Metagenomic analysis of rats with diarrhea treated with mixed probiotics: response to consecutive and alternate-hour supplementation
Highlight box
Key findings
• Consecutive supplementation of probiotics had a positive impact on improving the intestinal homeostasis of acute diarrhea in rats compared with alternate-hour supplementation of probiotics.
What is known and what is new?
• Probiotics have a beneficial effect on improving diarrhea.
• The goal of this study was to ascertain whether administering probiotics separately at different periods (simultaneous vs. 2-hour intervals) would have a possible beneficial effect on intestinal microecology and to investigate the effects of the two combinations on the composition and function of the intestinal microbial community using second-generation sequencing of the metagenome.
What is the implication, and what should change now?
• The present study, based on metagenomics sequencing analysis, found that the difference between simultaneous administration of probiotics was more significant than spaced administration of probiotics in terms of both intestinal flora and metabolic pathways. This finding theoretically suggests that the simultaneous administration group should have had better clinical efficacy, but this was not the case; more in-depth basic and clinical studies are required. This study helps to elucidate the mechanism of action of probiotics in improving diarrhea to a certain extent.
Introduction
Pediatric diarrhea is a gastrointestinal disease caused by a variety of factors and is characterized by increased frequency and changes in the stools (1). Despite a steady decline in childhood diarrhea deaths over the past three decades, diarrhea continues to be the leading contributory factor of sickness and mortality among children under five (2). Therefore, it is necessary to intervene and treat infantile diarrhea as early as possible. Lai et al. found that there were more Proteobacteria counts and lower Firmicutes, Actinobacteriota, and Bacteroidetes populations in the stool of children with diarrhea (3). Regardless of the cause of acute diarrhea, the main methods of treatment are rehydration therapy, dietary therapy, and medication, of which medication includes probiotics (4). Clinical studies have found that a variety of probiotics have high safety and effectiveness for the treatment of pediatric diarrhea, and probiotics are strain- and dose-dependent (5-7). Despite the increasing clinical use of probiotics, the mechanism of action of probiotics on specific diseases, especially the effect on pre-existing flora, and the interactions between different probiotics are still not fully understood. Therefore, it is worthwhile to further investigate how probiotics can improve diarrhea. Three probiotics [Clostridium butyricum (C. butyricum), Bifidobacterium longum (B. longum) subspecies infantis, Saccharomyces boulardii (S. boulardii)] have been shown to have the potential to reduce the duration of diarrhea and hospitalization in children (8,9). With the advancement of efficient bioinformatics tools and high level of throughput metagenomic technologies, the scientific understanding of probiotics’ impact on gut microbiota has grown (10). Metagenomic studies help elucidate probiotics and human-microbe interactions (11). Therefore, macrogenomics can be used to evaluate the effect of probiotics on the composition as well as function of the gut microbiota in order to gain a deeper comprehension of probiotic-host interactions on health and disease (12).
He et al. reported that the combined application of Lactobacillus reuteri, Bacillus coagulans, B. longum, and C. butyricum has a synergistic effect of restoring intestinal microecological balance (13). Tompkins et al. found that non-enteric-coated bacterial probiotics should not be taken with or just before a fatty meal (14). However, few studies have reported on the different dosing times of probiotic complexes. Our preliminary research results reported functional data, including the general condition of rats (mental state, diet, water intake, activity response, fur color, weight change, fecal characteristics), and serum cytokine levels [C-reactive protein (CRP), procalcitonin (PCT), interleukin 6 (IL-6), tumor necrosis factor-α (TNF-α)], rat liver function [alanine aminotransferase (ALT), aspartate transaminase (AST), albumin], histopathological changes in rat colon mucosa (colon length, colon histological score). The results showed that C. butyricum, B. infantis, and S. boulardii significantly improved the clinical symptoms of diarrhea in rats and alleviated body weight loss, reduced serum inflammation in diarrhea and had a certain degree of hepatoprotection, attenuated the shortening of the length of the colon induced by dextran sulfate sodium (DSS), and promoted the restoration of the structural integrity of the colon. Although these results were somewhat as expected, the two modalities (consecutive and alternate-hour supplementation) were similar in terms of their clinical outcomes, and there was no significant difference in the clinical results (15). The goal of this study was to ascertain whether administering probiotics separately at different periods (simultaneous vs. 2-hour intervals) would have a possible beneficial effect on intestinal microecology and to investigate the effects of the two combinations on the composition and function of the intestinal microbial community using second-generation sequencing of the metagenome. We present this article in accordance with the ARRIVE reporting checklist (available at https://tp.amegroups.com/article/view/10.21037/tp-24-129/rc).
Methods
Animals and sample collection
Female Sprague-Dawley (SD) rats, aged 5 weeks, and with body weight of 145.03±0.54 g (mean ± standard error of the mean) were acquired from SPF (Beijing) Biotechnology Co., Ltd., with a temperature of 25±1 ℃, air humidity of 15–20%, good ventilation, and 12 hours of light and 12 hours of dark circulation every day. A total of 40 rats were randomly divided into cages and fed with standard rat feed. The water was changed regularly every day, and the rats were free to move and eat throughout the experiment, housed in pathogen-free facilities for 5 days to adapt to the circumstances before the experiment (16). A computer-based random sequence generator was used to number the rats, and they were then randomly assigned to four experimental groups in a completely randomized design. The control rats were kept normally without any intervention, and 30 remaining rats in the model, probiotic A, and probiotic B groups were given 5% DSS drinking water to induce an acute diarrhea model. This experiment was conducted as a double-blind trial. For each animal, three different investigators were involved as follows: a first investigator administered the treatment based on the randomization table. This investigator was the only person aware of the treatment group allocation. A second investigator was responsible for the experimental procedure. Finally, a third investigator (also unaware of treatment) conducted data measurement. A protocol was prepared before the study without registration.
After modeling using the above method, the model group was given 0.9% saline by gavage, 2 mL each time, twice daily. Probiotic group A was given 1 mL of 1.35% combined C. butyricum and Bifidobacterium powders solution (270 mg/kg/day; drug batch number: S20020014; Shandong Kexing Bioproducts Co., Ltd., Jinan, China) and 0.45% S. boulardii sachets solution 1 mL (90 mg/kg/day; drug batch number: S20020014; Laboratoires Biocodex, Paris, France) by gavage at the same time, twice daily. Probiotics group B was first given 1 mL of 1.35% combined C. butyricum and Bifidobacterium powders solution by gavage, followed by 1 mL of 0.45% S. boulardii sachets solution by gavage 2 hours later, twice daily. The experiment was conducted for 7 consecutive days. The rest of the time, the rats in each group were allowed to eat and drink freely. We will refer to the control group as KB, the model group as MX, the probiotic group A as GY, and the probiotic group B as JG. During the 7-day experiment, the general condition of the rats (spirit, water intake, diet, activity response, and coat color), fecal traits, and body weight changes were recorded every day. A total of 40 rats were used in the experiment. These groups originally numbered 10 animals. The KB rats generally performed well, and none of the 30 rats in the MX, GY, and JG died throughout the entire modeling period. During the modeling process, the rats showing signs of mental fatigue, decreased activity, weight loss, and loose stools (without bloody stools), indicated successful modeling. Therefore, when the above symptoms appeared, no welfare measures were given to the animals. If the rats experienced hemafecia or died prematurely, they were excluded. These rats were successfully modeled. During the experimental process, adverse events were not observed and no animal reached any of the humane endpoints. Therefore, on the 7th day of the experiment, none of them were removed from the study; each group of rats was euthanized using cervical dislocation method, and cardiac puncture and centrifugation were used to collect fresh blood for serum samples for serum immunological index measurement. The feces of rats in each group were collected and then frozen and stored in a −80 °C refrigerator. Metagenomic next-generation sequencing was used to analyze the fecal samples (Figure 1). Animal experiments were conducted at Hubei Experimental Animal Research Center (within Hubei Disease Control and Prevention Center). All animal procedures were performed in accordance with the Guidelines for Care and Use of Laboratory Animals of Hubei Disease Control and Prevention Center and approved by the Animal Ethics Committee of Hubei Disease Control and Prevention Center (No. 202420035).
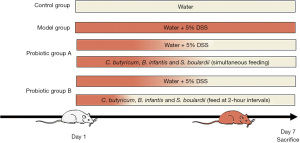
Measurement of immunological indicators
When the experiment had concluded, rats in each group were executed separately using the cervical dislocation method, fresh blood was collected by cardiac puncture, and serum samples were collected by centrifugation. Enzyme-linked immunosorbent assay (ELISA) kits for rats were used for the measurement of immunoglobulin G (IgG), rat immunoglobulin M (IgM), and rat immunoglobulin A (IgA). A Rayto RT-6100 Enzyme Analyzer (Rayto, Shenzhen, China) was used to determine the levels of IgG, IgM, and IgA in serum according to the manufacturer’s instructions.
DNA extraction
The DNeasy® PowerSoil® Pro Kit (QIAGEN, Germantown, MD, USA) was used for extracting DNA from fecal samples. DNA extraction was performed by centrifugation column method. The quality of DNA was checked using agarose gel electrophoresis. The concentration was detected by the Qubit 3.0 Analysis Kit (Thermo Fisher Scientific, Waltham, MA, USA), all the operations were carried out concerning the method of the instruction manual. Then, the samples were stored at −20 ℃ until use.
Sequencing of metagenomics and quality assurance
Library construction was performed using NEXTFLEX Rapid DNA-Seq Kit (Revvity, Waltham, MA, USA), with the following specific process: (I) joint linking; (II) use magnetic beads to screen and remove self-connected fragments of the connector; (III) enrichment of library templates using polymerase chain reaction (PCR) amplification; (IV) the magnetic beads were used to recover PCR products and obtain the final library. The sequencing platform Illumina NovaSeq 6000 (Illumina, San Diego, CA, USA) was used for metagenomic sequencing.
Strict quality assurance of the data was carried out by performing the following filtering methods: (I) eliminating junction containing reads; (II) elimination of low-quality reads, including those where more than 10% of the N was fired; removal of reads with more than 50% of the bases of the entire reads with quality value Q≤10, Following the aforementioned quality control steps, high-quality clean data was expressed in FASTQ format. Next, the reads of all the samples were merged and assembled by splicing using the splicing software MEGAHIT or IDBA-UD (17,18) founded on the de Brujin graph’s principles, and based on the overlap relationship between kmer. Using the de Brujin graph, the contigs were formed, and the screened contigs above 800 bp were counted and used for subsequent analyses (Table S1).
Using the splicing software MEGAHIT based on the principle of successful de Bruijn graphs (19) (https://github.com/voutcn/megahit) (Version 1.1.2), the optimized sequence was spliced and assembled. Based on genome assembly, the prediction analysis of genes was carried out, and the predicted genes were subjected to gene set construction as well as functional annotation analysis. (I) Open reading frame (ORF) prediction of the spliced contigs sequences was performed using Prodigal (20) (http://metagene.cb.k.u-tokyo.ac.jp/) software and converted into sequences of amino acid; (II) using CD-HIT (http://metagene.cb.k.u-tokyo.ac.jp/) software, the ORF prediction findings of each sample and hybrid assembly were subjected to de-redundancy to provide the first gene catalogue of non-redundant (genes are the nucleic acid sequences encoded by successive non-redundant genes), and clustered by default with 95% identity and 90% coverage, and the representative sequence was determined to be the longest; (III) the clean readings from each sample were compared individually (95% identity) with the non-redundant gene set using bowtie2 (https://bowtie-bio.sourceforge.net/bowtie2/index.shtml) software, and the abundance information of the genes in the associated samples were counted; (IV) the number of reads on the comparison and the gene length were used to compute the abundance information of each gene in each sample (21).
Taxonomy and functional annotation
The length of the gene catalogue was counted using Python (https://www.python.org/) and then analyzed for species abundance: (I) a comparison of genes with functional databases was performed using the DIAMOND (22) software to compare unigenes with bacterial (bacteria), fungal (fungi), archaeal (archaea), and viral (viruses) sequences sampled from the National Center for Biotechnology Information (NCBI)’s NR (Version: 2021.11) database (blastp, evalue ≤1e−5) (23); (II) filtering of the comparison results: those with an evalue ≤ minimal evalue*10 were selected for additional evaluation out of all the comparison results for each sequence; (III) after the filtering process, since there may be more than one comparison for each sequence, the comparison results were selected for subsequent analyses; its sequence could yield several comparison results as well as information on multiple species classifications, ensuring its biological significance. The classification level prior to the emergence of the first branch was utilized as the species annotation information of the sequence, and the lowest common ancestor (LCA) algorithm (applied to the systematic classification of MEGAN software) was adopted.
Each functional database was compared with non-redundant genes using the DIAMOND software, and annotations with evalue <1e−5 were taken to filter the proteins with the greatest sequence similarity, thus obtaining the functional annotation information. (I) Filtering of the comparison results: for the comparison results of each sequence, the comparison result with the highest score (one HSP >60 bits) was selected for the subsequent analysis (24); (II) based on the comparison outcomes, the relative abundance of various functional tiers was determined (each functional tier’s relative abundance was calculated as the total of the relative abundance of the genes associated with that functional tier) (25-27), in which the Kyoto Encyclopedia of Genes and Genomes (KEGG) database was divided into five tiers (28), the evolutionary genealogy of genes: Non-supervised Orthologous Groups (eggNOG) database was divided into three tiers (29), and the Carbohydrate-Active Enzyme (CAZy) database was divided into three tiers (30); (III) a table demonstrating the number of genes in each sample at each taxonomic level was created using the functional annotation results and gene abundance tables. For each function, the number of genes in a sample was equal to the number of genes for which the abundance among the genes annotated for that function was not zero.
Statistical analyses
The data were statistically analyzed using SPSS software, version 27.0.1 (IBM Corp., Armonk, NY, USA), and comparison between groups was performed with the t-test or Kruskal-Wallis test. Principal coordinate analysis (PCoA) was performed based on Bray-Curtis distance and the principal coordinate combination with the highest contribution rate was selected for graphical display. Differential species were detected between different groups using rank sum test and dimensionality reduction was implemented through linear discriminant analysis (LDA) to evaluate the impact of differential species, in order to screen for species with significant differences between groups as potential biomarkers. Correlation analysis was performed using Spearman’s analysis, with P<0.05 considered statistically significant, and graphs were generated using Origin 9.0 (OriginLab Corp., Northampton, MA, USA).
Results
Immunological changes after probiotics administration
Serum immunoglobulins (IgA, IgG, IgM) were slightly increased in JG compared to GY, but serum immunoglobulins (IgA, IgG, and IgM) were elevated in both GY and JG compared to KB and there was no significant difference between the two groups; additionally, there was a significant difference between the two groups and a significant increase in serum immunoglobulins (IgA, IgG, and IgM) compared to the model group (Figure 2, Table 1). In conclusion, the improvement of intestinal homeostasis by the combination of probiotics may be related to the ability to regulate serum immunoglobulins; however, there was no significant difference in the modulation of immunoglobulins between simultaneous and interval administration of probiotics.
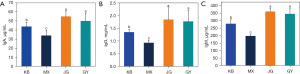
Table 1
Group | IgA (μg/mL) | IgG (mg/mL) | IgM (μg/mL) |
---|---|---|---|
Probiotic group A (GY) (%) | 49.882±8.594 | 1.766±0.430 | 344.940±34.892 |
Probiotic group B (JG) (%) | 54.891±5.367 | 1.838±0.465 | 360.603±21.767 |
Control group (KB) (%) | 43.740±4.941 | 1.342±0.122 | 278.928±28.244 |
Model group (MX) (%) | 33.858±5.875 | 0.926±0.102 | 197.474±8.760 |
t value | |||
KB vs. GY | 1.936 | 2.855 | 0.708 |
KB vs. JG | 4.717 | 3.105 | 6.998 |
GY vs. JG | −1.483 | −0.341 | −1.143 |
MX vs. GY | 4.789 | 5.715 | 12.334 |
MX vs. JG | 8.115 | 5.760 | 21.868 |
KB vs. MX | 4.071 | 8.306 | 8.711 |
P value | |||
KB vs. GY | 0.07 | 0.02 | <0.001 |
KB vs. JG | <0.001 | 0.01 | <0.001 |
GY vs. JG | 0.16 | 0.74 | 0.27 |
MX vs. GY | <0.001 | <0.001 | <0.001 |
MX vs. JG | <0.001 | <0.001 | <0.001 |
KB vs. MX | <0.001 | <0.001 | <0.001 |
Data are presented as mean ± standard error of the mean. Control group (KB): fed with water; model group (MX): fed with 5% DSS to induce acute diarrhea; probiotic group A (GY): acute diarrhea model fed with Clostridium butyricum, Bifidobacterium infantis, and Saccharomyces boulardii at the same time; probiotics group B (JG): acute diarrhea model fed with Clostridium butyricum, Bifidobacterium infantis, and Saccharomyces boulardii at 2-hour interval. Ig, immunoglobulin; DSS, dextran sodium sulfate.
Diversity modulation of gut microbiota by probiotics
Fecal samples were collected at the end of this experiment (day 7) to compare the changes in the alpha diversity of intestinal bacteria among the four groups, and the Shannon diversity index, Simpson index, and inverse Simpson (invsimpson) index were chosen to assess the bacterial diversity of the different groups (Figure 3, Table 2). The results showed that different associations of probiotics could not cause changes in Shannon index (H=4.03, P=0.26), Simpson index (H=4.68, P=0.20), and invsimpson index (H=4.68, P=0.20), and the groups did not differ significantly from one another.
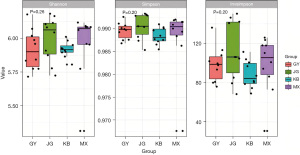
Table 2
Group | Shannon index | Simpson index | Invsimpson index |
---|---|---|---|
Probiotic group A | 5.901 (5.775, 6.032) | 0.990 (0.988, 0.991) | 98.782 (80.659, 109.309) |
Probiotic group B | 6.063 (5.851, 6.127) | 0.991 (0.988, 0.993) | 106.220 (83.770, 143.188) |
Control group | 5.917 (5.888, 5.959) | 0.988 (0.987, 0.990) | 84.399 (77.656, 101.461) |
Model group | 6.073 (5.909, 6.086) | 0.990 (0.988, 0.992) | 105.645 (83.000, 119.440) |
H value | 4.032 | 4.677 | 4.677 |
P value | 0.26 | 0.20 | 0.20 |
Data are presented as median (interquartile range). Control group: fed with water; model group: fed with 5% DSS to induce acute diarrhea; probiotic group A: acute diarrhea model fed with Clostridium butyricum, Bifidobacterium infantis, and Saccharomyces boulardii at the same time; probiotics group B: acute diarrhea model fed with Clostridium butyricum, Bifidobacterium infantis, and Saccharomyces boulardii at 2-hour interval. Invsimpson, inverse Simpson.
At the conclusion of the experiment, the intestinal flora of the four groups of rats did not differ statistically significantly: phylum level (F=1.143, P=0.35), class level (F=1.474, P=0.20), order level (F=1.471, P=0.24), family level (F=1.265, P=0.23), genus level (F=1.717, P=0.07), species level (F=1.670, P=0.057), according to PCoA based on Bray-Curtis distance (Figure 4, Table 3). These results indicate that neither the simultaneous administration of probiotics nor the administration of probiotics at 2-hour intervals had a significant effect on the intestinal bacterial structure of the rats.
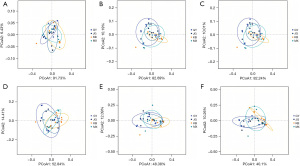
Table 3
Taxonomy | R2 | F value | P value |
---|---|---|---|
Phylum level | 0.087 | 1.143 | 0.35 |
Class level | 0.109 | 1.474 | 0.20 |
Order level | 0.109 | 1.471 | 0.24 |
Family level | 0.095 | 1.265 | 0.23 |
Genus level | 0.125 | 1.717 | 0.07 |
Species level | 0.122 | 1.670 | 0.057 |
Changes in gut microbiota
At the level of phylum, the abundance of Fusobacteria was decreased (t=2.110, P=0.049) in JG compared to GY. Compared to KB, the abundance of Actinobacteria (t=2.483, P=0.02), Fusobacteria (t=2.714, P=0.02) was increased in GY, whereas that of Fibrobacteres (t=−2.656, P=0.02), Euryarchaeota (t=−4.132, P<0.001), and Cyanobacteria (t=−4.311, P<0.001) decreased. In JG, Fibrobacteres (t=−2.789, P=0.01), Euryarchaeota (t=−3.866, P=0.001), and Cyanobacteria (t=−3.435, P=0.003) decreased in abundance, and Actinobacteria (t=1.810, P=0.09) increased in abundance. Cyanobacteria (t=−3.014, P=0.007) reduced in comparison to MX in GY (Figure 5, Table 4).
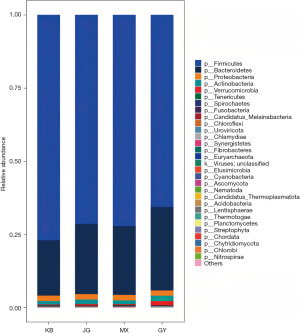
Table 4
Group | Fusobacteria | Actinobacteria | Fibrobacteres | Euryarchaeota | Cyanobacteria |
---|---|---|---|---|---|
Probiotic group A (GY) (%) | 0.10±0.03 | 1.85±0.72 | 0.03±0.01 | 0.02±0.01 | 0.02±0.01 |
Probiotic group B (JG) (%) | 0.07±0.03 | 1.55±0.48 | 0.03±0.01 | 0.02±0.01 | 0.02±0.00 |
Control group (KB) (%) | 0.07±0.01 | 1.16±0.50 | 0.04±0.01 | 0.03±0.01 | 0.03±0.00 |
Model group (MX) (%) | 0.08±0.02 | 1.29±0.33 | 0.04±0.01 | 0.03±0.01 | 0.02±0.01 |
t value | |||||
KB vs. GY | 2.714 | 2.483 | −2.656 | −4.132 | −4.311 |
KB vs. JG | 0.121 | 1.810 | −2.789 | −3.866 | −3.435 |
GY vs. JG | 2.110 | 1.076 | −0.329 | −0.455 | −1.443 |
MX vs. GY | 1.934 | 2.192 | −1.542 | −1.510 | −3.014 |
MX vs. JG | −0.498 | 1.398 | −1.420 | −1.130 | −1.921 |
KB vs. MX | −0.828 | −0.727 | 0.978 | 2.441 | 1.208 |
P value | |||||
KB vs. GY | 0.02 | 0.02 | 0.02 | <0.001 | <0.001 |
KB vs. JG | 0.91 | 0.09 | 0.01 | 0.001 | 0.003 |
GY vs. JG | 0.049 | 0.30 | 0.75 | 0.66 | 0.17 |
MX vs. GY | 0.07 | 0.048 | 0.14 | 0.15 | 0.007 |
MX vs. JG | 0.62 | 0.18 | 0.17 | 0.27 | 0.07 |
KB vs. MX | 0.42 | 0.48 | 0.34 | 0.03 | 0.24 |
Data are presented as mean ± standard error of the mean. Control group (KB): fed with water; model group (MX): fed with 5% DSS to induce acute diarrhea; probiotic group A (GY): acute diarrhea model fed with Clostridium butyricum, Bifidobacterium infantis, and Saccharomyces boulardii at the same time; probiotics group B (JG): acute diarrhea model fed with Clostridium butyricum, Bifidobacterium infantis, and Saccharomyces boulardii at 2-hour interval. DSS, dextran sodium sulfate.
At the level of genus, the relative abundance of Akkermansia (t=1.597, P=0.13) was increased in GY compared to JG. The abundance of Lactobacillus (t=3.053, P=0.01) and Akkermansia (t=2.125, P=0.06) was increased in GY compared to the KB, whereas Oscillibacter (t=−3.371, P=0.003), Roseburia (t=−2.447, P=0.03), and Lachnoclostridium (t=−2.929, P=0.009) decreased in abundance. JG had increased abundance of Lactobacillus (t=3.404, P=0.007), whereas the abundance of Prevotella (t=−2.963, P=0.02), Oscillibacter (t=−2.471, P=0.02), and Roseburia (t=−3.177, P=0.008) decreased. Compared with MX, Ruminococcus’s relative abundance in GY and JG (t=−4.079, P=0.001; t=−2.982, P=0.01) decreased; the decrease in GY was more obvious, and Lactobacillus (t=2.186, P=0.050) had increased in abundance in GY (Figure 6, Table 5).
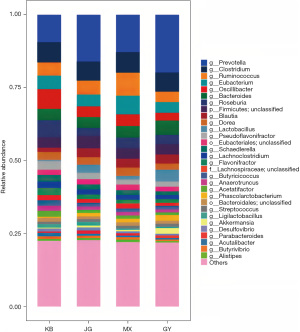
Table 5
Group | Akkermansia | Prevotella | Lactobacillus | Oscillibacter | Roseburia | Lachnoclostridium | Ruminococcus |
---|---|---|---|---|---|---|---|
Probiotic group A (GY) (%) | 1.95±2.43 | 0.01±0.00 | 3.91±3.59 | 2.85±2.61 | 3.25±1.81 | 1.41±0.52 | 3.66±1.43 |
Probiotic group B (JG) (%) | 0.64±0.97 | 0.01±0.01 | 2.65±2.02 | 3.82±2.85 | 2.80±1.07 | 1.77±0.59 | 4.75±1.49 |
Control group (KB) (%) | 0.31±0.36 | 0.11±0.11 | 0.41±0.49 | 6.96±2.83 | 5.88±2.87 | 2.20±0.67 | 4.50±2.38 |
Model group (MX) (%) | 0.48±0.63 | 0.06±0.12 | 1.25±1.41 | 4.04±2.07 | 3.67±2.08 | 1.59±0.31 | 7.82±2.89 |
t value | |||||||
KB vs. GY | 2.125 | −3.001 | 3.053 | −3.371 | −2.447 | −2.929 | −0.952 |
KB vs. JG | 1.002 | −2.963 | 3.404 | −2.471 | −3.177 | −1.509 | 0.288 |
GY vs. JG | 1.597 | −0.669 | 0.967 | −0.794 | 0.677 | −1.428 | −1.672 |
MX vs. GY | 1.856 | −1.351 | 2.186 | −1.125 | −0.476 | −0.945 | −4.079 |
MX vs. JG | 0.414 | −1.318 | 1.806 | −0.193 | −1.171 | 0.830 | −2.982 |
KB vs. MX | −0.775 | 1.011 | −1.752 | 2.635 | 1.974 | 2.590 | −2.806 |
P value | |||||||
KB vs. GY | 0.06 | 0.02 | 0.01 | 0.003 | 0.03 | 0.009 | 0.35 |
KB vs. JG | 0.33 | 0.02 | 0.007 | 0.02 | 0.008 | 0.15 | 0.78 |
GY vs. JG | 0.13 | 0.51 | 0.35 | 0.44 | 0.51 | 0.17 | 0.11 |
MX vs. GY | 0.08 | 0.21 | 0.050 | 0.28 | 0.64 | 0.36 | 0.001 |
MX vs. JG | 0.68 | 0.22 | 0.09 | 0.85 | 0.26 | 0.42 | 0.01 |
KB vs. MX | 0.45 | 0.33 | 0.10 | 0.02 | 0.06 | 0.02 | 0.01 |
Data are presented as mean ± standard error of the mean. Control group (KB): fed with water; model group (MX): fed with 5% DSS to induce acute diarrhea; probiotic group A (GY): acute diarrhea model fed with Clostridium butyricum, Bifidobacterium infantis, and Saccharomyces boulardii at the same time; probiotics group B (JG): acute diarrhea model fed with Clostridium butyricum, Bifidobacterium infantis, and Saccharomyces boulardii at 2-hour interval.
At the level of species, the relative abundance of Oscillibacter sp_1-3 (t=−2.513, P=0.02) and Bacteroidales bacterium_55_9 (t=−3.179, P=0.009) decreased in JG in contrast to KB. The decrease in these two species was more significant in GY [Oscillibacter sp_1-3 (t=−3.575, P=0.002) and the decrease in Bacteroidales bacterium_55_9 (t=−3.404, P=0.007)] in GY. Phascolarctobacterium succinatutens increased in abundance in JG (t=3.055, P=0.007), yet the increase was more pronounced in GY (t=3.933, P=0.002), and the difference between JG and GY was statistically significant. The abundance of Lactobacillus johnsonii was increased when comparing GY to KB (t=3.067, P=0.01). Compared with MX, the abundance of Lactobacillus johnsonii in GY increased (t=2.283, P=0.042) (Figure 7, Table 6).
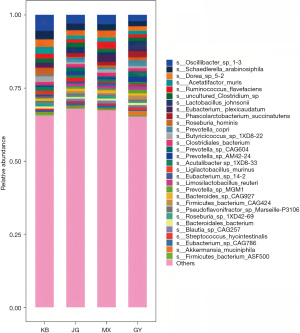
Table 6
Group | Oscillibacter_sp_1–3 | Bacteroidales bacterium_55_9 | Phascolarctobacterium succinatutens | Lactobacillus johnsonii |
---|---|---|---|---|
Probiotic group A (GY) (%) | 2.24±2.02 | 0.01±0.00 | 1.96±1.13 | 2.60±2.47 |
Probiotic group B (JG) (%) | 3.05±2.30 | 0.01±0.00 | 1.15±0.54 | 1.54±1.20 |
Control group (KB) (%) | 5.52±2.09 | 0.02±0.02 | 0.38±0.59 | 0.20±0.24 |
Model group (MX) (%) | 3.22±1.60 | 0.01±0.01 | 1.19±0.76 | 0.70±0.93 |
t value | ||||
KB vs. GY | −3.575 | −3.404 | 3.933 | 3.067 |
KB vs. JG | −2.513 | −3.179 | 3.055 | 3.478 |
GY vs. JG | −0.838 | −0.389 | 2.049 | 1.226 |
MX vs. GY | −1.208 | −1.585 | 1.787 | 2.283 |
MX vs. JG | −0.193 | −1.322 | −0.143 | 1.755 |
KB vs. MX | 2.768 | 1.879 | −2.679 | −1.644 |
P value | ||||
KB vs. GY | 0.002 | 0.007 | 0.002 | 0.01 |
KB vs. JG | 0.02 | 0.009 | 0.007 | 0.006 |
GY vs. JG | 0.41 | 0.70 | 0.06 | 0.24 |
MX vs. GY | 0.24 | 0.14 | 0.09 | 0.042 |
MX vs. JG | 0.85 | 0.21 | 0.89 | 0.10 |
KB vs. MX | 0.01 | 0.08 | 0.02 | 0.13 |
Data are presented as mean ± standard error of the mean. Control group (KB): fed with water; model group (MX): fed with 5% DSS to induce acute diarrhea; probiotic group A (GY): acute diarrhea model fed with Clostridium butyricum, Bifidobacterium infantis, and Saccharomyces boulardii at the same time; probiotics group B (JG): acute diarrhea model fed with Clostridium butyricum, Bifidobacterium infantis, and Saccharomyces boulardii at 2-hour interval. DSS, dextran sodium sulfate.
LDA effect size analysis
In order to screen for biomarkers with significant differences between groups, the difference species between different groups were first detected through rank sum test, and LDA was used to reduce dimensionality and evaluate the impact of different species, resulting in the LDA score. The enriched microbiota of GY included Prevotella_sp_CAG604, Phascolarctobacterium_succinatutens, Prevotella_copri, Prevotella_sp_AM42_24, Akkermansia_muciniphila, Clostridium_sp_CAG678, Coriobacteriia_bacerium, Prevotella_sp_CAG1058, Prevotella_sp_CAG1185, Blautia_argi, Prevotella_sp_CAG1124, and Anaeromassilibacillus_sp_An172. The enriched microbiota of JG was Roseburia_sp_CAG309. The enriched microbiota of MX was Blautia_sp_CAG257, Ruminococcus_sp_Marseille_P6503, and Blautia_sp_Marseille_P3201T. The enriched microbiota of KB was Oscillibacter_sp_1-3, Acetatifactor_muris, Butyricicoccus_sp_1XD8_22, Roseburia_sp_CAG303, Oscillibacter_sp_PC13, Anaerotruncus_sp_G32012, and Oscillibacter_sp_CAG155 (LDA threshold >3.0) (Figure 8).
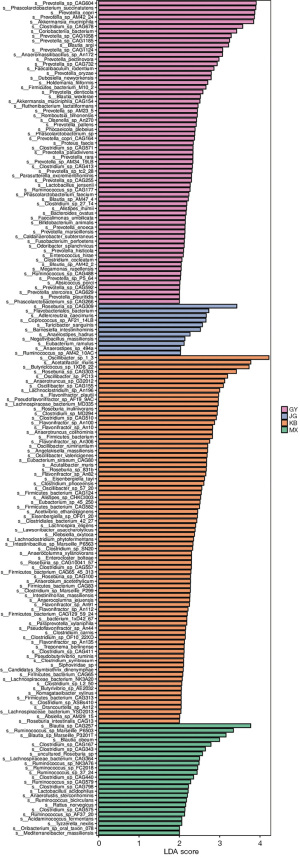
Functional gene alteration
In the eggNOG metabolic pathways, three metabolic pathways with significant differences were found in GY compared with KB, in which P: Inorganic ion transport and metabolism pathway (t=3.787, P=0.04), E: Amino acid transport and metabolism pathway (t=3.507, P=0.005) were increased in abundance in GY group, whereas K: Transcription pathway (t=−2.923, P=0.04) was decreased in abundance in GY compared to KB. Two metabolic pathways with significant differences were found in JG compared to KB, namely E: Amino acid transport and metabolism pathway (t=4.172, P=0.003), P: Inorganic ion transport and metabolism pathway (t=4.420, P=0.03); both metabolic pathways were higher in JG. In KEGG metabolic pathways, compared to KB, in GY, there were four significantly different KEGG pathways, three of which: one carbon pool by folate (PATH: ko00670) (t=4.612, P=0.02), porphyrin and chlorophyll metabolism (PATH: ko00860) (t=5.785, P=0.047), and selenocompound metabolism (PATH: ko00450) (t=3.781, P=0.03) were increased in abundance in GY, whereas cell motility (t=−5.528, P=0.01) was decreased in abundance in GY in contrast to KB. In the metabolic pathways of CAZy, one metabolic pathway, the GH8 (glycoside hydrolase family 8) metabolic pathway, was found to be significantly different in GY compared with KB (t=4.155, P=0.02) (Figures 9-11, Table 7).
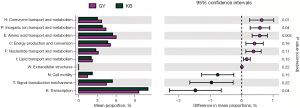
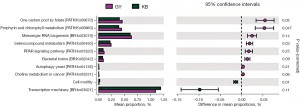
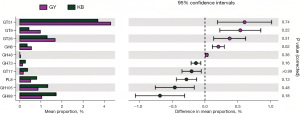
Table 7
Group | KEGG | CAZy | eggNOG | |||||||
---|---|---|---|---|---|---|---|---|---|---|
One carbon pool by folate (PATH: ko00670) | Porphyrin and chlorophyll metabolism (PATH: ko00860) | Selenocompound metabolism (PATH: ko00450) | Cell motility | Glycoside Hydrolase Family 8 | P: Inorganic ion transport and metabolism | E: Amino acid transport and metabolism | K: Transcription | |||
Probiotic group A (GY) (%) | 3,480.958±339.169 | 3,368.791±242.132 | 1,836.698±161.113 | 70.910±42.239 | 66.071±19.247 | 44,725.499±3,380.265 | 32,495.243±3,715.999 | 71,371.947±5,781.980 | ||
Probiotic group B (JG) (%) | 3,366.241±235.794 | 3,457.640±369.448 | 1,813.249±114.329 | 91.934±34.663 | 57.848±15.714 | 44,766.557±2,727.302 | 31,956.782±2,508.184 | 75,372.276±4,187.976 | ||
Control group (KB) (%) | 2,892.185±218.959 | 2,801.323±193.877 | 1,599.867±115.234 | 158.832±27.312 | 38.394±8.563 | 39,973.806±2,078.370 | 27,968.824±1,687.457 | 79,803.788±7,057.325 | ||
Model group (MX) (%) | 3,245.163±351.657 | 3,375.826±524.712 | 1,836.698±161.113 | 104.494±41.881 | 48.135±15.765 | 42,202.205±2,747.064 | 30,020.183±2,856.570 | 75,193.231±6,510.051 | ||
t value | ||||||||||
KB vs. GY | 4.612 | 5.785 | 3.781 | −5.528 | 4.155 | 3.787 | 3.507 | −2.923 | ||
KB vs. JG | 4.659 | 4.974 | 4.157 | −4.794 | 3.438 | 4.420 | 4.172 | −1.708 | ||
GY vs. JG | 0.878 | −0.636 | 0.375 | −1.217 | 1.046 | −0.030 | 0.380 | −1.772 | ||
MX vs. GY | 1.604 | −0.038 | 2.266 | −1.785 | 2.280 | 1.832 | 1.670 | −1.388 | ||
MX vs. JG | 0.967 | 0.403 | 2.267 | −0.731 | 1.380 | 2.095 | 1.611 | 0.073 | ||
KB vs. MX | −2.895 | −3.248 | −1.472 | 3.437 | −1.717 | −2.046 | −1.955 | 1.519 | ||
Adjusted P value | ||||||||||
KB vs. GY | 0.02 | 0.047 | 0.03 | 0.01 | 0.02 | 0.04 | 0.005 | 0.04 | ||
KB vs. JG | 0.24 | >0.99 | 0.19 | 0.01 | 0.48 | 0.03 | 0.003 | 0.12 | ||
GY vs. JG | >0.99 | >0.99 | >0.99 | >0.99 | >0.99 | >0.99 | >0.99 | >0.99 | ||
MX vs. GY | >0.99 | >0.99 | >0.99 | >0.99 | >0.99 | >0.99 | >0.99 | >0.99 | ||
MX vs. JG | >0.99 | >0.99 | >0.99 | >0.99 | >0.99 | >0.99 | >0.99 | >0.99 | ||
KB vs. MX | >0.99 | >0.99 | >0.99 | 0.62 | >0.99 | 0.86 | 0.44 | 0.99 |
Data are presented as mean ± standard error of the mean. Control group (KB): fed with water; model group (MX): fed with 5% DSS to induce acute diarrhea; probiotic group A (GY): acute diarrhea model fed with Clostridium butyricum, Bifidobacterium infantis, and Saccharomyces boulardii at the same time; probiotics group B (JG): acute diarrhea model fed with Clostridium butyricum, Bifidobacterium infantis, and Saccharomyces boulardii at 2-hour interval. KEGG, Kyoto Encyclopedia of Genes and Genomes; CAZy, Carbohydrate-Active Enzymes database; eggNOG, evolutionary genealogy of genes: Non-supervised Orthologous Groups.
Correlations between taxa and pathways
Utilizing Spearman correlation analysis, the association between gut microbiota and metabolic pathways was investigated, which may indicate potential associations between microorganisms and function. Some potentially beneficial bacteria such as Phascolarctobacterium spp. (r=0.903, r=0.782, r=0.915, r=−0.964) and Phascolarctobacterium succinatutens (r=0.903, r=0.903, r=0.976, r=−0.952) were positively correlated with the expression of glycoside hydrolases, amino acid, and inorganic ion transport metabolism, and negatively correlated with cell motility. Some potentially harmful bacteria such as Spirochaetes (r=0.867, r=−0.842, r=−0.733, r=−0.903), Fibrobacteres (r=0.745, r=−0.818, r=−0.733, r=−0.842), and Cyanobacteria (r=0.842, r=−0.806, r=−0.855, r=−0.964), the genus Lachnoclostridium (r=0.927, r=−0.879, r=−0.782, r=−0.867), the genus Aspergillus (Flavonifractor) (r=0.964, r=−0.879, r=−0.782, r=−0.879), Anaerotruncus (r=0.952, r=−0.879, r=−0.770, r=−0.879), Acetatifactor (r=0.939, r=−0.927, r=−0.733, r=−0.891), Acetalibacter (r=0.927, r=−0.855, r=−0.733, r=−0.879), Oscillibacter_sp_1-3 (r=0.939, r=−0.842, r=−0.745, r=−0.867), Butyricoccus_sp_1XD8-22 (r=0.964, r=−0.879, r=−0.782, r=−0.879) were positively correlated with cell motility, whereas the expression of glycoside hydrolases, and amino acid and inorganic ion transport metabolism were negatively correlated (Figure 12). Taken together, these results suggest that the homeostasis of the intestinal flora may be related to the expression of glycoside hydrolases and the amino acid and inorganic ion transport metabolism pathways, suggesting that these factors may work as a whole to ameliorate acute diarrhea.
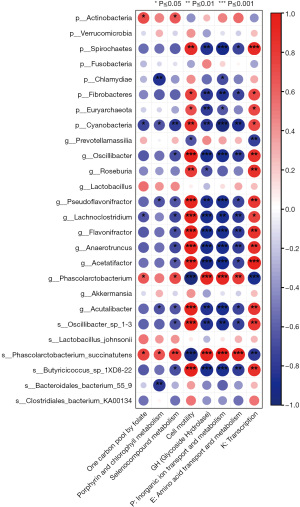
Discussion
The etiology of diarrhea in children is complex and related to age, diet, climate, living environment, socio-economic development, and genetics (10). Regardless of its cause, diarrhea itself can lead to an imbalance in the intestinal flora. Although the results of many randomized controlled trials suggest that probiotics can be used for the treatment of diarrhea (31), the exact mechanism by which probiotics can treat diarrhea is still unclear. In the present study, we assessed the modulation of the intestinal microbiota of rats by different temporal associations with probiotics, using metagenomics next-generation sequencing technology. Probiotics have been demonstrated in earlier research to enhance host health by modifying the intestinal microbiome (32). Our results suggest that giving multi-strain probiotics at intervals does not better improve the gut microbiota, whereas giving multi-strain probiotics has a more significant alteration of the gut flora and is more conducive to restoring a healthy gut microbiota.
At the phylum level, the relative abundance of Actinobacteriota was increased in GY compared with KB and MX, which may be related to the alleviation of diarrhea by probiotics. Actinobacteriota is a gram-positive bacterium, with high guanine-cytosine (GC) content, and one of the largest bacterial phyla. Together, Actinobacteria, Bacteroidetes, Firmicutes, Clostridium, and Proteobacteria dominate the gut microbiota (33). It has been reported that compared to healthy children, children with diabetes have a higher prevalence of Actinobacteriota, and Firmicutes is significantly reduced (34). In our study, the relative abundance of Fusobacterium was higher in GY than in JG and the difference was statistically significant, indicating that simultaneous administration of probiotics is more helpful in restoring a healthy gut than spaced use of probiotics. The results of the study by Suchodolsk showed that in inflammatory bowel disease (IBD) in dogs and cats, Fusobacterium was reduced, especially Clostridium clusters XIVa, and IV (i.e., Lachnospiraceae, Ruminococcaceae, Faecalibacterium spp.), indicating that these bacterial species are significant producers of short-chain fatty acids and might be involved in maintaining gut health (35).
At the genus level, the relative abundance of Ruminococcus was reduced in GY compared to the model group and the difference was statistically significant. The relative abundance of Ruminococcus was also reduced relatively in JG. Ruminococcus was one of the first stomach bacteria to be discovered; the Ruminococcus genus includes both beneficial and harmful bacteria, for example, Ruminococcus gnavus is considered a harmful bacterium, and Nishino et al. demonstrated that the abundance of Ruminococcus in patients with Crohn’s disease compared to patients with non-IBD was significantly increased (36). Crost et al. showed that elevated relative abundance of Ruminococcus gnavus correlated with symptom severity in patients with Crohn’s disease and found that Ruminococcus produce a pro-inflammatory polysaccharide, glucomannan, which can induce TNF-α secretion from dendritic cells in a toll-like receptor 4 (TLR4)-dependent manner (37), which, in combination with clinical manifestations and other results, led to the speculation that the GY may have had a higher reduction in harmful Ruminococcus bacteria.
The relative abundance of Lactobacillus was increased in both GY and JG compared to KB, with a greater increase in GY. It has been reported that, in intestinal microbiota research, Lactobacillus is the most prominent probiotic among the lactic acid bacteria (38). The probiotic Lactobacillus can adhere to the surface of host intestinal epithelial cells through the surface S-layer proteins, and competitively inhibit the invasion and colonization of intestinal pathogenic microorganisms (39), in addition to Lactobacillus inhibiting the pro-inflammatory cytokines (40). Lactobacilli are Gram-positive anaerobic or slightly aerobic rod-shaped bacteria. The relative abundance of Lachnoclostridium was reduced in both GY and JG compared to the control group, but the reduction was more prominent in GY, which was consistent with the study of Chen et al. (41), who showed that patients with ulcerative colitis had high levels of potentially pathogenic bacteria such as Lachnoclostridium detected in their feces and had less severe disease in remission. In contrast, Kang et al. showed that microbial diversity was reduced in fecal samples from patients with sarcopenia and probable sarcopenia, including Lachnoclostridium. Interestingly, the relative abundance was increased in irritable bowel lesions and colorectal tumors, and the relative increased abundance in liver steatosis and metabolic diseases (42); however, the relative abundance was decreased in autoimmune diseases (43); possible mechanisms need to be further investigated. The mean abundance of Akkermansia was increased in GY compared to KB. Chelakkot et al. demonstrated that Akkermansia muciniphila was more prevalent in the feces of individuals with ulcerative colitis and Crohn’s disease, compared to healthy individuals. Possible mechanisms need to be further investigated, which may be related to the extracellular vesicles of Akkermansia muciniphila (AmEVs) reducing intestinal permeability by regulating the tight junctions in mice (44).
At the species level, the relative abundance of Oscillibacter_sp_1-3 decreased in both GY and JG compared to the control group, with a more pronounced decrease in GY. Zhang et al. demonstrated that fasting blood glucose was reduced in the offspring of mice fed a diet rich in fat chow and given inulin, a probiotic, as compared to the progeny of mice fed high-fat chow alone. The area under the blood glucose curve of the glucose tolerance test was reduced, and it also improved intestinal dysbiosis. It was observed that the abundance of Oscillibacter_sp_1-3 in the cecum contents of the offspring of mice fed a high-fat diet and given inulin was significantly lower than that of the group fed a high-fat diet fed (45), which is in agreement with our findings. Little is known about the physiological effects of Oscillibacter_sp_1-3, yet it was observed that Oscillibacter_sp_1-3 was negatively correlated with proximal colonic barrier function score. This may be due to other gut microbial metabolites altering the abundance of Oscillibacter_sp_1-3 strains, or it is possible that Oscillibacter_sp_1-3 directly regulate the components that maintain the integrity of gut barrier function (46). The abundance of Lactobacillus johnsonii was increased in GY compared to KB and MX, and the difference was statistically significant. Lactobacillus johnsonii belongs to the Lactobacillus family. Its common mechanism of action as a potential anti-inflammatory bacterium in different diseases includes the adjustment of immunological response, the interaction with the intestinal flora, and the improvement of barrier function (47). Jia et al. showed that Lactobacillus johnsonii colonization was reduced in colitis mice, and they found that Lactobacillus johnsonii could relieve colitis through the TLR1/2-STAT3 pathway to promote the activation of interleukin 10 (IL-10) in CD206+ macrophages (48). Thus, Lactobacillus johnsonii may serve as an immunomodulating anti-inflammatory therapeutic target for colitis. The abundance of Phascolarctobacterium succinatutens was reduced in JG compared to GY, and the difference was statistically significant. Huang et al. showed that spraying a complex probiotic fermentation solution in the living environment of piglets changed the structure and diversity of the intestinal flora of the piglets and increased some of the abundance of beneficial bacteria, such as Phascolarctobacterium succinatutens (49).
We also observed relatively insignificant changes in functional genes compared to changes in intestinal microbiota, which is consistent with The Human Microbiome Project Consortium. The abundance of functional genes in gut microbes is more stable than the community structure (50). The application of probiotics also caused functional changes, with a decrease in cell motility in GY compared to KB. According to Xu et al. (51), cell motility was lower in fecal samples from diarrheic dogs in the probiotic-treated group compared to the control group, and these results suggest a relationship between cell motility and bacterial movement in liquid and its diffusion on the surface. Thus, increasing bacterial cell motility may increase bacterial virulence (52). Furthermore, we found that there was a negative correlation between cell motility and a few enhanced beneficial microorganisms, such as Acidaminocaccaceae and Phascolarctobacterium. Glycoside hydrolase expression was up-regulated in GY as compared to KB and the relative abundance of Prevotella was increased in JG. In the study by Aakko et al. (53), it was observed that exo-beta-(1,4)-xylanase, xylan-1,4-β-xylosidase, alph-1-arabinofuranosidase, and several other CAZy belonging to the glycosyl hydrolases family were associated with high abundance of Prevotella in the intestinal microbiota, whereas in subjects with low abundance of Prevotella among the microbiota, the population did not have Prevotella-derived CAZy, which is consistent with our findings. This suggests the possibility of determining the metabolic capacity of the microbiota at a certain level by macrogenomics CAZy. In addition, the application of probiotics also enhanced some microbial metabolic pathways, including amino acid and inorganic ion transporter metabolism, which may contribute to the production of more amino acids and inorganic ions for the maintenance of host intestinal homeostasis. The up-regulation of these pathways was positively correlated with beneficial bacteria such as Acidaminocaccaceae, and negatively correlated with potentially harmful bacteria such as the Spirochaetes, Fibrobacteres, and Cyanobacteria. These results suggest that the beneficial mechanism of probiotics may be related to the transport metabolism of amino acids and inorganic ions, as well as the enrichment of related genes that convert ingested dietary fiber and amino acids into host available nutrients (54). This can generate new amino acids, peptides, and secondary metabolites to combat diarrhea (55).
Of course, there are also shortcomings in this study, the most obvious of which is the relatively small sample size (10 rats per group), which may have led to the appearance of some false negatives or false positives. In this study, we used SD rats as test subjects to ensure homogeneity, but the same shortcoming exists, namely, the relatively low resolution of taxonomic mapping of the rat gut microbiota. Although a small number of species can be recognized in the rat gut microbiota, the lowest taxonomic unit of the rat gut microbiota with a high level of confidence is the genus (13). Few bacteria with reference genomes have been isolated from the rat gut and are available for public database access, and the rat gut microbiota is not well understood. Therefore, it is crucial to improve the availability of reference genomes of rat gut microorganisms to improve quasi-population classification in rat microbiome studies (56). The results of this study need to be confirmed by further clinical trials, which will require additional large-scale clinical trials using multi-omics and will need to consider inter-individual differences (dietary habits, age, gender, weight, and other physiological characteristics of the children).
In addition, the effect of probiotics has a significant dose-dependent effect (5). Wu et al. showed that the incidence of antibiotic-induced intestinal dysbiosis was significantly lower in the group of double-dose probiotic preparation compared to the group of conventional-dose probiotic preparation (57). Konstantis et al. found that a single probiotic in a low dose and a short course of treatment might be more effective in improving the symptoms and quality of life of irritable bowel syndrome more effectively (58), which warrants future studies on the quantitative-effectiveness relationship of probiotics.
Conclusions
The present study analyzed the effects of different time-linked probiotics on the intestinal microecology of rats with acute diarrhea based on metagenomics sequencing. The results showed that no significant changes were observed in microbial diversity after 7 days of simultaneous administration of probiotics; however, the composition and function of the intestinal microbiota underwent obvious changes, with an increase in the relative abundance of some beneficial species and a decrease in potentially harmful species, especially the alteration of the intestinal flora of the rats in GY. In addition, up-regulation of glycoside hydrolase expression and pathways related to the transport metabolism of amino acids and inorganic ions, and down-regulation of cell motility were also observed in GY. Although our previous studies have shown comparable clinical efficacy between simultaneous and spaced administration of probiotics (15), the present study, based on metagenomics sequencing analysis, found that the difference between simultaneous administration of probiotics was more significant than spaced administration of probiotics in terms of both intestinal flora and metabolic pathways. This theoretically suggests that GY should have had better clinical efficacy, but this was not the case and this calls for more in-depth basic and clinical studies. Continuous supplementation of probiotics may have a positive effect on improving intestinal homeostasis in rats with acute diarrhea. This study to some extent helps to elucidate the possible mechanisms by which probiotics can improve diarrhea.
Acknowledgments
This work was supported by Wuhan Igenebook Biotechnology Co., Ltd. and Yundi Biotechnology Co., Ltd.
Funding: None.
Footnote
Reporting Checklist: The authors have completed the ARRIVE reporting checklist. Available at https://tp.amegroups.com/article/view/10.21037/tp-24-129/rc
Data Sharing Statement: Available at https://tp.amegroups.com/article/view/10.21037/tp-24-129/dss
Peer Review File: Available at https://tp.amegroups.com/article/view/10.21037/tp-24-129/prf
Conflicts of Interest: All authors have completed the ICMJE uniform disclosure form (available at https://tp.amegroups.com/article/view/10.21037/tp-24-129/coif). All authors report receiving metagenomic next-generation sequencing technology from Wuhan Igenebook Biotechnology Co., Ltd. and Yundi Biotechnology Co., Ltd. The authors have no other conflicts of interest to declare.
Ethical Statement: The authors are accountable for all aspects of the work in ensuring that questions related to the accuracy or integrity of any part of the work are appropriately investigated and resolved. All animal procedures were performed in accordance with the Guidelines for Care and Use of Laboratory Animals of Hubei Disease Control and Prevention Center and approved by the Animal Ethics Committee of Hubei Disease Control and Prevention Center (No. 202420035).
Open Access Statement: This is an Open Access article distributed in accordance with the Creative Commons Attribution-NonCommercial-NoDerivs 4.0 International License (CC BY-NC-ND 4.0), which permits the non-commercial replication and distribution of the article with the strict proviso that no changes or edits are made and the original work is properly cited (including links to both the formal publication through the relevant DOI and the license). See: https://creativecommons.org/licenses/by-nc-nd/4.0/.
References
- Walker CLF, Rudan I, Liu L, et al. Global burden of childhood pneumonia and diarrhoea. Lancet 2013;381:1405-16. [Crossref] [PubMed]
- Black RE, Perin J, Yeung D, et al. Estimated global and regional causes of deaths from diarrhoea in children younger than 5 years during 2000-21: a systematic review and Bayesian multinomial analysis. Lancet Glob Health 2024;12:e919-28. [Crossref] [PubMed]
- Lai HH, Chiu CH, Kong MS, et al. Probiotic Lactobacillus casei: Effective for Managing Childhood Diarrhea by Altering Gut Microbiota and Attenuating Fecal Inflammatory Markers. Nutrients 2019;11:1150. [Crossref] [PubMed]
- Canani RB, Cirillo P, Terrin G, et al. Probiotics for treatment of acute diarrhoea in children: randomised clinical trial of five different preparations. BMJ 2007;335:340. [Crossref] [PubMed]
- Chen J, Wan CM, Gong ST, et al. Chinese clinical practice guidelines for acute infectious diarrhea in children. World J Pediatr 2018;14:429-36. [Crossref] [PubMed]
- Li Z, Zhu G, Li C, et al. Which Probiotic Is the Most Effective for Treating Acute Diarrhea in Children? A Bayesian Network Meta-Analysis of Randomized Controlled Trials. Nutrients 2021;13:4319. [Crossref] [PubMed]
- Cruchet S, Furnes R, Maruy A, et al. The use of probiotics in pediatric gastroenterology: a review of the literature and recommendations by Latin-American experts. Paediatr Drugs 2015;17:199-216. [Crossref] [PubMed]
- Fatima S, Altwaijry H, Abulmeaty MMA, et al. Combined Supplementation of Clostridium butyricum and Bifidobacterium infantis Diminishes Chronic Unpredictable Mild Stress-Induced Intestinal Alterations via Activation of Nrf-2 Signaling Pathway in Rats. Int J Mol Sci 2023;24:8264. [Crossref] [PubMed]
- Gopalan S, Ganapathy S, Mitra M, et al. Unique Properties of Yeast Probiotic Saccharomyces boulardii CNCM I-745: A Narrative Review. Cureus 2023;15:e46314. [Crossref] [PubMed]
- Middelbos IS, Vester Boler BM, Qu A, et al. Phylogenetic characterization of fecal microbial communities of dogs fed diets with or without supplemental dietary fiber using 454 pyrosequencing. PLoS One 2010;5:e9768. [Crossref] [PubMed]
- Cleary B, Brito IL, Huang K, et al. Detection of low-abundance bacterial strains in metagenomic datasets by eigengenome partitioning. Nat Biotechnol 2015;33:1053-60. [Crossref] [PubMed]
- Roy S, Dhaneshwar S. Role of prebiotics, probiotics, and synbiotics in management of inflammatory bowel disease: Current perspectives. World J Gastroenterol 2023;29:2078-100. [Crossref] [PubMed]
- He Q, Huang J, Zheng T, et al. Treatment with mixed probiotics induced, enhanced and diversified modulation of the gut microbiome of healthy rats. FEMS Microbiol Ecol 2021;97:fiab151. [Crossref] [PubMed]
- Tompkins TA, Mainville I, Arcand Y. The impact of meals on a probiotic during transit through a model of the human upper gastrointestinal tract. Benef Microbes 2011;2:295-303. [Crossref] [PubMed]
- Cui X. Effect comparison and mechanism study of different combination of probiotics on acute diarrhea. Henan University; 2023:52.
- Li S, Wang Y, Wu D, et al. Establishment and discussion of the method of preparing acute enteritis model in rats. Chinese Traditional Patent Medicine 2018;40:444-6.
- Li D, Liu CM, Luo R, et al. MEGAHIT: an ultra-fast single-node solution for large and complex metagenomics assembly via succinct de Bruijn graph. Bioinformatics 2015;31:1674-6. [Crossref] [PubMed]
- Li D, Luo R, Liu CM, et al. MEGAHIT v1.0: A fast and scalable metagenome assembler driven by advanced methodologies and community practices. Methods 2016;102:3-11. [Crossref] [PubMed]
- Li D, Liu CM, Luo R, et al. MEGAHIT: An ultra-fast single-node solution for large and complex metagenomics assembly via succinct de Bruijn graph. arXiv:1409.7208 [Preprint]. 2017. Available online: https://arxiv.org/abs/1702.01006
- Li J, Jia H, Cai X, et al. An integrated catalog of reference genes in the human gut microbiome. Nat Biotechnol 2014;32:834-41. [Crossref] [PubMed]
- Villar E, Farrant GK, Follows M, et al. Ocean plankton. Environmental characteristics of Agulhas rings affect interocean plankton transport. Science 2015;348:1261447. [Crossref] [PubMed]
- Nowicki M, Bzhalava D. BaŁa P. Massively Parallel Implementation of Sequence Alignment with Basic Local Alignment Search Tool Using Parallel Computing in Java Library. J Comput Biol 2018;25:871-81. [Crossref] [PubMed]
- Chen F, Dai X, Zhou CC, et al. Integrated analysis of the faecal metagenome and serum metabolome reveals the role of gut microbiome-associated metabolites in the detection of colorectal cancer and adenoma. Gut 2022;71:1315-25. [Crossref] [PubMed]
- Mercer EM, Ramay HR, Moossavi S, et al. Divergent maturational patterns of the infant bacterial and fungal gut microbiome in the first year of life are associated with inter-kingdom community dynamics and infant nutrition. Microbiome 2024;12:22. [Crossref] [PubMed]
- Qin J, Li Y, Cai Z, et al. A metagenome-wide association study of gut microbiota in type 2 diabetes. Nature 2012;490:55-60. [Crossref] [PubMed]
- Karlsson FH, Tremaroli V, Nookaew I, et al. Gut metagenome in European women with normal, impaired and diabetic glucose control. Nature 2013;498:99-103. [Crossref] [PubMed]
- Karlsson FH, Fåk F, Nookaew I, et al. Symptomatic atherosclerosis is associated with an altered gut metagenome. Nat Commun 2012;3:1245. [Crossref] [PubMed]
- Kanehisa M, Furumichi M, Tanabe M, et al. KEGG: new perspectives on genomes, pathways, diseases and drugs. Nucleic Acids Res 2017;45:D353-61. [Crossref] [PubMed]
- Powell S, Forslund K, Szklarczyk D, et al. eggNOG v4.0: nested orthology inference across 3686 organisms. Nucleic Acids Res 2014;42:D231-9. [Crossref] [PubMed]
- Cantarel BL, Coutinho PM, Rancurel C, et al. The Carbohydrate-Active EnZymes database (CAZy): an expert resource for Glycogenomics. Nucleic Acids Res 2009;37:D233-8. [Crossref] [PubMed]
- Szajewska H, Berni Canani R, Domellöf M, et al. Probiotics for the Management of Pediatric Gastrointestinal Disorders: Position Paper of the ESPGHAN Special Interest Group on Gut Microbiota and Modifications. J Pediatr Gastroenterol Nutr 2023;76:232-47. [Crossref] [PubMed]
- Redondo-Useros N, Gheorghe A, Díaz-Prieto LE, et al. Associations of Probiotic Fermented Milk (PFM) and Yogurt Consumption with Bifidobacterium and Lactobacillus Components of the Gut Microbiota in Healthy Adults. Nutrients 2019;11:651. [Crossref] [PubMed]
- Binda C, Lopetuso LR, Rizzatti G, et al. Actinobacteria: A relevant minority for the maintenance of gut homeostasis. Dig Liver Dis 2018;50:421-8. [Crossref] [PubMed]
- Mokhtari P, Metos J, Anandh Babu PV. Impact of type 1 diabetes on the composition and functional potential of gut microbiome in children and adolescents: possible mechanisms, current knowledge, and challenges. Gut Microbes 2021;13:1-18. [Crossref] [PubMed]
- Suchodolski JS. Companion animals symposium: microbes and gastrointestinal health of dogs and cats. J Anim Sci 2011;89:1520-30. [Crossref] [PubMed]
- Nishino K, Nishida A, Inoue R, et al. Analysis of endoscopic brush samples identified mucosa-associated dysbiosis in inflammatory bowel disease. J Gastroenterol 2018;53:95-106. [Crossref] [PubMed]
- Crost EH, Coletto E, Bell A, et al. Ruminococcus gnavus: friend or foe for human health. FEMS Microbiol Rev 2023;47:fuad014. [Crossref] [PubMed]
- Azad MAK, Sarker M, Li T, et al. Probiotic Species in the Modulation of Gut Microbiota: An Overview. Biomed Res Int 2018;2018:9478630. [Crossref] [PubMed]
- Zhang MM, Jiang N, Zhang AZ. Maintenance mechanism of intestinal barrier function integrity by intestinal microbes. Microbiology China 2020;47:933-40.
- Zhao Z, Chen L, Zhao Y, et al. Lactobacillus plantarum NA136 ameliorates nonalcoholic fatty liver disease by modulating gut microbiota, improving intestinal barrier integrity, and attenuating inflammation. Appl Microbiol Biotechnol 2020;104:5273-82. [Crossref] [PubMed]
- Chen W, Tan D, Yang Z, et al. Fermentation patterns of prebiotics fructooligosaccharides-SCFA esters inoculated with fecal microbiota from ulcerative colitis patients. Food Chem Toxicol 2023;180:114009. [Crossref] [PubMed]
- Kang L, Li P, Wang D, et al. Alterations in intestinal microbiota diversity, composition, and function in patients with sarcopenia. Sci Rep 2021;11:4628. [Crossref] [PubMed]
- Fan Y, Pedersen O. Gut microbiota in human metabolic health and disease. Nat Rev Microbiol 2021;19:55-71. [Crossref] [PubMed]
- Chelakkot C, Choi Y, Kim DK, et al. Akkermansia muciniphila-derived extracellular vesicles influence gut permeability through the regulation of tight junctions. Exp Mol Med 2018;50:e450. [Crossref] [PubMed]
- Zhang Q, Xiao X, Zheng J, et al. Improvement in glucose metabolism in adult male offspring of maternal mice fed diets supplemented with inulin via regulation of the hepatic long noncoding RNA profile. FASEB J 2021;35:e22003. [Crossref] [PubMed]
- Lam YY, Ha CW, Campbell CR, et al. Increased gut permeability and microbiota change associate with mesenteric fat inflammation and metabolic dysfunction in diet-induced obese mice. PLoS One 2012;7:e34233. [Crossref] [PubMed]
- Zhang Z, Zhao L, Wu J, et al. The Effects of Lactobacillus johnsonii on Diseases and Its Potential Applications. Microorganisms 2023;11:2580. [Crossref] [PubMed]
- Jia DJ, Wang QW, Hu YY, et al. Lactobacillus johnsonii alleviates colitis by TLR1/2-STAT3 mediated CD206(+) macrophages(IL-10) activation. Gut Microbes 2022;14:2145843. [Crossref] [PubMed]
- Huang W, Ma T, Liu Y, et al. Spraying compound probiotics improves growth performance and immunity and modulates gut microbiota and blood metabolites of suckling piglets. Sci China Life Sci 2023;66:1092-107. [Crossref] [PubMed]
- Human Microbiome Project Consortium. Structure, function and diversity of the healthy human microbiome. Nature 2012;486:207-14. [Crossref] [PubMed]
- Xu H, Zhao F, Hou Q, et al. Metagenomic analysis revealed beneficial effects of probiotics in improving the composition and function of the gut microbiota in dogs with diarrhoea. Food Funct 2019;10:2618-29. [Crossref] [PubMed]
- Josenhans C, Suerbaum S. The role of motility as a virulence factor in bacteria. Int J Med Microbiol 2002;291:605-14. [Crossref] [PubMed]
- Aakko J, Pietilä S, Toivonen R, et al. A carbohydrate-active enzyme (CAZy) profile links successful metabolic specialization of Prevotella to its abundance in gut microbiota. Sci Rep 2020;10:12411. [Crossref] [PubMed]
- Abdul Hakim BN, Xuan NJ, Oslan SNH. A Comprehensive Review of Bioactive Compounds from Lactic Acid Bacteria: Potential Functions as Functional Food in Dietetics and the Food Industry. Foods 2023;12:2850. [Crossref] [PubMed]
- Nealon NJ, Yuan L, Yang X, et al. Rice Bran and Probiotics Alter the Porcine Large Intestine and Serum Metabolomes for Protection against Human Rotavirus Diarrhea. Front Microbiol 2017;8:653. [Crossref] [PubMed]
- Pan H, Guo R, Zhu J, et al. A gene catalogue of the Sprague-Dawley rat gut metagenome. Gigascience 2018;7:giy055. [Crossref] [PubMed]
- Wu J, Gan T, Zhang Y, et al. The prophylactic effects of BIFICO on the antibiotic-induced gut dysbiosis and gut microbiota. Gut Pathog 2020;12:41. [Crossref] [PubMed]
- Konstantis G, Efstathiou S, Pourzitaki C, et al. Efficacy and safety of probiotics in the treatment of irritable bowel syndrome: A systematic review and meta-analysis of randomised clinical trials using ROME IV criteria. Clin Nutr 2023;42:800-9. [Crossref] [PubMed]