornithine, lysine, arginine, and nitric oxide were the common target drugs for SLC7A1 and SLC7A2.
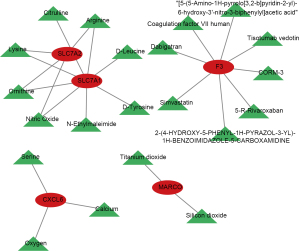
The expression levels of the biomarkers
We further analyzed the expression of the biomarkers between TOF and control groups in GSE36761 and GSE35776 via Wilcox test (Figure 10A,10B). The results demonstrated that the expression of all biomarkers between TOF and control groups was markedly different in GSE36761 (Figure 10A). In the GSE35776, the expression of CXCL6, MARCO, and SLC7A1 between TOF and control groups was significantly different (Figure 10B). Therefore, in both datasets, the expression trends of CXCL6, MARCO, SLC7A1, and SLC7A2 were the same, with low expression in the TOF group. These results indicated that all biomarkers have good diagnostic value for TOF.
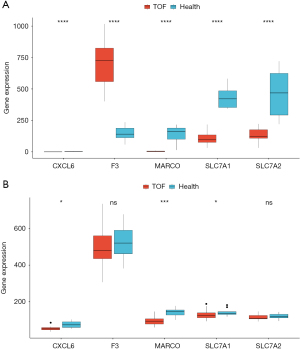
Discussion
To date, surgery has been the primary treatment option for TOF. However, there is an increasing trend of patients requiring subsequent surgeries (23). Therefore, it is important to explore effective biomarkers that can help explore better treatment options for TOF, which may ultimately provide additional treatment options for heart failure in TOF, thereby delaying re-intervention. However, this does not mean that initial repair is not required. Inflammation might be a factor that correlates heart failure in CHD with its complications. However, it has not yet received sufficient attention in clinical diagnosis or routine treatment.
With both LASSO and RF machine learning algorithms, five biomarkers associated with inflammation (CXCL6, F3, MARCO, SLC7A1, and SLC7A2) were obtained for TOF. CXCL6, also known as granulocyte chemotactic protein-2, is primarily expressed in the lungs, heart, liver, pancreas, brain, kidneys, and placenta, and initiates chemotaxis by binding to chemokine receptors C-X-C motif chemokine receptor 1 (CXCR1) and CXCR2 (24). Waehre et al. performed Affymetrix analysis of the right ventricle in mice with right ventricular pressure overload. The results showed upregulated expression of chemokines CXCL10, CXCL6, CX3CL1, CCL5, CXCL16, and CCL2, while CXCL16, CX3CL1, and CCL5 regulated SLRP expression in cardiac fibroblasts and post-translational modifications, suggesting that inflammatory mechanisms are associated with the development of right ventricular dysfunction (25). Wang et al. showed that CXCL6 and Sirt3 were downstream of HIF-1α and that CXCL6 regulates Sirt3 expression through AKT/FOXO3a activation, which in turn regulates permeability, proliferation, and apoptosis in human brain microvascular endothelial cells (HBMEC) after ischemia-reperfusion injury (26). F3, as the gene encoding coagulation factor iii, is a cell surface glycoprotein. Patients infected with severe acute respiratory syndrome coronavirus 2 (SARS-CoV-2) showed elevated levels of F3 transcripts and raised circulating extracellular vesicles, which may contribute to disease-associated coagulation, thrombosis, and increased mortality (27). MARCO, a macrophage receptor with collagenous structure is found on the surface of mucosal plasma membranes. Its function involves the incorporation of various extracellular substances into cells through macro-pinocytosis and endocytic pathways (28). It is closely associated with the prognosis of several tumors, such as bladder cancer, breast cancer, and lung squamous cell carcinoma (29). The cationic amino acid transporter proteins SLC7A1 (CAT1) and SLC7A2 (CAT2) are important arginine transporter proteins for T cells. They are members of the SLC7 family, which, together with members of the SLC1 family that are glutamate transporters, function in a variety of situations involving immune and inflammatory responses (such as viral infections, chronic intestinal inflammation, and tumor immunotherapy) by regulating astrocyte, macrophage, and T cell functions (30). In our study, we found for the first time that CXCL6, F3, MARCO, SLC7A1, and SLC7A2 are associated with associated with TOF and may lay the foundation for studies on the mechanisms of heart failure in TOF.
GSEA enrichment analysis showed that biomarkers were enriched to immune-related pathways, such as ‘activation of immune response’, ‘adaptive immune response’, ‘cytokine-cytokine receptor interaction’, etc. Chemokines can be categorized into four subfamilies, CXC, CC, XC, and CX3C, based on the arrangement of their cysteine residues near the amino terminus. The receptors corresponding to these chemokines are designated as CXCR, C-C motif chemokine receptor (CCR), XCR1, and CX3CR1, respectively. Sauce et al. showed that in pediatric patients with CHD, the level of serum CCL5 (RANTES) and macrophage migration inhibitory factor (MIF) were elevated, while the concentrations of angiogenic chemokine GROα, which is associated with impaired lung function, were decreased. Moreover, the plasma of adult patients with CHD exhibited higher levels of IL-1β, IL-8, and eotaxin, an eosinophilic protein of the CC chemokine subfamily (31). Wienecke et al. suggested that since CXCR4 or fractalkine and its receptor CX3CR1 regulate monocyte recruitment, platelet activation, and inflammation in cardiovascular disease, their study could be further developed in CHD regarding inflammation (12). However, overall, the current research in CHD regarding chemokines and their receptors is still not widely studied.
The current study showed that activated dendritic cells, immature dendritic cells, central memory CD4 T cells, central memory CD8 T cells, neutrophil, monocyte, natural killer T cells, mast cells, regulatory T cells (type 1), and bone marrow-derived suppressor cells (MDSCs) showed significant differences in the abundance of immune infiltration between TOF and controls. These differences also showed a significant correlation with biomarkers. Neutrophils are the first responders to the inflammatory signals released by cell and tissue injury, and the interaction between neutrophils and cardiomyocytes results in an elevated neutrophil-lymphocyte ratio (NLR) in patients with CHD before surgery (15,32-35). A study by Manuel et al. showed that for pediatric patients with CHD undergoing extracorporeal circulation, patients with cyanotic CHD had higher preoperative NLR levels (36). For patients with TOF undergoing postoperative repair, higher preoperative NLR is associated with longer intensive care unit (ICU) and hospital stays (37). Human monocytes are divided into three subpopulations, CD14++CD16− (Mon1, classical), CD14+CD16+ (Mon2, intermediate), and CD14+CD16++ (Mon3, non-classical), among which Mon2 and Mon3 are considered closely associated with inflammation. Their levels were found to be elevated in adult patients with CHD, and they increase in response to escalating severity of heart failure (38). Hamada et al. showed that increased expression of mast cell chymotrypsin was associated with early pulmonary vascular disease in the lung tissue of patients with CHD. However, the exact biological mechanism is still unknown (39). There are fewer studies on TOF and CHD from an immunological perspective, and there is a need for further research to enhance our understanding in this area.
In addition, it was predicted that lncRNAs, miRNAs, and TFs have regulatory roles with biomarkers through online databases. Zhang et al. showed that FGD5-AS1 is a pivotal lncRNA in the TOF cardiac ceRNA network and might play a repressive role in cardiac development by regulating the transcription of genes associated with CHD (40). Liang et al. showed that reducing miRNA-940 expression affects the proliferation and migration of progenitor cells in the secondary heart field by targeting JARID2, leading to the development of TOF (41). Yu et al. showed that hsa-miR-16 and hsa-miR-124 are key miRNAs for TOF and mainly regulate the expression of NT5DC1, ECHDC1, HSDL2, FCHO2, and ACAA2, which are involved in the rate of ATP conversion and fatty acid metabolism in mitochondria (42). However, to learn more about how the lncRNA-miRNA-mRNA and TF-mRNA connection pairs with regulatory effects discovered in our work are regulated by one another, further research is required. Previous study has shown that miRNA-related SNPs may affect disease susceptibility and phenotype in an SNP genotype-dependent manner by altering the regulation of miRNAs (43). In the present study, a miRNA-SNP-mRNA network was established. The findings propose that nine SNPs could potentially function as intermediaries between miRNAs and mRNAs. However, the exact mechanisms through which they operate require a more comprehensive study. Moreover, potential drugs were identified using biomarkers as targets, but there are fewer studies on the correlation between the predicted drugs and TOF, and further in-depth studies are needed.
However, this study has several limitations. First, further experimental studies should be conducted to elaborate the molecular mechanism of TOF, focusing on inflammation. Second, more TOF samples need to be collected and studied to elaborate the role of biomarkers and their associated pathways in the mechanism of TOF. Additionally, the sample sizes in the database were small and information on the clinical status of subjects in these datasets was not provided. Moreover, there were also limitations bioinformatics tools. In LASSO analyses, multiple comparisons are required to control the false discovery rate when choosing appropriate regularisation parameter values to avoid false positive results due to too many variables. While in RF, for data with differently valued attributes, attributes with more value divisions will have a greater impact on RFs. And RFs have been shown to overfit some noisy classification or regression problems.
Conclusions
In summary, this research identified five inflammation-related genes in TOF bases on bioinformatics techniques, which may help us to think about the potential adaptive and maladaptive responses to TOF in patients and may provide a theoretical basis for the study of future prognostic indicators or treatment targets of TOF.
Acknowledgments
Funding: This work was supported by
Footnote
Reporting Checklist: The authors have completed the TRIPOD reporting checklist. Available at https://tp.amegroups.com/article/view/10.21037/tp-24-8/rc
Peer Review File: Available at https://tp.amegroups.com/article/view/10.21037/tp-24-8/prf
Conflicts of Interest: All authors have completed the ICMJE uniform disclosure form (available at https://tp.amegroups.com/article/view/10.21037/tp-24-8/coif). The authors have no conflicts of interest to declare.
Ethical Statement: The authors are accountable for all aspects of the work in ensuring that questions related to the accuracy or integrity of any part of the work are appropriately investigated and resolved. The study was conducted in accordance with the Declaration of Helsinki (as revised in 2013).
Open Access Statement: This is an Open Access article distributed in accordance with the Creative Commons Attribution-NonCommercial-NoDerivs 4.0 International License (CC BY-NC-ND 4.0), which permits the non-commercial replication and distribution of the article with the strict proviso that no changes or edits are made and the original work is properly cited (including links to both the formal publication through the relevant DOI and the license). See: https://creativecommons.org/licenses/by-nc-nd/4.0/.
References
- van der Bom T, Bouma BJ, Meijboom FJ, et al. The prevalence of adult congenital heart disease, results from a systematic review and evidence based calculation. Am Heart J 2012;164:568-75. [Crossref] [PubMed]
- van der Linde D, Konings EE, Slager MA, et al. Birth prevalence of congenital heart disease worldwide: a systematic review and meta-analysis. J Am Coll Cardiol 2011;58:2241-7. [Crossref] [PubMed]
- Forman J, Beech R, Slugantz L, et al. A Review of Tetralogy of Fallot and Postoperative Management. Crit Care Nurs Clin North Am 2019;31:315-28. [Crossref] [PubMed]
- Bittel DC, Zhou XG, Kibiryeva N, et al. Ultra high-resolution gene centric genomic structural analysis of a non-syndromic congenital heart defect, Tetralogy of Fallot. PLoS One 2014;9:e87472. [Crossref] [PubMed]
- Kan Z, Yan W, Wang N, et al. Identification of circRNA-miRNA-mRNA Regulatory Network and Crucial Signaling Pathway Axis Involved in Tetralogy of Fallot. Front Genet 2022;13:917454. [Crossref] [PubMed]
- Weisenberger DJ, Campan M, Long TI, et al. Analysis of repetitive element DNA methylation by MethyLight. Nucleic Acids Res 2005;33:6823-36. [Crossref] [PubMed]
- Sheng W, Qian Y, Wang H, et al. DNA methylation status of NKX2-5, GATA4 and HAND1 in patients with tetralogy of fallot. BMC Med Genomics 2013;6:46. [Crossref] [PubMed]
- McElhinney DB, Driscoll DA, Levin ER, et al. Chromosome 22q11 deletion in patients with ventricular septal defect: frequency and associated cardiovascular anomalies. Pediatrics 2003;112:e472. [Crossref] [PubMed]
- Soemedi R, Wilson IJ, Bentham J, et al. Contribution of global rare copy-number variants to the risk of sporadic congenital heart disease. Am J Hum Genet 2012;91:489-501. [Crossref] [PubMed]
- Li X, Li X, Li H, et al. A case-control study on the correlation between parental environmental risk factor exposure and congenital heart disease of their children. Guowai Yixue 2017;38:348-51. (Yixue Dili Fence).
- Gorini F, Chiappa E, Gargani L, et al. Potential effects of environmental chemical contamination in congenital heart disease. Pediatr Cardiol 2014;35:559-68. [Crossref] [PubMed]
- Wienecke LM, Cohen S, Bauersachs J, et al. Immunity and inflammation: the neglected key players in congenital heart disease? Heart Fail Rev 2022;27:1957-71. [Crossref] [PubMed]
- Hoyer FF, Naxerova K, Schloss MJ, et al. Tissue-Specific Macrophage Responses to Remote Injury Impact the Outcome of Subsequent Local Immune Challenge. Immunity 2019;51:899-914.e7. [Crossref] [PubMed]
- Gu Q, Kong Y, Yu ZB, et al. Hypoxia-induced SOCS3 is limiting STAT3 phosphorylation and NF-κB activation in congenital heart disease. Biochimie 2011;93:909-20. [Crossref] [PubMed]
- Qing M, Schumacher K, Heise R, et al. Intramyocardial synthesis of pro- and anti-inflammatory cytokines in infants with congenital cardiac defects. J Am Coll Cardiol 2003;41:2266-74. [Crossref] [PubMed]
- Grunert M, Dorn C, Schueler M, et al. Rare and private variations in neural crest, apoptosis and sarcomere genes define the polygenic background of isolated Tetralogy of Fallot. Hum Mol Genet 2014;23:3115-28. [Crossref] [PubMed]
- O'Brien JE Jr, Kibiryeva N, Zhou XG, et al. Noncoding RNA expression in myocardium from infants with tetralogy of Fallot. Circ Cardiovasc Genet 2012;5:279-86. [Crossref] [PubMed]
- Love MI, Huber W, Anders S. Moderated estimation of fold change and dispersion for RNA-seq data with DESeq2. Genome Biol 2014;15:550. [Crossref] [PubMed]
- Wu T, Hu E, Xu S, et al. clusterProfiler 4.0: A universal enrichment tool for interpreting omics data. Innovation (Camb) 2021;2:100141. [Crossref] [PubMed]
- Newman AM, Liu CL, Green MR, et al. Robust enumeration of cell subsets from tissue expression profiles. Nat Methods 2015;12:453-7. [Crossref] [PubMed]
- Shannon P, Markiel A, Ozier O, et al. Cytoscape: a software environment for integrated models of biomolecular interaction networks. Genome Res 2003;13:2498-504. [Crossref] [PubMed]
- Gong J, Liu C, Liu W, et al. An update of miRNASNP database for better SNP selection by GWAS data, miRNA expression and online tools. Database (Oxford) 2015;2015:bav029. [Crossref] [PubMed]
- Cedars A, Benjamin L, Vyhmeister R, et al. Contemporary Hospitalization Rate Among Adults With Complex Congenital Heart Disease. World J Pediatr Congenit Heart Surg 2016;7:334-43. [Crossref] [PubMed]
- Mittal P, Romero R, Kusanovic JP, et al. CXCL6 (granulocyte chemotactic protein-2): a novel chemokine involved in the innate immune response of the amniotic cavity. Am J Reprod Immunol 2008;60:246-57. [Crossref] [PubMed]
- Waehre A, Vistnes M, Sjaastad I, et al. Chemokines regulate small leucine-rich proteoglycans in the extracellular matrix of the pressure-overloaded right ventricle. J Appl Physiol 1985;2012:1372-82. [Crossref] [PubMed]
- Wang X, Dai Y, Zhang X, et al. CXCL6 regulates cell permeability, proliferation, and apoptosis after ischemia-reperfusion injury by modulating Sirt3 expression via AKT/FOXO3a activation. Cancer Biol Ther 2021;22:30-9. [Crossref] [PubMed]
- Girard TJ, Antunes L, Zhang N, et al. Peripheral blood mononuclear cell tissue factor (F3 gene) transcript levels and circulating extracellular vesicles are elevated in severe coronavirus 2019 (COVID-19) disease. J Thromb Haemost 2023;21:629-38. [Crossref] [PubMed]
- Hirano S, Kanno S. Macrophage Receptor with Collagenous Structure (MARCO) Is Processed by either Macropinocytosis or Endocytosis-Autophagy Pathway. PLoS One 2015;10:e0142062. [Crossref] [PubMed]
- Dong Q, Zhang S, Zhang H, et al. MARCO is a potential prognostic and immunotherapy biomarker. Int Immunopharmacol 2023;116:109783. [Crossref] [PubMed]
- Sheng L, Luo Q, Chen L. Amino Acid Solute Carrier Transporters in Inflammation and Autoimmunity. Drug Metab Dispos 2022;DMD-AR-2021-000705.
- Sauce D, Larsen M, Fastenackels S, et al. Evidence of premature immune aging in patients thymectomized during early childhood. J Clin Invest 2009;119:3070-8. [Crossref] [PubMed]
- Güvener M, Korun O, Demirtürk OS. Risk factors for systemic inflammatory response after congenital cardiac surgery. J Card Surg 2015;30:92-6. [Crossref] [PubMed]
- Bhatia M, Moochhala S. Role of inflammatory mediators in the pathophysiology of acute respiratory distress syndrome. J Pathol 2004;202:145-56. [Crossref] [PubMed]
- Eltzschig HK, Carmeliet P. Hypoxia and inflammation. N Engl J Med 2011;364:656-65. [Crossref] [PubMed]
- Vinten-Johansen J. Involvement of neutrophils in the pathogenesis of lethal myocardial reperfusion injury. Cardiovasc Res 2004;61:481-97. [Crossref] [PubMed]
- Manuel V, Miana LA, Solla DJF, et al. Preoperative level of neutrophil-lymphocyte ratio: Comparison between cyanotic and acyanotic congenital heart disease. J Card Surg 2021;36:1376-80. [Crossref] [PubMed]
- Manuel V, Miana LA, Guerreiro GP, et al. Preoperative Neutrophil-Lymphocyte Ratio Can Predict Outcomes for Patients Undergoing Tetralogy of Fallot Repair. Braz J Cardiovasc Surg 2021;36:607-13. [Crossref] [PubMed]
- Wienecke LM, Fraccarollo D, Galuppo P, et al. Pro-inflammatory intermediate monocytes relate to right ventricular pressure in heart failure of adult congenital heart disease. European Heart Journal 2019;40:3905.
- Hamada H, Terai M, Kimura H, et al. Increased expression of mast cell chymase in the lungs of patients with congenital heart disease associated with early pulmonary vascular disease. Am J Respir Crit Care Med 1999;160:1303-8. [Crossref] [PubMed]
- Zhang X, Gao Y, Zhang X, et al. FGD5-AS1 Is a Hub lncRNA ceRNA in Hearts With Tetralogy of Fallot Which Regulates Congenital Heart Disease Genes Transcriptionally and Epigenetically. Front Cell Dev Biol 2021;9:630634. [Crossref] [PubMed]
- Liang D, Xu X, Deng F, et al. miRNA-940 reduction contributes to human Tetralogy of Fallot development. J Cell Mol Med 2014;18:1830-9. [Crossref] [PubMed]
- Yu Y, Ge X, Wang LS, et al. Abnormalities of hsa-mir-16 and hsa-mir-124 Affect Mitochondrial Function and Fatty Acid Metabolism in Tetralogy of Fallot. Comb Chem High Throughput Screen 2023;26:373-82. [Crossref] [PubMed]
- Zhang L, Li G, Liang B, et al. Integrative analyses of immune-related biomarkers and associated mechanisms in coronary heart disease. BMC Med Genomics 2022;15:219. [Crossref] [PubMed]