Metabolomics and lipidomics in pectus excavatum: preliminary screening of biomarkers for early diagnosis
Highlight box
Key findings
• This study revealed significant metabolic anomalies between patients with pectus excavatum (PE) and normal control groups through untargeted metabolomics and lipidomics analyses based on liquid chromatography-mass spectrometry (LC-MS). Furthermore, indole-3-acetaldehyde was identified as a potential biomarker for PE.
What is known and what is new?
• PE is the most common chest wall deformity, often accompanied by emaciation and distinctive metabolic characteristics. Previous studies have identified significant abnormalities in urinary hydroxyproline levels in PE patients.
• In our study, we employed a multiomics approach to comprehensively examine dysregulated serological molecules in PE patients. These findings will serve as crucial clues, provide an experimental foundation for understanding the onset and progression of PE, and suggest that indole-3-acetaldehyde can potentially be used as a biomarker for the diagnosis of early-stage PE.
What is the implication, and what should change now?
• The discovery of these biomarkers offers a foundation for early PE diagnosis and insights into its pathophysiology.
• Implement metabolomic and lipidomic screening in clinical practice for early detection and monitoring of PE, enabling timely intervention and improved patient outcomes.
• Furthermore, the identification of PE-related metabolic characteristics may offer potential insights for the future development of pharmacological treatments.
Introduction
Pectus excavatum (PE) is the most common chest wall deformity in children (1). The specific depression of the sternum compresses the thoracic cavity, resulting in varying degrees of cardiopulmonary dysfunction (2). Many children experience various symptoms such as upper respiratory tract infections and reduced exercise tolerance. Currently, the Nuss procedure is the primary treatment for PE (3), but it is typically reserved for severe cases and carries the risk of complications such as bleeding, infection, and even scoliosis. Therefore, early diagnosis and identification of high-risk patients can enable closer monitoring and timely interventions, which may contribute to preventing further progression of the deformity, ultimately improving clinical outcomes and quality of life.
PE patients are generally more emaciated than their peers, often suffering from malnutrition and growth retardation (4). Metabolic disorder is closely associated with many emaciation-related diseases, such as cancer, diabetes (5), and Huntington’s disease (HD) (6,7). In PE patients, emaciation often precedes the appearance of deformity. Our previous investigation revealed that, in accordance with the body mass index (BMI) criteria set forth by the World Health Organization (WHO), which spans from 18.50 to 24.99 kg/m2, the incidence of emaciation among PE patients was 93.61%. While BMI increased after Nuss correction, it still did not reach the lower threshold of the normal WHO reference range (8). Park et al. reported that the computed tomography (CT) index and mean BMI of 1,371 PE patients were 4.30±1.53 and 17.5 kg/m2, respectively, indicating that children with PE generally experience delayed nutrition and growth (9). Furthermore, Prozorovskaia et al. identified significant abnormalities in urine hydroxyproline levels in PE patients compared to healthy children of the same age (10). Therefore, we speculate that PE is not only a structural deformity but also more likely associated with overall metabolic disorders. Thus, clarifying the metabolic characteristics of PE is highly beneficial for gaining insight into its pathophysiological mechanisms, which may aid in understanding the progression of the disease and even provide a foundation for future research on new therapeutic targets.
The diagnosis of PE depends on physical examination and imaging studies, specifically CT scans. However, these methods lag behind disease progression and have limited utility for the early diagnosis. Instead, changes in the microenvironment occur earlier than structural deformities. Metabolomics techniques can quantitatively measure the dynamic metabolic profiles of living organisms under specific pathophysiological conditions and detect numerous small molecule metabolites (11,12). This facilitates early diagnosis of diseases and exploration of new biomarkers.
Herein, we aim to investigate the metabolic characteristics of PE patients using liquid chromatography-mass spectrometry (LC-MS)-based untargeted metabolomics and lipidomics analyses, with the goal of identifying potential biomarkers for early diagnosis. By elucidating these metabolic profiles, we seek to enhance the assessment of disease severity and potential progression, thereby facilitating the selection of appropriate clinical strategies. Furthermore, the identification of metabolic features associated with PE may offer new perspectives for the future development of therapeutic interventions. We present this article in accordance with the STARD reporting checklist (available at https://tp.amegroups.com/article/view/10.21037/tp-24-288/rc).
Methods
Patients and setting
Participants were recruited from Guangzhou Women and Children’s Medical Center (located in Guangzhou, Guangdong Province, China) between November 2017 and September 2018. This study was approved by the institutional review board of Guangzhou Women and Children’s Medical Center (No. 2017103001) and was registered at the Clinical Trial Registry (ChiCTR-ROC-17013308). Written informed consent was obtained from the patients’ parents and the study was conducted in accordance with the Declaration of Helsinki (as revised in 2013). A total of 100 children were recruited in this study, including 50 patients diagnosed with PE from the Department of Thoracic Surgery and 50 non-PE healthy controls from the Department of Child Healthcare. The exclusion criterion for the PE group were: (I) patients with congenital heart disease; (II) any syndromes associated with PE (e.g., Marfan syndrome); (III) other skeletal deformities such as scoliosis, pectus carinatum, or clubfoot; (IV) metabolic-related diseases (e.g., diabetes mellitus); and (V) conditions associated with emaciation, such as gastrointestinal disease. In the PE group, 8 patients withdrew from the study for personal reasons, 12 patients had unavailable serum samples, and 10 patients met the exclusion criteria, resulting in a final inclusion of 20 PE patients. The healthy control group was matched with the PE group based on age, gender, and BMI, resulting in the inclusion of 30 non-PE children (Figure 1).
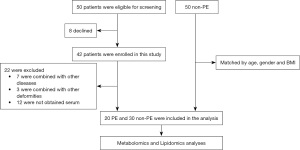
Sample preparation for metabolomics and lipidomics
For untargeted metabolomic and lipidomic analysis, the extraction of metabolites and lipids from serum samples followed established protocols (13); 100 µL of serum sample was combined with 400 µL of a chloroform/methanol mixture (2:1, V/V) inside a 1.5-mL centrifuge tube. Following 15 min of vortexing and shaking, the mixture was subjected to centrifugation at 12,000 rpm for 20 min at 4 ℃. The upper aqueous layer containing hydrophilic metabolites and the lower organic layer containing hydrophobic metabolites were individually collected and evaporated under vacuum at room temperature. The evaporated samples were all preserved at −80 ℃ until high-performance liquid chromatography (HPLC)-mass spectrometry (MS) based lipidomic analysis was performed. All samples were processed in the same laboratory to avoid bias.
HPLC for metabolomics
Metabolomics and lipidomics were performed on an Ultimate 3000 UHPLC system (Thermo Fisher Scientific, Milan, Italy) coupled with Q Exactive MS system (Thermo Fisher Scientific). For metabolomics analysis of the aqueous phase, a 100×2.1 mm XBridge amide column (3.5 µm; Waters, Milford, MA, USA) was used to separate compounds at 30 ℃. The samples were dissolved in 100 µL of water, with an injection volume of 10 µL. Mobile phase A was composed of 5 mM ammonium acetate in water which containing 5% acetonitrile, while mobile phase B was pure acetonitrile. The flow rate was set to 0.4 mL/min with the following linear gradient: starting at 95% B at 0 min, decreasing to 90% B at 3 min, 50% B at 13 and 14 min, returning to 95% B at 15 min, and maintaining 95% B until 17 min.
For lipidomic analysis, chromatographic separation was carried out using a reversed-phase X-select CSH C18 column (4.6 mm × 100 mm, 2.5 µm, Waters) at 40 ℃. A gradient elution was applied with two solvents, both containing 10 mM ammonium acetate and 0.1% formic acid: solvent A was ACN/water (3:2, V/V), and solvent B was IPA/ACN (9:1, V/V). The linear gradient was as follows: 0 min with 40% B, 2 min with 43% B, 2.1 min with 50% B, 10 min with 60% B, 10.1 min with 75% B, 16 min with 99% B, held with 99% B until 17 min, then dropped with 40% B at 18 min and held with 40% B until 19 min. The column temperature was kept at 50 ℃, with a flow rate of 0.6 mL/min. Samples were initially dissolved in 100 µL of a chloroform:methanol (1:1, V/V) solution and further diluted threefold with an isopropanol:acetonitrile:H2O (2:1:1, V/V/V) mixture. The injected sample volume was 10 µL.
MS for metabolomics
Untargeted lipidomics analysis was conducted utilizing a Q Exactive mass spectrometer fitted with a heated electrospray ionization (HESI) source, operating in electrospray ionization (ESI) mode. Data from the MS were acquired in a data-dependent acquisition mode, focusing on the top 10 most intense ions per scan. A full-scan MS spectrum was acquired with a resolution (R) of 35,000 with m/z ranging from 190 to 1,200. Maximum injection time was 80 ms. Automatic gain control (AGC) target was 5e6. MS/MS fragmentation utilized high-energy c-trap dissociation (HCD) was set as 17,500, with a maximum injection time of 70 ms and an AGC target of 1e5. Stepped normalized collision energies were set as 15, 30, and 45. Dynamic exclusion was configured for 8 seconds. External mass calibration was performed before each sequence run. The spray voltage was maintained at 3.3 kV for positive-ion mode and 3 kV for negative-ion mode, separately. The interface settings for both positive-ion and negative-ion mode analyses were kept consistent. The capillary temperature was set to 320 ℃, the sheath gas flow was set to 40 ℃, the auxiliary gas flow was set to 10 ℃, and the probe heater temperature was set to 300 ℃. A pooled serum sample was used for quality control (QC) to evaluate the stability of the HPLC-MS system and ensure data reliability. QC samples were injected and analyzed before and after the sequence, as well as after every 15 samples during the run.
DDA-MS data analysis for metabolomics
The raw data generated from data-dependent acquisition MS were processed using MS-DIAL software (https://systemsomicslab.github.io/compms/msdial/main.html) following the instructions provided in the user guide. In summary, the raw MS data were converted to the Reifycs Inc. format (.abf) using the Reifycs ABF converter (http://www.reifycs.com/AbfConverter/index.html). After conversion, MS-DIAL software was applied for feature detection, spectral deconvolution, metabolite identification, and peak alignment across samples. Metabolite identification was performed by searching the acquired MS/MS spectra against the MassBank database integrated into MS-DIAL, which contains MS1 and MS/MS data for metabolites. Lipid identification used the MS-DIAL internal in silico MS/MS spectra database (version: Lipid-DBs-VS23-FiehnO), which includes MS1 and MS/MS data for common lipid species. The tolerance levels for MS1 and MS/MS were set at 0.01 and 0.05 Da, respectively. Other MS-DIAL parameters were left at their default settings.
Statistical analysis
Participant characteristics were analyzed using IBM SPSS Statistics software (version 25). Continuous variables were presented as mean ± standard deviation, with normality assessed by the Shapiro-Wilk test. For normally distributed data, comparisons between the PE and non-PE groups were performed using the Student’s t-test, while the Wilcoxon rank-sum test was applied for non-normally distributed data, considering a P value of less than 0.05 as statistically significant. Principal component analysis (PCA) and partial least squares discriminant analysis (PLS-DA) were used to assess the quality of metabolic profiling and to identify metabolic differences between the PE and non-PE groups. Metabolites that were significantly altered, with variable importance in the projection (VIP) values greater than 1.5 in the PLS-DA analysis, along with P values less than 0.05 from Student’s t-test, were chosen for comparison between the groups. Analyses for metabolomic and lipidomic data such as PCA, PLS-DA, pathway enrichment analysis, and hierarchical clustering were conducted using MetaboAnalyst 5.0 (https://www.metaboanalyst.ca/). Additionally, the database of Kyoto Encyclopedia of Genes and Genomes (KEGG) was used to explore the pathways related to the metabolites altered in PE group.
Results
Participant characteristics
In this study, serum samples were obtained from 20 patients diagnosed with PE, alongside 30 samples collected from healthy control individuals. The sternum of all PE patients, particularly the sternal body and xiphoid process, showed a marked posterior depression. Clinical indices of participants, including age, sex, weight, height, BMI, and Haller CT index, were examined. We used a Haller index value of 2.5 from normal individuals as a reference for our control group. Detailed information on the PE and non-PE patients is listed in Table 1. The participants in the PE group exhibited higher Haller CT index levels (P<0.001). Moreover, there were no differences in age, sex, weight, height, or BMI (P>0.05).
Table 1
Characteristics | PE group (n=20) | Non-PE group (n=30) | P value |
---|---|---|---|
Age (years), n (%) | 0.78 | ||
<3 | 1 (5.0) | 5 (16.7) | |
3–5 | 12 (60.0) | 9 (30.0) | |
>5 | 7 (35.0) | 16 (53.3) | |
Male, n (%) | 16 (80.0) | 21 (70.0) | 0.16 |
Weight (kg), mean ± SD | 20.68±10.50 | 22.52±11.16 | 0.66 |
Height (cm), mean ± SD | 114.65±21.82 | 117.59±20.28 | 0.57 |
BMI (kg/m2), mean ± SD | 15.16±1.93 | 15.46±2.38 | 0.90 |
Haller CT index, mean ± SD | 5.41±3.07 | 2.5 | <0.001 |
PE, pectus excavatum; SD, standard deviation; BMI, body mass index; CT, computed tomography.
Untargeted metabolic profiling of PE patient serum
To explore the difference in metabolic profiles between PE and non-PE patients, we used LC-MS to determine that a battery of metabolites, such as 2-oxobutyric acid, hydroxymethylglutaric acid, threonine, indole-3-acetaldehyde and phytosphingosine, was useful in distinguishing PE patients and non-PE patients. The PE and non-PE groups were classified successfully in the PLS-DA model (Figure 2). The pooled QC samples showed clear clustering in the PCA score plots. This observation presented convincing stability within the analytical system (Figure 2A,2B). Metabolomics analysis of the serum samples revealed significant alterations in metabolite profiles between the PE and non-PE groups. The score plot of the PLS-DA demonstrated clear separation between the metabolite profiles of the PE group and the non-PE group (Figure 2C,2D). Following peak alignment and the exclusion of missing values, 139 metabolites in positive-ion mode and 144 metabolites in negative-ion mode were identified through MS/MS spectral comparison. Subsequent statistical analysis uncovered 14 significantly dysregulated metabolites between the PE and non-PE groups [VIP >1.5, fold change (FC) >2, P value <0.05, Table 2]. We found that the FCs of 2-oxobutyric acid, hydroxymethylglutaric acid and threonine were larger. Moreover, the FCs of indole-3-acetaldehyde and phytosphingosine were lower. These findings suggest that these metabolites differ markedly between PE patients and non-PE patients.
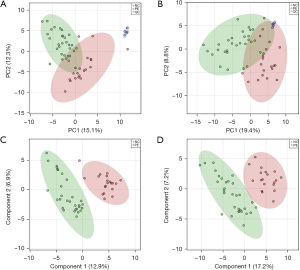
Table 2
Metabolites | VIP | P value | FC (P/N) |
---|---|---|---|
2-oxobutyric acid | 1.8309 | <0.001 | 5.3907 |
Hydroxymethylglutaric acid | 2.0003 | <0.001 | 2.9329 |
Threonine | 1.5508 | 0.001 | 2.9233 |
1,3-dimethylurate | 1.5252 | 0.001 | 2.2817 |
Glycocholic acid | 1.6304 | 0.002 | 2.1651 |
LPC 16:0 | 1.6892 | 0.002 | 2.0522 |
LPC 18:1 | 1.8833 | <0.001 | 2.0366 |
Dehydroascorbic acid | 1.5877 | <0.001 | 2.0062 |
4-pyridoxic acid | 1.7021 | <0.001 | 0.4948 |
Eicosenoic acid | 1.5447 | 0.001 | 0.4745 |
9-trans-palmitelaidic acid | 1.5367 | 0.001 | 0.4718 |
Docosahexanoic acid | 1.8964 | <0.001 | 0.4176 |
Indole-3-acetaldehyde | 2.0403 | <0.001 | 0.3827 |
Phytosphingosine | 1.6782 | 0.002 | 0.2930 |
PE, pectus excavatum; VIP, variable importance in the projection; FC, fold change; P, pectus excavatum; N, non-pectus excavatum; LPC, lysophosphatidylcholine.
Untargeted lipidomic profiling of PE patient serum
Similar to the analysis processing of metabolomic data, PCA and PLS-DA were applied to assess the expression patterns of lipids across all samples (Figure 3). The lipid QC samples displayed tight clustering in the PCA score plots in both positive- (Figure 3A) and negative-ion (Figure 3B) modes. A total of 743 lipid features in positive-ion mode and 251 in negative-ion mode were reliably identified through MS/MS spectral comparison. Thirteen lipids were found to be significantly dysregulated between the PE and non-PE groups (VIP >1.5, FC >2, P value <0.05). The seven most significantly dysregulated lipids in PE patients, ranked by VIP score, are presented in Table 3. The molecules that were significantly different between PE patients and non-PE controls were PE 36:3|PE 18:0_18:3, PE 36:4, TG 58:4|TG 22:0_18:2_18:2, TG 60:4|TG 24:0_18:2_18:2, PE 34:2, TG 54:0|TG 18:0_18:0_18:0 and SM 30:0;2O.
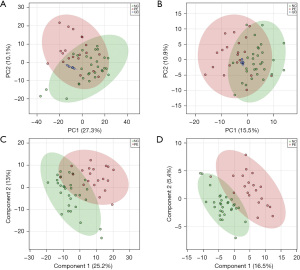
Table 3
Metabolites | VIP | P value | FC (P/N) |
---|---|---|---|
PE 36:3|PE 18:0_18:3 | 1.7578 | 0.003 | 5.5630 |
PE 36:4 | 1.8377 | 0.002 | 5.5193 |
TG 58:4|TG 22:0_18:2_18:2 | 1.6743 | 0.005 | 2.8599 |
TG 60:4|TG 24:0_18:2_18:2 | 1.5338 | 0.01 | 2.2471 |
PE 34:2 | 2.0365 | <0.001 | 2.1164 |
TG 54:0|TG 18:0_18:0_18:0 | 1.9477 | <0.001 | 2.0660 |
SM 30:0;2O | 1.6059 | 0.007 | 0.4559 |
PE, pectus excavatum; VIP, variable importance in the projection; FC, fold change; P, pectus excavatum; N, non-pectus excavatum; PE, phosphatidylethanolamine; TG, triacylglycerol; SM, sphingomyelin.
Metabolites associated with potential biomarkers for PE
To further identify the most potential metabolite molecules as biomarkers for PE, we performed receiver operating characteristic (ROC) analysis, which indicated that 9 metabolites and 4 lipids showed an area under the curve (AUC) exceeding 0.800 (Table 4). Indole-3-acetaldehyde exhibited the highest AUC value of 0.9400, indicating its potential as a biomarker for PE (Figure 4).
Table 4
Biomarkers | AUC (95% CI) | P value | SE |
---|---|---|---|
Indole-3-acetaldehyde | 0.9400 (0.8798 to 1.000) | <0.001 | 0.0307 |
2-oxobutyric acid | 0.9283 (0.8380 to 1.000) | <0.001 | 0.0461 |
PC 40:10 | 0.8867 (0.7961 to 0.9772) | <0.001 | 0.0462 |
Docosahexanoic acid | 0.8833 (0.7887 to 0.9780) | <0.001 | 0.0483 |
TG 27:0|TG 8:0_9:0_10:0 | 0.8767 (0.7836 to 0.9697) | <0.001 | 0.0475 |
Hydroxymethylglutaric acid | 0.8767 (0.7551 to 0.9982) | <0.001 | 0.0620 |
4-pyridoxic acid | 0.8667 (0.7467 to 0.9867) | <0.001 | 0.0612 |
Phytosphingosine | 0.8600 (0.7586 to 0.9614) | <0.001 | 0.0518 |
PE 36:4 | 0.8474 (0.7293 to 0.9654) | <0.001 | 0.0602 |
Dehydroascorbic acid | 0.8283 (0.7118 to 0.9448) | <0.001 | 0.0595 |
2-(hydroxymethyl)-4(3H)-quinazolinone | 0.8233 (0.7019 to 0.9448) | <0.001 | 0.0620 |
Eicosenoic acid | 0.8133 (0.6862 to 0.9404) | <0.001 | 0.0649 |
TG 59:2|TG 16:0_25:0_18:2 | 0.8100 (0.6892 to 0.9308) | <0.001 | 0.0616 |
AUC, area under the curve; ROC, receiver operating characteristic; CI, confidence interval; SE, standard error; PC, phosphatidylcholine; TG, triacylglycerol; PE, phosphatidylethanolamine.
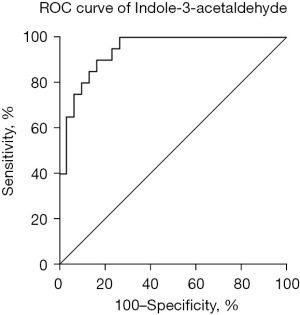
Perturbed metabolic pathways identified in PE patients
Additional bioinformatic analyses were conducted to identify disrupted pathways and offer insights into the underlying pathological mechanisms of PE. The 14 altered metabolites and 7 dysregulated lipids were cross-referenced with the pathway databases of KEGG. The top four metabolomic and lipidomic related pathways according to pathway impact and P value of <0.05 that were found to be significantly perturbed in PE patients (Figure 5) were valine, leucine, and isoleucine biosynthesis (2 hits; threonine, 2-oxobutanoate); sphingolipid metabolism (2 hits; sphingomyelin, phytosphingosine); glycine, serine, and threonine metabolism (2 hits; threonine, 2-oxobutanoate); and glycerophospholipid metabolism (2 hits; phosphatidylethanolamine, 1-acyl-sn-glycero-3-phosphocholine).
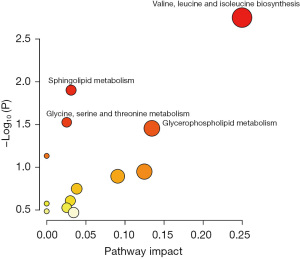
Discussion
In this study, we primarily employed LC-MS as the detection technique, successfully applying the PLS-DA model to distinguish between the PE and non-PE groups. Consequently, we identified a total of 14 dysregulated metabolites and 13 dysregulated lipids between these groups. Subsequently, ROC analysis indicated that indole-3-acetaldehyde exhibited the largest AUC value of 0.9400, suggesting its potential as a biomarker for PE. Furthermore, we conducted searches against KEGG pathway databases using the 14 dysregulated metabolites and the top 7 dysregulated lipids in PE patients. The findings of this study revealed that the top four metabolic pathways were valine, leucine, and isoleucine biosynthesis; sphingolipid metabolism; glycine, serine, and threonine metabolism; and glycerophospholipid metabolism. This study comprises the first metabolomics analysis of the serum of PE patients.
The metabolic profile of PE patients exhibits significantly elevated levels of 2-oxobutyric acid, threonine, and hydroxymethylglutaric acid and significantly decreased levels of indole-3-acetaldehyde. 2-oxobutanoic acid is a product of the enzymatic cleavage of cystathionine and one of the degradation products of threonine, which is involved in the metabolism of many amino acids as well as propanoate metabolites (14). However, its role in PE disease is unclear, and its abnormality may be related to the increased threonine content. Threonine is an essential amino acid involved in lipid metabolism, protein synthesis, the regulation of the intestinal microbiota, the immune response, and other processes (15). Study has noted that threonine generates glycine and serine, which are necessary for the production of collagen, elastin, and muscle tissue in the body (16). Thus, threonine, glycine, and serine play an important role in keeping connective tissues and muscles strong and resilient throughout the growth and development process (17). The insoluble collagen ratio and endogenous collagen dissolution rate of costal cartilage collagen is lower in PE patients than in normal controls (18). Unsurprisingly, we revealed that PE involves the glycine, serine, and threonine metabolism pathway, which directly or indirectly promotes the dysplasia of costal cartilage and the formation of sternal depression. Moreover, the results of the present study have also shown an increased level of hydroxymethylglutaric acid. Previous studies revealed that this metabolite is mainly involved in cholesterol metabolism, which can strongly reduce cholesterol biosynthesis in vivo and in vitro and promote lipid and protein oxidative impairment in the rat cerebral cortex (19,20). However, there is no direct evidence elucidating the abnormal cholesterol metabolism in patients with PE.
Our research has also revealed that the content of indole-3-acetaldehyde, a metabolite of tryptophan, was significantly decreased in PE patients compared with in non-PE subjects. Indole metabolites are the main products produced by tryptophan in the intestinal bacterial pathway (21). The metabolites produced by the bacterial pathway of tryptophan can regulate the differentiation and function of immune-related cells in the body and can affect the occurrence and development of many diseases (22,23). For example, methylindole has been identified as a pneumococcal toxin in ruminants, leading to fatal syndromes such as severe pulmonary edema and emphysema (24). Moreover, a ROC analysis showed that indole-3-acetaldehyde exhibited the largest AUC value, which suggests its potential as a biomarker for PE. Thus far, the pathogenic mechanism of indole-3-acetaldehyde in PE-related diseases has not been reported, which indicates that it may warrant further investigation. Furthermore, these data appear to hint that altering the gut microbiota may benefit PE patients.
Branched-chain amino acids (BCAAs), including valine, leucine, and isoleucine, play an important physiological role in glycolipid metabolism, protein synthesis, intestinal health, and immunity (25,26). The catabolic balance of BCAAs is closely associated with health and disease, and BCAAs and their derivatives have been reported as potential biomarkers for diseases such as type 2 diabetes, cancer, and cardiovascular disease (27,28). The mechanism of BCAAs in PE has not been reported. It has been established that BCAA deficiency inhibits the growth of cells due to protein metabolism (29). PE patients tend to be very asthenic, with long limbs, delicate bone structure and poor muscular development (30). Therefore, we speculate that abnormalities in the valine, leucine and isoleucine biosynthesis pathways are the potential cause of the lean body appearance in most PE patients.
In addition, we found abnormalities in another pathway, that is, sphingolipid metabolism. Sphingolipids are mainly located in the cell membrane, plasma compartment and lipoproteins, which are one of the main types of eukaryotic lipids (31,32). It has been reported that sphingolipids and their metabolite profiles change in patients with cardiovascular, renal, and metabolic disease, regulating cellular processes such as differentiation, proliferation, growth, senescence, and apoptosis (33,34). Sphingolipid metabolism also affects several important processes in skeletal muscle, from differentiation, regeneration, fatigue, and contraction to the insulin response, which is quite complex and depends on a variety of enzymes and signaling pathways (35). Even though the pathogenicity of sphingolipids and their metabolites in PE diseases has not been researched, these metabolites may be involved in muscular dysplasia in PE patients.
There are some limitations in this study. First, power calculations were not performed. We recruited 20 PE patients and 30 non-PE patients for our metabolomic and lipidomic analyses. Thus, further validation analysis needs to be performed in larger cohorts in future studies. Second, this work could be improved by performing multiple reaction monitoring-mass spectrometry (MRM-MS) validation on some of the metabolites and lipids between PE and non-PE patients.
Conclusions
It is highly important to explore the etiological mechanism of PE at the metabolic level, and our results revealed that there are significant differences in the serum metabolic profiles between PE patients and non-PE patients. Currently, research on the metabolism of PE is limited to sporadic international reports, with no studies conducted domestically. While our findings are preliminary, they provide a critical experimental foundation for future research. Notably, indole-3-acetaldehyde has emerged as a potential biomarker for the early diagnosis of PE, which could aid in the timely identification of high-risk patients and the development of targeted interventions. Further studies, particularly those incorporating larger and more diverse populations as well as longitudinal designs, are necessary to validate these findings and to explore the potential of these biomarkers in predicting disease progression and informing clinical decisions.
Acknowledgments
We thank Rf. Pang, Yy. Yuan, J. Zhou, H. Song, and J. Liu for their technical assistance and critical discussions and the Department of Thoracic Surgery (2017–2018) and Clinical Biological Resource Bank of Guangzhou Women and Children’s Medical Center for providing all the clinical samples.
Funding: This work was supported by grants from
Footnote
Reporting Checklist: The authors have completed the STARD reporting checklist. Available at https://tp.amegroups.com/article/view/10.21037/tp-24-288/rc
Data Sharing Statement: Available at https://tp.amegroups.com/article/view/10.21037/tp-24-288/dss
Peer Review File: Available at https://tp.amegroups.com/article/view/10.21037/tp-24-288/prf
Conflicts of Interest: All authors have completed the ICMJE uniform disclosure form (available at https://tp.amegroups.com/article/view/10.21037/tp-24-288/coif). The authors have no conflicts of interest to declare.
Ethical Statement: The authors are accountable for all aspects of the work in ensuring that questions related to the accuracy or integrity of any part of the work are appropriately investigated and resolved. The study was conducted in accordance with the Declaration of Helsinki (as revised in 2013). This retrospective study was approved by the institutional review board of Guangzhou Women and Children’s Medical Center (No. 2017103001). Written informed consent was obtained from the patients’ parents.
Open Access Statement: This is an Open Access article distributed in accordance with the Creative Commons Attribution-NonCommercial-NoDerivs 4.0 International License (CC BY-NC-ND 4.0), which permits the non-commercial replication and distribution of the article with the strict proviso that no changes or edits are made and the original work is properly cited (including links to both the formal publication through the relevant DOI and the license). See: https://creativecommons.org/licenses/by-nc-nd/4.0/.
References
- Chung CS, Myrianthopoulos NC. Factors affecting risks of congenital malformations. I. Analysis of epidemiologic factors in congenital malformations. Report from the Collaborative Perinatal Project. Birth Defects Orig Artic Ser 1975;11:1-22.
- de Loos ER, Höppener PF, Busari JO, et al. Pectus excavatum: not just a cosmetic problem. Ned Tijdschr Geneeskd 2020;164:D4509.
- Velazco CS, Arsanjani R, Jaroszewski DE. Nuss procedure in the adult population for correction of pectus excavatum. Semin Pediatr Surg 2018;27:161-9. [Crossref] [PubMed]
- Shi CR, Jin XQ, Li ZZ. Pediatric surgery. 4th edition. Beijing: People’s Medical Publishing House; 2009.
- Cheng Y, Sibusiso L, Hou L, et al. Sargassum fusiforme fucoidan modifies the gut microbiota during alleviation of streptozotocin-induced hyperglycemia in mice. Int J Biol Macromol 2019;131:1162-70. [Crossref] [PubMed]
- Lakra P, Aditi K, Agrawal N. Peripheral Expression of Mutant Huntingtin is a Critical Determinant of Weight Loss and Metabolic Disturbances in Huntington's Disease. Sci Rep 2019;9:10127. [Crossref] [PubMed]
- Muraleedharan R, Dasgupta B. AMPK in the brain: its roles in glucose and neural metabolism. FEBS J 2022;289:2247-62. [Crossref] [PubMed]
- Wang H, Liu W, Su ZH, et al. Screening and influencing factors analysis for nutritional status in hospitalized children with pectus excavatum. Chin J ApplClin Pediatr 2019;34:1415-7. [In Chinese]. Available online: https://rs.yiigle.com/cmaid/1163881
- Park HJ, Kim JJ, Park JK, et al. A cross-sectional study for the development of growth of patients with pectus excavatum. Eur J Cardiothorac Surg 2016;50:1102-9. [Crossref] [PubMed]
- Prozorovskaia NN, Glinianaia SV, Del'vig AA, et al. Urinary excretion of hydroxyproline in funnel chest deformity. Vopr Med Khim 1987;33:59-62.
- Kim HK, Choi YH, Verpoorte R. NMR-based metabolomic analysis of plants. Nat Protoc 2010;5:536-49. [Crossref] [PubMed]
- Sévin DC, Kuehne A, Zamboni N, et al. Biological insights through nontargeted metabolomics. Curr Opin Biotechnol 2015;34:1-8. [Crossref] [PubMed]
- Zhou J, Li Y, Chen X, et al. Development of data-independent acquisition workflows for metabolomic analysis on a quadrupole-orbitrap platform. Talanta 2017;164:128-36.
- Kobayashi S, Lee J, Takao T, et al. Increased ophthalmic acid production is supported by amino acid catabolism under fasting conditions in mice. Biochem Biophys Res Commun 2017;491:649-55. [Crossref] [PubMed]
- Tang Q, Tan P, Ma N, et al. Physiological Functions of Threonine in Animals: Beyond Nutrition Metabolism. Nutrients 2021;13:2592. [Crossref] [PubMed]
- Adeva-Andany M, Souto-Adeva G, Ameneiros-Rodríguez E, et al. Insulin resistance and glycine metabolism in humans. Amino Acids 2018;50:11-27. [Crossref] [PubMed]
- Razak MA, Begum PS, Viswanath B, et al. Multifarious Beneficial Effect of Nonessential Amino Acid, Glycine: A Review. Oxid Med Cell Longev 2017;2017:1716701. [Crossref] [PubMed]
- Prozorovskaya NN, Kozlov EA, Voronov AV, et al. Characterization of costal cartilage collagen in funnel chest. Biomed Sci 1991;2:576-80.
- Di Padova C, Bosisio E, Cighetti G, et al. 3-Hydroxy-3-methylglutaric acid (HMGA) reduces dietary cholesterol induction of saturated bile in hamster. Life Sci 1982;30:1907-14. [Crossref] [PubMed]
- Leipnitz G, Seminotti B, Haubrich J, et al. Evidence that 3-hydroxy-3-methylglutaric acid promotes lipid and protein oxidative damage and reduces the nonenzymatic antioxidant defenses in rat cerebral cortex. J Neurosci Res 2008;86:683-93. [Crossref] [PubMed]
- Yokoyama MT, Carlson JR. Microbial metabolites of tryptophan in the intestinal tract with special reference to skatole. Am J Clin Nutr 1979;32:173-8. [Crossref] [PubMed]
- Su X, Gao Y, Yang R. Gut Microbiota-Derived Tryptophan Metabolites Maintain Gut and Systemic Homeostasis. Cells 2022;11:2296. [Crossref] [PubMed]
- Chung KT, Fulk GE, Slein MW. Tryptophanase of fecal flora as a possible factor in the etiology of colon cancer. J Natl Cancer Inst 1975;54:1073-8. [Crossref] [PubMed]
- Yost GS. Mechanisms of 3-methylindole pneumotoxicity. Chem Res Toxicol 1989;2:273-9. [Crossref] [PubMed]
- Nie C, He T, Zhang W, et al. Branched Chain Amino Acids: Beyond Nutrition Metabolism. Int J Mol Sci 2018;19:954. [Crossref] [PubMed]
- Le Couteur DG, Solon-Biet SM, Cogger VC, et al. Branched chain amino acids, aging and age-related health. Ageing Res Rev 2020;64:101198. [Crossref] [PubMed]
- Holeček M. Branched-chain amino acids in health and disease: metabolism, alterations in blood plasma, and as supplements. Nutr Metab (Lond) 2018;15:33. [Crossref] [PubMed]
- Neinast M, Murashige D, Arany Z. Branched Chain Amino Acids. Annu Rev Physiol 2019;81:139-64. [Crossref] [PubMed]
- Holeček M. The role of skeletal muscle in the pathogenesis of altered concentrations of branched-chain amino acids (valine, leucine, and isoleucine) in liver cirrhosis, diabetes, and other diseases. Physiol Res 2021;70:293-305. [Crossref] [PubMed]
- Puri P, Höllwarth ME. Pediatric Surgery: Diagnosis and Management. Springer; 2009:252.
- Hannun YA, Obeid LM. Sphingolipids and their metabolism in physiology and disease. Nat Rev Mol Cell Biol 2018;19:175-91. [Crossref] [PubMed]
- Calzada C, Vors C, Penhoat A, et al. Role of circulating sphingolipids in lipid metabolism: Why dietary lipids matter. Front Nutr 2023;9:1108098. [Crossref] [PubMed]
- Hernández-Bello F, Franco M, Pérez-Méndez Ó, et al. Sphingolipid metabolism and its relationship with cardiovascular, renal and metabolic diseases. Arch Cardiol Mex 2023;93:88-95. [Crossref] [PubMed]
- Shayman JA. Sphingolipids. Kidney Int 2000;58:11-26. [Crossref] [PubMed]
- Jiang XC, Li Z. Sphingolipids and Cholesterol. Adv Exp Med Biol 2022;1372:1-14. [Crossref] [PubMed]