Nomogram for prediction of in-hospital mortality rate in children with congenital heart disease in pediatric intensive care: establishment and external validation
Highlight box
Key findings
• Key risk factors for in-hospital mortality in children with congenital heart disease (CHD) in pediatric intensive care units include lymphocyte percentage, magnesium ion, neutrophil percentage, oxygen partial pressure, partial thromboplastin time, and ventricular septal defect repair surgery.
• A novel nomogram model has been developed using these independent predictors to predict in-hospital mortality in CHD patients, providing a new tool for clinical decision-making.
What is known and what is new?
• The nomogram demonstrated robust predictive performance with high area under the curves (AUCs) of 0.940, 0.857, and 0.776 for predicting 7-, 14-, and 30-day mortality in the training cohort, respectively.
• The model’s predictive accuracy was externally validated in the MIMIC-III database, with AUCs for 7-, 14-, and 30-day mortality being 0.732, 0.722, and 0.629, respectively, ensuring the model’s applicability in different datasets.
What is the implication, and what should change now?
• Neutrophil percentage emerged as the strongest predictor within the model. The calibration curves indicated a favorable consistency with actual outcomes, suggesting that the model offers clinically valuable predictive information.
Introduction
Congenital heart disease (CHD) is a global pediatric concern, affecting approximately 1% of newborns (1). In recent years, advancements in perinatal care, diagnosis, medical management, and surgical repair techniques have significantly improved the prognosis of affected children (2,3). However, the heterogeneity of CHD phenotypes and its complex, multifactorial etiology make it challenging to uncover precise pathogenesis, hindering prenatal interventions for CHD patients. Consequently, despite the advancements (4,5), the incidence of CHD has remained unchanged.
Additionally, CHD is a common reason for children to be admitted to the intensive care unit (ICU) (6). CHD children admitted to the ICU tend to have more severe and complex conditions, leading to relatively higher in-hospital mortality rates. However, there is limited research on risk factors for in-hospital mortality in CHD children, and no specific models have been developed to predict their in-hospital mortality rates. The in-hospital mortality rate among CHD patients may be influenced by various factors, such as heart rate, blood pressure, and oxygen saturation; however, the relative contributions of each factor remain unclear. Nomograms, as a visualization tool, can quantify the weights of variables in a model and calculate the probability of outcomes (7), greatly enhancing interpretability.
Therefore, this study aimed to identify risk factors for in-hospital mortality in CHD children in the ICU and develop a predictive nomogram model utilizing these risk factors to estimate the in-hospital mortality rate. We present this article in accordance with the TRIPOD reporting checklist (available at https://tp.amegroups.com/article/view/10.21037/tp-2024-506/rc).
Methods
Study population and data
The study population was derived from the paediatric intensive care unit (PICU) database. The PICU database is a large, pediatric-specific, single-center database maintained by the Laboratory for Computational Physiology at the Massachusetts Institute of Technology (8). This database contains information related to children admitted to the ICU of a large children’s hospital in China from 2010 to 2018, including vital sign measurements, medications, laboratory measurements, fluid balance records, diagnostic codes, demographic information, etc. (9). Patient information in the PICU database has been anonymized, eliminating the need for informed consent.
Inclusion criteria were as follows: (I) children diagnosed with CHD were diagnosed according to the International Classification of Diseases, ninth and tenth editions (ICD-9 and ICD-10); (II) intensive care treatment was required, with definitive admission to the ICU; (III) the medical record was complete, including follow-up data and all medical records from the hospital stay. Exclusion criteria were: (I) patients who were not being treated in an ICU; (II) lack of complete follow-up records to provide subsequent clinical data; (III) patients who died within 24 hours of birth; (IV) patients with shock; (V) age >12 years. For patients with multiple admissions, data at the first admission were used. The Medical Information Mart for Intensive Care III (MIMIC-III) dataset is consistent with the PICU dataset. The study was conducted in accordance with the Declaration of Helsinki and its subsequent amendments.
Patient characteristics
Structured query language (SQL) was used to extract patient demographics, comorbidities, surgical history, laboratory indicators, in-hospital mortality rates, and other data from the PICU database. Demographic information included gender, age in months; comorbidities included prematurity, low birth weight, respiratory failure (RF), sepsis, respiratory distress syndrome (RDS), pneumonia, intracranial haemorrhage; surgical history included ventricular septal defect (VSD) repair, atrial septal defect (ASD) repair, patent ductus arteriosus (PDA) repair (without extracorporeal circulation), tetralogy of Fallot repair, cardiac angiography, total anomalous pulmonary venous drainage (TAPVC); laboratory markers included adenosine deaminase, serum albumin-globulin ratio (ALB/GLB), albumin, alkaline phosphatase, albumin, alanine transaminase (ALT), amylase, anion gap, aspartate aminotransferase (AST), brain natriuretic peptide (BNP), base excess, basophils, direct bilirubin, indirect bilirubin, total bilirubin, calcium, bicarbonate, carboxyhemoglobin, chloride, creatine kinase MB (CKMB), creatine kinase, creatinine, cystatin C, D-dimer, eosinophils, fibrinogen, γ-glutamyltransferase, globulin, hematocrit, hemoglobin, lactate dehydrogenase, lactate, lymphocytes, magnesium ion, mean corpuscular hemoglobin (MCH), mean corpuscular hemoglobin concentration (MCHC), mean corpuscular volume (MCV), mean platelet volume, monocytes, neutrophils, oxygen saturation, partial pressure of carbon dioxide (pCO2), plateletcrit (PCT), pH, phosphate, platelet distribution width (RDW), platelet count, oxygen partial pressure (pO2), red blood cells, sodium, total cholic acid, triglycerides, urea, uric acid, and white blood cells (WBC). Variables with missing values exceeding 30% were excluded. If the patient had multiple admissions, the first admission was analysed. For laboratory indicators, data were extracted within 48 hours of the patient’s admission, and the first measurement was analysed if there were multiple measurements within 48 hours.
Statistical analysis
Normally distributed continuous variables were presented as mean ± standard deviation and were compared using the t-test, non-normally distributed continuous variables were presented as median with interquartile range and compared using the Mann-Whitney U test. Categorical variables were presented as frequencies and percentages, and between-group comparison was performed using Pearson’s Chi-squared test or Fisher’s exact test. To prevent overfitting, the total study cohort was randomly divided into a training cohort and a validation cohort. The training cohort was used for model training, and the validation cohort was used to validate the performance of the model. Variable selection was performed using single-factor Cox regression and stepwise Cox regression based on Akaike information criterion (AIC) in the training cohort. Variables with statistical significance in univariate analysis were included in a stepwise Cox regression. The significant variables from the stepwise Cox regression were then incorporated into the final Cox regression model and visualized as a nomogram. Calibration curves and receiver operator characteristic (ROC) curves were generated by comparing observed values with predicted values from the nomogram to assess the predictive performance of the constructed nomogram. To avoid multicollinearity between variables, variables with a variance inflation factor (VIF) ≥5 were excluded. All analyses were conducted using R 4.3.0, and a two-tailed P value <0.05 was considered statistically significant.
Results
Study population
A total of 2,274 children with CHD were identified from the PICU database. After excluding patients with missing data (n=34) and patients with a follow-up time <1 day (n=9), 2,231 patients were finally included in the analysis, with a mean age of 25.61 months. In the total study cohort, 77 (3.45%) patients died in the hospital. There were 1,070 male patients (48.0%) and 1,161 female patients (52.0%). The patients were randomly divided into a training cohort (n=1,562) and a validation cohort (n=669) at a 7:3 ratio. The two cohorts were comparable without statistically significant differences in patient characteristics (Table 1). A total of 601 children with congenital heart disease in the MIMIC-III dataset were included in the study.
Table 1
Factors | Overall (n=2,231) | Training set (n=1,562) | Validation set (n=669) | P value |
---|---|---|---|---|
Demographic | ||||
Gender (male), n (%) | 1,070 (48.0) | 752 (48.1) | 318 (47.5) | 0.83† |
Age, months, mean (SD) | 25.61 (33.50) | 24.83 (31.80) | 27.41 (37.14) | 0.10‡ |
Complications, n (%) | ||||
Premature | 76 (3.4) | 50 (3.2) | 26 (3.9) | 0.49† |
RF | 38 (1.7) | 31 (2.0) | 7 (1.0) | 0.16† |
Sepsis | 46 (2.1) | 31 (2.0) | 15 (2.2) | 0.82† |
RDS | 39 (1.7) | 28 (1.8) | 11 (1.6) | 0.95† |
Pneumonia | 57 (2.6) | 38 (2.4) | 19 (2.8) | 0.68† |
Low birth weight | 53 (2.4) | 35 (2.2) | 18 (2.7) | 0.63† |
Intraventricular hemorrhage | 34 (1.5) | 23 (1.5) | 11 (1.6) | 0.91† |
Therapy, n (%) | ||||
VSD repair closure | 960 (43.0) | 673 (43.1) | 287 (42.9) | 0.97† |
ASD repair closure | 767 (34.4) | 551 (35.3) | 216 (32.3) | 0.19† |
PDA closure | 272 (12.2) | 184 (11.8) | 88 (13.2) | 0.40† |
Tetralogy of Fallot repair | 74 (3.3) | 48 (3.1) | 26 (3.9) | 0.40† |
Angiocardiography | 37 (1.7) | 27 (1.7) | 10 (1.5) | 0.83† |
TAPVC repair | 16 (0.7) | 12 (0.8) | 4 (0.6) | 0.87† |
Laboratory data, mean (SD) | ||||
Adenosine deaminase, U/L | 14.17 (6.11) | 14.20 (6.03) | 14.10 (6.28) | 0.73‡ |
ALB/GLB | 2.16 (0.52) | 2.17 (0.52) | 2.14 (0.53) | 0.20‡ |
Albumin, g/L | 42.24 (5.37) | 42.29 (5.22) | 42.13 (5.71) | 0.51‡ |
Alkaline phosphatase, U/L | 267.11 (128.86) | 266.72 (128.11) | 268.01 (130.67) | 0.83‡ |
ALT, U/L | 24.46 (36.55) | 24.74 (40.93) | 23.80 (23.33) | 0.58‡ |
Amylase, U/L | 52.48 (129.29) | 51.57 (118.75) | 54.62 (151.13) | 0.61‡ |
Anion gap, mmol/L | 7.29 (4.85) | 7.36 (4.68) | 7.15 (5.23) | 0.36‡ |
AST, U/L | 56.97 (107.49) | 58.10 (117.63) | 54.33 (78.89) | 0.45‡ |
BNP, pg/mL | 3,219.47 (5,337.35) | 3,080.67 (5,065.80) | 3,543.54 (5,914.48) | 0.06‡ |
Base excess, mmol/L | −1.92 (3.40) | −1.88 (3.42) | −2.01 (3.34) | 0.41‡ |
Basophils, % | 0.50 (0.43) | 0.50 (0.44) | 0.51 (0.42) | 0.82‡ |
Bilirubin direct, μmol/L | 4.09 (7.14) | 4.02 (6.49) | 4.26 (8.46) | 0.46‡ |
Bilirubin indirect, μmol/L | 19.53 (40.22) | 19.13 (39.48) | 20.45 (41.91) | 0.48‡ |
Bilirubin total, μmol/L | 23.61 (44.76) | 23.15 (43.64) | 24.69 (47.28) | 0.46‡ |
Calcium total, mmol/L | 2.39 (0.19) | 2.39 (0.20) | 2.39 (0.18) | 0.76‡ |
Calculated bicarbonate, mmol/L | 22.21 (3.04) | 22.26 (3.00) | 22.10 (3.13) | 0.26‡ |
Carboxyhemoglobin, % | 1.09 (0.46) | 1.08 (0.46) | 1.11 (0.47) | 0.19‡ |
Chloride whole blood, mmol/L | 109.52 (4.71) | 109.51 (4.73) | 109.54 (4.67) | 0.88‡ |
Cholesterol total, mmol/L | 3.49 (1.08) | 3.50 (1.08) | 3.47 (1.09) | 0.56‡ |
Creatine kinase MB, U/L | 51.49 (102.37) | 51.87 (100.46) | 50.60 (106.76) | 0.79‡ |
Creatine kinase, U/L | 246.01 (500.49) | 247.85 (495.89) | 241.71 (511.42) | 0.79‡ |
Creatinine, μmol/L | 49.82 (20.50) | 49.83 (21.73) | 49.80 (17.29) | 0.98‡ |
Cystatin C, mg/L | 1.07 (0.39) | 1.06 (0.39) | 1.07 (0.37) | 0.89‡ |
D-dimer, mg/L | 0.92 (2.01) | 0.96 (2.22) | 0.84 (1.39) | 0.21‡ |
Eosinophils, % | 2.68 (2.26) | 2.68 (2.22) | 2.70 (2.34) | 0.86‡ |
Fibrinogen functional, g/L | 2.00 (0.60) | 2.00 (0.61) | 1.98 (0.59) | 0.50‡ |
Gamma glutamyl transferase, U/L | 38.07 (69.28) | 36.41 (63.08) | 41.96 (81.87) | 0.08‡ |
Globulin, g/L | 20.50 (5.10) | 20.40 (4.91) | 20.75 (5.51) | 0.13‡ |
Hematocrit, % | 37.83 (6.59) | 37.75 (6.58) | 38.02 (6.63) | 0.39‡ |
Hemoglobin, g/L | 124.20 (21.73) | 123.97 (21.59) | 124.73 (22.08) | 0.45‡ |
Lactate dehydrogenase, U/L | 403.13 (392.31) | 405.88 (398.21) | 396.70 (378.37) | 0.61‡ |
Lactate, mmol/L | 1.75 (1.98) | 1.74 (2.00) | 1.79 (1.93) | 0.60‡ |
Lymphocytes, % | 51.55 (16.89) | 51.50 (16.73) | 51.67 (17.26) | 0.83‡ |
Magnesium, mmol/L | 0.88 (0.12) | 0.88 (0.12) | 0.88 (0.11) | 0.54‡ |
MCH, pg | 28.10 (3.78) | 28.10 (3.75) | 28.10 (3.84) | 0.98‡ |
MCHC, g/L | 328.60 (14.15) | 328.73 (13.85) | 328.29 (14.82) | 0.50‡ |
MCV, fL | 85.44 (10.56) | 85.41 (10.52) | 85.52 (10.66) | 0.81‡ |
Mean platelet volume, fL | 9.46 (1.17) | 9.46 (1.18) | 9.47 (1.16) | 0.80‡ |
Monocytes, % | 6.77 (3.15) | 6.71 (3.04) | 6.89 (3.41) | 0.22‡ |
Neutrophils, % | 38.38 (16.88) | 38.48 (16.78) | 38.15 (17.13) | 0.67‡ |
Oxygen saturation, % | 95.01 (11.95) | 95.07 (12.02) | 94.88 (11.79) | 0.73‡ |
pCO2, mmHg | 37.64 (9.01) | 37.71 (9.37) | 37.48 (8.13) | 0.60‡ |
PCT, % | 0.30 (0.10) | 0.30 (0.10) | 0.30 (0.10) | 0.52‡ |
pH | 7.39 (0.07) | 7.39 (0.08) | 7.39 (0.07) | 0.58‡ |
Phosphate, mmol/L | 1.85 (0.52) | 1.85 (0.52) | 1.84 (0.50) | 0.55‡ |
Platelet count, 109/L | 322.52 (110.34) | 321.86 (107.90) | 324.08 (115.92) | 0.66‡ |
pO2, mmHg | 163.55 (76.91) | 164.79 (75.81) | 160.65 (79.42) | 0.24‡ |
Potassium, mmol/L | 3.74 (0.53) | 3.74 (0.54) | 3.75 (0.52) | 0.75‡ |
Prealbumin, g/L | 0.15 (0.05) | 0.15 (0.04) | 0.15 (0.05) | 0.85‡ |
Protein total, g/L | 62.74 (8.70) | 62.67 (8.55) | 62.88 (9.05) | 0.60‡ |
PTT, s | 36.75 (12.73) | 36.90 (12.90) | 36.42 (12.31) | 0.42‡ |
RDW, % | 14.12 (2.22) | 14.13 (2.25) | 14.11 (2.17) | 0.85‡ |
Red blood cells, 1012/L | 4.45 (0.69) | 4.44 (0.70) | 4.47 (0.68) | 0.39‡ |
Sodium whole blood, mmol/L | 135.50 (3.87) | 135.55 (3.93) | 135.39 (3.74) | 0.39‡ |
Total bile acid, μmol/L | 10.71 (13.52) | 10.56 (13.38) | 11.07 (13.85) | 0.41‡ |
Triglycerides, mmol/L | 1.34 (0.89) | 1.32 (0.83) | 1.38 (1.02) | 0.17‡ |
Urea, mmol/L | 3.96 (1.68) | 3.98 (1.71) | 3.93 (1.60) | 0.52‡ |
Uric acid urine, μmol/L | 269.68 (106.28) | 270.35 (107.62) | 268.11 (103.14) | 0.65‡ |
WBC count, 109/L | 10.02 (5.01) | 10.01 (4.75) | 10.04 (5.58) | 0.91‡ |
†, Chi-squared test; ‡, Mann-Whitney U test. ALB/GLB, serum albumin-globulin ratio; ALT, alanine aminotransferase; ASD, atrial septal defect; AST, aspartate aminotransferase; BNP, B-type natriuretic peptide; MCH, mean corpuscular hemoglobin; MCHC, mean corpuscular hemoglobin concentration; MCV, mean corpuscular volume; pCO2, partial pressure of carbon dioxide; PCT, procalcitonin; PDA, patent ductus arteriosus; pO2, oxygen partial pressure; PTT, partial thromboplastin time; RDS, respiratory distress syndrome; RDW, red cell distribution width; RF, respiratory failure; SD, standard deviation; TAPVC, total anomalous pulmonary venous drainage; VSD, ventricular septal defect; WBC, white blood cell count.
Model building
In-hospital mortality was used as the outcome measure. Feature selection was performed through single-factor Cox regression and stepwise Cox regression (Table 2). According to the single-factor Cox regression, such variables as patient age, albumin, amylase, ASD repair, AST, base excess, direct bilirubin, indirect bilirubin, total bilirubin, BNP, calcium ion, chloride ion, total cholesterol, creatine kinase, creatinine, cystatin C, D-dimer, eosinophils, fibrinogen, glutamyltransferase, globulin, hematocrit, hemoglobin, lactate, lactate dehydrogenase, lymphocytes, magnesium ion, MCH, MCHC, MCV, neutrophils, oxygen saturation, pCO2, PCT, pH, phosphate, platelet count, PDW, pO2, potassium, prealbumin, total protein, partial thromboplastin time (PTT), TAPVC repair, VSD repair showed statistical significance and were considered as potential predictive factors. These variables were further screened using stepwise Cox regression. Lymphocyte percentage [hazard ratio (HR): 1.097, 95% confidence interval (CI): 1.038–1.160], magnesium ion (HR: 1.002, 95% CI: 1.001–1.002), neutrophil percentage (HR: 1.111, 95% CI: 1.050–1.175), pO2 (HR: 0.987, 95% CI: 0.981–0.993), PTT (HR: 1.033, 95% CI: 1.020–1.047), and VSD repair (HR: 0.117, 95% CI: 0.028–0.494) were identified as statistically significant predictors of patient prognosis. These variables were included in the final Cox proportional hazards model and visualized as a nomogram (Figure 1). At the same time, we extracted the variables from the column-line diagrams in the MIMIC-III database for external validation. The total points on the nomogram were the sum of the scores assigned to each variable and vertically corresponded to the scales on the predictor (7-, 14-, and 30-day), which represent the survival probability of patients at specific time intervals.
Table 2
Factors | Univariate analysis | Stepwise Cox regression | |||
---|---|---|---|---|---|
HR (95% CI) | P value | HR (95% CI) | P value | ||
Adenosine deaminase | 1.003 (0.961–1.047) | 0.90 | |||
Age | 0.898 (0.853–0.946) | <0.001 | |||
ALB/GLB | 0.924 (0.559–1.528) | 0.79 | |||
Albumin | 0.882 (0.850–0.916) | <0.001 | |||
Alkaline phosphatase | 0.998 (0.995–1.000) | 0.10 | |||
ALT | 1.002 (1.000–1.005) | 0.07 | |||
Amylase | 0.970 (0.956–0.985) | <0.001 | |||
Angiocardiography | 2.456 (0.861–7.005) | 0.09 | |||
Anion gap | 1.010 (0.956–1.066) | 0.73 | |||
ASD repair closure | 0.235 (0.093–0.592) | 0.002 | |||
AST | 1.001 (1.000–1.002) | 0.02 | |||
Base excess | 0.903 (0.857–0.951) | <0.001 | |||
Basophils | 0.667 (0.282–1.577) | 0.37 | |||
Bilirubin direct | 1.027 (1.013–1.041) | <0.001 | |||
Bilirubin indirect | 1.008 (1.005–1.012) | <0.001 | |||
Bilirubin total | 1.008 (1.005–1.011) | <0.001 | |||
BNP | 1.000 (1.000–1.000) | <0.001 | |||
Calcium total | 0.204 (0.118–0.352) | <0.001 | |||
Calculated bicarbonate | 0.984 (0.906–1.068) | 0.70 | |||
Carboxyhemoglobin | 0.736 (0.418–1.298) | 0.29 | |||
Chloride whole blood | 0.925 (0.889–0.962) | <0.001 | |||
Cholesterol total | 0.579 (0.456–0.736) | <0.001 | |||
Creatine kinase | 1.000 (1.000–1.000) | 0.01 | |||
Creatine kinase MB | 1.001 (0.999–1.002) | 0.26 | |||
Creatinine | 1.007 (1.004–1.011) | <0.001 | |||
Cystatin C | 2.960 (2.044–4.285) | <0.001 | |||
D-dimer | 1.100 (1.054–1.148) | <0.001 | |||
Eosinophils | 0.777 (0.645–0.936) | 0.009 | |||
Fibrinogen functional | 0.323 (0.186–0.562) | <0.001 | |||
Gamma glutamyl transferase | 1.003 (1.001–1.006) | 0.01 | |||
Gender (male) | 1.310 (0.759–2.259) | 0.33 | |||
Globulin | 0.893 (0.843–0.947) | <0.001 | |||
Hematocrit | 1.048 (1.018–1.079) | 0.002 | |||
Hemoglobin | 1.013 (1.003–1.022) | 0.01 | |||
Intraventricular hemorrhage | 0 (0–Inf) | >0.99 | |||
Lactate | 1.199 (1.142–1.259) | <0.001 | |||
Lactate dehydrogenase | 1.000 (1.000–1.001) | <0.001 | |||
Low birth weight | 0 (0–Inf) | >0.99 | |||
Lymphocytes percent | 0.958 (0.944–0.972) | <0.001 | 1.097 (1.038–1.160) | 0.001 | |
Magnesium | 1.002 (1.001–1.003) | <0.001 | 1.002 (1.001–1.002) | <0.001 | |
MCH | 1.123 (1.062–1.188) | <0.001 | |||
MCHC | 0.979 (0.965–0.994) | 0.007 | |||
MCV | 1.049 (1.030–1.069) | <0.001 | |||
Mean platelet volume | 0.941 (0.753–1.174) | 0.59 | |||
Monocytes | 0.960 (0.881–1.047) | 0.35 | |||
Neutrophils % | 1.044 (1.030–1.059) | <0.001 | 1.111 (1.050–1.175) | <0.001 | |
Oxygen saturation | 0.959 (0.948–0.971) | <0.001 | |||
pCO2 | 1.037 (1.025–1.048) | <0.001 | |||
PCT | 0.004 (0.000–0.060) | <0.001 | |||
PDA closure | 1.137 (0.534–2.421) | 0.74 | |||
pH | 0.001 (0.000–0.010) | <0.001 | |||
Phosphate | 1.891 (1.525–2.345) | <0.001 | |||
Platelet count | 0.995 (0.993–0.998) | <0.001 | |||
Platelet distribution width | 1.163 (1.059–1.278) | 0.002 | |||
Pneumonia | 0 (0–Inf) | >0.99 | |||
pO2 | 0.981 (0.976–0.986) | <0.001 | 0.987 (0.981–0.993) | <0.001 | |
Potassium | 2.150 (1.534–3.015) | <0.001 | |||
Prealbumin | 0.000 (0.000–0.000) | <0.001 | |||
Premature | 0 (0–Inf) | >0.99 | |||
Protein total | 0.927 (0.905–0.949) | <0.001 | |||
PTT | 1.032 (1.024–1.040) | <0.001 | 1.033 (1.020–1.047) | <0.001 | |
RDS | 0 (0–Inf) | >0.99 | |||
RF | 0 (0–Inf) | >0.99 | |||
Sepsis | 0 (0–Inf) | >0.99 | |||
TAPVC repair | 8.837 (3.172–24.623) | <0.001 | |||
Tetralogy of Fallot repair | 0.714 (0.172–2.958) | 0.64 | |||
VSD repair closure | 0.052 (0.013–0.216) | <0.001 | 0.117 (0.028–0.494) | 0.004 |
ALB/GLB, serum albumin-globulin ratio; ALT, alanine aminotransferase; ASD, atrial septal defect; AST, aspartate aminotransferase; BNP, B-type natriuretic peptide; CI, confidence interval; HR, hazard ratio; MCH, mean corpuscular hemoglobin; MCHC, mean corpuscular hemoglobin concentration; MCV, mean corpuscular volume; pCO2, partial pressure of carbon dioxide; PCT, procalcitonin; PDA, patent ductus arteriosus; pO2, oxygen partial pressure; PTT, partial thromboplastin time; RDS, respiratory distress syndrome; RF, respiratory failure; TAPVC, total anomalous pulmonary venous drainage; VSD, ventricular septal defect; WBC, white blood cell count.
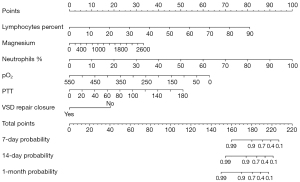
Model validation
ROC curves and calibration curves were plotted to validate the performance of the model. The ROC curve analysis showed that the model had a favorable discriminative ability with AUCs of 0.940, 0.857, and 0.776 for predicting in-hospital mortality at 7, 14, and 30 days, respectively, in the training cohort, and AUCs of 0.921, 0.858, and 0.699 in the validation cohort (Figure 2). The calibration curves demonstrated good consistency of the model without significant over- or underestimation of patient mortality risk (Figure 3). In the external dataset, the AUC of the model for predicting 7-, 14-, and 30-day in-hospital mortality were 0.732, 0.722, and 0.629, respectively (Figure 4A). The calibration curve also shows equally good consistency (Figure 4B). The model exhibited excellent predictive performance and could provide adequate prognostic information.


Discussion
The final model constructed in this study included six predictive factors: lymphocyte percentage, magnesium ion, neutrophil percentage, pO2, PTT, and VSD repair. These indicators in the model are clinically accessible and simple. The analysis of ROC curves and calibration curves revealed that the model had favorable predictive performance and could provide effective prognostic information to aid in clinical decision making.
Among the significant predictive factors, our study results indicated that elevated levels of lymphocyte percentage (HR: 1.097, 95% CI: 1.038–1.160, P=0.0010), neutrophil percentage (HR: 1.111, 95% CI: 1.050–1.175, P=0.0002), magnesium ion (HR: 1.002, 95% CI: 1.001–1.002, P<0.0001), and PTT (HR: 1.033, 95% CI: 1.020–1.047, P<0.0001) significantly increased the risk of in-hospital mortality in CHD patients. Based on the results of this study, elevated neutrophil percentages, lymphocyte percentages, and decreased partial pO2 were found to be significantly associated with the risk of death in children with CHD. Specifically, elevated neutrophil percentages may reflect the body’s stress response to inflammation or infection, which is particularly common in children with congenital heart disease in intensive care. As the main effector cells of the inflammatory response, an increase in the percentage of neutrophils often signals an increase in the inflammatory state, which in turn leads to deterioration of cardiac function and poor prognosis (10). In addition, an elevated percentage of lymphocytes likewise reveals a poor prognosis. This may be related to the role of lymphocytes in the immune response. Although lymphocytes play an important role in normal immune function, their abnormal elevation may indicate the presence of chronic inflammation or infection, which negatively affects the survival of children with congenital heart disease (11,12). In addition, the results also showed that pO2 (HR: 0.987, 95% CI: 0.981–0.993, P<0.0001) and VSD repair surgery (HR: 0.117, 95% CI: 0.028–0.494, P=0.0035) were significant protective factors for in-hospital mortality. In patients with CHD, pO2 reflects the oxygen content in the blood and provides information about the patient’s oxygenation status (13). For CHD patients, a lower pO2 than the normal range indicates worsening cardiac condition. Furthermore, high-oxygen therapy is considered a potential prenatal intervention for genetic heart abnormalities. The study by Doll et al. has shown that intermittent mild hyperoxia during pregnancy can partially rescue genetic heart abnormalities in mouse models (14). A decrease in the partial pO2, on the other hand, is a direct reflection of the deterioration of the oxygenation status of the child. Our findings suggest that low pO2 is significantly associated with poor prognosis in children with CHD in the ICU. This finding is consistent with previous studies that hypoxemia may be a manifestation of acute RDS, while hypoxemia may increase the risk of death in children by triggering systemic inflammatory response and multi-organ dysfunction (15). In addition, low pO2 may further exacerbate cardiac load and lead to deterioration of cardiac function, thus creating a vicious circle (16,17). Therefore, early recognition and correction of hypoxemia are essential to improve the prognosis of children with CHD. Magnesium, the most abundant intracellular divalent cation, plays a key role in regulating neuronal excitation, cardiac conduction, and myocardial contraction by modulating various ion transporters, including potassium and calcium channels, in the heart. Therefore, magnesium may have significant implications for the pathogenesis and progression of cardiovascular diseases (18). Some studies have indicated that intraoperative magnesium intake can reduce the risk of postoperative arrhythmias in congenital heart surgery (19,20), but have not shown a direct effect of elevated magnesium ions in the blood on patient mortality. In our study cohort, the mean and standard deviation of blood magnesium ions were 0.88 (0.12) mmol/L, and there were 175 patients (7.84% of the total cohort) with higher magnesium ion levels (>1.0 mmol/L). This suggested that the blood magnesium levels in the majority of patients were within the normal range. The elevation of blood magnesium ions may be attributed to prophylactic intake during or before surgery, and patients who require magnesium intake for arrhythmia prevention have a higher risk of mortality. However, the possibility that higher than normal magnesium levels increase the risk of mortality in patients with CHD cannot be ruled out. Furthermore, the results indicated that an elevated PTT significantly increased the risk of patient mortality. High PTT levels are typically caused by deficiencies in clotting factors or excessive anticoagulant factors (21). Patients with CHD may experience two types of coagulation disorders. On one hand, these patients may develop secondary erythrocytosis, leading to increased blood viscosity and a higher risk of thrombus formation. On the other hand, the reduced plasma volume results in severe deficiencies of various clotting proteins, including platelets, fibrinogen, and other clotting factors, which increases the risk of perioperative bleeding (22). Therefore, monitoring the coagulation status during the perioperative period is crucial for CHD patients.
In this study, we utilized a nomogram to visualize the Cox proportional hazards model. The nomogram is intuitive, capable of presenting multivariable relationships, and easy to calculate. By presenting the relationships between multiple variables graphically, the nomogram makes complex mathematical relationships visually accessible and easily understood, eliminating the need for users to delve into mathematical details while extracting information from the nomogram. Additionally, it enables the display of relationships among multiple variables, facilitating the analysis of multifactorial influences. Within the nomogram, total points can be calculated by simply adding the points for each variable, allowing for the estimation of patients’ survival probabilities at different time intervals without complex calculations (23). Compared with complex machine learning models, the nomogram is highly interpretable (24).
In summary, our model exhibits strong interpretability, ease of use, data accessibility, and favorable predictive performance. However, there are several limitations in this study. Firstly, our model was established based on a single-center database from China, and its applicability to populations with significant genetic differences may be limited. Second, due to the restrictive nature of ICD-9 and ICD-10 codes, some diseases cannot be clearly distinguished, such as ASD and patent foramen ovale. In addition, we did not include some variables in the analysis, such as echocardiographic examination data, due to a high level of missing data in the database. Finally, due to the limitations of the variables in the database, some variables of interest to us were not included in the analysis, such as the Glenn and Fontan surgeries, and the history of drug, tobacco and alcohol use of the parents of the children.
Conclusions
We have developed a nomogram model based on Cox regression, which has incorporated six predictive factors, including lymphocyte percentage, magnesium ion, neutrophil percentage, pO2, PTT, and VSD repair. The model has favorable performance and can assist in clinical decision-making by providing clinicians with effective predictive information.
Acknowledgments
None.
Footnote
Reporting Checklist: The authors have completed the TRIPOD reporting checklist. Available at https://tp.amegroups.com/article/view/10.21037/tp-2024-506/rc
Peer Review File: Available at https://tp.amegroups.com/article/view/10.21037/tp-2024-506/prf
Funding: None.
Conflicts of Interest: All authors have completed the ICMJE uniform disclosure form (available at https://tp.amegroups.com/article/view/10.21037/tp-2024-506/coif). The authors have no conflicts of interest to declare.
Ethical Statement: The authors are accountable for all aspects of the work in ensuring that questions related to the accuracy or integrity of any part of the work are appropriately investigated and resolved. All analyses were based on previous published studies, and thus no ethical approval and patient consent are required. The study was conducted in accordance with the Declaration of Helsinki and its subsequent amendments.
Open Access Statement: This is an Open Access article distributed in accordance with the Creative Commons Attribution-NonCommercial-NoDerivs 4.0 International License (CC BY-NC-ND 4.0), which permits the non-commercial replication and distribution of the article with the strict proviso that no changes or edits are made and the original work is properly cited (including links to both the formal publication through the relevant DOI and the license). See: https://creativecommons.org/licenses/by-nc-nd/4.0/.
References
- Tsao CW, Aday AW, Almarzooq ZI, et al. Heart Disease and Stroke Statistics-2022 Update: A Report From the American Heart Association. Circulation 2022;145:e153-639. Erratum in: Circulation 2022;146:e141. [Crossref] [PubMed]
- Lynn MM, Salemi JL, Kostelyna SP, et al. Lesion-Specific Congenital Heart Disease Mortality Trends in Children: 1999 to 2017. Pediatrics 2022;150:e2022056294. [Crossref] [PubMed]
- Ison HE, Griffin EL, Parrott A, et al. Genetic counseling for congenital heart disease - Practice resource of the National Society of Genetic Counselors. J Genet Couns 2022;31:9-33. [Crossref] [PubMed]
- Pierpont ME, Brueckner M, Chung WK, et al. Genetic Basis for Congenital Heart Disease: Revisited: A Scientific Statement From the American Heart Association. Circulation 2018;138:e653-711. [Crossref] [PubMed]
- Cerrone M, Remme CA, Tadros R, et al. Beyond the One Gene-One Disease Paradigm: Complex Genetics and Pleiotropy in Inheritable Cardiac Disorders. Circulation 2019;140:595-610. [Crossref] [PubMed]
- Dempsey EM, El-Khuffash AF. Objective cardiovascular assessment in the neonatal intensive care unit. Arch Dis Child Fetal Neonatal Ed 2018;103:F72-7. [Crossref] [PubMed]
- Wang X, Lu J, Song Z, et al. From past to future: Bibliometric analysis of global research productivity on nomogram (2000-2021). Front Public Health 2022;10:997713. [Crossref] [PubMed]
- Reyna MA, Sadr N, Alday EAP, Gu A, Shah AJ, Robichaux C, et al., editors. Will two do? Varying dimensions in electrocardiography: the PhysioNet/Computing in Cardiology Challenge 2021. 2021 Computing in Cardiology (CinC); 2021: IEEE.
- Li H, Zeng X, Yu G. Paediatric Intensive Care database (version 1.1.0). (2020). PhysioNet. doi:
10.13026/32x9-wv38 .10.13026/32x9-wv38 - Hardee I, Wright L, McCracken C, et al. Maternal and Neonatal Outcomes of Pregnancies in Women With Congenital Heart Disease: A Meta-Analysis. J Am Heart Assoc 2021;10:e017834. [Crossref] [PubMed]
- Neunert CE, Arnold DM, Grace RF, et al. The 2022 review of the 2019 American Society of Hematology guidelines on immune thrombocytopenia. Blood Adv 2024;8:3578-82. [Crossref] [PubMed]
- Taksande A, Jameel PZ. Critical Congenital Heart Disease in Neonates: A Review Article. Curr Pediatr Rev 2021;17:120-6. [Crossref] [PubMed]
- Temel HH, Kumbasar U, Büber E, et al. Comparison of antioxidant reserve capacity of children with acyanotic & cyanotic congenital heart disease. Indian J Med Res 2020;152:626-32. [Crossref] [PubMed]
- Doll CF, Pereira NJ, Hashimi MS, et al. Gestational intermittent hyperoxia rescues murine genetic congenital heart disease in part. Sci Rep 2021;11:6608. [Crossref] [PubMed]
- Chang CC, Lin YC, Chen TC, et al. High-Flow Nasal Cannula Therapy in Children With Acute Respiratory Distress With Hypoxia in A Pediatric Intensive Care UnitA Single Center Experience. Front Pediatr 2021;9:664180. [Crossref] [PubMed]
- Berman W Jr, Wood SC, Yabek SM, et al. Systemic oxygen transport in patients with congenital heart disease. Circulation 1987;75:360-8. [Crossref] [PubMed]
- Taka H, Douguchi T, Miyamoto A, et al. Oxygen Delivery During Cardiopulmonary Bypass in Pediatric Patients With Congenital Heart Disease: Association With Postoperative Acute Kidney Injury. J Cardiothorac Vasc Anesth 2025;39:702-10. [Crossref] [PubMed]
- Tangvoraphonkchai K, Davenport A. Magnesium and Cardiovascular Disease. Adv Chronic Kidney Dis 2018;25:251-60. [Crossref] [PubMed]
- Khalil AAMA, Ali ASA, Soliman AMRA, et al. Amiodarone and Magnesium Sulphate in Arrhythmia Prophylaxis in Pediatric Congenital Heart Diseases Patients Undergoing Cardiopulmonary Bypass. The Egyptian Journal of Hospital Medicine 2021;83:882-8. [Crossref]
- He D, Aggarwal N, Zurakowski D, et al. Lower risk of postoperative arrhythmias in congenital heart surgery following intraoperative administration of magnesium. J Thorac Cardiovasc Surg 2018;156:763-770.e1. [Crossref] [PubMed]
- Adcock DM, Moore GW, Montalvão SL, et al. Activated Partial Thromboplastin Time and Prothrombin Time Mixing Studies: Current State of the Art. Semin Thromb Hemost 2023;49:571-9. [Crossref] [PubMed]
- Zabala LM, Guzzetta NA. Cyanotic congenital heart disease (CCHD): focus on hypoxemia, secondary erythrocytosis, and coagulation alterations. Paediatr Anaesth 2015;25:981-9. [Crossref] [PubMed]
- Balachandran VP, Gonen M, Smith JJ, et al. Nomograms in oncology: more than meets the eye. Lancet Oncol 2015;16:e173-80. [Crossref] [PubMed]
- Guidotti R, Monreale A, Ruggieri S, et al. A survey of methods for explaining black box models. ACM computing surveys (CSUR) 2018;51:1-42. [Crossref]