Nomogram construction based on characteristic genes and clinical variables to predict the risk of multiple organ dysfunction syndrome caused by influenza in children
Highlight box
Key findings
• We established a predictive prolonged multiple organ dysfunction syndrome (MODS) nomogram model based on SLC12A7, GNA15, EMP1, and clinical variables (age, influenza virus type, and bacterial co-infection status) demonstrated better predictive performance for the risk of MODS in children with influenza.
What is known and what is new?
• Children, owing to their immature immune systems, are at high risk of developing MODS as a result of influenza, although the specific mechanisms involved remain unknown. Identifying risk factors for MODS caused by childhood influenza is a crucial step toward enhancing treatment interventions and prognosis stratification.
What is the implication, and what should change now?
• This study, which analyzed messenger ribonucleic acid in blood samples from children with influenza, identified characteristic genes in prolonged MODS samples, demonstrated the correlation between these characteristic genes and clinical variables, outlined the trends in expression level changes during the progression of MODS.
Introduction
Influenza is an acute respiratory infectious disease caused by influenza viruses, which has repeatedly led to outbreaks and pandemics worldwide (1-3), resulting in up to 650,000 deaths annually (4). According to the World Health Organization, the global annual incidence of childhood influenza ranges from 20% to 30% (5). During each influenza season, children under 5 years old account for nearly 900,000 hospitalizations and over 100,000 deaths (6,7).
Influenza typically manifests abruptly, and some cases progress to severe influenza due to complications such as pneumonia, with a minority experiencing rapid deterioration due to multi-organ failure, ultimately leading to death (8-11). Children, owing to their immature immune systems, are at high risk of developing multiple organ dysfunction syndrome (MODS) as a result of influenza, although the specific mechanisms involved remain incompletely understood (12-16).
Currently, the clinical prevention and control of MODS caused by influenza or severe influenza in children primarily depend on early identification through the analysis of risk factors in conjunction with clinical presentations. There is ongoing controversy regarding the criteria for diagnosing severe influenza in children, generally, cases that necessitate hospitalization, transfer to intensive care units (ICUs), or result in death are classified as severe influenza (17,18). However, these criteria exhibit heterogeneity and delays. Standards for hospitalization or ICU admission due to childhood influenza vary across regions. Furthermore, this diagnostic approach does not inform the development of prevention strategies or treatment plans. Identifying risk factors for MODS caused by childhood influenza is a crucial step toward enhancing treatment interventions and prognosis stratification.
Previous studies have preliminarily identified risk factors for severe influenza in children based on clinical variables. Principi et al. (14) noted that cases of severe influenza requiring hospitalization, ICU transfer, or resulting in death often occur in infants under 2 years old (seasonal influenza) and school-aged children (pandemic influenza). Wheezing and an increased neutrophil count may serve as risk factors for severe influenza in children (19). These risk factors have been identified from clinical features; however, their diagnostic efficacy and stability require further enhancement. Changes in gene expression levels offer a more objective foundation for predicting severe influenza in children. Researchers have identified that abnormal expression of the Mx1 gene, induced by interferon (IFN)-α/β, serves as a susceptibility factor for severe influenza in mice within an influenza model. Furthermore, it has been discovered that certain genes influencing the immune response of IFN-α/β and IFN-λ also contribute to the severity of influenza (20). Additionally, abnormalities in the IRF7 gene were identified through whole-genome sequencing in a case of a patient with severe influenza, further implicating its role in the immune function associated with IFN-α/β and IFN-λ, thereby suggesting that gene abnormalities are a contributing factor to severe influenza (20). Prior to this, a study reported a 2.5-year-old child with severe influenza who had a heterozygous mutation in IRF7 (21). Consequently, this study, which analyzed messenger ribonucleic acid (mRNA) in blood samples from children with influenza, identified characteristic genes in prolonged MODS samples, demonstrated the correlation between these characteristic genes and clinical variables, outlined the trends in expression level changes during the progression of MODS, and established a predictive nomogram model for prolonged MODS. We present this article in accordance with the TRIPOD reporting checklist (available at https://tp.amegroups.com/article/view/10.21037/tp-24-386/rc).
Methods
Data download
The study was conducted in accordance with the Declaration of Helsinki (as revised in 2013). We downloaded the pediatric influenza blood mRNA dataset (GSE236877) from the Gene Expression Omnibus (GEO) database (https://www.ncbi.nlm.nih.gov/geo). The GEO database is a gene expression database created and maintained by the National Center for Biotechnology Information (NCBI) in the United States. GEO has collected gene expression data from research institutions worldwide, encompassing the vast majority of diseases.
GSE236877 cohort included 38 prolonged MODS (PM) samples, of which 23 were retained. Twenty-seven recovered MODS (RM) samples and 126 never MODS (NM) samples. PM was defined as having MODS at the time of first blood collection plus one of the following: (I) MODS on/after PICU day 7; and/or (II) ECMO on/after PICU day 7; or (III) death while in hospital. RM was defined as having MODS at the time of first blood collection but survived with resolution of MODS by PICU day 7. Patients who never had MODS were assigned to the NM group.
The data were preprocessed for analysis. We converted the counts data format to fragments per kilobase of exon model per million mapped fragments (FPKM). We obtained the expression profile and downloaded the gff3 file. Subsequently, we extracted gene length data from the gff3 file. Based on the gene length and gene count data, we assessed the FPKM (Fragments Per Kilobase of transcript per Million mapped reads) for each gene, using the following conversion formula: FPKM = total exon reads/[mapped reads (Millions) × exon length (KB)]. We calculated the relative expression levels of all genes using the following formula: relative expression level = log2(FPKM + 1).
Differentially expressed genes (DEGs) screening
We used R software (version 3.5.1) and R packages (limma) to filter DEGs. The fold change (FC) was calculated as the ratio of the average gene expression in the PM samples to the average gene expression in the NM samples. The criteria for DEGs were set as |log2FC| >1 and a false discovery rate (FDR) <0.05.
Enrichment analysis
Based on the DEGs between PM and NM samples, Gene Ontology (GO) enrichment analysis and Kyoto Encyclopedia of Genes and Genomes (KEGG) enrichment analysis were conducted using the R software and the R package (clusterProfiler). We retained GO items and KEGG pathways with q values less than 0.05
Least absolute shrinkage and selection operator (LASSO) regression analysis
The principle of LASSO regression is to add a penalty term on top of least squares to compress the estimated coefficients. When the coefficients tend to zero under the penalty term, the variables with a significant impact on the dependent variable and their corresponding coefficient estimates can be selected. LASSO regression is commonly used to handle sample data with multicollinearity. The parameter λ determines the degree of compression of the penalty term in LASSO regression, with a larger λ resulting in a stronger impact of the penalty term. Through LASSO regression, variables included in the final model are all significantly correlated with the dependent variable (P<0.05) and consider the issue of variable multicollinearity. LASSO regression can avoid overfitting of parameters and is suitable for constructing diagnostic models with good performance but relatively few independent variables. We conducted LASSO regression analysis via R software and the R package (glmnet). We obtained the optimal lambda value within one standard error when the binomial separation constant was minimized. Based on this, feature variables with non-zero coefficients were selected as characteristic genes.
Principal component analysis (PCA)
PCA is an approximation of the covariance matrix used to explain and reduce the dimensionality of a dataset. By means of an orthogonal transformation, multiple indicators with certain correlations are transformed into principal components. These principal components are linear combinations of the original variables and are mutually uncorrelated. Kaiser-Meyer-Olkin (KMO) and Bartlett’s sphericity test are conducted on the data. We conducted PCA via R software and the R package (limma). In this study, PCA was conducted based on the expression levels of characteristic genes. Based on eigenvalues and cumulative variance contribution, principal components are extracted. The first and second principal components are selected to construct a two-dimensional coordinate system. Then, samples are marked in the coordinate system to assess the distinguishing ability of characteristic genes regarding the intrinsic properties of the samples, thereby indirectly validating the accuracy and reliability of the feature genes.
Statistical analysis
This study utilized R (V3.5.1) software for statistical analysis. Multiple logistic regression analyses were employed to screen for risk factors and independent risk factors, and to establish nomogram model. The receiver operating characteristic (ROC) curve was used to evaluate the predictive efficacy of variables on disease occurrence, where a larger area under the curve (AUC) indicates better predictive performance. Calibration curves and the Hosmer-Lemeshow goodness-of-fit test were utilized to describe whether the curves exhibited deviation. Decision curve analysis (DCA) was employed to assess the predictive efficacy of the model. Quantitative data that conform to a normal distribution are expressed as mean ± standard deviation, and intergroup comparisons are conducted using independent samples t-test. Quantitative data that do not conform to a normal distribution are presented as median (interquartile range) [M (Q1, Q3)], with intergroup comparisons performed using the Mann-Whitney U test. Categorical data are represented as the number of cases (percentage) and intergroup comparisons are assessed using the χ2 test. A two-tailed P value <0.05 was considered statistically significant.
Results
DEGs screening
The prolonged MODS and never MODS groups exhibited no statistically significant differences in terms of gender ratio, viral co-infections, and ethnicity. The never MODS group was characterized by an older age, a higher proportion of bacterial co-infections, and a greater incidence of influenza B infections (Table 1). Using log2|FC| >1 and FDR <0.05 as the screening criteria, a total of 77 DEGs were obtained between the NM and PM blood samples, with 40 genes down-regulated and 37 genes up-regulated in the PM samples (Figure 1A). KEGG enrichment analysis revealed significant enrichment of DEGs in pathways such as influenza A, IL-17 signaling pathway, coronavirus disease 2019 (COVID-19), nucleotide binding oligomerization domain (NOD)-like receptor signaling pathway, cytokine-cytokine receptor interaction (Figure 1B). GO enrichment analysis indicated that the DEGs were significantly enriched in biological process items such as defense response to virus, defense response to symbiont response to virus (Figure 1C). The DEGs were significantly enriched in cellular component items such as specific granule lumen, specific granule, cytoplasmic vesicle lumen (Figure 1C). The DEGs were significantly enriched in molecular function items such as double-stranded RNA binding, endopeptidase activity, cytokine activity (Figure 1C).
Table 1
Characteristic | Prolonged MODS (n=126) | Never MODS (n=38) | Statistical value | P value |
---|---|---|---|---|
Age (years) | 5.50 (1.70, 9.90) | 11.30 (6.25, 14.25) | 17.087 | <0.001†* |
Hours in PICU | 86.50 (52.25, 154.75) | 481.50 (282.75, 888.50) | 70.969 | <0.001†* |
Sex, male | 69 (54.76) | 24 (63.16) | 0.838 | 0.36‡ |
Viral co-infection | 24 (19.05) | 8 (21.05) | 0.075 | 0.79‡ |
Bacterial co-infection | 28 (22.22) | 26 (68.42) | 38.248 | <0.001‡* |
Shock requiring vasopressors | 11 (8.73) | 23 (60.53) | 47.662 | <0.001‡* |
Mechanical ventilation (invasive) | 71 (56.35) | 38 (100.00) | 24.957 | <0.001‡* |
ECMO | 2 (1.59) | 23 (60.53) | 78.496 | <0.001‡* |
Ethnicity | 1.260 | 0.74‡ | ||
Hispanic | 30 (23.81) | 7 (18.42) | 0.485 | 0.49‡ |
White or White-Hispanic | 96 (76.19) | 33 (86.84) | 1.973 | 0.16‡ |
Black or African American | 27 (21.43) | 6 (15.79) | 0.578 | 0.45‡ |
Previously healthy | 77 (61.11) | 26 (68.42) | 0.668 | 0.41‡ |
Influenza type | 14.914 | 0.005‡* | ||
A, H1 | 47 (37.30) | 8 (21.05) | ||
A, H3 | 35 (27.78) | 9 (23.68) | ||
B | 18 (14.29) | 16 (42.11) | ||
A+B | 4 (3.17) | 0 (0.00) | ||
Not subtyped | 22 (17.46) | 5 (13.16) |
Data are presented as n (%) or median (IQR). ‡, Chi-squared test; †, Mann-Whitney U test; *, statistical difference. MODS, multiple organ dysfunction syndrome; PICU, pediatric intensive care unit; ECMO, extracorporeal membrane oxygenation; IQR, interquartile range.
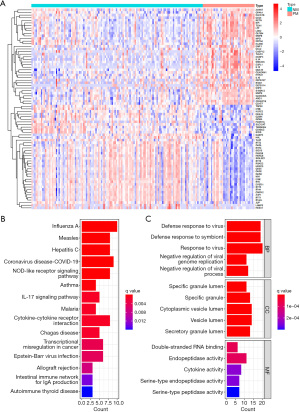
Characteristic genes screening
DEGs exhibited coefficient changes with parameter variation in LASSO regression, as shown in Figure 2A. The optimal lambda value within one standard error was obtained when the binomial separation constant was minimized. Based on this, 12 feature variables with non-zero coefficients (Figure 2B) were selected as characteristic genes, as listed in Table 2. PCA revealed that NM and PM were distributed in different regions in the plane formed by the first and second principal components, indicating good discriminative ability (Figure 2C). RM and PM were also distributed in different regions in the plane formed by the first and second principal components, showing good discriminative ability (Figure 2D). RM and NM, however, exhibited similar distributions in the plane formed by the first and second principal components (Figure 2E).
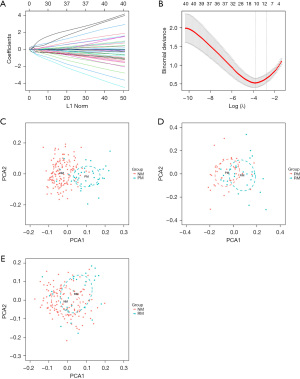
Table 2
Gene | Full name |
---|---|
LY86 | Lymphocyte antigen 86 |
TGFBI | Transforming growth factor beta induced |
SLC12A7 | Solute carrier family 12 member 7 |
HAL | Histidine ammonia-lyase |
CASP5 | Caspase 5 |
ESPL1 | Extra spindle pole bodies like 1 |
RETN | Resistin |
TULP2 | TUB like protein 2 |
DEFB114 | Defensin beta 114 |
EMP1 | Epithelial membrane protein 1 |
GNA15 | G protein subunit alpha 15 |
GPAA1 | Glycosylphosphatidylinositol anchor attachment 1 |
Comparison of gene expression levels of characteristic genes
The expression levels of TGFBI, SLC12A7, LY86 and HAL decreased successively in NM, RM and PM samples (Figure 3A-3D). The expression levels of CASP5 were the lowest in PM samples, lower than NM samples and RM samples (Figure 3E). The expression of RETN increased successively in NM, RM, and PM samples (Figure 3F). The expression levels of ESPL1, TULP2 and DEFB114 were the highest in PM samples, higher than NM samples and RM samples (Figure 3G-3I). The expressions of EMP1 and GNA15 in PM samples were higher than those in NM samples (Figure 3J,3K). There was no statistical significance in the difference of GPAA1 between each two of NM, RM and PM samples (Figure 3L).
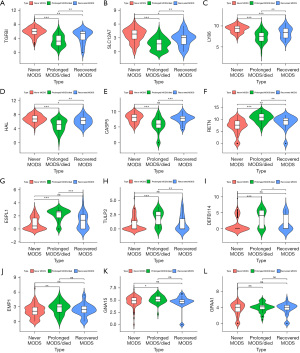
Correlation between characteristic genes and clinical features
In PM samples, the expression levels of RETN (R=−0.56, P<0.001) and ESPL1 (R=−0.38, P=0.02) were negatively correlated with neutrophil count (Figure 4A,4B). In the PM sample, CASP5 (R=0.47, P=0.004), LY86 (R=0.43, P=0.009), HAL (R=0.53, P<0.001), TGFBI (R=0.4, P=0.02) was positively correlated with neutrophil count (Figure 4C-4F). In PM samples, DEFB114, EMP1, GNA15, SLC12A7, TULP2, and GPAA1 did not show correlation due to neutrophil count (Figure 4G-4L). In PM samples, the expression levels of ESPL1 (R=−0.42, P=0.008) and RETN (R=−0.55, P<0.001) were negatively correlated with white blood cells (WBCs) count (Figure 5A,5B). In the PM sample, HAL (R=0.55, P<0.001), LY86 (R=0.52, P<0.001), TGFBI (R=0.50, P=0.001), CASP5 (R=0.47, P=0.003), EMP1 (R=0.33, P=0.04) were positively correlated with neutrophil count (Figure 5C-5G). In PM samples, GPAA1, TULP2, SLC12A7, GNA15, DEFB114 did not show correlation due to neutrophil count (Figure 5H-5L). In the PM sample, RETN (R=0.39, P=0.03) and ESPL1 (R=0.56, P=0.001) were positively correlated with pediatric sequential organ failure assessment (pSOFA) scores (Figure 6A,6B). CASP5 (R=−0.66, P<0.001) was negatively correlated with pSOFA score (Figure 6C). The remaining genes did not show a correlation with pSOFA scores (Figure 6D-6L).
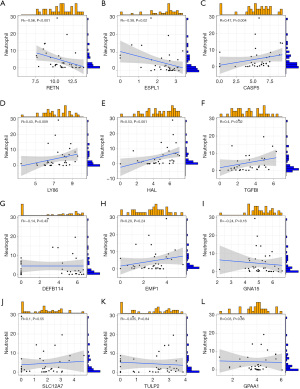
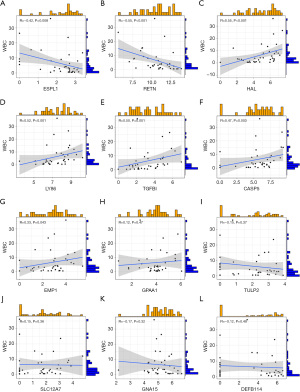
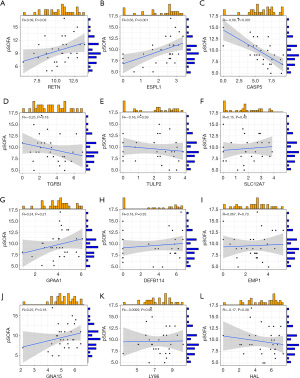
Diagnostic model construction
In order to predict the risk of PM, we performed multivariate Logistic regression analysis based on characteristic genes to screen independent predictors. SLC12A7 was an independent risk factor that increased the risk of PM (OR =0.356, P<0.001). GNA15 (OR =4.598, P<0.001) and EMP1 (OR =2.158, P=0.002) were protective factors that reduced the risk of PM occurrence (Table 3). The above three genes combined with clinical variables, including age, influenza virus type, and bacterial co-infection, to construct a nomogram model for predicting the risk of MODS in children with influenza (Figure 7A). The calibration curve showed that Bias-corrected curve fitted the ideal curve well, mean absolute error =0.032, mean squared error =0.00232, and 0.9 quantile of absolute error =0.088 (Figure 7B). ROC curve showed that the AUC of the nomogram score was 0.946, which was larger than the AUC of individual genes and clinical variables (Figure 7C). The DCA showed that using the nomogram model to predict the incidence of MODS can increase the net benefit of patients compared with clinical variables, intervention for all patients, and no intervention for all patients at the same threshold probability (Figure 7D).
Table 3
Gene | Coef | OR | 95% CI | P value | |
---|---|---|---|---|---|
OR.95L | OR.95H | ||||
SLC12A7 | −1.033 | 0.356 | 0.215 | 0.542 | <0.001 |
GNA15 | 1.526 | 4.598 | 2.293 | 11.069 | <0.001 |
EMP1 | 0.769 | 2.158 | 1.347 | 3.694 | 0.002 |
CI, confidence interval; OR, odds ratio; L, low; H, high.
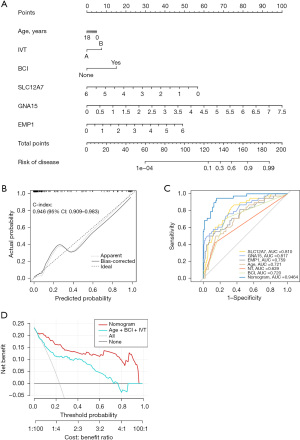
Discussion
We screened DEGs in normal (NM) and pathological (PM) samples. Enrichment analysis revealed that these differential genes were significantly associated with biological processes such as the defense response to viruses, the defense response to symbionts, and the response to viruses within the KEGG pathway of Influenza A. These biological processes and KEGG pathways are closely linked to viral infections, underscoring the representativeness, accuracy, and necessity for a comprehensive investigation of the screened differential genes. Furthermore, the enrichment analysis indicated that DEGs in NM and PM samples were notably enriched in immune-related functional processes and pathways, including cytokine activity, the IL-17 signaling pathway, the NOD-like receptor signaling pathway, and cytokine-cytokine receptor interactions.
These findings align with previous research and provide valuable insights into the mechanisms underlying MODS caused by influenza. The phenomenon known as the ‘cytokine storm’ and the excessive inflammatory response induced by influenza virus infection are significant contributors to high viral loads and increased mortality (22-25). Children are particularly susceptible to severe influenza, likely due to the immaturity of their innate immune systems. The incomplete development of immune recognition receptors results in impaired viral clearance, an excessive inflammatory response, and an increased risk of secondary MODS. These factors, whether acting independently or in concert, contribute to the incidence of severe influenza. One study (26) has demonstrated that 21-day-old mice infected with the influenza A virus are unable to clear the virus, fail to effectively recruit CD4+ T lymphocytes to the lungs, exhibit a diminished lung T lymphocyte response to the virus, and show reduced B lymphocyte antibody production. The decline in protein secretion adversely affects the production of cytokines such as interleukin (IL)-12, IFN-α, and IFN-γ, which are critical in the ongoing progression of the disease (26). Furthermore, IFN-γ production in the lungs of these mice is diminished throughout the course of the infection. Deficiencies in IFN-γ production, stemming from signal transducer and activator of transcription factors and transcription factor-4 within the IL-12 signaling pathway, suggest a maturation barrier in the signal transduction pathways of young animals. In macaques infected with the influenza A virus, researchers found that the upregulation of IFN-induced immune genes in individuals with severe infections was inhibited, affecting early innate immune functions, cell apoptosis, and antigen-presenting cell functions. This inhibition leads to ineffective viral clearance during the early stages of infection, an excessive inflammatory response, and late manifestations of multi-organ damage (27). In severe H1N1 infection in mice, the proportion and number of γδT17 cells among total γδT cells in lung tissues are significantly higher than those observed in the control group, as well as significantly higher than the levels of Th17 and Tc17 cells (28). This indicates that γδT17 cells are the primary infiltrating cells in the lungs during early severe H1N1 infection in mice, as opposed to Th17 cells and Tc17 cells. It is possible that γδT17 cells are activated in a γδTCR-independent manner and contribute to the early inflammatory damage in lung tissue during severe H1N1 infection by releasing IL-17A (29,30). Severe influenza frequently complicates bacterial infections, resulting in challenges for clinical treatment. One study has found that the influenza virus induces the production of type I IFN through the STAT1 signaling pathway, which inhibits the activation of type 17 immune responses, including IL-17 and IL-22. This inhibition increases susceptibility to methicillin-resistant Staphylococcus aureus (29).
To further elucidate the characteristics of PM and NM samples through mRNA analysis, we employed LASSO regression to eliminate redundant information and retain feature genes with the maximum explanatory power for the samples. A total of 12 feature genes were identified. PCA revealed that these feature genes could be distinctly distributed across the plane defined by the first and second principal components, effectively differentiating NM samples from PM samples, as well as RM samples from PM samples. This suggests that the 12 feature genes can accurately characterize the distinctions between PM and NM samples. However, these genes were not able to differentiate between NM and RM samples. In our correlation analysis, we observed that in PM samples, the expression levels of RETN and ESPL1 were negatively correlated with neutrophil count and WBC count, while exhibiting a positive correlation with the pSOFA score. Conversely, CASP5 displayed an opposite trend. These three genes may serve as potential biological markers for assessing the severity of the disease.
We selected three genes, SLC12A7, GNA15, and EMP1, as independent predictive factors for MODS through multi-factor logistic regression analysis of 12 candidate genes. We constructed a nomogram model by integrating these clinical variables with the identified independent predictive factors. The NM group was characterized by an older age, a higher proportion of bacterial co-infections, and a greater incidence of influenza B infections. These are the intrinsic characteristics of the patients, independent of immune responses and gene expression levels in the blood. In constructing the nomogram model, we incorporated the aforementioned three clinical features that exhibited group differences to enhance the model’s accuracy. The two groups also demonstrated differences in the use of vasopressors, extracorporeal membrane oxygenation (ECMO), mechanical ventilation, and duration of pediatric intensive care unit (PICU) stay; however, we consider these factors to be related to the progression of the condition. Consequently, we did not include them in the nomogram model at the later stages of the temporal axis. The clinical variables included age, concurrent bacterial infection, and influenza virus type, all of which have been demonstrated to be associated with severe influenza in children (31-34). Severe influenza in children frequently complicates with bacterial infections, particularly Streptococcus pneumoniae, and these two factors have a synergistic effect on inducing host immune responses (35-37). Influenza virus can create opportunities for pneumococcal infection through the following mechanisms: (I) by reducing the production of IFN-γ by T lymphocytes, influenza virus affects the phagocytosis of alveolar macrophages; (II) by reducing the production of IFN-I, influenza virus affects the aggregation of neutrophils; (III) influenza virus infection leads to an increase in serum cortisol levels, which can inhibit the inflammatory response against secondary pneumococcal infection (38). At the same time, a high load of pneumococcal carriage can also affect the prognosis of children with influenza. Vaccination against pneumococcus in children can to some extent reduce the possibility of secondary bacterial infection during severe influenza. It should be noted that in addition to Streptococcus pneumoniae infection, influenza is also prone to co-infections with Staphylococcus aureus, Streptococcus pyogenes, Haemophilus influenzae, and Neisseria meningitidis, but there is currently limited research on the interaction mechanisms among them (38).
The calibration curve indicates a good fit between the bias-corrected column chart curve and the ideal curve. The ROC curve shows that the AUC for the column chart score is 0.946, which is higher than the AUC for individual genes and clinical variables. The decision curve shows that, at the same threshold probability, the use of the nomogram model to predict the incidence of MODS can increase the net benefit for patients compared to using clinical variables, intervening in all patients, or not intervening in any patients. The column chart score performs better than clinical variables and individual genes in predicting MODS caused by childhood influenza.
There are still some limitations in this study. Firstly, whole genome data lacks the ability to identify cell subsets that may have an outsized impact on overall immune response. Secondly, external validation with an independent cohort is lacking, and further validation is needed to confirm the accuracy of the nomogram model. Thirdly, in terms of mechanism exploration, this study only conducted enrichment analysis based on DEGs, providing a preliminary picture, but further basic experiments are still needed for in-depth exploration. Lastly, we did not observe the change trend of gene expression levels over time.
Conclusions
TGFBI, SLC12A7, LY86, HAL, CASP5, RETN, ESPL1, TULP2, DEFB114, EMP1, GNA15, and GPAA1 were characteristic genes that distinguished between NM and PM samples. RETN and ESPL1 expression levels were negatively correlated with neutrophil count, WBC count, and positively correlated with pSOFA score, while CASP5 showed the opposite trend. SLC12A7, GNA15, and EMP1 can serve as independent predictive factors for MODS. The nomogram model based on SLC12A7, GNA15, EMP1, and clinical variables (age, influenza virus type, and bacterial co-infection status) demonstrated better predictive performance for the risk of MODS in children with influenza compared to clinical variables and single genes.
Acknowledgments
Funding: This work was supported by
Footnote
Reporting Checklist: The authors have completed the TRIPOD reporting checklist. Available at https://tp.amegroups.com/article/view/10.21037/tp-24-386/rc
Peer Review File: Available at https://tp.amegroups.com/article/view/10.21037/tp-24-386/prf
Conflicts of Interest: All authors have completed the ICMJE uniform disclosure form (available at https://tp.amegroups.com/article/view/10.21037/tp-24-386/coif). The authors have no conflicts of interest to declare.
Ethical Statement: The authors are accountable for all aspects of the work in ensuring that questions related to the accuracy or integrity of any part of the work are appropriately investigated and resolved. The study was conducted in accordance with the Declaration of Helsinki (as revised in 2013).
Open Access Statement: This is an Open Access article distributed in accordance with the Creative Commons Attribution-NonCommercial-NoDerivs 4.0 International License (CC BY-NC-ND 4.0), which permits the non-commercial replication and distribution of the article with the strict proviso that no changes or edits are made and the original work is properly cited (including links to both the formal publication through the relevant DOI and the license). See: https://creativecommons.org/licenses/by-nc-nd/4.0/.
References
- Uyeki TM. Influenza. Ann Intern Med 2021;174:ITC161-ITC176. [Crossref] [PubMed]
- Nypaver C, Dehlinger C, Carter C. Influenza and Influenza Vaccine: A Review. J Midwifery Womens Health 2021;66:45-53. [Crossref] [PubMed]
- Uyeki TM, Hui DS, Zambon M, et al. Influenza. Lancet 2022;400:693-706. [Crossref] [PubMed]
- Iuliano AD, Roguski KM, Chang HH, et al. Estimates of global seasonal influenza-associated respiratory mortality: a modelling study. Lancet 2018;391:1285-300. [Crossref] [PubMed]
- Somes MP, Turner RM, Dwyer LJ, et al. Estimating the annual attack rate of seasonal influenza among unvaccinated individuals: A systematic review and meta-analysis. Vaccine 2018;36:3199-207. [Crossref] [PubMed]
- Lafond KE, Nair H, Rasooly MH, et al. Global Role and Burden of Influenza in Pediatric Respiratory Hospitalizations, 1982-2012: A Systematic Analysis. PLoS Med 2016;13:e1001977. [Crossref] [PubMed]
- Nair H, Brooks WA, Katz M, et al. Global burden of respiratory infections due to seasonal influenza in young children: a systematic review and meta-analysis. Lancet 2011;378:1917-30. [Crossref] [PubMed]
- Hill D, Moreno MA. Influenza Prevention. JAMA Pediatr 2020;174:108. [Crossref] [PubMed]
- Influenza Barnett R. Lancet 2019;393:396. [Crossref] [PubMed]
- Influenza Brody H. Nature 2019;573:S49. [Crossref] [PubMed]
- Su J, Chen D, Zheng R, et al. Duvira Antarctic polysaccharide inhibited H1N1 influenza virus-induced apoptosis through ROS mediated ERK and STAT-3 signaling pathway. Mol Biol Rep 2022;49:6225-33. [Crossref] [PubMed]
- Nayak J, Hoy G, Gordon A. Influenza in Children. Cold Spring Harb Perspect Med 2021;11:a038430. [Crossref] [PubMed]
- Kondrich J, Rosenthal M. Influenza in children. Curr Opin Pediatr 2017;29:297-302. [Crossref] [PubMed]
- Principi N, Esposito S. Severe influenza in children: incidence and risk factors. Expert Rev Anti Infect Ther 2016;14:961-8. [Crossref] [PubMed]
- Kumar V. Influenza in Children. Indian J Pediatr 2017;84:139-43. [Crossref] [PubMed]
- Wolf RM, Antoon JW. Influenza in Children and Adolescents: Epidemiology, Management, and Prevention. Pediatr Rev 2023;44:605-17. [Crossref] [PubMed]
- Tsai CF, Liu YC, Chang TH, et al. The clinical predictors of and vaccine protection against severe influenza infection in children. J Med Virol 2023;95:e28638. [Crossref] [PubMed]
- Li-Kim-Moy J, Yin JK, Blyth CC, et al. Influenza hospitalizations in Australian children. Epidemiol Infect 2017;145:1451-60. [Crossref] [PubMed]
- Huang W, Niu W, Chen H, et al. Development of a nomogram for severe influenza in previously healthy children: a retrospective cohort study. J Int Med Res 2023;51:3000605231153768. [Crossref] [PubMed]
- Ciancanelli MJ, Abel L, Zhang SY, et al. Host genetics of severe influenza: from mouse Mx1 to human IRF7. Curr Opin Immunol 2016;38:109-20. [Crossref] [PubMed]
- Ciancanelli MJ, Huang SX, Luthra P, et al. Infectious disease. Life-threatening influenza and impaired interferon amplification in human IRF7 deficiency. Science 2015;348:448-53. [Crossref] [PubMed]
- Gu Y, Zuo X, Zhang S, et al. The Mechanism behind Influenza Virus Cytokine Storm. Viruses 2021;13:1362. [Crossref] [PubMed]
- Guo XJ, Thomas PG. New fronts emerge in the influenza cytokine storm. Semin Immunopathol 2017;39:541-50. [Crossref] [PubMed]
- Liu Q, Zhou YH, Yang ZQ. The cytokine storm of severe influenza and development of immunomodulatory therapy. Cell Mol Immunol 2016;13:3-10. [Crossref] [PubMed]
- Teijaro JR. The role of cytokine responses during influenza virus pathogenesis and potential therapeutic options. Curr Top Microbiol Immunol 2015;386:3-22. [Crossref] [PubMed]
- Verhoeven D, Perry S, Pryharski K. Control of influenza infection is impaired by diminished interferon-γ secretion by CD4 T cells in the lungs of toddler mice. J Leukoc Biol 2016;100:203-12. [Crossref] [PubMed]
- Muramoto Y, Shoemaker JE, Le MQ, et al. Disease severity is associated with differential gene expression at the early and late phases of infection in nonhuman primates infected with different H5N1 highly pathogenic avian influenza viruses. J Virol 2014;88:8981-97. [Crossref] [PubMed]
- Xue C, Wen M, Bao L, et al. Vγ4+γδT Cells Aggravate Severe H1N1 Influenza Virus Infection-Induced Acute Pulmonary Immunopathological Injury via Secreting Interleukin-17A. Front Immunol 2017;8:1054. [Crossref] [PubMed]
- Lee B, Gopal R, Manni ML, et al. STAT1 Is Required for Suppression of Type 17 Immunity during Influenza and Bacterial Superinfection. Immunohorizons 2017;1:81-91. [Crossref] [PubMed]
- Wang X, Lin X, Zheng Z, et al. Host-derived lipids orchestrate pulmonary γδ T cell response to provide early protection against influenza virus infection. Nat Commun 2021;12:1914. [Crossref] [PubMed]
- Zaraket H, Hurt AC, Clinch B, et al. Burden of influenza B virus infection and considerations for clinical management. Antiviral Res 2021;185:104970. [Crossref] [PubMed]
- Yazici Özkaya P, Turanli EE, Metin H, et al. Severe influenza virus infection in children admitted to the PICU: Comparison of influenza A and influenza B virus infection. J Med Virol 2022;94:575-81. [Crossref] [PubMed]
- Obermeier PE, Seeber LD, Alchikh M, et al. Incidence, Disease Severity, and Follow-Up of Influenza A/A, A/B, and B/B Virus Dual Infections in Children: A Hospital-Based Digital Surveillance Program. Viruses 2022;14:603. [Crossref] [PubMed]
- Esposito S, Molteni CG, Daleno C, et al. Clinical and socioeconomic impact of different types and subtypes of seasonal influenza viruses in children during influenza seasons 2007/2008 and 2008/2009. BMC Infect Dis 2011;11:271. [Crossref] [PubMed]
- Wang QY, Yuan L, Lin JY, et al. Clinical characteristics of severe influenza virus-associated pneumonia complicated with bacterial infection in children: a retrospective analysis. BMC Infect Dis 2023;23:545. [Crossref] [PubMed]
- Chien SJ, Hsieh YJ, Shih YL, et al. Clinical characteristics and outcomes of mixed virus or bacterial infection in children with laboratory-confirmed influenza infection. J Formos Med Assoc 2022;121:2074-84. [Crossref] [PubMed]
- Spaeder MC, Milstone AM, Fackler JC. Association of bacterial pneumonia and respiratory failure in children with community-acquired influenza infection. Pediatr Crit Care Med 2011;12:e181-3. [Crossref] [PubMed]
- Short KR, Habets MN, Hermans PW, et al. Interactions between Streptococcus pneumoniae and influenza virus: a mutually beneficial relationship? Future Microbiol 2012;7:609-24. [Crossref] [PubMed]