Synergistic machine learning models utilizing ferroptosis-related genes for improved neuroblastoma outcome prediction
Highlight box
Key findings
• This study identified ferroptosis-related genes (FRGs) as critical regulators in neuroblastoma (NB), with MYCN and RRM2 being central to this process. The developed ferroptosis-related prognostic signature (FRPS) and a machine learning (ML)-based recurrence model demonstrated superior predictive capabilities for NB prognosis and recurrence compared to existing models.
What is known and what is new?
• Ferroptosis, an iron-dependent form of cell death, has been previously recognized for its involvement in various cancers, including its impact on tumor biology and progression.
• This study expands the understanding by identifying specific FRGs implicated in NB, linking them to key pathways such as ribosome and cell cycle regulation, and demonstrates their prognostic value through the novel FRPS and recurrence prediction models.
What is the implication, and what should change now?
• The findings suggest that targeting FRGs, particularly MYCN and RRM2, could improve therapeutic outcomes for NB patients. Further research should focus on validating these prognostic models in clinical settings and exploring potential therapeutic interventions that leverage ferroptosis pathways to enhance treatment efficacy for NB.
Introduction
Neuroblastoma (NB), a solid tumor derived from the neural crest during embryonic development, is the most prevalent extracranial malignancy in children (1). Its heterogeneity is a notable characteristic, encompassing localized or disseminated disease with varying outcomes, which significantly impacts pathogenesis, prognosis, and treatment considerations (2). Clinical manifestations in NB patients are contingent upon the location of the primary tumor and may manifest as abdominal mass, abdominal pain, respiratory distress, or neurological symptoms resulting from spinal cord involvement. Unfortunately, many patients succumb to recurrent or refractory metastatic disease. The 5-year survival rates differ depending on risk stratification, with low- to intermediate-risk NB exhibiting rates of 90–95%, whereas high-risk patients face survival rates of 40–50% (3). Particularly, the 5-year survival rate for NB patients experiencing recurrence is even lower (4). Presently, imaging techniques and cytology are the commonly employed methods for detecting tumor recurrence and metastases; however, they are only applicable once the tumor has already developed (5). Consequently, there is an urgent need to identify biomarkers capable of accurately predicting tumor recurrence and prognosis.
Ferroptosis is a form of iron-dependent regulated cell death that is characterized by an excessive buildup of lipid peroxidation on cellular membranes distinct from apoptosis and other forms of cell death which center on cell death executioner proteins (6,7). It represents a dynamic interplay between the requirements for ferroptosis and the cellular defense systems against it. The lethal accumulation of lipid peroxidation, the main feature of ferroptosis, leads to membrane rupture and the demise of cells undergoing ferroptotic cell death when the activities promoting ferroptosis surpass the detoxification capacities provided by the cellular defense systems against it (8).
Unique metabolic pathways of cancer cells, such as enrichment of polyunsaturated fatty acid-containing phospholipid (PUFA-PL), iron overload, and imbalance of the ferroptosis defense system, may represent targetable vulnerabilities to ferroptosis and have sparked great interest in the cancer research community that ferroptosis may constitute a targetable vulnerability of cancer in certain contexts (8). Although ferroptosis is generally viewed as a mechanism for inducing cancer cell death, MYCN-amplified NB cells can leverage this pathway to their advantage. These cells enhance iron uptake through transferrin receptor (TFRC) upregulation, which may foster an iron-rich environment that could potentially promote lipid peroxidation and oxidative stress. In the early stages, this iron-induced oxidative stress supports NB cell proliferation and tumor growth (9). To counteract sustained oxidative damage, MYCN-amplified cells increase glutathione (GSH) synthesis, heightening their dependence on cysteine (10). If this antioxidant defense is compromised, these cells become highly susceptible to ferroptosis, affecting tumor heterogeneity and resistance (11).
In recent years, the remarkable therapeutic potential of ferroptosis has sparked significant interest in cancer research. Recent studies have indicated that ferroptosis may not only influence tumor cell death but also modulate the immune microenvironment, impacting the tumor’s response to therapies, including immunotherapies (12,13). It is also worth noting that cell rupture from ferroptosis may result in the release of oxidized lipids and immunosuppressive cytokines, which could potentially activate regulatory T cells and myeloid-derived suppressor cells (MDSCs). This could lead to a reduction in antitumor immune responses, thereby allowing for immune evasion (14). In NB, which is known for its high metabolic activity and sensitivity to oxidative stress, the regulation of ferroptosis could play a particularly crucial role. It has been demonstrated that the upregulation of key ferroptosis inhibitors, such as GPX4 and SLC7A11, may contribute to a reduction in tumor cell sensitivity to agents such as cisplatin and doxorubicin (15).
Although ferroptosis has been widely studied in many cancers, its specific mechanisms in NB remain underexplored (8). Given the complexity of NB and its interactions with the immune system, understanding how ferroptosis-related genes (FRGs) contribute to tumor progression and patient outcomes is of paramount importance. Investigating FRGs may provide critical insights into novel therapeutic approaches that could enhance the efficacy of existing treatments and offer new strategies for combating treatment-resistant NB.
We speculate that ferroptosis may also play a role in the regulation of NB and could provide avenues for the discovery of novel therapeutic drugs. In this study, we investigated the potential pathways associated with ferroptosis using 232 FRGs identified through univariate Cox analysis. Through differential analysis and the weighted correlation network analysis (WGCNA) algorithm, we identified two hub-FRGs. Based on our findings, we proposed new therapeutic drugs for NB. Additionally, we developed a prognosis model utilizing the expression data of five FRGs and employed machine learning (ML) algorithms to construct a recurrence model for NB. We present this article in accordance with the TRIPOD reporting checklist (available at https://tp.amegroups.com/article/view/10.21037/tp-24-323/rc).
Methods
Publicly available data collection and processing
The study was conducted in accordance with the Declaration of Helsinki (as revised in 2013). For constructing the NB prognostic model, we included RNA-seq datasets with a sample size greater than 50 that contain comprehensive overall survival (OS) information, including OS status, OS time, adverse event, and event-free time. Based on these criteria, GSE62564, GSE16476, and E-TABM-38 were selected for our analysis. Similarly, to develop an NB recurrence model, we selected RNA-seq datasets with a sample size exceeding 50 that provide complete relapse survival information, including relapse occurrence and time to relapse. Accordingly, E-MTAB-179, GSE3446, and GSE8248 were incorporated into our study. The data of 1,650 NB patients from 6 independent public datasets were accessed from the ArrayExpress database and Gene Expression Omnibus (GEO) in total, which were downloaded from R2, a web-based genomics analysis and visualization website (http://r2.amc.nl). A total of 511 FRGs were selected for our analysis from the FerrDb website (http://www.zhounan.org/ferrdb) (16).
Unsupervised clustering
Unsupervised clustering of patients was performed by the consensusClusterPlus package using the expression of the FRGs (17). The clustering method used was pam, with a sampling ratio of 0.8, which is a commonly utilized approach to ensure the reliability of clustering outcomes while preventing overfitting. Subsequently, we utilized the prcomp function of the stats package to conduct principal component analysis (PCA) based on the cluster groups. The survival package was used to compare the prognostic differences between the groups.
WGCNA
The co-expression network of GSE62564 and an appropriate soft threshold β were generated using the WGCNA package (18). Additionally, the weighted adjacency matrix was converted into a topological overlap matrix (TOM), and the corresponding dissimilarity (1-TOM) was calculated. Clustering was performed using dynamic shear trees, and modules with a similarity greater than 0.75 were merged. Modules that were significantly relevant to the clusters were then identified for analysis. Genes with gene significance (GS) >0.5 and module membership (MM) >0.6 were considered as hub cluster-related genes.
Identifying differentially expressed genes (DEGs) and gene set enrichment analysis (GESA)
The calculation of DEGs (ss) [|log2 (fold-change, FC)| >1 and false discovery rate (FDR) <0.05] was implemented using the limma package, and GSEA was performed using the clusterProfiler package to identify the biological terms of the DEGs (19,20). We took the intersection of the two groups of genes screened by WGCNA and differential analysis and then performed the Gene Ontology (GO) and Kyoto Encyclopedia of Genes and Genomes (KEGG) enrichment analysis (21).
Construction of the gene expression-based prognosis model
According to a 7:3 ratio, which was chosen based on the characteristics of the dataset and the need for sufficient test data to ensure robust evaluation of the model’s generalizability, 498 samples of GSE62564 were divided into a training set (n=349) and a testing set (n=149). E-TABM-38 and GSE16476 with the same clinical information were used as validation sets. The training set was employed to construct a signature for predicting the prognostic risk in NB patients, utilizing the OS status and the OS time as the outcome. The least absolute shrinkage and selection operator (LASSO), an ML regression algorithm, was performed using the R package glmnet to narrow down the FRGs. The penalty parameter (λ) was determined based on the minimum parameters. Multivariate Cox regression analysis was used, followed by LASSO regression analysis, to establish the prognosis model, with a significance threshold of P<0.05. Afterwards, we the following formula was applied to calculate the risk score of the FRG for each patient:
where Coef represents the coefficient of the multivariate Cox regression analysis and Exp represents the expression level of the FRGs. Furthermore, we used receiver operating characteristic (ROC) and Kaplan-Meier (KM) analyses to examine the efficacy of the model in each set. The area under the curve (AUC) and concordance index (C-index) were calculated for comparisons with the other 10 models.
Cells infiltration estimation
To assess whether signatures can be used to predict response to immune checkpoint inhibitors (ICIs), we calculated the Tumor Immune Dysfunction and Exclusion (TIDE) scores using the TIDE website (http://tide.dfci.harvard.edu) with the GSE62564 dataset (22). Tumor immune escape mechanisms are 2-fold: some tumors inhibit T cell infiltration through various immunosuppressive factors; others, despite having a high level of cytotoxic T cell infiltration, harbor T cells that are functionally exhausted. The TIDE score quantitatively predicts a tumor’s potential for immune escape by evaluating these two mechanisms. The estimate package was adopted to calculate the StromalScore, ImmuneScore, ESTIMATEScore, and TumorPurity of two different risk groups for exploring the differences in the degree of immune cell infiltration. Single-sample GSEA (ssGSEA) was employed to quantify the relative infiltration of cytotoxic T cells in the GSE62564 using the GSVA package. Additionally, four other algorithms including Xcell, quanTIseq, MCP-counter, and EPIC were used to verify the stability and robustness of the ssGSEA results.
Construction of a nomogram
Signatures and patients’ clinical characteristics together were entered into univariate and multifactorial Cox regression analyses using P<0.05 and HR ≠1 as the criterion. To improve the predictive performance of signatures, we used the rms package to integrate signatures, patient age, and tumor stage to construct the nomogram, which was used to predict 1-, 3-, 5-, and 7-year survival probability. The nomogram was built according to the clinical phenotypes, filtered by the univariate and multivariate Cox regression analysis. The calibration and decision curves of 1–5 years were calculated to evaluate the performance of the nomogram. Time-ROC analysis was used to measure the effectiveness of the nomogram.
Construction and comparison of the recurrence model from ML-based approaches
It is widely acknowledged that tumor recurrence is often closely related to a poor prognosis. In order to further verify the predictive utility of FRGs in NB, we established an ML model to predict tumor recurrence based on the E-MTAB-179 dataset. GSE3446 and GSE8248 were the validation sets. Genes for the recurrence model were selected based on the importance scores calculated using the filtering algorithm with the mlr3filters package. The ML algorithms including random forest (RF), XGboost, support vector machine (SVM), linear discriminant analysis (LDA), and K-nearest neighbors (KNN) were generated to leverage the unique strengths of each model in handling complex, high-dimensional biological data. The mlr3 package was used to develop a model with high accuracy and stable performance to predict whether the tumor will recur. For RF, we specified parameter ranges, including num.trees [100–1,000] to control forest size, mtry (1–total features) for feature diversity at splits, min.node.size [1–20] to prevent overfitting by limiting leaf size, and max.depth [1–15] to control tree complexity. For XGBoost, we detailed parameters such as nrounds [50–500] for boosting iterations, eta [0.01–1] as the learning rate to gradually fit the model and prevent overfitting, gamma [0–5] to penalize complex trees, max_depth [1–10] to limit tree size, and subsample [0.5–1] to increase model diversity. In SVM, we used cost [0.1–10], which balances margin width with misclassification tolerance, and gamma [0.01–1], the kernel coefficient to adjust decision boundary curvature for better pattern fitting in high-dimensional data. For KNN, we defined k [1–30] to select neighbor count, balancing sensitivity, and smoothness, and chose distance metrics (Manhattan or Euclidean) to control similarity measures, as each metric affects model neighborhood selection. To evaluate the performance of the model, we adopted parameters including accuracy (ACC), AUC, the area under the precision-recall curve (PRAUC), F-beta, recall, and precision.
Statistical analysis
All statistical analyses were conducted using R software (version 4.1.2; R Foundation for Statistical Computing, Vienna, Austria). Survival probabilities were calculated using the KM method, and differences between survival curves were assessed with the log-rank test. Univariate and multivariate Cox proportional hazards regression were applied to determine independent prognostic factors, whereas feature selection utilized the LASSO regression. The accuracy of prognostic and recurrence models was measured via ROC curves and AUC. Statistical significance was defined as a 2-sided P value no more than 0.05.
Results
Construction and evaluation of ferroptosis-related consensus clusters
The workflow of this research is depicted in Figure S1. Based on the expression of 232 FRGs screened by the univariate Cox analysis (Table S1), we conducted consensus clustering of NB samples, testing k values ranging from 2 to 10 (17). Analysis of the cumulative distribution function (CDF) curves suggested that the optimal clustering occurred at k=2 (23) (Figure 1A and Figure S2A-S2C). Prognostic analysis revealed significant survival differences between the two clusters, C1 and C2, with C2 showing a substantially poorer OS rate than C1 (Figure 1B,1C). At the same time, the PCA results showed that C1 and C2 have a clear distinction (Figure 1D). Furthermore, we performed ssGSEA and found a clear difference in immune infiltration between C1 and C2, with poorer prognosis and a lower degree of immune infiltration in C2, which is the same as reported that NB is the “immune-cold tumor” (24). To rule out potential biases introduced by the analytical algorithm, the stability and robustness of the ssGSEA results were further validated using three alternative algorithms: quanTIseq, MCP-counter, and EPIC (Figure S2D). The alluvial diagram was used to visualize the attribute changes of FRGs in clinical features (Figure 1E). A total of 673 DEGs (400 upregulated and 273 downregulated genes) with |log2 FC| >1 and FDR <0.05 were identified and shown in the volcano plot (Figure 1F).
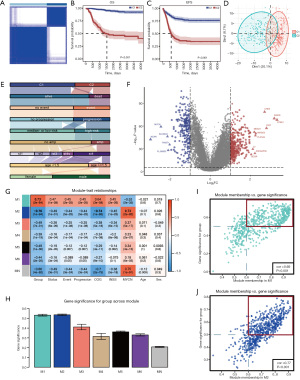
Identification of modules derived from the FRGs patterns
During the WGCNA process, a soft threshold β of 12 (scale R2 =0.88) was applied, providing an optimal power for co-expression network construction (Figure S3A,S3B). A total of 7 modules were identified, with the eigengene (the first principal component of module gene expression) representing each module (Figure S3C,S3D). A heatmap illustrated the adjacency relationships among module eigengenes (Figure S3E). Moreover, the relationships between modules and clinical features, such as FRGs clusters, status, event, progression, Children’s Oncology Group (COG) risk, International Neuroblastoma Staging System (INSS) stages, MYCN status, age at diagnosis, and sex, were calculated. The M1 and the M2 modules have higher correlation and GS than other modules, in which the M1 module is positively correlated and the M2 module is negatively correlated (Figure 1G,1H). The GS-MM correlation coefficient was 0.69 in the M1 module and 0.77 in the M2 module, reflecting the reliability of the FRGs module structure. A total of 552 genes contained in the red rectangles, meeting the criteria of GS >0.5 and MM >0.6, were identified as ferroptosis-related regulatory genes (FRRPs), representing hub genes linked to FRGs patterns within the M1 and M2 modules (Figure 1I,1J).
Exploration of the regulatory pathways of FRGs and potential therapeutic targets
By incorporating expression data of all genes into GSEA analysis based on the result of differential analysis, we found that C2, with a relatively poor prognosis, is mainly enriched in pathways such as ribosome and DNA replication, whereas C1, with a relatively good prognosis, is mainly enriched in immune-related pathways such as cell adhesion molecules and Th17 cell differentiation. This suggests that FRGs may participate in the process of NB tumors through immune-related and ribosome-related pathways (Figure 2A). To verify this conjecture, we intersected 673 DEGs and 552 FRRGs with the aim of refining our selection to genes that are not only co-expressed but also differentially regulated under NB-related conditions, resulting in 279 hub-FRRGs (Figure 2B). GO and KEGG analysis were performed based on the hub-FRRGs, and the results confirmed our conjecture that ribosome and cell cycle-related pathways are crucial in the process of ferroptosis participating in the regulation of NB tumors (Figure 2C-2F). In our continued efforts to elucidate the role of ferroptosis in NB and identify key genes through which ferroptosis may exert its effects, we intersected 232 FRGs with FRRGs. This analysis yielded two notable genes: MYCN and RRM2. We observed that not only do MYCN and RRM2 show significant expression differences between C1 and C2, but their expression levels are also closely associated with the prognosis of NB patients (Figure 2G-2L).
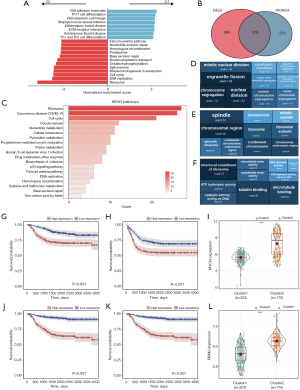
Construction and validation of a prognostic signature
A total of 232 FRGs screened by the univariate Cox analysis were used to develop a ferroptosis-related prognostic signature (FRPS). Some 498 NB patients from GSE62564 were divided into a training set (349 patients) and a testing set (149 patients) randomly. E-TABM-38 and GSE16476 were used as validation sets.
In the LASSO regression, the optimal λ was obtained when the partial likelihood deviance reached the minimum value based on the LOOCV framework (Figure 3A,3B). Multivariate Cox proportional hazards regression identified a final set of 5 FRGs (ATG7, ELAVL1, PPARA, RDX6, and TERT) to construct the FRPS. The FRPS of each patient was calculated according to the formula: FRPS = e(−1.0092289 * the expression of ATG7) + (1.7393591 * the expression of ELAVL1) + (0.4928858 * the expression of PPARA) + (1.4565915 * the expression of RDX6) + (0.2471766 * the expression of TERT). The C-index [95% confidence interval (CI)] was 0.850 (0.0.833–0.867), 0.852 (0.807–0.900), 0.820 (0.789–0.852), and 0.741 (0.700–0.784) in the 4 cohorts. Time-ROC analysis measured the discrimination of FRPS, with 1-, 3-, 5-, and 7-year AUCs of 0.86, 0.91, 0.91, and 0.9 in the training set; 0.87, 0.89, 0.85, and 0.85 in the testing set; 0.91, 0.84, 0.84, and 0.87 in E-TABM-38; 0.72, 0.81, 0.83, and 0.85 in GSE16476 (Figure 3C-3F), respectively. All patients were assigned into the high- and low-risk groups according to the Youden index determined by the survminer package (Figure 3G-3J). Furthermore, high-risk patients had significantly dismal OS and event-free survival (EFS) relative to the low-risk group in the four cohorts (all P<0.001) (Figure 3K-3R).
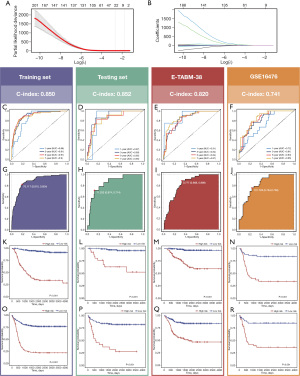
Comparison and exploration of FRPS in NB
With the developments of next-generation sequencing and big-data technologies, ML has enabled the creation of various prognostic and predictive gene expression signatures. To benchmark FRPS, we obtained 10 NB signatures from published studies that were applicable for validation in GSE62564, as multiple signatures cannot be satisfied at the same time in other datasets. These signatures were associated with ferroptosis, cuproptosis, pyroptosis, transcription factor, immunity, RNA binding protein, INSS stages, malignant-cell marker, and m6A (25-34). Notably, 1 of these signatures is also based on the FRGs (25).
We performed time-ROC analysis on each signature and obtained 1-, 3-, 5-, and 7-year AUCs and observed that our FRPS had the best performance. At the same time, comparison of the C-index with that of other models revealed that the FRPS yielded the highest C-index (Figure 4A). Furthermore, we compared the C-index of FRPS with its constituent genes and clinical features showing that FRPS can better predict the prognosis of NB patients (Figure 4B,4C).
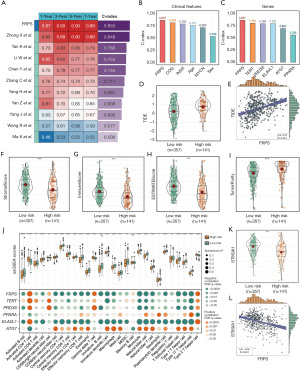
Predictive value of immune checkpoint inhibition therapy benefits
The 498 patients from GSE62564 were divided into a high-risk group (141 patients) and a low-risk group (357 patients) by the Youden index. By calculating the TIDE score of each patient to predict the tumor’s potential for immune escape, we found that the TIDE scores in the high-risk group were significantly higher than those in the low-risk group, and FRPS was positively correlated with the TIDE score (correlation value: 0.37, P<0.001) (Figure 4D,4E), indicating that high-risk patients may have diminished responsiveness to ICI therapy.
To explore whether the higher TIDE scores in the high-risk group were caused by highly infiltrated cytotoxic T cells in a dysfunctional state or by less infiltrated cytotoxic T cells, we investigated the difference in the degree of immune infiltration between the two groups using the ESTIMATE algorithm. The results showed that the degree in the low-risk group was significantly higher than that in the high-risk group, whereas the TumorPurity scores were significantly lower than those in the high-risk group (Figure 4F-4I).
We used the ssGSEA algorithm to further explore the degree of infiltration of different immune cells in the two groups, showing that the degree of infiltration of cytotoxic T cell (activated CD8+ T cell) was significantly different between the two groups (P=0.03). The difference was validated using the Xcell, quanTIseq, MCP-counter, and EPIC algorithms (Figure S4). These results suggested that the high TIDE scores in the high-risk group were due to the lower degree of infiltration of cytotoxic T cells (Figure 4J).
To verify our conclusion, we explored the difference in the expression of the synthase gene of GD2, the only monoclonal antibody that is currently effective in NB (35). Studies have shown that only ST8SIA1 is strongly correlated with GD2 expression in the ganglioside synthesis pathway (36-38). We explored the expression differences of the ST8SIA1 gene between high and low FRPS groups as well as the correlation between ST8SIA1 and FRPS, and results showed that ST8SIA1 was highly expressed in the low-risk group with a negative correlation between ST8SIA1 expression and FRPS (Figure 4K,4L). These findings provided evidence that FRPS can effectively predict the efficacy of immune therapy.
Prognostic nomogram construction using clinical features and FRPS
In order to enable FRPS to better predict the prognosis of NB patients, we screened three clinical features that are the independent risk factors to participate in the construction of the nomogram through univariate analysis and multivariate analysis (HR ≠1, P<0.05) (Figure 5A,5B). Using these 4 variables, a nomogram model was subsequently developed and the NOMO-score (NS) was calculated for each patient (Figure 5C). The C-index of the NS was 0.885, which is higher than that of FRPS and other clinical features. Time-ROC analysis was used to verify the predictive power, and the results showed that NS has the best AUCs at 1-, 3-, 5-, and 7-year time points (0.88, 0.93, 0.93, 0.92) (Figure 5D-5G). We adopted the Youden index to divide patients into high- and low-risk groups based on the NS and showed an excellent prognostic difference through KM analysis (Figure 5H). In order to further explore the stability and performance of the nomogram, we conducted a calibration curve analysis, which demonstrated strong agreement between predicted and observed values for 1-, 3-, 5-, and 7-year OS (Figure 5I).
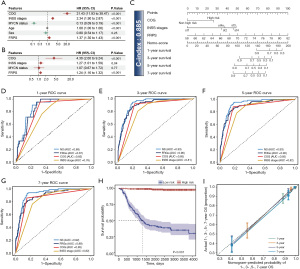
Predicting recurrence based on ML algorithms using FRGs
In order to further verify the predictive ability of FRGs on the prognosis of NB patients, we selected three datasets encompassing complete RFS information, of which E-MTAB-179 was used as the training set, while GSE3446 and GSE8248 were used as validation sets. We built a model based on the ML algorithm to predict whether the tumor will recur after treatment. With recurrence as the response variable, we assessed each FRG with an importance score via the flt algorithm using the mlr3filters package and selected the top eight genes as recurrence-related genes (RRGs) (ALDH3A2, TERT, ULK2, AKR1C1, MFN2, SLC16A1, TF, DDR2) with the highest importance scores (Figure 6A).
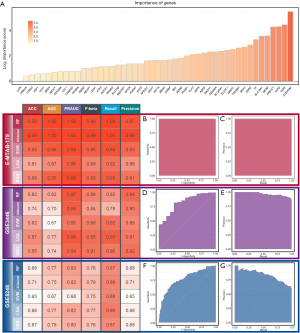
We used the RF, XGboost, SVM, LDA, and KNN algorithms, respectively, with recurrence as the response variable and RRGs as the predictor variable using the mlr3 package. The 5 learners were provided by the mlr3learners package. The selection of hyperparameters of RF, XGboost, SVM, and KNN was implemented by the random-search algorithm of the mlr3tuning package, with internal 20-fold cross-validation and external 5-fold cross-validation, using accuracy as the evaluation index. The values of the hyperparameters in the RF model were num.threads =1, num.trees =403, mtry =2, min.node.size =8, and max.depth =9. The values of the hyperparameters in the XGboost model were nrounds =161, nthread =1, verbose =0, eta =1, gamma =1, max_depth =3, and subsample =0.9922. The values of the hyperparameters in the SVM model were cost =5.082, kernel =rbf, gamma =0.3157, and type = C-classification. The values of the hyperparameters in the KNN model were k=20, and distance =1. Leave-one-out cross-validation was used in the LDA model.
ACC, AUC, PRAUC, F-beta, recall, and precision were selected to evaluate the model using the mlr3measures package. The results showed that the RF model and the XGboost model have similar performance in the training set (E-MTAB-179) and 1 of the validation sets (GSE8248), but the RF model performed significantly better than the XGboost model in the other validation set (GSE3446). Poor generalization performance may have been due to the higher degree of overfitting of the XGboost model compared to the RF model. We therefore choose the RF model as our final recurrence model, with the AUC of 1 and PRAUC of 1 in the training set (Figure 6B,6C), the AUC of 0.82 and PRAUC of 0.97 in the GSE3446 (Figure 6D,6E), and the AUC of 0.77 and PRAUC of 0.83 in the GSE8248 (Figure 6F,6G).
Discussion
Ferroptosis is an iron-dependent regulated form of cell death driven by lipid peroxidation overload on the cell membrane. It differs morphologically and mechanistically from apoptosis and other types of regulated cell death.
NB is a tumor of sympathetic nervous system origin, and its clinical presentation is highly heterogeneous, ranging from localized disease to widespread metastasis, and even spontaneous regression (35). Currently, the commonly used methods for assessing the prognosis of NB patients include the pretreatment INSS and post-treatment COG risk stratification. In recent years, researchers have been exploring the use of RNA-seq data to identify prognostic markers or biomarkers that can assist clinicians in determining optimal treatment approaches for NB patients.
To investigate the potential pathways involved in NB development and their association with ferroptosis, we analyzed RNA-seq data based on FRGs. We identified key genes involved in this regulatory process and proposed potential drugs that may improve the prognosis of NB patients. Furthermore, we explored the prognostic and predictive value of FRGs in NB patients using a stepwise regression Cox model and nomogram analysis. Additionally, we validated the stability and effectiveness of FRGs in prognostic prediction by constructing a recurrence model using ML algorithms. According to our knowledge, this is the first study to explore the relationship between ferroptosis and NB recurrence employing ML algorithms while investigating the association between ferroptosis and the prognosis of NB patients using the RNA-seq data.
Based on the expression data of FRGs, we performed unsupervised clustering and identified two distinct groups of patients with significant differences in both prognosis and immune infiltration levels, indicating the potential involvement and significant roles of FRGs in regulating the occurrence and development of NB. Through an intersecting analysis of module genes from WGCNA, DEGs, and FRGs, we identified hub-FRRGs and conducted an enrichment analysis of hub genes. The results revealed that ferroptosis likely regulates NB through pathways related to ribosomes and the cell cycle. Moreover, we identified two hub-FRGs, MYCN and RRM2, a rate-limiting enzyme involved in DNA synthesis and repair.
Recent studies have highlighted the crucial role of ferroptosis in NB progression, particularly through MYCN amplification. MYCN-amplified NB cells demonstrate increased susceptibility to ferroptosis due to altered iron metabolism and GSH dependency, which are driven by MYCN overexpression (9,39). Specifically, MYCN regulates the expression of TFRC, a key protein involved in iron uptake. The resulting increase in cellular iron contributes to the labile iron pool, promoting lipid peroxidation—a hallmark of ferroptosis. This TFRC-dependent iron influx sensitizes MYCN-amplified cells to GPX4-targeting ferroptosis inducers, which inhibit the primary antioxidant defense against lipid peroxides, leading to cell death (10).
In addition, MYCN-amplified NB cells exhibit a unique vulnerability to cysteine deprivation, as MYCN intensifies cellular cysteine demand to maintain GSH levels and counteract oxidative stress. Depletion of cysteine disrupts GSH synthesis, further predisposing these cells to ferroptosis via lipid peroxidation accumulation, as corroborated by recent studies highlighting MYCN-mediated cysteine addiction in NB (9,40). This relationship underscores ferroptosis as a critical modality in targeting MYCN-driven NB, as dual targeting of iron uptake (via TFRC) and antioxidant defenses (via GPX4 inhibition) has shown promising effects in reducing tumor viability (8,41).
RRM2, another hub-gene associated with DNA repair and redox homeostasis, is upregulated in MYCN-amplified cells and plays a complementary role in promoting resistance to oxidative stress.
Nunes et al. found in their study that RRM2 overexpression accelerated NB formation and increased tumor incidence in the MYCN-driven zebrafish NB model. Elevated RRM2 expression levels aided NB tumor cells in coping with replication stress and activating the ATR-CHK1 pathway (42). Treatment of NB cell lines with the RRM2 inhibitor 3AP (triapine, an iron chelator) resulted in inhibited growth of high-risk NB-derived cell lines upon suppression of RRM2 levels. Subsequently, the authors employed translocation-adjacent-end ligation sequencing (TrAEL-seq) and observed replication fork stalling induced by 3AP treatment.
Furthermore, Li et al. demonstrated that the expression of RRM2 in NB tissue was significantly higher at both the messenger RNA (mRNA) and protein levels compared to adjacent non-cancerous tissues. Elevated RRM2 levels were significantly correlated with clinical staging (43). Compared to tumors from the pre-chemotherapy subgroup, RRM2 levels were inhibited in stage III and IV tumors of the chemotherapy subgroup. RRM2 small interfering RNA (siRNA) significantly suppressed the cell viability of SH-SY5Y cells, induced cell cycle arrest at the G0/G1 phase, and enhanced cell apoptosis.
However, its interaction with ferroptosis mechanisms in MYCN-amplified NB requires further investigation. Future research should focus on combinational therapies that target ferroptosis vulnerabilities, such as the simultaneous inhibition of TFRC and RRM2, to enhance therapeutic efficacy against high-risk, MYCN-amplified NB cases (44).
In this study, we developed the FRPS to predict NB outcomes and explore its potential in evaluating immunotherapy effectiveness. Through univariate analysis, we identified FRGs that are not only associated with NB prognosis but may also impact the tumor immune microenvironment. By analyzing gene expression data across ML algorithms such as LASSO regression and multivariate Cox analysis, we narrowed the gene set to a smaller number of key FRGs that demonstrated strong predictive value. These genes were incorporated into the prognostic model, with FRPS calculated based on their expression levels. The FRPS not only had robust performance in the validation set but also exhibited superior outcomes when benchmarked against alternative signatures. Furthermore, the prognosis of cancer patients is often closely related to the efficacy of drug treatment, with ICI therapy attracting particular attention. In order to investigate whether FRPS can predict the efficacy of ICI therapy in NB patients, we evaluated the TIDE scores. The results revealed significant differences in TIDE scores between the high- and low-risk groups based on FRPS, with significantly higher scores observed in the high-risk group. Also, there was a positive correlation between TIDE scores and FRPS. We believe that FRPS can not only predict the prognosis of children with NB well but also predict the efficacy of ICI therapy to a certain extent, thereby helping clinicians to better provide precise treatment for each child.
To further explore the reasons behind the significantly higher TIDE scores in the high-risk group compared to the low-risk group, we utilized ESTIMATE and ssGSEA analyses and examined the differential expression of monoclonal antibody GD2’s glycosyltransferase gene between the two groups.
Our analysis revealed significant differences in the tumor immune microenvironment between the high- and low-risk groups. The high-risk group, as defined by the FRPS, which exhibited significantly lower levels of cytotoxic T-cell infiltration compared to the low-risk group (P=0.03), may evade immune detection and thus be less responsive to ICIs. The correlation between FRPS and ST8SIA1 highlights a possible mechanism through which ferroptosis-related pathways may influence tumor immunity. High GD2 expression is linked to enhanced susceptibility to GD2-targeted immunotherapies, suggesting that FRPS may serve as an indirect predictor of immunotherapy effectiveness. These findings underscore the clinical relevance of the FRPS in not only predicting NB prognosis but also in assessing which patients may benefit more from immunotherapy. The ability to stratify patients based on their immune microenvironment could inform more personalized treatment strategies, with high-risk patients potentially requiring combination therapies that address immune evasion mechanisms.
Furthermore, by incorporating clinical characteristics of NB patients into single and multiple-factor Cox analysis, we identified two independent prognostic risk factors. These two independent prognostic risk factors, along with FRPS, were incorporated into the construction of a nomogram. Compared to FRPS alone, the predictive performance of the nomogram, referred to as NS, showed a significant improvement, with a C-index of 0.885 and AUCs of 0.88, 0.93, 0.93, and 0.92 at 1-, 3-, 5-, and 7-year time points, respectively.
Traditional clinical risk stratification relies heavily on a variety of clinical data, including age, imaging findings, and pathological diagnoses. Currently, the integration of genetic data into risk stratification models has not been widely adopted in clinical practice, primarily due to the high costs associated with sequencing technologies, which remain unaffordable for many families. However, we are optimistic that as technological advancements continue to drive down the costs of sequencing, genetic testing will eventually become a routine component of clinical diagnosis. When this transition occurs, our NS, which incorporates gene expression data, will enable more tailored and precise treatment options for patients, thereby facilitating the realization of personalized medicine. This approach not only enhances prognostic accuracy but also aligns with the evolving trends in medical practice towards more data-driven and individualized patient care.
The prognosis of tumor patients is largely influenced by the occurrence of recurrence after treatment. Therefore, we constructed models using multiple ML algorithms based on FRGs to predict the recurrence of NB patients. The results showed that RF and XGboost performed remarkably well in both the training and validation sets. In one of the validation sets, RF outperformed XGboost, whereas in other datasets, both models performed similarly. As a result, we ultimately selected the RF model as our final predictive model for post-treatment recurrence in NB patients.
In recent years, ferroptosis has emerged as a promising therapeutic strategy for high-risk NB, particularly in cases with MYCN amplification. Several studies have highlighted that MYCN amplification enhances ferroptosis sensitivity by increasing iron uptake and altering the oxidative balance in NB cells. For instance, studies have shown that MYCN-mediated upregulation of the TFRC leads to intracellular iron accumulation, promoting lipid peroxidation and enhancing susceptibility to GPX4 inhibition-induced ferroptosis (9,10,39,41).
However, further research is needed to address the challenges of inducing ferroptosis in a controlled manner. Unresolved issues include the need to understand alternative pathways that cancer cells may use to evade ferroptosis, such as through alternative antioxidant mechanisms. Additionally, there is a need to refine ferroptosis inducers to reduce potential toxicities when used clinically, as in vivo applications of GPX4 inhibitors have been limited by systemic toxicity concerns. Research into selective inducers that minimize damage to normal cells and optimize iron metabolism dysregulation in NB remains a critical future direction.
Furthermore, the potential synergy of ferroptosis with immunotherapy in NB represents an exciting yet underexplored avenue. Given that ferroptosis and immune response pathways can influence each other, exploring the interactions between ferroptosis induction and immune modulation may provide a foundation for combination therapies, enhancing the efficacy of existing immunotherapeutic strategies.
Compared to other studies, our research not only established a more effective and robust prognosis model but also utilized ML algorithms to construct a recurrence model, thereby enhancing the reliability of our conclusions. One of the key strengths of our model lies in its ability to integrate multiple datasets, which enhances its robustness and generalizability. Additionally, we investigated the potential regulatory mechanisms of ferroptosis in NB and identified hub-RRGs, offering more possibilities for improving the prognosis of NB patients through targeted drug interventions.
Our study still has certain limitations. Firstly, the relatively small sample size in some datasets may reduce the model’s ability to generalize to larger, more diverse patient populations. This limitation is compounded by the heterogeneity between datasets, which may introduce variability in gene expression profiles and clinical outcomes. Secondly, although our model shows high predictive performance, it has not yet been validated in a large, independent clinical cohort, which is necessary to assess its real-world applicability. Thirdly, although the FRPS exhibited good prognostic efficacy in the overall sample, its performance was generally inferior in the MYCN-amplified subgroup compared to the MYCN non-amplified subgroup across the GSE62565, E-TABM-38, and GSE16476 datasets. We attempted to develop a specific prognostic model for the MYCN-amplified group; however, the limited sample size in this subgroup hindered the construction of a model with robust efficacy. Additionally, this study did not extensively explore the functional roles of FRGs in NB development. Further research can investigate the importance of ribosome and cell cycle-related pathways involving MYCN and RRM2 in NB development and their potential as therapeutic targets.
Conclusions
Our study has identified the significant role of FRGs in the occurrence and development of NB. We have also explored the relevant pathways that may be involved and identified two hub-FRGs with potential therapeutic value. Furthermore, through the establishment of prognosis Cox models, nomograms, and recurrence models, we have demonstrated the effective and robust predictive capability of FRGs for the prognosis of NB patients. This further emphasizes the significant impact of ferroptosis on the prognosis of NB patients.
Acknowledgments
We would like to acknowledge the providers of gene expression data and clinical information for the included datasets, the GEO database, the ArrayExpress website, and the R2 website.
Funding: This work was supported by
Footnote
Reporting Checklist: The authors have completed the TRIPOD reporting checklist. Available at https://tp.amegroups.com/article/view/10.21037/tp-24-323/rc
Peer Review File: Available at https://tp.amegroups.com/article/view/10.21037/tp-24-323/prf
Conflicts of Interest: All authors have completed the ICMJE uniform disclosure form (available at https://tp.amegroups.com/article/view/10.21037/tp-24-323/coif). The authors have no conflicts of interest to declare.
Ethical Statement: The authors are accountable for all aspects of the work in ensuring that questions related to the accuracy or integrity of any part of the work are appropriately investigated and resolved. The study was conducted in accordance with the Declaration of Helsinki (as revised in 2013).
Open Access Statement: This is an Open Access article distributed in accordance with the Creative Commons Attribution-NonCommercial-NoDerivs 4.0 International License (CC BY-NC-ND 4.0), which permits the non-commercial replication and distribution of the article with the strict proviso that no changes or edits are made and the original work is properly cited (including links to both the formal publication through the relevant DOI and the license). See: https://creativecommons.org/licenses/by-nc-nd/4.0/.
References
- Johnsen JI, Dyberg C, Wickström M. Neuroblastoma-A Neural Crest Derived Embryonal Malignancy. Front Mol Neurosci 2019;12:9. [Crossref] [PubMed]
- Swift CC, Eklund MJ, Kraveka JM, et al. Updates in Diagnosis, Management, and Treatment of Neuroblastoma. Radiographics 2018;38:566-80. [Crossref] [PubMed]
- Pinto NR, Applebaum MA, Volchenboum SL, et al. Advances in Risk Classification and Treatment Strategies for Neuroblastoma. J Clin Oncol 2015;33:3008-17. [Crossref] [PubMed]
- DuBois SG, Mody R, Naranjo A, et al. MIBG avidity correlates with clinical features, tumor biology, and outcomes in neuroblastoma: A report from the Children’s Oncology Group. Pediatr Blood Cancer 2017;
- Su Y, Wang L, Jiang C, et al. Increased plasma concentration of cell-free DNA precedes disease recurrence in children with high-risk neuroblastoma. BMC Cancer 2020;20:102. [Crossref] [PubMed]
- Dixon SJ, Patel DN, Welsch M, et al. Pharmacological inhibition of cystine-glutamate exchange induces endoplasmic reticulum stress and ferroptosis. Elife 2014;3:e02523. [Crossref] [PubMed]
- Galluzzi L, Vitale I, Aaronson SA, et al. Molecular mechanisms of cell death: recommendations of the Nomenclature Committee on Cell Death 2018. Cell Death Differ 2018;25:486-541. [Crossref] [PubMed]
- Lei G, Zhuang L, Gan B. Targeting ferroptosis as a vulnerability in cancer. Nat Rev Cancer 2022;22:381-96. [Crossref] [PubMed]
- Lu Y, Yang Q, Su Y, et al. MYCN mediates TFRC-dependent ferroptosis and reveals vulnerabilities in neuroblastoma. Cell Death Dis 2021;12:511. [Crossref] [PubMed]
- Alborzinia H, Flórez AF, Kreth S, et al. MYCN mediates cysteine addiction and sensitizes neuroblastoma to ferroptosis. Nat Cancer 2022;3:471-85. [Crossref] [PubMed]
- Floros KV, Cai J, Jacob S, et al. MYCN-Amplified Neuroblastoma Is Addicted to Iron and Vulnerable to Inhibition of the System Xc-/Glutathione Axis. Cancer Res 2021;81:1896-908. [Crossref] [PubMed]
- Nakaya M, Xiao Y, Zhou X, et al. Inflammatory T cell responses rely on amino acid transporter ASCT2 facilitation of glutamine uptake and mTORC1 kinase activation. Immunity 2014;40:692-705. [Crossref] [PubMed]
- Gao M, Monian P, Quadri N, et al. Glutaminolysis and Transferrin Regulate Ferroptosis. Mol Cell 2015;59:298-308. [Crossref] [PubMed]
- Yan R, Lin B, Jin W, et al. NRF2, a Superstar of Ferroptosis. Antioxidants (Basel) 2023;12:1739. [Crossref] [PubMed]
- Zuo Y, Xie J, Li X, et al. Ferritinophagy-Mediated Ferroptosis Involved in Paraquat-Induced Neurotoxicity of Dopaminergic Neurons: Implication for Neurotoxicity in PD. Oxid Med Cell Longev 2021;2021:9961628. [Crossref] [PubMed]
- Zhou N, Bao J. FerrDb: a manually curated resource for regulators and markers of ferroptosis and ferroptosis-disease associations. Database (Oxford) 2020;2020:baaa021. [Crossref] [PubMed]
- Wilkerson MD, Hayes DN. ConsensusClusterPlus: a class discovery tool with confidence assessments and item tracking. Bioinformatics 2010;26:1572-3. [Crossref] [PubMed]
- Langfelder P, Horvath S. WGCNA: an R package for weighted correlation network analysis. BMC Bioinformatics 2008;9:559. [Crossref] [PubMed]
- Ritchie ME, Phipson B, Wu D, et al. limma powers differential expression analyses for RNA-sequencing and microarray studies. Nucleic Acids Res 2015;43:e47. [Crossref] [PubMed]
- Wu T, Hu E, Xu S, et al. clusterProfiler 4.0: A universal enrichment tool for interpreting omics data. Innovation (Camb) 2021;2:100141. [Crossref] [PubMed]
- Kanehisa M, Furumichi M, Tanabe M, et al. KEGG: new perspectives on genomes, pathways, diseases and drugs. Nucleic Acids Res 2017;45:D353-61. [Crossref] [PubMed]
- Jiang P, Gu S, Pan D, et al. Signatures of T cell dysfunction and exclusion predict cancer immunotherapy response. Nat Med 2018;24:1550-8. [Crossref] [PubMed]
- Șenbabaoğlu Y, Michailidis G, Li JZ. Critical limitations of consensus clustering in class discovery. Sci Rep 2014;4:6207. [Crossref] [PubMed]
- El-Hajjar M, Gerhardt L, Hong MMY, et al. Inducing mismatch repair deficiency sensitizes immune-cold neuroblastoma to anti-CTLA4 and generates broad anti-tumor immune memory. Mol Ther 2023;31:535-51. [Crossref] [PubMed]
- Chen Y, Li Z, Cao Q, et al. Ferroptosis-related gene signatures in neuroblastoma associated with prognosis. Front Cell Dev Biol 2022;10:871512. [Crossref] [PubMed]
- Yang H, Yang J, Bian H, et al. A novel cuproptosis-related gene signature predicting overall survival in pediatric neuroblastoma patients. Front Pediatr 2022;10:1049858. [Crossref] [PubMed]
- Li W, Li X, Xia Y, et al. Pyroptosis-Related Gene Signature Predicts the Prognosis and Immune Infiltration in Neuroblastoma. Front Genet 2022;13:809587. [Crossref] [PubMed]
- Wang R, Wang Q. Identification and External Validation of a Transcription Factor-Related Prognostic Signature in Pediatric Neuroblastoma. J Oncol 2021;2021:1370451. [Crossref] [PubMed]
- Ma K, Zhang P, Xia Y, et al. A signature based on five immune-related genes to predict the survival and immune characteristics of neuroblastoma. BMC Med Genomics 2022;15:242. [Crossref] [PubMed]
- Yang J, Zhou J, Li C, et al. Integrated analysis of the functions and prognostic values of RNA-binding proteins in neuroblastoma. PLoS One 2021;16:e0260876. [Crossref] [PubMed]
- Zhong X, Liu Y, Liu H, et al. Identification of Potential Prognostic Genes for Neuroblastoma. Front Genet 2018;9:589. [Crossref] [PubMed]
- Yan Z, Liu Q, Cao Z, et al. Multi-omics integration reveals a six-malignant cell maker gene signature for predicting prognosis in high-risk neuroblastoma. Front Neuroinform 2022;16:1034793. [Crossref] [PubMed]
- Tan K, Wu W, Zhu K, et al. Identification and Characterization of a Glucometabolic Prognostic Gene Signature in Neuroblastoma based on N6-methyladenosine Eraser ALKBH5. J Cancer 2022;13:2105-25. [Crossref] [PubMed]
- Wang Z, Cheng H, Xu H, et al. A five-gene signature derived from m6A regulators to improve prognosis prediction of neuroblastoma. Cancer Biomark 2020;28:275-84. [Crossref] [PubMed]
- Qiu B, Matthay KK. Advancing therapy for neuroblastoma. Nat Rev Clin Oncol 2022;19:515-33. [Crossref] [PubMed]
- Mabe NW, Huang M, Dalton GN, et al. Transition to a mesenchymal state in neuroblastoma confers resistance to anti-GD2 antibody via reduced expression of ST8SIA1. Nat Cancer 2022;3:976-93. [Crossref] [PubMed]
- Kasprowicz A, Sophie GD, Lagadec C, et al. Role of GD3 Synthase ST8Sia I in Cancers. Cancers (Basel) 2022;14:1299. [Crossref] [PubMed]
- Ohkawa Y, Zhang P, Momota H, et al. Lack of GD3 synthase (St8sia1) attenuates malignant properties of gliomas in genetically engineered mouse model. Cancer Sci 2021;112:3756-68. [Crossref] [PubMed]
- Valenti GE, Roveri A, Venerando R, et al. PTC596-Induced BMI-1 Inhibition Fights Neuroblastoma Multidrug Resistance by Inducing Ferroptosis. Antioxidants (Basel) 2023;13:3. [Crossref] [PubMed]
- Stockwell BR. Ferroptosis turns 10: Emerging mechanisms, physiological functions, and therapeutic applications. Cell 2022;185:2401-21. [Crossref] [PubMed]
- Wang Q, Li X, Cao Z, et al. Enzyme-Mediated Bioorthogonal Cascade Catalytic Reaction for Metabolism Intervention and Enhanced Ferroptosis on Neuroblastoma. J Am Chem Soc 2024;146:8228-41. [Crossref] [PubMed]
- Nunes C, Depestel L, Mus L, et al. RRM2 enhances MYCN-driven neuroblastoma formation and acts as a synergistic target with CHK1 inhibition. Sci Adv 2022;8:eabn1382. [Crossref] [PubMed]
- Li J, Pang J, Liu Y, et al. Suppression of RRM2 inhibits cell proliferation, causes cell cycle arrest and promotes the apoptosis of human neuroblastoma cells and in human neuroblastoma RRM2 is suppressed following chemotherapy. Oncol Rep 2018;40:355-60. [Crossref] [PubMed]
- Alborzinia H, Chen Z, Yildiz U, et al. LRP8-mediated selenocysteine uptake is a targetable vulnerability in MYCN-amplified neuroblastoma. EMBO Mol Med 2023;15:e18014. [Crossref] [PubMed]