Identification of novel mitophagy-related biomarkers for Kawasaki disease by integrated bioinformatics and machine-learning algorithms
Highlight box
Key findings
• The mitophagy genes that may be related to the occurrence of Kawasaki disease (KD) were identified.
What is known and what is new?
• In recent years, mitophagy, which is closely related to the occurrence and development of a variety of inflammatory diseases, has become a research hotspot.
• The identified key mitophagy-related genes were associated with the development and progression of KD.
What is the implication, and what should change now?
• Further research needs to be conducted to determine whether the key genes screened and identified in this study are related to the regulation of mitophagy and whether these key genes can influence the development and progression of KD through mitophagy.
Introduction
Kawasaki disease (KD) initially identified as “mucocutaneous lymph node syndrome” by Tomisaku Kawasaki in 1967, stands as the predominant cause of acquired heart disease in children under 5 years old (1). Approximately 25% of affected individuals develop coronary artery lesions (CALs), leading to serious cardiovascular diseases (CVDs), including myocardial infarction, angina pectoris, and sudden cardiac death (2). The diagnosis of KD relies on clinical features such as prolonged fever (usually ≥39 °C), strawberry tongue, cleft lips, bilateral conjunctivitis, cervical lymphadenopathy, limb edema, generalized rash, and CALs detected by echocardiography. However, these diagnostic methods, which are based on inflammatory biomarkers like the erythrocyte sedimentation rate, leukocyte/leukocyte count, platelet count, C-reactive protein, interleukin-6, and serum albumin levels, are non-specific (3,4), which often leads to delays in treatments and exacerbates the risks of complications. The treatment of KD relies primarily on high-dose intravenous immunoglobulin (IVIG) within the first 10 days of fever onset. Nonetheless, the mechanism underlying the effect of IVIG is still elusive. Additionally, around 10–20% of individuals with KD develop resistance to IVIG, resulting in an elevated risk of CALs (5). Hence, it is imperative to explore novel diagnostic biomarkers and treatment approaches to improve the outcomes of individuals with KD.
Mitophagy, the selective autophagy of mitochondria, has garnered attention for its role in CVDs. It ensures mitochondrial quality by removing damaged mitochondria, crucial for cellular homeostasis. Research using mouse models injected with lactobacillus casei cell wall extract (LCWE) to simulate KD vasculitis has highlighted a compromised mitochondrial clearance process (6). These models exhibited a reduction in autophagic activity and an obstruction of autophagy due to mechanistic target of rapamycin (mTOR) pathway activation, leading to the activation of the NACHT, LRR, and PYD domains-containing protein 30 (NLRP3) inflammasome and an increase in reactive oxygen species. Additionally, studies have noted an upsurge in the expression of genes related to the NLRP3 inflammasome pathway in both KD patients’ blood and in cardiovascular tissues from LCWE-injected mice (7,8). The expression of autophagy-related genes, such as LC3B, BECN1, and ATG16L1, is lower in KD patients than febrile and healthy individuals. Nonetheless, IVIG treatment elevates their expression levels. Notably, low ATG16L1 messenger RNA (mRNA) levels persist in patients with CALs, indicating a significant disruption in mitophagy, which may be a potential therapeutic target (9). The regulation of mitophagy, both temporal and spatial, influences various disease stages and involves multiple signaling pathways, including the PINK1/Parkin and BNIP3/NIX pathways. These pathways regulate mitophagy stages through modifications such as phosphorylation and ubiquitination (10,11). For instance, a decrease in MCM8 gene expression or blockage of the NO pathway inhibits mitophagy, exacerbating coronary arteritis in KD. Conversely, activating the NO pathway or enhancing MCM8-mediated mitophagy could offer new strategies to alleviate KD symptoms by reducing inflammation and promoting the clearance of damaged mitochondria (12). As a result, further research is warranted to further examine the role of mitophagy in the occurrence of CALs in KD
The advent of microarray technology and integrated bioinformatics has paved the way for the identification of disease-related novel genes (13-15). By employing bioinformatics methods, like the weighted gene co-expression network analysis (WGCNA), and machine-learning algorithms, such as random forest-recursive feature elimination (RF-RFE) and support vector machine-recursive feature elimination (SVM-RFE), this study sought to elucidate the role of mitophagy-related genes (MRGs) in KD. Our approach sought to identify the hub genes associated with KD progression and their correlation with immune infiltration, offering new diagnostic insights and an understanding of the pathophysiology of KD. We present this article in accordance with the TRIPOD reporting checklist (available at https://tp.amegroups.com/article/view/10.21037/tp-24-230/rc).
Methods
Data downloading and pre-processing
Microarray expression data and clinical information for KD were sourced from the Gene Expression Omnibus (GEO) database across the following four datasets: GSE68004, GSE73461, GSE100154, and GSE18606. These datasets included a total of 289 samples, of which 174 were from KD patients and 115 were from healthy controls. The GSE68004 and GSE73461 datasets generated on the GPL10558 platform, along with GSE100154 on GPL6884 and GSE18606 on GPL6480, provided a diverse dataset for our analysis. KD was diagnosed following the American Heart Association guidelines (16). The SVA R package merged the first three datasets to create a comprehensive set, while GSE18606 served as a separate verification dataset (17).
Identification of the DE-MRGs
Using the limma R package, the differentially expressed genes (DEGs) were identified based on a threshold of a |log fold change (FC)| >1 and an adjusted P value <0.05 (18). The MRGs were curated from the Genecards database, and the intersections between the DEGs and MRGs pinpointed the differentially expressed mitophagy-related genes (DE-MRGs).
Functional enrichment analysis
Gene Ontology (GO) is a publicly accessible resource used for large-scale functional enrichment research. Kyoto Encyclopedia of Genes and Genomes (KEGG) is an extensively used database that offers genomic, biomolecular, and metabolic information (19). The GO and KEGG enrichment analyses were executed using The Database for Annotation, Visualization and Integrated Discovery (DAVID, https://david.ncifcrf.gov/), and the ggplot2 R package was used to visualize the results. An adjusted P value <0.05 was indicative of a statistically significant difference.
Construction of the gene co-expression network
Weighted co-expression networks were built using the WGCNA R package to analyze the DE-MRGs (20). Initially, the optimal soft threshold (β) was determined using the “pickSoftThreshold” function, after which a Pearson correlation coefficient analysis was performed to calculate the gene correlations. These correlations were then used to create weighted adjacency matrices, which were further transformed into topological overlap matrices. Scale-free networks were constructed using the block module function, and gene co-expression modules were identified through a module partitioning analysis. These modules were visualized in different colors and summarized by their module eigengenes (ME). The analysis also involved linking modules with phenotypic data to assess gene significance (GS) and module significance (MS), thereby evaluating the relationship between gene expression levels and clinical information. Module membership (MM) for each gene was calculated to further explore GS in the modules. Modular signature genes, representing each module’s collective gene expression profile, were identified as crucial elements. The module showing the highest correlation with KD was selected as the hub module for detailed examination. The candidate hub genes were those with high connectivity in the modules. Genes with high absolute GS values were focused on in the subsequent studies, the following criteria for gene selection was applied: absolute GS >0.20; absolute MM >0.80.
Hub gene identification via machine learning
The SVM-RFE operates on a recursive feature elimination method that integrates feature selection within the SVM model training. It progressively removes less important features by identifying the most representative subset. Similarly, the RF-RFE method combines the RF algorithm with RFE. This approach uses RF to assess feature importance and then recursively eliminates the less significant features to enhance feature selection accuracy (21). The hub genes were identified using the RF-RFE and SVM-RFE machine-learning algorithms, implemented through the randomForest (22), caret, e1071, and caret R packages (23). The intersection of genes identified by both algorithms pinpointed the key mitophagy-related hub genes for KD.
Nomogram construction and analysis of the hub gene expression levels
Nomograms enabling the prediction of disease outcomes based on hub gene expression are instrumental in clinical KD diagnosis (24). Using the rms R package, a nomogram was developed of the identified hub genes, assigning scores to each and calculating a total score for diagnosis prediction (25). The ggpubr and reshape2 R packages facilitated the comparison of the hub gene expression levels between the KD patients and healthy controls, while the ComplexHeatmap R package aided in the clustering analysis (26). Visualization was achieved using the ggplot2 R package (27).
Diagnostic validation of the hub genes
A receiver operating characteristic (ROC) curve analysis of the merged dataset (comprising GSE68004, GSE73461, and GSE100154) was conducted using the pROC R package to assess the diagnostic accuracy of the identified hub genes in differentiating between patients with KD and healthy controls (28). GSE18606 served as the validation set. Areas under the curve (AUCs) and 95% confidence intervals (CIs) were used to quantify the diagnostic efficacy of these genes. An AUC >0.7 indicated robust diagnostic value.
Immune cell infiltration assessment and correlation with hub genes
CIBERSORT, a tool based on linear support vector regression, performs deconvolution analysis to estimate the composition and abundance of various immune cells in mixed cell populations (29). The Cibersort algorithm was used to quantify the infiltration of 22 immune cell types, and box plots were used to visualize the differential expression of these cells (29). Correlations between these cells and the hub genes were analyzed using the psych R package and depicted using the ggplot2 R package.
TFs and gene-miRNAs interactions with hub genes
Our research utilized the ENCODE database to construct TF-gene interaction networks and the MiRTarBase to build gene-miRNA interaction networks, predicting regulatory associations between hub genes and these elements. These networks were developed and visualized using the NetworkAnalyst platform.
Quantitative reverse-transcription polymerase chain reaction (qRT-PCR) validation
Blood samples from 10 KD patients and 10 healthy children of similar age were collected at the Children’s Hospital of Soochow University. Leukocytes were separated using Red Blood Cell Lysis Buffer (Solarbio, Beijing, China), and total RNA was extracted using Trizol (Thermo, California, USA). After ensuring the quality of the RNA, it was converted to complementary DNA (cDNA) using PrimeScriptTM RT Master Mix (TaKaRa, Tokyo, Japan). qRT-PCR was conducted with SYBR Green Master Mix (Thermo, California, USA) on the cDNA in accordance with the provided protocols. The primer sequences for the target genes are listed in Table 1, with gene expression normalized to β-actin levels. Relative mRNA expression was calculated using the 2−ΔΔCT method, with each experiment replicated three times for accuracy. The data analysis was conducted using GraphPad Prism 8.0.2 software, and group differences were compared using the Student’s t-test. A two-tailed P value <0.05 was considered statistically significant. The study was conducted in accordance with the Declaration of Helsinki (as revised in 2013). The study was approved by Ethics Committee of Soochow University (ECSU) (No. SUDA20220906A01) and informed consent was taken from all the patients’ guardians.
Table 1
Genes | Forward | Reverse |
---|---|---|
CKAP4 | GCAGCCACCAGGACTTCTC | TCACTCTCCCCTTGCTTCAC |
SRPK1 | GCAACAGAATGGCAGCGATC | CTGGCGCTTCTGCTTCTTC |
β-actin | TGTCCACCTTCCAGCAG | CGCAGCTCAGTAACAGTCC |
qRT-PCR, quantitative reverse transcriptase polymerase chain reaction; CKAP4, cytoskeleton-associated protein 4; SRPK1, serine-arginine protein kinase 1.
Statistical analysis
All the statistical analyses were conducted using R software (version 4.2.0). The Pearson test was used to evaluate the correlation between the MEs and KD, while the Wilcoxon test was used to compare the immune cell proportions between the groups. The Spearman test was used to assess the correlation between the hub genes and 22 immune cells. A P value <0.05 was considered statistically significant.
Results
Identification of the DE-MRGs
The study design is depicted in Figure 1. The study included 174 KD patients and 115 healthy controls. A total of 2,786 DEGs were identified between the KD patients and healthy controls with their distribution illustrated in volcano plots (Figure 2A). The DE-MRGs were determined by intersecting the DEGs with the mitophagy-associated genes from the Genecards database (Figure 2B).
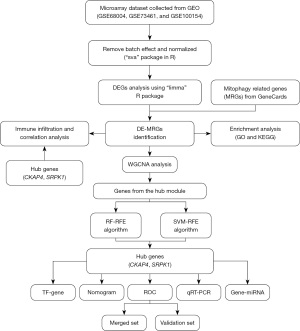
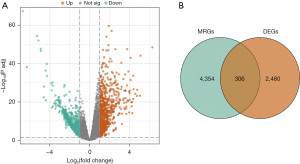
Functional enrichment analysis of the DE-MRGs
The study examined the biological functions and pathways of the DE-MRGs to reveal their involvement in various cellular components (CCs), biological processes (BPs), and molecular functions (MFs). The top 10 enriched GO terms for each category are illustrated in Figure 3A-3C. In terms of the CCs, the DE-MRGs were mainly found in the extracellular matrix, cytosol, focal adhesion points, endoplasmic reticulum, and cytoplasm. In terms of the BPs, the genes were significantly involved in enhancing the nuclear factor κB (NF-κB) transcription factor (TF) activity, protein phosphorylation, protein transport to mitochondria, apoptosis, and mitochondrial organization. In terms of the MFs, the DE-MRGs were mainly enriched in ATP binding, heparan sulfate binding, cadherin binding, and protein serine/threonine/tyrosine kinase activity. The KEGG pathway analysis identified tight junctions, autophagy, ubiquitin-mediated proteolysis, axon guidance, and the insulin signaling pathway as the most enriched pathways for the DE-MRGs (Figure 3D).
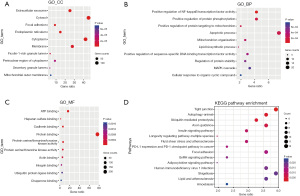
Co-expression network construction and hub module identification
This study conducted a WGCNA to identify the genes associated with KD from the DE-MRGs. The chosen soft threshold power was 16, achieving a scale independence value of 0.8 and enhancing the mean connectivity of the adjacency matrix (Figure 4A). This analysis led to the identification of two distinct co-expression modules. These were determined through dynamic tree cutting, which merged modules with a difference <10% (Figure 4B) and fewer than 100 minimal modules into larger modules. A further correlation analysis between these modules and the KD clinical traits was conducted. The MEturquoise module, comprising 47 genes, exhibited the most substantial positive correlation with KD as indicated by a correlation coefficient (r) value of 0.6729 and a highly significant P value of 1.86e−39 (Figure 4C). In this module, a significant correlation was also found between GS and MM, with a correlation (cor) value of 0.77 and a P value of 7.3e−19 (Figure 4D). Given these findings, the MEturquoise module was selected as the central module for further investigation due to its strong association with KD.
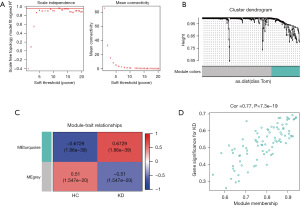
Identification of the hub genes by a machine-learning algorithm
In the MEturquoise module, 47 genes with the highest connectivity were selected as potential hub genes and analyzed using the RF-RFE and SVM-RFE algorithms. The RF-RFE method pinpointed 9 DE-MRGs for closer examination, ranking them by importance (Figure 5A,5B). Similarly, the SVM-RFE technique identified 16 DE-MRGs (Figure 5C). The cross-referencing of the results from both the RF-RFE and SVM-RFE revealed two common genes as central hub genes; that is, cytoskeleton-associated protein 4 (CKAP4) and serine-arginine protein kinase 1 (SRPK1) (Figure 5D).
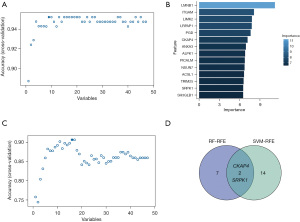
Nomogram model construction and expression of the hub genes
A nomogram evaluation model was developed using the two central hub genes to predict the risk associated with the occurrence of KD (Figure 6A). The expression levels of these hub genes, observed in a combined dataset, are displayed in Figure 6B, which showed that these genes were more highly expressed in the KD patients than the healthy controls. Further, a heatmap (Figure 6C) effectively differentiated the KD group from the healthy group based on the expression of these two hub genes.
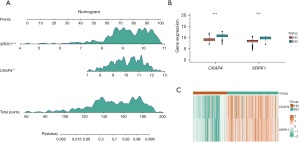
Assessment of the diagnostic value of the hub genes
The diagnostic potential of the two hub genes was evaluated using a ROC curve analysis of both the internal and external datasets. In the merged dataset, the AUC value of CKAP4 was 0.933 (95% CI: 0.901 to 0.964), and that of SRPK1 was 0.936 (95% CI: 0.906 to 0.966) (Figure 7A,7B). In the GSE18606 validation set, the AUC value of CKAP4 was 0.872 (95% CI: 0.741 to 1.000) and that of SRPK1 was 0.878 (95% CI: 0.750 to 1.000) (Figure 7C,7D).
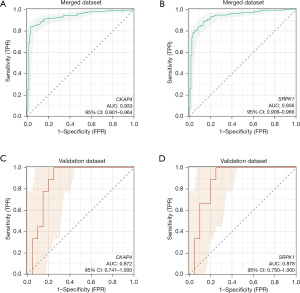
Immune cell infiltration analysis and correlation with the hub genes
To further examine the effects of the DE-MRGs on immune cells in KD, an immune cell infiltration analysis was performed. As Figure 8A shows, the results revealed a significant increase in memory B cells, activated memory cluster of differentiation (CD)4+ T cells, M2 macrophages, and activated mast cells in the KD group compared to the healthy control group. Conversely, CD8+ T cells, gamma delta T cells, and resting natural killer (NK) cells were significantly more abundant in the healthy control group than the KD group. These results revealed distinct immune microenvironments between the KD patients and healthy controls.
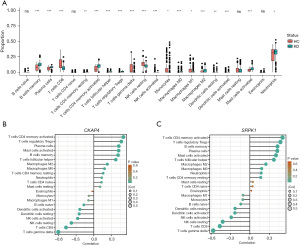
Additionally, an analysis was conducted to explore the correlations between the 22 immune cells and two pivotal genes. CKAP4 was found to be positively correlated with activated memory CD4+ T cells (cor value: 0.399, P value: 1.85E−12), regulatory T cells (Tregs) (cor value: 0.348, P value: 1.20E−09), and plasma cells (cor value: 0.317, P value: 3.46E−08), but negatively correlated with CD8+ T cells (cor value: –0.446, P value: 1.63E−15), gamma delta T cells (cor value: –0.413, P value: 2.64E−13), and resting NK cells (cor value: –0.288, P value: 1.63E−15) (Figure 8B). While SRPK1 was found to be positively correlated with activated memory CD4+ T cells (cor value: 0.481, P value: 3.69E−18), plasma cells (cor value: 0.435, P value: 9.30E−15), and memory B cells (cor value: 0.407, P value: 5.67E−13), but negatively correlated with CD8+ T cells (cor value: –0.479, P value: 5.20E−18), gamma delta T cells (cor value: –0.452, P value: 5.90E−16), and resting NK cells (cor value: –0.376, P value: 3.74E−11) (Figure 8C).
TFs-gene and gene-miRNAs interactions of hub genes
To investigate the potential modulatory relationships of hub genes, the ENCODE database was utilized to identify TFs targeting these genes. The interactions were visualized using NetworkAnalyst, which displayed 38 nodes and 40 edges (Figure 9A). TFs such as myc-associated zinc finger protein (MAZ), Sin3A-associated protein 30 (SAP30), homeodomain finger protein 8 (PHF8), and lysine demethylase 5B (KDM5B) are identified as potential novel diagnostic and therapeutic targets for KD.
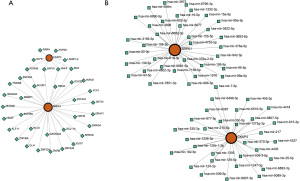
The gene-miRNA interactions were also explored using the miRTarBase v9.0 database, with the resulting network visualized by NetworkAnalyst, featuring 66 nodes and 67 edges (Figure 9B). Notably, hsa-mir-7-5p was linked to multiple hub genes.
qRT-PCR validation of the hub genes
At last, qRT–PCR was performed on human blood samples to analyze the expression of CKAP4 and SRPK1. Compared to that in the healthy controls, the expression of CKAP4 (P<0.001) and SRPK1 (P=0.002) was significantly higher in the KD samples (Figure 10A,10B). These results were consistent with our previous analysis through public databases.
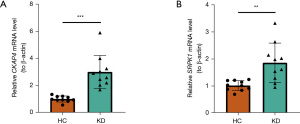
Discussion
KD, as the main cause of acquired heart disease in children, is believed to be triggered by various factors, including genetics and the environment. A CAL is a serious complication of KD, and the early diagnosis and intervention of KD can reduce the incidence of this complication. Due to the non-specificity of current diagnostic methods, the early identification of KD is challenging. Accordingly, the identification of precise biomarkers is crucial.
Mitophagy has been linked to several CVDs, including atherosclerosis (30), hypertension (31), cardiac hypertrophy, and heart failure (32), and thus it may serve as a potential therapeutic target. Its involvement in KD, particularly in regulating mitochondrial function and reducing oxidative stress, is increasingly recognized. For instance, thymic stromal lymphopoietin (TSLP)-induced mitophagy in platelets, via a novel TSLP receptor/Parkin/voltage-dependent anion channel 1 (VDAC1) pathway, suggests a therapeutic target against KD-associated thrombosis (33). Additionally, the dysregulation of mitochondrial division and mitophagy triggered by dynamin related protein-1 (DRP-1) overexpression was shown to result in damage to artery endothelial cells in a Candida albicans water soluble fraction-induced KD mouse model (34). During the acute phase of KD, infiltrating neutrophils and macrophages in the coronary arteries, significant producers of reactive oxidative species (ROS) (35,36), stimulate the NF-κB pathway and cytokine expression, enhancing the inflammatory response (37). Inhibition of ROS production has been shown to alleviate CALs in KD (38). In the LCWE murine model of KD vasculitis, impaired mitophagy and increased ROS levels were noted. Modulating mitophagy may reduce CALs in KD by curbing ROS production (6). Further studies on mitophagy in KD need to be conducted.
This study was the first to employ a bioinformatics analysis to uncover 306 DE-MRGs between KD patients and healthy controls through GEO and Genecards database exploration. The GO analysis revealed several mitophagy-related enrichment terms, such as the positive regulation of proteins targeting mitochondrion and mitochondrion organization, which suggests that these DE-MRGs play crucial roles in KD development. The KEGG analysis also highlighted autophagy and pathways like extracellular exosome, NF-kB TF activity, and ubiquitin-mediated proteolysis (39-41), indicating the multifaceted effects of these DE-MRGs on KD.
To identify potential diagnostic biomarkers for KD, we applied a WGCNA and two machine-learning algorithms (i.e., RF-RFE and SVM-RFE) to analyze the 306 DE-MRGs. This process identified CKAP4 and SRPK1 as the key hub genes, which were further validated using an additional dataset. A ROC curve analysis confirmed their excellent diagnostic efficacy, and the qRT-PCR results supported the distinct expression of these genes in KD patients compared to healthy controls.
CKAP4 is found in various tissues and cells and functions as a specific membrane receptor. Its aberrant expression has been linked to poor prognosis in several cancers (42-44). Notably, its involvement in the pathogenesis of coronavirus disease 2019 (COVID-19) (45), which can trigger autoimmune responses leading to KD (46), highlights its significance. Yazici et al. identified plasma CKAP4 as a biomarker for disease progression and mortality in COVID-19 (47), suggesting its potential role in KD pathophysiology through mechanisms such as endothelial cell adherence junction integrity (48).
SRPK1 regulates the phosphorylation of splicing factors enriched in serine/arginine domains, and plays a pivotal role in apoptosis, the cell cycle, the innate immune response, and inflammation regulation. This is achieved through its interactions with various TFs and signaling pathways (49-51). The dysregulation of SRPK1 is associated with cancers, heart diseases, and immune disorders (52,53). Yao et al. found that SRPK1 enhances the lipopolysaccharide-induced inflammatory response and contributes to acute lung injury development by interacting with AKT serine/threonine kinase 3 (AKT3) (54). Tumour necrosis factor-alpha (TNF-α) is a key inflammatory factor in KD, and TNF-α inhibitors, such as infliximab, can improve outcomes in IVIG-resistant KD patients (55). TNF-α elicits an inflammatory response mainly by activating the NF-κB pathway; thus, inhibiting this pathway may also offer new treatment strategies for KD (56). Research has shown that SRPK1 increases anti-apoptotic ability and activates the NF-κB pathway, thus contributing to the progression of colon cancer (57). Thus, it may be a potential therapeutic target for KD.
In the immune correlation analysis, significant associations were observed between both genes and various T cell types, including activated memory CD4+ T cells, Tregs, gamma delta T cells, and CD8+ T cells. Single-cell RNA sequencing data revealed that KD patients showed a reduced percentage of CD4+ T cells before therapy compared to after therapy, and a lower percentage of CD8+ T cells than healthy controls (58). Treg clones specific to Fc regions have been generated from KD patients without arterial complications post-IVIG therapy; however, KD patients with CALs seem incapable of expanding these Fc-specific Treg populations even after IVIG treatment (59). Similar Treg specificities were found between IVIG-treated KD patients and healthy controls, which suggests that the absence of Fc-specific Treg cells in acute KD may stem from inflammation (60). Another study on plasma exchange (PE) treatment in KD showed an increase in Tregs in CD4+ T cells post-PE treatment, suggesting that PE might alleviate KD inflammation by normalizing Treg levels (61). These findings underscore the crucial role of Tregs in KD and highlight the need for further research on how mitophagy influences KD through immune cell modulation.
This study constructed TF-gene and gene-microRNA interaction networks to identify key regulatory actors at the transcriptional and post-transcriptional levels. TFs such as MAZ, SAP30, PHF8, and KDM5B play critical roles in modulating the expression of hub genes. Previous research indicates MAZ regulates interferon-gamma (IFN-γ)-stimulated genes via signal transducer and activator of transcription 1 (62). In cerebral ischemic stroke, reduced SAP30 expression decreases apoptosis in HMC3 cells and ROS and MDA production (63). A study has shown that PHF8 is essential for vascular endothelial cell function, and its knockdown reduces cell proliferation and survival (64). Recently, KDM5B is necessary for activating the NF-κB signaling cascade and cytokine production in macrophages; inhibiting KDM5B protected mice from immune injury in models of collagen-induced arthritis and endotoxin shock (65). Notably, hsa-mir-7-5p was found to regulate hub genes by binding to the three major untranslated regions (3'-UTR) of ataxin 1, reducing its expression, and indirectly enhancing NF-κB activity, thus inducing proinflammatory cytokines (66).
This study’s advantage lies in its sophisticated approach that combined bioinformatics and various machine-learning algorithms to pinpoint crucial genes linked to KD and mitophagy. However, it should be noted that it had a few limitations. First, the reliance on public databases might have introduced a bias due to the limited data in the validation set. However, the qRT-PCR validation of the two genes across the different groups affirmed their relevance and potential applicability. Moreover, the analysis, while insightful, was based on theoretical models, underscoring the need for further empirical research through cellular and animal studies to confirm the precise roles of these molecules in KD. Additionally, the mechanisms by which the identified genes influence immune infiltration and mitophagy require detailed exploration.
Conclusions
To sum up, using advanced bioinformatics and machine-learning techniques, this study identified two MRGs (i.e., CKAP4 and SRPK1) that were significant in KD. Using these genes, personalized nomogram models were developed and validated, offering a novel diagnostic tool for KD. Further, immune infiltration and correlation analyses were conducted to shed light on potential pathways for the prevention and treatment of KD. These findings lay the foundation for future research aimed at understanding KD’s molecular underpinnings and improving therapeutic strategies.
Acknowledgments
Funding: This study was supported by
Footnote
Reporting Checklist: The authors have completed the TRIPOD reporting checklist. Available at https://tp.amegroups.com/article/view/10.21037/tp-24-230/rc
Data Sharing Statement: Available at https://tp.amegroups.com/article/view/10.21037/tp-24-230/dss
Peer Review File: Available at https://tp.amegroups.com/article/view/10.21037/tp-24-230/prf
Conflicts of Interest: All authors have completed the ICMJE uniform disclosure form (available at https://tp.amegroups.com/article/view/10.21037/tp-24-230/coif). The authors have no conflicts of interest to declare.
Ethical Statement: The authors are accountable for all aspects of the work in ensuring that questions related to the accuracy or integrity of any part of the work are appropriately investigated and resolved. The study was conducted in accordance with the Declaration of Helsinki (as revised in 2013). The study was approved by Ethics Committee of Soochow University (ECSU) (No. SUDA20220906A01) and informed consent was taken from all the patients’ guardians.
Open Access Statement: This is an Open Access article distributed in accordance with the Creative Commons Attribution-NonCommercial-NoDerivs 4.0 International License (CC BY-NC-ND 4.0), which permits the non-commercial replication and distribution of the article with the strict proviso that no changes or edits are made and the original work is properly cited (including links to both the formal publication through the relevant DOI and the license). See: https://creativecommons.org/licenses/by-nc-nd/4.0/.
References
- Marek-Iannucci S, Yildirim AD, Hamid SM, et al. Targeting IRE1 endoribonuclease activity alleviates cardiovascular lesions in a murine model of Kawasaki disease vasculitis. JCI Insight 2022;7:e157203. [Crossref] [PubMed]
- Hedrich CM, Schnabel A, Hospach T. Kawasaki Disease. Front Pediatr 2018;6:198. [Crossref] [PubMed]
- McCrindle BW, Rowley AH, Newburger JW, et al. Diagnosis, Treatment, and Long-Term Management of Kawasaki Disease: A Scientific Statement for Health Professionals From the American Heart Association. Circulation 2017;135:e927-99. [Crossref] [PubMed]
- Li Y, Zheng Q, Zou L, et al. Kawasaki disease shock syndrome: clinical characteristics and possible use of IL-6, IL-10 and IFN-γ as biomarkers for early recognition. Pediatr Rheumatol Online J 2019;17:1. [Crossref] [PubMed]
- Wallace CA, French JW, Kahn SJ, et al. Initial intravenous gammaglobulin treatment failure in Kawasaki disease. Pediatrics 2000;105:E78. [Crossref] [PubMed]
- Marek-Iannucci S, Ozdemir AB, Moreira D, et al. Autophagy-mitophagy induction attenuates cardiovascular inflammation in a murine model of Kawasaki disease vasculitis. JCI Insight 2021;6:e151981. [Crossref] [PubMed]
- Hoang LT, Shimizu C, Ling L, et al. Global gene expression profiling identifies new therapeutic targets in acute Kawasaki disease. Genome Med 2014;6:541. [Crossref] [PubMed]
- Porritt RA, Zemmour D, Abe M, et al. NLRP3 Inflammasome Mediates Immune-Stromal Interactions in Vasculitis. Circ Res 2021;129:e183-200. [Crossref] [PubMed]
- Huang FC, Huang YH, Kuo HC, et al. Identifying Downregulation of Autophagy Markers in Kawasaki Disease. Children (Basel) 2020;7:166. [Crossref] [PubMed]
- Palikaras K, Lionaki E, Tavernarakis N. Mechanisms of mitophagy in cellular homeostasis, physiology and pathology. Nat Cell Biol 2018;20:1013-22. [Crossref] [PubMed]
- D'Arcy MS. Mitophagy in health and disease. Molecular mechanisms, regulatory pathways, and therapeutic implications. Apoptosis 2024; Epub ahead of print. [Crossref] [PubMed]
- Lin M, Xian H, Chen Z, et al. MCM8-mediated mitophagy protects vascular health in response to nitric oxide signaling in a mouse model of Kawasaki disease. Nature Cardiovascular Research 2023;2:778-92. [Crossref]
- Liu T, Li X, Cui Y, et al. Bioinformatics Analysis Identifies Potential Ferroptosis Key Genes in the Pathogenesis of Intracerebral Hemorrhage. Front Neurosci 2021;15:661663. [Crossref] [PubMed]
- Shu Q, She H, Chen X, et al. Identification and experimental validation of mitochondria-related genes biomarkers associated with immune infiltration for sepsis. Front Immunol 2023;14:1184126. [Crossref] [PubMed]
- Guo C, Hua Y, Qian Z. Differentially expressed genes, lncRNAs, and competing endogenous RNAs in Kawasaki disease. PeerJ 2021;9:e11169. [Crossref] [PubMed]
- Newburger JW, Takahashi M, Gerber MA, et al. Diagnosis, treatment, and long-term management of Kawasaki disease: a statement for health professionals from the Committee on Rheumatic Fever, Endocarditis and Kawasaki Disease, Council on Cardiovascular Disease in the Young, American Heart Association. Circulation 2004;110:2747-71. [Crossref] [PubMed]
- Wang Z, Liu J, Li M, et al. Integrated bioinformatics analysis uncovers characteristic genes and molecular subtyping system for endometriosis. Front Pharmacol 2022;13:932526. [Crossref] [PubMed]
- Wang Y, Huang Z, Xiao Y, et al. The shared biomarkers and pathways of systemic lupus erythematosus and metabolic syndrome analyzed by bioinformatics combining machine learning algorithm and single-cell sequencing analysis. Front Immunol 2022;13:1015882. [Crossref] [PubMed]
- Pan S, Li Y, He H, et al. Identification of ferroptosis, necroptosis, and pyroptosis-associated genes in periodontitis-affected human periodontal tissue using integrated bioinformatic analysis. Front Pharmacol 2022;13:1098851. [Crossref] [PubMed]
- Li YK, Zeng T, Guan Y, et al. Validation of ESM1 Related to Ovarian Cancer and the Biological Function and Prognostic Significance. Int J Biol Sci 2023;19:258-80. [Crossref] [PubMed]
- Grissa D, Pétéra M, Brandolini M, et al. Feature Selection Methods for Early Predictive Biomarker Discovery Using Untargeted Metabolomic Data. Front Mol Biosci 2016;3:30. [Crossref] [PubMed]
- Cutler A, Stevens JR. Random forests for microarrays. Methods Enzymol 2006;411:422-32. [Crossref] [PubMed]
- Sanz H, Valim C, Vegas E, et al. SVM-RFE: selection and visualization of the most relevant features through non-linear kernels. BMC Bioinformatics 2018;19:432. [Crossref] [PubMed]
- Huang H, Jiang J, Shi X, et al. Nomogram to predict risk of resistance to intravenous immunoglobulin in children hospitalized with Kawasaki disease in Eastern China. Ann Med 2022;54:442-53. [Crossref] [PubMed]
- Liu Y, Li L, Jiang D, et al. A Novel Nomogram for Survival Prediction of Patients with Spinal Metastasis From Prostate Cancer. Spine (Phila Pa 1976) 2021;46:E364-73. [Crossref] [PubMed]
- Feng J, Tang X, Song L, et al. Potential biomarkers and immune characteristics of small bowel adenocarcinoma. Sci Rep 2022;12:16204. [Crossref] [PubMed]
- Lopez G, Costanza J, Colleoni M, et al. Molecular Insights into the Classification of Luminal Breast Cancers: The Genomic Heterogeneity of Progesterone-Negative Tumors. Int J Mol Sci 2019;20:510. [Crossref] [PubMed]
- Robin X, Turck N, Hainard A, et al. pROC: an open-source package for R and S+ to analyze and compare ROC curves. BMC Bioinformatics 2011;12:77. [Crossref] [PubMed]
- Newman AM, Liu CL, Green MR, et al. Robust enumeration of cell subsets from tissue expression profiles. Nat Methods 2015;12:453-7. [Crossref] [PubMed]
- Jin Y, Liu Y, Xu L, et al. Novel role for caspase 1 inhibitor VX765 in suppressing NLRP3 inflammasome assembly and atherosclerosis via promoting mitophagy and efferocytosis. Cell Death Dis 2022;13:512. [Crossref] [PubMed]
- Liu R, Xu C, Zhang W, et al. FUNDC1-mediated mitophagy and HIF1α activation drives pulmonary hypertension during hypoxia. Cell Death Dis 2022;13:634. [Crossref] [PubMed]
- Sonn SK, Song EJ, Seo S, et al. Peroxiredoxin 3 deficiency induces cardiac hypertrophy and dysfunction by impaired mitochondrial quality control. Redox Biol 2022;51:102275. [Crossref] [PubMed]
- Fu L, MacKeigan DT, Gong Q, et al. Thymic stromal lymphopoietin induces platelet mitophagy and promotes thrombosis in Kawasaki disease. Br J Haematol 2023;200:776-91. [Crossref] [PubMed]
- An X, Ma X, Liu H, et al. Inhibition of PDGFRβ alleviates endothelial cell apoptotic injury caused by DRP-1 overexpression and mitochondria fusion failure after mitophagy. Cell Death Dis 2023;14:756. [Crossref] [PubMed]
- Takahashi K, Oharaseki T, Naoe S, et al. Neutrophilic involvement in the damage to coronary arteries in acute stage of Kawasaki disease. Pediatr Int 2005;47:305-10. [Crossref] [PubMed]
- Griendling KK, Sorescu D, Ushio-Fukai M. NAD(P)H Oxidase. Circulation Research 2000;86:494-501. [Crossref] [PubMed]
- Tejero J, Shiva S, Gladwin MT. Sources of Vascular Nitric Oxide and Reactive Oxygen Species and Their Regulation. Physiol Rev 2019;99:311-79. [Crossref] [PubMed]
- Xu M, Qi Q, Men L, et al. Berberine protects Kawasaki disease-induced human coronary artery endothelial cells dysfunction by inhibiting of oxidative and endoplasmic reticulum stress. Vascul Pharmacol 2020;127:106660. [Crossref] [PubMed]
- Xie XF, Chu HJ, Xu YF, et al. Proteomics study of serum exosomes in Kawasaki disease patients with coronary artery aneurysms. Cardiol J 2019;26:584-93. [Crossref] [PubMed]
- Tang B, Lo HH, Lei C, et al. Adjuvant herbal therapy for targeting susceptibility genes to Kawasaki disease: An overview of epidemiology, pathogenesis, diagnosis and pharmacological treatment of Kawasaki disease. Phytomedicine 2020;70:153208. [Crossref] [PubMed]
- Huang C, Wang W, Huang H, et al. Kawasaki disease: ubiquitin-specific protease 5 promotes endothelial inflammation via TNFα-mediated signaling. Pediatr Res 2023;93:1883-90. [Crossref] [PubMed]
- Nagoya A, Sada R, Kimura H, et al. CKAP4 is a potential exosomal biomarker and therapeutic target for lung cancer. Transl Lung Cancer Res 2023;12:408-26. [Crossref] [PubMed]
- Kimura H, Yamamoto H, Harada T, et al. CKAP4, a DKK1 Receptor, Is a Biomarker in Exosomes Derived from Pancreatic Cancer and a Molecular Target for Therapy. Clin Cancer Res 2019;25:1936-47. [Crossref] [PubMed]
- Li SX, Li J, Dong LW, et al. Cytoskeleton-Associated Protein 4, a Promising Biomarker for Tumor Diagnosis and Therapy. Front Mol Biosci 2020;7:552056. [Crossref] [PubMed]
- Patel H, Ashton NJ, Dobson RJB, et al. Proteomic blood profiling in mild, severe and critical COVID-19 patients. Sci Rep 2021;11:6357. [Crossref] [PubMed]
- Zebardast A, Hasanzadeh A, Ebrahimian Shiadeh SA, et al. COVID-19: A trigger of autoimmune diseases. Cell Biol Int 2023;47:848-58. [Crossref] [PubMed]
- Yazici D, Cagan E, Tan G, et al. Disrupted epithelial permeability as a predictor of severe COVID-19 development. Allergy 2023;78:2644-58. [Crossref] [PubMed]
- Li K, Yao L, Wang J, et al. SARS-CoV-2 Spike protein promotes vWF secretion and thrombosis via endothelial cytoskeleton-associated protein 4 (CKAP4). Signal Transduct Target Ther 2022;7:332. [Crossref] [PubMed]
- Li Z, Li A, Yan L, et al. Downregulation of long noncoding RNA DLEU1 attenuates hypersensitivity in chronic constriction injury-induced neuropathic pain in rats by targeting miR-133a-3p/SRPK1 axis. Mol Med 2020;26:104. [Crossref] [PubMed]
- Manley JL, Krainer AR. A rational nomenclature for serine/arginine-rich protein splicing factors (SR proteins). Genes Dev 2010;24:1073-4. [Crossref] [PubMed]
- Zhou Z, Fu XD. Regulation of splicing by SR proteins and SR protein-specific kinases. Chromosoma 2013;122:191-207. [Crossref] [PubMed]
- Li XG, Wang YB. SRPK1 gene silencing promotes vascular smooth muscle cell proliferation and vascular remodeling via inhibition of the PI3K/Akt signaling pathway in a rat model of intracranial aneurysms. CNS Neurosci Ther 2019;25:233-44. [Crossref] [PubMed]
- Tzelepis K, De Braekeleer E, Aspris D, et al. SRPK1 maintains acute myeloid leukemia through effects on isoform usage of epigenetic regulators including BRD4. Nat Commun 2018;9:5378. [Crossref] [PubMed]
- Yao Y, Wang H, Xi X, et al. miR-150 and SRPK1 regulate AKT3 expression to participate in LPS-induced inflammatory response. Innate Immun 2021;27:343-50. [Crossref] [PubMed]
- Burns JC, Roberts SC, Tremoulet AH, et al. Infliximab versus second intravenous immunoglobulin for treatment of resistant Kawasaki disease in the USA (KIDCARE): a randomised, multicentre comparative effectiveness trial. Lancet Child Adolesc Health 2021;5:852-61. [Crossref] [PubMed]
- Ichiyama T, Yoshitomi T, Nishikawa M, et al. NF-kappaB activation in peripheral blood monocytes/macrophages and T cells during acute Kawasaki disease. Clin Immunol 2001;99:373-7. [Crossref] [PubMed]
- Huang JQ, Li HF, Zhu J, et al. SRPK1/AKT axis promotes oxaliplatin-induced anti-apoptosis via NF-κB activation in colon cancer. J Transl Med 2021;19:280. [Crossref] [PubMed]
- Wang Z, Xie L, Ding G, et al. Single-cell RNA sequencing of peripheral blood mononuclear cells from acute Kawasaki disease patients. Nat Commun 2021;12:5444. [Crossref] [PubMed]
- Franco A, Touma R, Song Y, et al. Specificity of regulatory T cells that modulate vascular inflammation. Autoimmunity 2014;47:95-104. [Crossref] [PubMed]
- Burns JC, Touma R, Song Y, et al. Fine specificities of natural regulatory T cells after IVIG therapy in patients with Kawasaki disease. Autoimmunity 2015;48:181-8. [Crossref] [PubMed]
- Koizumi K, Hoshiai M, Moriguchi T, et al. Plasma Exchange Downregulates Activated Monocytes and Restores Regulatory T Cells in Kawasaki Disease. Ther Apher Dial 2019;23:92-8. [Crossref] [PubMed]
- Xiao T, Li X, Felsenfeld G. The Myc-associated zinc finger protein epigenetically controls expression of interferon-γ-stimulated genes by recruiting STAT1 to chromatin. Proc Natl Acad Sci U S A 2024;121:e2320938121. [Crossref] [PubMed]
- Cao Y, Yao W, Lu R, et al. Reveal the correlation between hub hypoxia/immune-related genes and immunity and diagnosis, and the effect of SAP30 on cell apoptosis, ROS and MDA production in cerebral ischemic stroke. Aging (Albany NY) 2023;15:15161-82. [Crossref] [PubMed]
- Gu L, Hitzel J, Moll F, et al. The Histone Demethylase PHF8 Is Essential for Endothelial Cell Migration. PLoS One 2016;11:e0146645. [Crossref] [PubMed]
- Zhang Y, Gao Y, Jiang Y, et al. Histone demethylase KDM5B licenses macrophage-mediated inflammatory responses by repressing Nfkbia transcription. Cell Death Differ 2023;30:1279-92. [Crossref] [PubMed]
- Lou LQ, Zhou WQ, Song X, et al. Elevation of hsa-miR-7-5p level mediated by CtBP1-p300-AP1 complex targets ATXN1 to trigger NF-κB-dependent inflammation response. J Mol Med (Berl) 2023;101:223-35. [Crossref] [PubMed]