Exploring the predictive value of pH in stratified mortality risk of NEC patients undergoing surgery: a retrospective study based on the PIC database
Highlight box
Key findings
• Acidic pH levels (a marker of systemic acidosis) are independently associated with mortality in both surgical and non-surgical neonates with necrotizing enterocolitis (NEC).
• In surgical NEC patients, acidic pH emerged as a significant risk factor for adverse outcomes (adjusted odds ratio reported).
• Stratification by pH revealed the highest mortality rate in neonates with acidic pH compared to normal or alkaline subgroups.
• Beyond traditional acidosis drivers [e.g., lactate, potassium (K+)], elevated white blood cells (WBCs) and neutrophils were novel contributors to pH derangements.
What is known and what is new?
• Known: NEC is a leading cause of neonatal mortality, and systemic acidosis (reflected by low pH) correlates with disease severity.
• New: This study establishes pH as a prognostic marker across surgical/non-surgical subgroups and links inflammatory surges (WBC/neutrophils) to pH dynamics, proposing a previously underrecognized interplay between inflammation and acid-base imbalance in NEC.
What is the implication, and what should change now?
• Acidic pH should be integrated into early risk stratification tools for NEC mortality, particularly in surgical decision-making contexts. Clinicians should monitor inflammatory markers (WBC, neutrophils) alongside conventional acidosis indicators to refine prognostic models. Future research should validate pH-guided protocols and explore mechanisms connecting neutrophilic inflammation to pH alterations in NEC.
Introduction
Necrotizing enterocolitis (NEC) has a high mortality rate, reportedly reaching 17–20% (1,2). NEC is a common and devastating inflammatory gastrointestinal disease, representing a leading cause of death in neonatal intensive care units (NICUs), accounting for approximately 10% of all NICU mortality cases (3,4).
The pathogenesis of NEC is complex and multifactorial, involving gut dysbiosis, immune responses, ischemia-reperfusion injury, intestinal immaturity, and genetic predispositions (5). Researchers have extensively investigated various clinical factors related to these mechanisms (6). However, the role of acid-base imbalance in the prognosis of NEC has received insufficient attention. Dysbiosis of the gut microbiome can lead to the overgrowth of harmful bacteria, which produce metabolic byproducts such as lactic acid, creating an acidic environment (7,8). Patients with NEC also experience exaggerated immune and inflammatory responses, with inflammatory mediators potentially inducing increased production of acidic metabolic byproducts (9). Furthermore, ischemia-reperfusion injury not only disrupts intestinal function but also contributes to systemic acid-base imbalances (10).
The Pediatric Intensive Care (PIC) database (http://pic.nbscn.org), established by the National Center for Children’s Health Clinical Research, is a unique, single-center, bilingual, and large-scale dataset designed for pediatric critical care research (11). It encompasses clinical data for 12,881 critically ill children treated in the intensive care unit (ICU) from 2010 to 2018, including laboratory measurements, patient observation records during critical care, vital signs monitoring data from operating rooms, and structured symptom data extracted from patient hospitalization records.
This study retrospectively analyzed the clinical and laboratory data of patients with NEC from the PIC database. By stratifying these patients based on the type of surgical intervention received, the study explores the relationship between acid-base levels, particularly pH values, and the outcomes of neonates diagnosed with NEC. We present this article in accordance with the TRIPOD reporting checklist (available at https://tp.amegroups.com/article/view/10.21037/tp-2025-3/rc).
Methods
Inclusion and exclusion
This retrospective cohort study analyzed data from the PIC database (2010–2018), a single-center registry maintained by the National Center for Children’s Health Clinical Research. Diagnoses were recorded according to the International Classification of Diseases, 10th Revision (ICD-10). NEC was identified using the code P77.x00 in both admission and discharge records. Initially, 225 patients were included in this retrospective cohort study. After eliminating duplicate hospitalizations, 193 unique patients remained. The inclusion criteria required an age of less than 31 days at admission, resulting in the exclusion of 66 cases. Furthermore, 3 cases were excluded due to insufficient data resulting from hospitalization lasting less than 24 hours. A total of 124 patients were ultimately included in the analysis.
NEC diagnosis
Neonatal gastrointestinal diseases, including NEC, congenital megacolon, and congenital intestinal malrotation, often present with overlapping symptoms such as abdominal distension, bloody stools, vomiting, and diarrhea. For each neonate, we meticulously reviewed the primary symptoms as well as both admission and discharge diagnoses to confirm NEC as the primary diagnosis and to exclude other gastrointestinal diseases.
Outcome measures
Neonatal outcomes were classified into two categories: the discharge group and the mortality group. Records of who were neonates readmitted for a second hospitalization were excluded from this analysis. Only outcomes from the initial hospitalization were considered, with successful discharges categorized accordingly and NEC identified as the primary diagnosis in cases resulting in death. Mortality data were extracted as an endpoint from the ‘patients’ section of the PIC dataset.
Clinical data extraction
Access to the PIC database was granted upon successful completion of the Collaborative Institutional Training Initiative (CITI) program. Clinical data were extracted using unique hospitalization IDs from the PIC database. All included patients were directly admitted to this center (i.e., non-transferred cases), and only their first hospitalization was analyzed to ensure uniformity in the definition of ‘time of admission’. The parameters collected within the first 24 hours of admission included age at admission, admission weight, gender, treatment modality (surgical or non-surgical), and laboratory markers such as blood glucose, pH, albumin, total protein, C-reactive protein (CRP), creatinine, lactate, total bilirubin, fecal occult blood test (FOBT), hemoglobin, WBC, potassium (K+), sodium (Na+), and chloride (Cl−). This 24-hour window was selected to capture early physiological parameters potentially predictive of NEC, even if the definitive diagnosis was later confirmed during hospitalization.
The completeness rate for all predictor variables exceeded 80%. Missing values were imputed using the Generative Adversarial Imputation Network (GAIN), a machine learning model based on generative adversarial networks (GANs). Within this framework, a generator synthesizes plausible imputations by learning the underlying data distribution, while a discriminator assesses both the authenticity of the imputed values and the patterns of missing data. This dual process enhances robustness in clinical scenarios where the occurrence of missing data may correlate with unobserved severity. As demonstrated by Weinan Dong (12), GAIN outperforms traditional methods such as multiple imputation by chained equations (MICE) and missForest in terms of accuracy, effectiveness, and efficiency, even with missing rates of up to 50%. This innovative machine learning approach was utilized to address missing values in this study. The GAIN model was executed with the following hyperparameters: batch_size =128, hint_rate =0.9, alpha =100, and iterations =10,000.
Ethics approval and informed consent
The use of data from the PIC database was approved in accordance with its governance protocols. All data were de-identified following HIPAA standards, with protected health information permanently removed. Researchers accessing the database are bound by data security agreements to prevent re-identification. Given the fully anonymized and retrospective nature of this study, ethical approval and informed consent requirements were waived. The study was conducted in accordance with the Declaration of Helsinki and its subsequent amendments.
Statistical analysis
Statistical analyses were conducted using R version 4.4.1. Due to the limited sample size, continuous variables were expressed as median (Q25, Q75), and intergroup comparisons were conducted using either the Wilcoxon rank-sum test. Categorical variables were reported as frequencies and percentages, with intergroup comparisons performed using the chi-square test or Fisher’s exact test. Patients were stratified into surgical and non-surgical groups based on their treatment modality. Within each group, comparisons were made between the discharge and mortality subgroups. Laboratory variables that exhibited statistically significant differences were included in a multivariate regression analysis to construct predictive models. Results were reported as odds ratios (ORs) with 95% confidence intervals (CIs). Subsequently, patients were further stratified based on pH levels to compare mortality rates across pH groups. Utilizing pH as the dependent variable, stepwise generalized linear regression was performed to identify factors influencing pH changes. A P value of less than 0.05 was deemed statistically significant.
Results
Clinical data of all NEC patients
This retrospective cohort study included a total of 124 neonatal patients diagnosed with NEC, of whom 17 (8.7%) succumbed during hospitalization. The median age at admission to the ICU was 9 days [interquartile range (IQR): 3 to 19.5 days], and the median admission weight was 2.34 kg (IQR: 1.90 to 2.80 kg). The cohort consisted of 43 females and 81 males. Among these patients, 56 underwent surgical intervention, while 68 were managed non-surgically.
Comparison of clinical indicators in the surgical NEC group
Significant differences were observed in serum creatinine, pH, and lactate levels between the discharge and death groups. The pH level in the death group was 7.25 (7.19, 7.26), which was significantly lower than the 7.40 (7.34, 7.44) observed in the discharge group (P=0.001). Serum creatinine levels in the death group were 96.50 (91.00, 115.00) µmol/L, significantly higher than the 48.00 (36.00, 62.00) µmol/L recorded in the discharge group (P=0.002). Furthermore, lactate concentrations were markedly elevated in the death group, measuring 12.55 (6.20, 20.00) mmol/L, in contrast to 2.30 (1.48, 3.17) mmol/L in the discharge group. No statistically significant differences were observed in demographic parameters (age: P=0.52; weight: P=0.40) or cellular components of complete blood count (lymphocytes P=0.67, monocytes P=0.40, neutrophils P>0.99, platelets P=0.71). Similarly, glucose metabolism (P=0.82) and electrolyte profiles (Na+ P=0.73, K+ P=0.77, Cl− P=0.11) showed comparable results between groups (see Table 1).
Table 1
Variable | Discharge (n=52) | Death (n=4) | U/χ2 | P |
---|---|---|---|---|
Gender | <0.01 | >0.99 | ||
Female | 21 (40.38) | 2 (50.00) | ||
Male | 31 (59.62) | 2 (50.00) | ||
Prematurity | <0.01 | >0.99 | ||
No | 41 (78.85) | 3 (75.00) | ||
Yes | 11 (21.15) | 1 (25.00) | ||
Stool culture | <0.01 | >0.99 | ||
No | 44 (84.62) | 3 (75.00) | ||
Yes | 8 (15.38) | 1 (25.00) | ||
Age (days) | 7.50 (1.75, 19.25) | 4.50 (0.75, 11.00) | 124.5 | 0.52 |
Weight (kg) | 2.39 (1.88, 2.91) | 2.65 (2.45, 2.7) | 19 | 0.40 |
Lymphocyte (×109/L) | 3.27 (1.78, 4.94) | 3.58 (2.54, 4.97) | 90 | 0.67 |
Monocyte (×109/L) | 0.90 (0.66, 1.93) | 0.75 (0.36, 1.23) | 131 | 0.40 |
Neutrophils (×109/L) | 5.06 (2.89, 8.08) | 9.54 (1.65, 17.45) | 104 | >0.99 |
RDW | 15.80 (14.90, 17.32) | 15.20 (14.55, 16.88) | 127 | 0.47 |
RBC (×1012/L) | 3.85 (3.15, 4.58) | 3.50 (2.35, 4.46) | 127 | 0.47 |
WBC (×109/L) | 10.32 (7.23, 16.03) | 15.49 (6.43, 23.90) | 95 | 0.79 |
Hb (g/L) | 117.00 (98.50, 142.25) | 128.50 (83.00, 172.50) | 98.5 | 0.87 |
PCT (ng/mL) | 0.19 (0.03, 1.52) | 0.70 (0.08, 1.49) | 100 | 0.91 |
CRP (mg/L) | 13.50 (5.00, 36.75) | 17.50 (9.50, 30.25) | 99 | 0.89 |
Albumin (g/L) | 29.50 (25.93, 32.08) | 21.00 (19.15, 25.88) | 156 | 0.10 |
Lactate (mmol/L) | 2.30 (1.48, 3.17) | 12.55 (6.20, 20.00) | 11.5 | 0.003 |
Fibrinogen (g/L) | 1.67 (1.33, 2.03) | 2.32 (1.31, 3.23) | 84.5 | 0.55 |
Platelet (×109/L) | 259.00 (156.25, 321.75) | 282.50 (213.75, 347.75) | 92 | 0.71 |
Oxygen saturation (%) | 98.20 (92.40, 98.85) | 81.55 (76.42, 88.65) | 161 | 0.07 |
PO2 (mmHg) | 102.00 (63.00, 147.50) | 43.55 (36.77, 57.67) | 166 | 0.051 |
Total protein (g/L) | 43.35 (38.50, 48.17) | 30.20 (25.72, 38.20) | 161 | 0.07 |
Creatinine (μmol/L) | 48.00 (36.00, 62.00) | 96.50 (91.00, 115.00) | 6 | 0.002 |
Blood glucose (mmol/L) | 2.37 (1.91, 2.86) | 2.01 (1.82, 2.94) | 112 | 0.82 |
Na+ (mmol/L) | 137.00 (135.00, 140.00) | 137.50 (135.75, 139.75) | 92.5 | 0.73 |
K+ (mmol/L) | 4.10 (3.70, 4.50) | 4.15 (3.60, 4.83) | 94.5 | 0.77 |
Cl− (mmol/L) | 111.00 (108.00, 115.00) | 107.00 (104.25, 109.25) | 154.5 | 0.11 |
pH | 7.40 (7.34, 7.44) | 7.25 (7.19, 7.26) | 205 | 0.001 |
Data are presented as median (interquartile range) or n (%). U: Mann-Whitney U statistic (non-parametric test for between-group comparisons). Albumin: serum albumin concentration; CRP, C-reactive protein; Cl–, chloride; Hb, hemoglobin; K+, potassium; Na+, sodium; NEC, necrotizing enterocolitis; PO2, partial pressure of oxygen; PCT, procalcitonin; RDW, red cell distribution width; RBC, red blood cell count; WBC, white blood cell.
Comparison of clinical indicators in the non-surgical NEC group
In the non-surgical group, neonates were categorized into discharge and mortality groups. The pH values exhibited significant differences, with the mortality group showing lower pH levels 7.24 (7.12, 7.30) compared to the discharge group 7.37 (7.33, 7.42) (P<0.001). The red blood cell (RBC) counts in the mortality group 2.76 (2.43, 3.76) ×1012/L were significantly lower than those in the discharge group 3.86 (3.33, 4.47) ×1012/L (P=0.02). Albumin levels were also reduced in the mortality group 22.70 (19.87, 31.00) g/L compared to the discharge group 29.70 (25.70, 32.40) g/L (P=0.04). Furthermore, platelet counts were significantly lower in the mortality group 122.00 (42.00, 200.00) ×109/L compared to the discharge group 225.00 (152.50, 289.00) ×109/L (P=0.006). Other variables, including age (P=0.78), weight (P=0.19), RDW (P=0.058), lymphocytes (P=0.09), PCT (P=0.71), fibrinogen (P=0.56) and monocytes (P=0.09), did not show significant differences (see Table 2).
Table 2
Variable | Discharge (n=55) | Death (n=13) | U/χ2 | P |
---|---|---|---|---|
Gender | 0.05 | 0.83 | ||
Female | 17 (30.91) | 3 (23.08) | ||
Male | 38 (69.09) | 10 (76.92) | ||
Prematurity | 0.55 | 0.46 | ||
No | 42 (76.36) | 8 (61.54) | ||
Yes | 13 (23.64) | 5 (38.46) | ||
Stool culture | <0.01 | >0.99 | ||
No | 50 (90.91) | 12 (92.31) | ||
Yes | 5 (9.09) | 1 (7.69) | ||
Age (days) | 11.00 (5.00, 18.50) | 15.00 (0.00, 20.00) | 375.5 | 0.78 |
Weight (kg) | 2.31 (1.92, 3.00) | 2.10 (1.75, 2.60) | 407 | 0.19 |
RDW | 16.30 (15.25, 17.95) | 17.00 (16.70, 18.40) | 235.5 | 0.058 |
RBC (×1012/L) | 3.86 (3.33, 4.47) | 2.76 (2.43, 3.76) | 503 | 0.02 |
WBC (×109/L) | 11.15 (8.34, 14.28) | 8.59 (3.41, 12.09) | 456 | 0.13 |
Lymphocyte (×109/L) | 3.69 (1.71, 4.70) | 1.92 (1.00, 3.77) | 466 | 0.09 |
Monocyte (×109/L) | 1.11 (0.74, 1.65) | 0.43 (0.20, 1.20) | 466.5 | 0.09 |
Neutrophils (×109/L) | 4.66 (3.64, 7.33) | 5.34 (1.96, 13.43) | 341 | 0.80 |
CRP (mg/L) | 10.00 (4.00, 54.00) | 34.00 (4.00, 95.00) | 300.5 | 0.34 |
PCT (ng/mL) | 0.13 (0.03, 1.11) | 0.20 (0.03, 0.39) | 382 | 0.71 |
Albumin (g/L) | 29.70 (25.70, 32.40) | 22.70 (19.87, 31.00) | 490 | 0.04 |
Lactate (mmol/L) | 1.80 (1.25, 2.70) | 2.00 (1.50, 7.70) | 285 | 0.26 |
Fibrinogen (g/L) | 1.79 (1.46, 2.27) | 1.65 (1.21, 2.21) | 395 | 0.56 |
Platelet (×109/L) | 225.00 (152.50, 289.00) | 122.00 (42.00, 200.00) | 533 | 0.006 |
Oxygen saturation (%) | 97.80 (93.95, 99.00) | 95.00 (80.50, 98.40) | 436.5 | 0.22 |
PO2 (mmHg) | 84.50 (62.95, 122.50) | 69.60 (46.80, 84.80) | 453 | 0.14 |
Total protein (g/L) | 41.10 (37.85, 47.30) | 36.10 (26.50, 39.80) | 535 | 0.006 |
Hb (g/L) | 123.00 (105.50, 138.00) | 131.00 (90.00, 157.00) | 348 | 0.89 |
Creatinine (μmmol/L) | 53.00 (42.50, 70.00) | 63.50 (54.00, 68.00) | 309.5 | 0.46 |
Blood glucose (mmol/L) | 2.10 (1.48, 2.45) | 2.12 (1.11, 3.56) | 356 | 0.99 |
Na+ (mmol/L) | 136.00 (133.00, 139.00) | 132.00 (128.00, 136.00) | 509.5 | 0.02 |
K+ (mmol/L) | 4.00 (3.60, 4.45) | 4.40 (3.90, 4.90) | 265 | 0.15 |
Cl− (mmol/L) | 108.00 (105.00, 111.00) | 105.00 (101.00, 111.00) | 462.5 | 0.10 |
pH | 7.37 (7.33, 7.42) | 7.24 (7.12, 7.30) | 581.5 | <0.001 |
Data are presented as median (interquartile range) or n (%). U: Mann-Whitney U statistic (non-parametric test for between-group comparisons). Albumin, serum albumin concentration; CRP, C-reactive protein; Cl−, chloride; K+, potassium; Hb, hemoglobin; Na+, sodium; NEC, necrotizing enterocolitis; PCT, procalcitonin; PO2, partial pressure of oxygen; RDW, red cell distribution width; RBC, red blood cell count; WBC, white blood cell.
Construction and evaluation of multivariate regression models
Significant variables from the non-surgical group, including RBC, albumin levels, platelet count, total protein, and pH were incorporated into a multivariate logistic regression model to predict mortality outcomes (0= discharge, 1= death). Although Na+ levels differed between groups, these deviations were minor and thus excluded from the model. To accurately reflect the relationship between pH and mortality, pH values were dichotomized into acidic pH (pH<7.35, coded as 1) and non-acidic (coded as 0). Acidic pH was subsequently included in the regression model to evaluate its impact alongside other biomarkers. Acidic pH demonstrated the strongest association with mortality (coefficient =1.184), suggesting that an acidic pH environment significantly increases the risk of death (Table 3). Other variables exhibited weak associations, including RBC (coefficient =−0.571), albumin (0.064), platelet count (−0.008), and total protein (−0.075). Although some variables did not reach statistical significance, the findings underscore clinically relevant markers associated with mortality. The model’s predictive performance was visualized using a nomogram and evaluated with calibration and ROC curves, yielding an area under the curve (AUC) of 0.841 (95% CI: 0.694–0.987), with a good calibrated curve fit (Figures 1-3). Decision curve analysis (DCA) curves demonstrated that the predicted risk had a moderate clinical predictive value in the range of 1% to 83% (Figure 4).
Table 3
Variable | B | SE | Wald | P | OR | 95% CI |
---|---|---|---|---|---|---|
RBC (×1012/L) | −0.571 | 0.381 | 2.243 | 0.13 | 0.565 | 0.268–1.193 |
Albumin (g/L) | 0.064 | 0.093 | 0.469 | 0.49 | 1.066 | 0.888–1.279 |
Platelet (×109/L) | −0.008 | 0.004 | 3.305 | 0.07 | 0.992 | 0.983–1.001 |
Total protein (g/L) | −0.075 | 0.069 | 1.188 | 0.28 | 0.928 | 0.811–1.061 |
Acidic pH | 1.184 | 0.934 | 1.605 | 0.21 | 3.267 | 0.523–20.388 |
Const | 2.399 | 2.322 | 1.068 | 0.30 | 11.016 | – |
B, regression coefficient; OR, odds ratio; RBC, red blood cell count; SE, standard error; Wald, Wald statistic; 95% CI, 95% confidence interval.
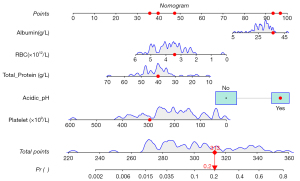
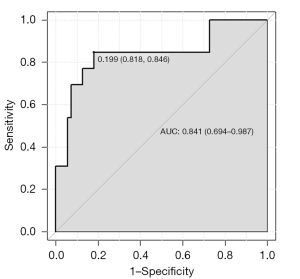
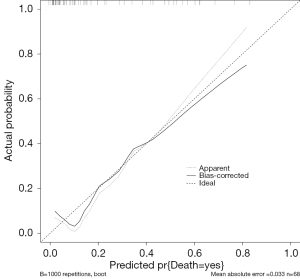
Mortality risk in different pH groups
Patients were categorized into acidic, normal, and alkaline pH groups to further investigate the relationship between pH levels and mortality. The Mortality rate was highest in the acidic group at 28.31%, which was significantly greater than the 3.92% observed in the normal pH group (P<0.001). Notably, no deaths were recorded in the alkaline pH group. These findings indicate a distinct association between pH levels and mortality risk in neonates with NEC (refer to Table 4 and Figure 5).
Table 4
pH level | Definition | Assigned value | Discharge | Death | χ2 | P |
---|---|---|---|---|---|---|
Acidic pH | pH <7.35 | 1 | 38 (71.69%) | 15 (28.31%) | 16.85 | <0.001 |
Normal pH | 7.35≤ pH ≤7.45 | 2 | 49 (96.08%) | 2 (3.92%) | ||
Alkaline pH | pH >7.45 | 3 | 20 (100%) | 0 |
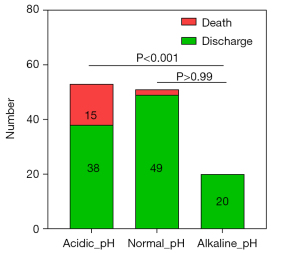
Exploring clinical factors influencing pH
A generalized linear regression model was developed to identify clinical factors affecting pH levels among patients with NEC. The model demonstrated a reasonable fit, with the following statistics: AIC =−247.30, BIC =−553.48, Deviance =0.85, and Pearson Chi-square =0.85. The regression equation is expressed as follows: pH = 6.9895 − 0.0041 × Neutrophils + 0.0052 × WBC − 0.0075 × Lactate + 0.0021 × Oxygen Saturation + 0.0005 × PO2 + 0.0049 × Total Protein − 0.0274 × K+ + 0.0018 × Age (Table 5). Key findings indicate that Neutrophils are negatively correlated with pH (P=0.005), suggesting that higher counts contribute to a reduction in pH. Conversely, WBC exhibited a positive correlation with pH (P<0.001), indicating that increased counts are associated with higher pH levels. Lactate also showed a negative correlation with pH (P<0.002), reaffirming the relationship between metabolic acidosis and pH decline. Additionally, oxygen saturation (P=0.006) and PO2 (P=0.02) were positively correlated with pH. Total protein (P<0.001) demonstrated a positive correlation, whereas K+ (P=0.02) exhibited a negative correlation. Age was positively correlated with pH (P=0.03). These results suggest that inflammation, metabolic acidosis, and electrolyte imbalances are critical factors influencing pH levels in neonates with NEC.
Table 5
Variable | Coefficient | SE | 95% CI | Wald Chi-square | P |
---|---|---|---|---|---|
Const | 6.9895 | 0.0998 | 6.7940 to 7.1851 | 4907.2391 | <0.001 |
Neutrophils (×109/L) | −0.0041 | 0.0015 | −0.0070 to −0.0012 | 7.7374 | 0.005 |
WBC (×109/L) | 0.0052 | 0.0014 | 0.0026 to 0.0079 | 14.9998 | <0.001 |
Lactate (mmol/L) | −0.0075 | 0.0024 | −0.0123 to −0.0028 | 9.5472 | 0.002 |
Oxygen saturation | 0.0021 | 0.0008 | 0.0006 to 0.0036 | 7.5183 | 0.006 |
PO2 (mmHg) | 0.0005 | 0.0002 | 0.0001 to 0.0010 | 5.4669 | 0.02 |
Total protein (g/L) | 0.0049 | 0.0009 | 0.0030 to 0.0067 | 26.9823 | <0.001 |
K+ (mmol/L) | −0.0274 | 0.0121 | −0.0510 to −0.0038 | 5.1581 | 0.02 |
Age (day) | 0.0018 | 0.0008 | 0.0002 to 0.0034 | 4.8966 | 0.03 |
Coefficient, regression coefficient; NEC, necrotizing enterocolitis; SE, standard error of the coefficient; 95% CI, 95% confidence interval.
Discussion
High mortality and complexity of NEC
NEC remains a severe condition characterized by a high mortality rate and diverse clinical presentations. Early identification of NEC is hindered by the absence of specific laboratory markers. The complex pathogenesis of NEC involves gut immune dysfunction, microbiota dysbiosis, oxidative stress, breastfeeding practices, transfusion, and inflammatory mediators (13). These mechanisms frequently result in acid-base disturbances, which play a critical role in the progression and fatal outcomes of NEC. Therefore, exploring the relationship between acid-base imbalances and NEC prognosis is essential for advancing our understanding in this area.
Role of acid-base imbalances in prognosis
Critically ill patients with NEC in intensive care often present with severe and multifaceted conditions, leading to high mortality rates. This study utilized the PIC database, a globally recognized clinical dataset for pediatric patients, to analyze outcomes and laboratory parameters of NEC patients (11).
The stratification of NEC patients into surgical and non-surgical groups aimed to isolate the impact of surgical intervention from disease severity on outcomes. The findings indicate that both surgical and non-surgical NEC patients who succumbed exhibited significantly acidic pH levels. Based on our data and previous studies, a pH threshold of <7.25, particularly when combined with elevated lactate, may serve as a pragmatic warning sign for clinicians to prioritize surgical evaluation. These results align with previous studies, such as those by Tefera et al., which reported that lower pH and elevated CRP levels increase the likelihood of requiring surgical intervention (14). Furthermore, other research has shown that patients with persistent metabolic acidosis are less likely to recover (15), while a 1 mmol/L increase in lactate is associated with a 40–45% rise in mortality risk (16). Collectively, these studies suggest that severe acidosis correlates with poorer outcomes in NEC patients.
Predictive model insights
This study developed a predictive model for non-surgical NEC patients, incorporating acidic pH alongside RBC, albumin, platelet count, and total protein. Although the model did not achieve statistical significance at α=0.05 due to a limited sample size, it demonstrated good discrimination and calibration. Acidic pH emerged as the strongest risk factor for mortality, emphasizing its clinical relevance (17,18).
Decreased RBC counts suggest anemia and impaired oxygen transport, which may exacerbate intestinal damage in preterm infants (19). Research indicates that reduced hemoglobin levels significantly increase the risk of NEC, underscoring the prognostic impact of anemia (20,21). Fluctuations in plasma proteins, including total protein and albumin, were associated with NEC outcomes (22). Interestingly, the opposing coefficients for total protein and albumin suggest that the albumin-to-total protein ratio warrants further exploration as a potential indicator. Additionally, platelet reduction was linked to poor prognosis, reflecting possible coagulopathy. Prior studies have identified thrombocytopenia as an independent prognostic factor in NEC, indicating more severe disease progression in patients with low platelet counts (23).
Stratification by pH and mortality risk
Stratifying patients by pH levels revealed the highest mortality rates among those with acidic pH, underscoring the prognostic significance of acid-base disturbances. Research conducted in pediatric intensive care units (PICU) indicates that metabolic acidosis occurs in 60.2% of critically ill children, demonstrating a significant correlation between the severity of acidosis and mortality (24). These findings emphasize the necessity of evaluating and addressing acid-base imbalances in the management of NEC.
Factors influencing pH changes
A generalized linear regression model identified several factors influencing pH levels, including lactate, oxygen saturation, PO2, total protein, K+, age, neutrophils, and WBC counts. Age exhibited a positive correlation with pH, likely reflecting the development and maturation of the neonatal respiratory system (23,25). WBC counts were also positively associated with pH, indicating the role of the systemic inflammatory response in mitigating acidosis. Conversely, lactate displayed a negative correlation with pH, underscoring its involvement in metabolic acidosis and adverse outcomes. Furthermore, oxygen saturation and PO2 positively influenced pH, highlighting the significance of oxygenation in maintaining acid-base balance (26). Neutrophils showed a negative association with pH, suggesting that inflammation may contribute to acid-base disturbances through oxidative stress and the release of inflammatory mediators (27,28).
Limitations
This study has several limitations: (I) retrospective design: the absence of perinatal factors in the analysis constrains the breadth of conclusions that can be drawn. Future research should stratify data according to perinatal variables, particularly gestational age. (II) Sample size: the limited sample size within the surgical and stratified subgroups restricted the statistical significance of the predictive models, although clinical relevance was maintained. (III) Single-center dataset: multi-center studies are essential to validate the findings and evaluate the prognostic value of pH across diverse clinical settings.
Conclusions
Acidic pH is strongly associated with adverse outcomes in patients with NEC. Inflammatory markers, such as neutrophils and WBC, may reflect the underlying changes that lead to acidic pH. This study enhances the understanding of the prognostic significance of pH in NEC and underscores the necessity for future research to visualize and elucidate the factors driving acid-base disturbances. The findings offer valuable insights for assessing mortality risks in NEC and guiding clinical management strategies.
Acknowledgments
We thank all the children and their guardians who participated in this study, and express gratitude to contributors for uploading their valuable datasets.
Footnote
Reporting Checklist: The authors have completed the TRIPOD reporting checklist. Available at https://tp.amegroups.com/article/view/10.21037/tp-2025-3/rc
Peer Review File: Available at https://tp.amegroups.com/article/view/10.21037/tp-2025-3/prf
Funding: This study was financially supported by
Conflicts of Interest: All authors have completed the ICMJE uniform disclosure form (available at https://tp.amegroups.com/article/view/10.21037/tp-2025-3/coif). The authors have no conflicts of interest to declare.
Ethical Statement: The authors are accountable for all aspects of the work in ensuring that questions related to the accuracy or integrity of any part of the work are appropriately investigated and resolved. Given the fully anonymized and retrospective nature of this study, ethical approval and informed consent requirements were waived. The study was conducted in accordance with the Declaration of Helsinki and its subsequent amendments.
Open Access Statement: This is an Open Access article distributed in accordance with the Creative Commons Attribution-NonCommercial-NoDerivs 4.0 International License (CC BY-NC-ND 4.0), which permits the non-commercial replication and distribution of the article with the strict proviso that no changes or edits are made and the original work is properly cited (including links to both the formal publication through the relevant DOI and the license). See: https://creativecommons.org/licenses/by-nc-nd/4.0/.
References
- Travers CP, Gentle S, Freeman AE, et al. A Quality Improvement Bundle to Improve Outcomes in Extremely Preterm Infants in the First Week. Pediatrics 2022;149:e2020037341. [Crossref] [PubMed]
- Raba AA, O'Sullivan A, Miletin J. Pathogenesis of necrotising enterocolitis: The impact of the altered gut microbiota and antibiotic exposure in preterm infants. Acta Paediatr 2021;110:433-40. [Crossref] [PubMed]
- Stoll BJ, Hansen NI, Bell EF, et al. Trends in Care Practices, Morbidity, and Mortality of Extremely Preterm Neonates, 1993-2012. JAMA 2015;314:1039-51. [Crossref] [PubMed]
- Feng J, Lee J, Vesoulis ZA, et al. Predicting mortality risk for preterm infants using deep learning models with time-series vital sign data. NPJ Digit Med 2021;4:108. [Crossref] [PubMed]
- Singh DK, Miller CM, Orgel KA, et al. Necrotizing enterocolitis: Bench to bedside approaches and advancing our understanding of disease pathogenesis. Front Pediatr 2022;10:1107404. [Crossref] [PubMed]
- Li QY, An Y, Liu L, et al. Differences in the Clinical Characteristics of Early- and Late-Onset Necrotizing Enterocolitis in Full-Term Infants: A Retrospective Case-Control Study. Sci Rep 2017;7:43042. [Crossref] [PubMed]
- Stanikova A, Jouza M, Bohosova J, et al. Role of the microbiome in pathophysiology of necrotising enterocolitis in preterm neonates. BMJ Paediatr Open 2023;7:e002172. [Crossref] [PubMed]
- Sang T, Wang Y. Intestinal microecology and neonatal necrotizing small bowel colitis. China Pediatric Emergency Medicine 2023;30:90-7.
- Zhang L, Sun L, Wu M, et al. Identification of Inflammatory Genes, Pathways, and Immune Cells in Necrotizing Enterocolitis of Preterm Infant by Bioinformatics Approaches. Biomed Res Int 2021;2021:5568724. [Crossref] [PubMed]
- Sánchez EC. Pathophysiology of ischemia-reperfusion injury and its management with hyperbaric oxygen (HBO): a review. J Emerg Crit Care Med 2019;3:22. [Crossref]
- Zeng X, Yu G, Lu Y, et al. PIC, a paediatric-specific intensive care database. Sci Data 2020;7:14. [Crossref] [PubMed]
- Dong W, Wan EYF, Fong DYT, et al. Development and validation of 10-year risk prediction models of cardiovascular disease in Chinese type 2 diabetes mellitus patients in primary care using interpretable machine learning-based methods. Diabetes Obes Metab 2024;26:3969-87. [Crossref] [PubMed]
- Zhang F. Progress in the pathogenesis of neonatal necrotizing enterocolitis. International Journal of Pediatrics 2020;2:116-9.
- Tefera BA, Ahmed AM, Yehualashet SS. Time to recovery from necrotizing enterocolitis and its predictors among neonates admitted to Neonatal Intensive Care Unit in Bahir Dar, Ethiopia: A retrospective follow up study, 2022. PLoS One 2024;19:e0311890. [Crossref] [PubMed]
- Zhou B, Tang J. Current status of research related to necrotizing small bowel colitis in neonates. Chinese Journal of Maternal and Child Clinical Medicine 2018;14:125-32. (electronic version).
- Wang Y, Lai L, Zhang Q, et al. Lactate acid level and prognosis of neonatal necrotizing enterocolitis: a retrospective cohort study based on pediatric-specific critical care database. J Pediatr (Rio J) 2023;99:278-83. [Crossref] [PubMed]
- Tenny S, Abdelgawad I. Statistical Significance. In: StatPearls [Internet]. Treasure Island (FL): StatPearls Publishing; 2025.
- Di Leo G, Sardanelli F. Statistical significance: p value, 0.05 threshold, and applications to radiomics-reasons for a conservative approach. Eur Radiol Exp 2020;4:18. [Crossref] [PubMed]
- Kalteren WS, Bos AF, van Oeveren W, et al. Neonatal anemia relates to intestinal injury in preterm infants. Pediatr Res 2022;91:1452-8. [Crossref] [PubMed]
- Maheshwari A, Patel RM, Christensen RD. Anemia, red blood cell transfusions, and necrotizing enterocolitis. Semin Pediatr Surg 2018;27:47-51. [Crossref] [PubMed]
- Saroha V, Josephson CD, Patel RM. Epidemiology of Necrotizing Enterocolitis: New Considerations Regarding the Influence of Red Blood Cell Transfusions and Anemia. Clin Perinatol 2019;46:101-17. [Crossref] [PubMed]
- Jiang YN, Muk T, Stensballe A, et al. Early Protein Markers of Necrotizing Enterocolitis in Plasma of Preterm Pigs Exposed to Antibiotics. Front Immunol 2020;11:565862. [Crossref] [PubMed]
- Ibrohim IS, Pratama HA, Fauzi AR, et al. Association between prognostic factors and the clinical deterioration of preterm neonates with necrotizing enterocolitis. Sci Rep 2022;12:13911. [Crossref] [PubMed]
- Datta M, Haldar S, Biswas A, et al. Metabolic acidosis as a predictor of outcome in critically ill children – a single-center prospective observational study. Journal of Pediatric Critical Care 2024;11:99-105. [Crossref]
- Lock JY, Carlson TL, Yu Y, et al. Impact of Developmental Age, Necrotizing Enterocolitis Associated Stress, and Oral Therapeutic Intervention on Mucus Barrier Properties. Sci Rep 2020;10:6692. [Crossref] [PubMed]
- Farwell WR, Taylor EN. Serum anion gap, bicarbonate and biomarkers of inflammation in healthy individuals in a national survey. CMAJ 2010;182:137-41. [Crossref] [PubMed]
- Kellum JA, Song M, Li J. Science review: extracellular acidosis and the immune response: clinical and physiologic implications. Crit Care 2004;8:331-6. [Crossref] [PubMed]
- Sekiya Y, Shimada K, Takahashi H, et al. Evaluation of a simultaneous adsorption device for cytokines and platelet-neutrophil complexes in vitro and in a rabbit acute lung injury model. Intensive Care Med Exp 2021;9:49. [Crossref] [PubMed]