Evaluation of radiomics as an assistant tool for radiologists in the diagnosis of necrotizing enterocolitis
Highlight box
Key findings
• Radiomics can be used as a tool to assist radiologists in the diagnosis of necrotizing enterocolitis (NEC).
What is known and what is new?
• NEC is a serious neonatal gastrointestinal disorder and early diagnosis is important.
• This study confirmed that radiomics can reduce missed necrotizing enterocolitis diagnoses.
What is the implication, and what should change now?
• Radiomics can be used as an assistant tool to improve the sensitivity of NEC diagnosis and help physicians take timely interventions.
Introduction
Necrotizing enterocolitis (NEC) is a serious gastrointestinal emergency that primarily affects premature infants, and it is one of the foremost causes of neonatal mortality. Despite advances in perinatal care and neonatal management, preterm infants are still at risk of NEC due to their underdeveloped intestinal function, making them more susceptible to gut microbiota infections and intestinal wall damage (1). Once NEC develops, it can progress rapidly, posing a significant risk to the newborn’s life and potentially resulting in long-lasting complications such as short bowel syndrome, intestinal narrowing, and neurological developmental abnormalities (2). Therefore, timely diagnosis of NEC can help physicians to make appropriate measures to reduce the risk of serious complications, decrease mortality, and improve the effectiveness of NEC treatment (3).
Imaging examination plays an important role in the diagnosis of NEC and in the follow-up of disease changes. In recent years, many studies have shown that abdominal ultrasound has a high positive rate in the early diagnosis of NEC (4,5), can dynamically monitor and evaluate the condition of children, and has high clinical value (6). Nonetheless, abdominal ultrasound is not widely used in the clinical diagnosis and monitoring of NEC, and abdominal radiograph is still the imaging method of choice for evaluating children with suspected NEC. Doctors rely on clinical manifestations and radiograph examinations to diagnose and stage NEC (2). However, the early clinical symptoms and imaging features of NEC are often nonspecific and can be easily mistaken for other gastrointestinal diseases. Such misidentification can lead to delays in treatment, potentially leading to severe health repercussions for the patient. To address this issue, researchers are exploring biomarkers as a tool for predicting NEC (7). Radiomics is a promising tool for improving the diagnosis, treatment, and management of diseases (8). By analyzing the radiomics features of imaging data, including complex patterns that are difficult for the human eye to recognize or quantify, it can discover new disease characteristics and identify biomarkers (8,9). Through the analysis of radiomics features, there is an opportunity to discover new diagnostic and prognostic markers for NEC, which can then be used to develop targeted therapies that selectively inhibit these pathways or processes, potentially leading to more effective treatments with fewer side effects. This allows doctors to intervene early, providing more precise treatment and better health management for affected children, reducing the occurrence of severe complications associated with NEC.
Currently, there are some radiomics studies based on NEC radiographs. Some studies (10,11) have shown that extracting radiomics features from radiographic images of children with NEC and constructing a radiomics model helped physicians to predict whether a patient with NEC needed surgical treatment. However, their radiomics study did not take portal venous gas on radiographs into account when considering the region of interest (ROI), an imaging sign that is important in the actual radiologist’s work (12). Portal venous gas is a highly significant imaging marker in NEC, occurring in 10–30% of cases. It is regarded as an extension of intramural gas in NEC and serves as a specific indicator of panintestinal necrosis. It demonstrates high specificity but low sensitivity for diagnosing NEC stage ≥II (13). In addition to this, their research primarily assessed the model’s sensitivity and specificity, often seeking direct comparison with radiologists’ performance. However, it suffered from a lack of external validation and did not assess the value of radiomics as assist tool when integrated with radiologists for diagnostic purposes.
Herein, our research employed three datasets (training set, test set and temporal validation set) to develop and validate a radiomics model. In the temporal validation set, we conducted a parallel test using radiologists’ diagnoses and radiomics diagnoses to evaluate the assistant diagnostic role of the radiomics model. We present this article in accordance with the TRIPOD reporting checklist (available at https://tp.amegroups.com/article/view/10.21037/tp-2024-496/rc).
Methods
The workflow chart in this study is shown in Figure 1. The steps included model design, statistics generation.
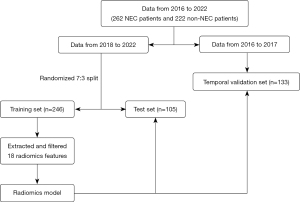
Data source
Our study was a retrospective analysis approved by the institutional ethics committee of the Children’s Hospital of Fudan University (No. 2023-196). The study was conducted in accordance with the Declaration of Helsinki and its subsequent amendments. Informed consent was obtained from all the patients’ legal guardians, and the data were anonymized to ensure confidentiality. We collected abdominal radiographs of NEC patients at the time they were diagnosed in accordance with the modified Bell staging system (14) and included them in the NEC group. We reviewed the electronic medical records and abdominal radiograph requests of hospitalized NEC patients, and selected the abdominal radiograph at the time clinicians suspected NEC-related symptoms. Meanwhile, we included in the non-NEC group patients within six months of age who were hospitalized during the same period, had not been diagnosed with NEC, and did not suffer from other intestinal diseases. Finally, we collected 484 images (Table 1) from NEC group (n=262) and non-NEC group (n=222), who underwent abdominal radiography examinations from 2016–2022. The data from 2018-2022 were divided into a training set (n=246) and a test set (n=105) in a ratio of 7:3, with the data from 2016–2017 serving as a temporal validation set (n=133). In order to understand the diagnostic situation of NEC by radiologists, we analyzed the radiological reports of 86 NEC patients in the temporal validation set to see if the conclusions indicated NEC.
Table 1
Characteristic | NEC group vs. Non-NEC group | Datasets | ||||||
---|---|---|---|---|---|---|---|---|
NEC group (n=262) |
Non-NEC group (n=222) |
P value | Training set (n=246) |
Test set (n=105) |
Temporal validation set (n=133) | P value | ||
Age* (days) | 15 [10–29] | 9.5 [2–22] | <0.001 | 13 [5–25] | 13 [6–25] | 15 [8.5–27] | 0.37 | |
Sex, male | 158 (60.3) | 123 (55.4) | 0.28 | 142 (57.7) | 61 (58.1) | 78 (58.6) | 0.99 | |
Gestational age (days) | 234 [218–254.8] | 251.5 [228–269.3] | <0.001 | 243 [225–268] | 242 [224–264] | 233 [210–257.3] | 0.01 | |
Birth weight (g) | 2,000 [1,546.3–2,597.5] |
2,597.5 [1,763.8–3,223.8] |
<0.001 | 2,232.5 [1,732.5–3,060] |
2,200 [1,697.5–3,082.5] |
1,935 [1,450–2,596.3] |
0.002 | |
Apgar score | 7 [1–9] | 9 [9–10] | <0.001 | 9 [6–9] | 8 [3–9] | 8 [1.25–9] | 0.10 | |
Modified Bell staging | ||||||||
IIA | 128 (48.9) | |||||||
IIB | 57 (21.8) | |||||||
IIIA | 59 (22.5) | |||||||
IIIB | 18 (6.9) |
Data are presented as n (%) or median [IQR]. *, age refers to the age at the time patients underwent abdominal radiography. IQR, interquartile range; NEC, necrotizing enterocolitis.
Imaging segmentation
All patients had undergone abdominal imaging on CR or DR. We acquired images from the picture archiving and communication system (PACS). We performed segmentation of radiograph image ROI using 3D Slicer software (version 5.2.2, www.slicer.org) (15). Due to the complexity of NEC lesions on radiograph images and the need for meticulous image segmentation by experienced diagnostic physicians (11), which is time-consuming, we opted for a simple rectangular segmentation approach for the abdominal area (Figure 2). The upper boundary of the rectangle is aligned with the horizontal level of the right diaphragm, and the lower boundary is aligned with the upper edge of the hip joint, encompassing the entire abdomen while making an effort to exclude the femoral heads. The work of image segmentation task was performed by a physician with 1 year of working experience in radiology supervised by radiologists with 18 and 28 years of working experience.
Feature extraction
We utilized the open-source software FeAture Explorer (FAE) V.0.5.5 (16) to extract features from ROI. This software employs Pyradiomics as the backend for feature extraction. Before extracting radiomics features, we normalized all images by scaling their intensities to a range of 0 to 1,000 and resampling them to the same resolution (1 mm × 1 mm × 1 mm) to mitigate heterogeneity biases among the data. Subsequently, we performed radiomics feature extraction on the FAE platform. These images encompassed 9 types, namely “Original”, “Wavelet Transform”, “Laplacian of Gaussian”, “Square”, “Square Root”, “Logarithm”, “Exponential”, “Gradient”, and “LBP2D”. From these 9 types of images, we extracted 7 classes of features, including first order, shape-based, gray level co-occurrence matrix (GLCM), gray level run length matrix (GLRLM), gray level size zone matrix (GLSZM), gray level dependence matrix (GLDM), neighbourhood gray tone difference matrix (NGTDM). The definitions and formulas of the extracted radiomic features were obtained from the official pyradiomics documentation (https://pyradiomics.readthedocs.io/).
Features screening and model development
We standardized radiomics features using the Z-score method. In the training set, we initially applied the minimum redundancy maximum relevance algorithm (mRMR) to select the top 20 features (17). Subsequently, we employed ten-fold cross-validation for the least absolute shrinkage and selection operator regression (LASSO). The mRMR algorithm is a feature selection method designed to identify the most representative subset of features from high-dimensional data. Its core principle is to select features that are highly correlated with the target variable while maintaining low redundancy among themselves. This approach ensures that the selected features are both informative and non-redundant, improving model performance and interpretability. The fundamental principle of the LASSO algorithm involves introducing a penalty term based on the L1 norm, which is the sum of the absolute values of all regression coefficients, to shrink the regression coefficients of all variables. As the penalty parameter (λ) increases, more coefficients are shrunk to zero, while variables not shrunk to zero are retained for model training (18). Finally, we used the selected features to construct the diagnostic model. We calculated weights for these features and their regression coefficients to obtain the predicted probability and evaluated the model on different datasets.
Statistical analysis
We employed Chi-square tests or Fisher’s exact tests to compare categorical variables. For the comparison of quantitative variables, t-tests or Mann-Whitney U tests were utilized. Logistic regression models were applied for analysis, and we calculated 95% confidence interval (CI). Receiver operating characteristic (ROC) curve analysis and the calculation of the area under the curve (AUC) were performed to assess the predictive performance of image features. The cutoff value was determined by Youden index, and accuracy, sensitivity, and specificity were calculated accordingly. All tests were two-tailed, and P<0.05 was considered statistically significant. Statistical analyses were conducted using R software (version 4.2.2, www.rproject.org) and SPSS 20. P values less than 0.05 were deemed to indicate statistically significant differences.
Results
Demographic information analysis
The final dataset included 484 patients. The clinical characteristics of these patients are summarized in Table 1. Among the 262 NEC patients, the distribution across stages was as follows: 128 cases at Stage IIA, 57 cases at Stage IIB, 59 cases at Stage IIIA, and 18 cases at Stage IIIB. Significant differences were observed in age, gestational age, birth weight and Apgar score between the NEC group and the non-NEC group. Additionally, gestational age and birth weight showed significant differences across all three datasets. Across the three datasets, there were no significant differences in patient sex (P=0.99) or age (P=0.37). There were more boys than girls in all three datasets.
Radiomics model performance
In this study, a total of 1,037 features were initially extracted, and after eliminating irrelevant features, 983 features remained. Through mRMR and LASSO feature dimensionality reduction (Figure 3), 18 features were selected for constructing the radiomics model, with the corresponding LASSO coefficients for radiomics model presented in Table 2. Among the 18 radiomic features selected in abdominal radiographs of NEC, the distribution can be categorized into four main types. Texture features represent the largest proportion with 10 features (55.6%), including GLCM-based (4 features), GLRLM-based (2 features), and GLSZM-based metrics (4 features). Wavelet features constitute the second-largest group with 5 features (27.8%). First-order features account for 2 features (11.1%), while shape features represent the smallest category with only 1 feature (5.6%). Figure 4 illustrates the heatmap of feature correlations in the training set, ranging from −0.44 to 0.52. The AUCs of the radiomics model (Table 3) on the training set, test set, and temporal validation set were 0.82, 0.74, and 0.71, respectively; the sensitivities were 0.75, 0.57, and 0.69, respectively. Figure 5 shows the ROC curve and calibration curve of different datasets.
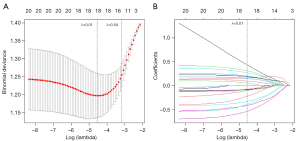
Table 2
Feature name | Coefficient | Description |
---|---|---|
(Intercept) | 0.034 | |
exponential_glcm_ClusterShade | −0.402 | The asymmetry of the mean value |
exponential_glszm_LargeAreaLowGrayLevelEmphasis | 0.432 | The proportion of lower gray-level values |
exponential_glszm_ZoneVariance | −0.105 | The variance in zone size volumes for the zones |
gradient_firstorder_Minimum | −0.080 | The minimum gray level intensity within the ROI |
gradient_glcm_MCC | 0.263 | The complexity of the texture |
lbp.2D_firstorder_90Percentile | −0.103 | The 90th percentile gray level intensity within the ROI |
lbp.2D_glrlm_RunLengthNonUniformityNormalized | 0.195 | The similarity of run lengths throughout the image |
logarithm_glcm_Correlation | 0.145 | The linear dependency of gray level values to their respective voxels |
logarithm_glrlm_ShortRunLowGrayLevelEmphasis | 0.091 | The joint distribution of shorter run lengths with lower gray-level values |
original_shape_Elongation | −0.334 | The relationship between the two largest principal components in the ROI shape |
square_glcm_ClusterShade | −0.085 | The skewness and uniformity of the GLCM |
squareroot_glszm_LowGrayLevelZoneEmphasis | 0.159 | The distribution of lower gray-level size zones |
squareroot_glszm_ZoneEntropy | 0.183 | The uncertainty/randomness in the distribution of zone sizes and gray levels |
wavelet.HH_firstorder_Mean | 0.049 | The average gray level intensity within the ROI |
wavelet.HH_glcm_Imc1 | −0.418 | The correlation between the probability distributions of and (quantifying the complexity of the texture) |
wavelet.LH_firstorder_Skewness | −0.541 | The asymmetry of the distribution of values about the Mean value |
wavelet.LL_glszm_LargeAreaLowGrayLevelEmphasis | −0.227 | The proportion in the image of the joint distribution of larger size zones with lower gray-level values |
wavelet.LL_glszm_SmallAreaLowGrayLevelEmphasis | 0.305 | The proportion in the image of the joint distribution of smaller size zones with lower gray-level values |
GLCM, gray level co-occurrence matrix; HH, high-high; GLSZM, gray level size zone matrix; GLRLM, gray level run length matrix; IMC, information measure of correlation; LASSO, the least absolute shrinkage and selection operator; LH, low-high; LL, low-low; LBP, local binary pattern; MCC, maximal correlation coefficient; ROI, region of interest.
Table 3
Dataset | Training set (n=246) | Test set (n=103) | Temporal validation set (n=133) |
---|---|---|---|
AUC (95% CI) | 0.82 (0.77–0.87) | 0.74 (0.64–0.83) | 0.71 (0.64–0.79) |
Accuracy (%) | 75 | 64 | 66 |
Sensitivity (%) | 75 | 57 | 69 |
Specificity (%) | 76 | 71 | 63 |
PPV (%) | 75 | 67 | 64 |
NPV (%) | 75 | 62 | 67 |
AUC, area under the curve; CI, confidence Interval; NPV, negative predictive value; PPV, positive predictive value.
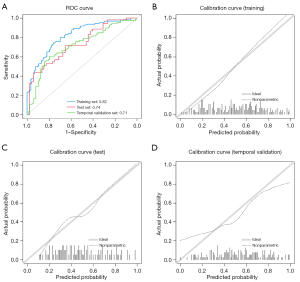
Validation in temporal validation set
In the temporal validation set of 86 NEC patients, radiologists missed five cases due to the absence of typical features such as pneumatosis intestinalis or portal venous gas. However, the radiomics model successfully identified three of these five missed cases, demonstrating its potential to detect subtle imaging patterns that may be overlooked in conventional radiological assessments.
The Chi-square test showed that the combined approach (parallel testing) of radiologists and the radiomics model was better than the diagnosis made by the radiologist alone (P=0.003). Table 4 shows the results of the chi-square test of this comparison. The combined diagnostic approach of radiologists and radiomics outperformed the radiologist-only assessment, showing improved diagnostic accuracy and fewer missed diagnoses. This result highlights the value of integrating radiomics with radiologist interpretation, potentially enhancing the detection rate and reducing the likelihood of missed cases compared to radiologist assessments alone.
Table 4
Methods | Radiologist | P value | |
---|---|---|---|
Positive | Negative | ||
Parallel testing | 0.003 | ||
Positive | 81 | 3 | |
Negative | 0 | 2 |
Discussion
In this study, we employed a rectangular ROI segmentation to extract radiomics features, resulting in a conventional radiomics model with certain predictive performance. This model not only exhibited certain performance on both the training and test sets, but also showed consistent stability when applied to the temporal validation set, with AUC values of 0.82, 0.74, and 0.71, respectively. The combination of radiomics models and radiologists in parallel diagnosis has increased the sensitivity for diagnosing NEC and reduced the rate of missed diagnoses, which illustrates the value of radiomics models as an assist tool.
Compared to the Duke score (12), our use of rectangular segmentation and radiomics quantified image information, reducing reliance on physician subjectivity. In contrast to previous radiomics studies (10,11), our study included the liver in the ROI, because radiologists should take into account the possibility of dendritic portal venous gas in the liver area in real-world situations when diagnosing NEC. From this perspective, our approach may have more meaningful implications for translational application in medical practice.
Previous studies have shown a 90% agreement in the value of plain abdominal radiographs for confirming the diagnosis of NEC, providing guidance for decision-making regarding surgery and abdominal radiography as the preferred treatment modality and the most important radiographic sign, respectively (6). However, relatively few studies have applied machine learning (ML) and artificial intelligence (AI) to NEC. There is a study that use radiological features to predict NEC surgery (10), constructing a joint model based on four radiomics features and clinical signs when abdominal radiographs do not suggest an absolute surgical indication for free pneumoperitoneum. Additionally, there is a study that use a multimodal AI system built with feature engineering, ML, and deep learning (DL) for NEC diagnosis and surgery prediction using plain abdominal radiographs, and find that NEC diagnostic efficacy reaches 0.93, and then this efficacy is a combination of 40 abdominal imaging features and 18 clinical parameters (11). This study, on the other hand, used only abdominal radiomics features and also achieved a stable performance in the test set and temporal validation set.
The distribution of radiomic features in our study provides insights into the characterization of NEC radiographic patterns. Firstly, the predominance of texture features (55.6%) highlights the importance of spatial relationships and gray-level patterns in identifying NEC-related changes (8). Particularly, the balanced distribution among GLCM (4 features), GLRLM (2 features), and GLSZM (4 features) captures various aspects of image heterogeneity, from local pixel relationships to regional intensity patterns (19,20). Secondly, the substantial proportion of wavelet features (27.8%) suggests the significance of multi-scale analysis in detecting subtle radiographic changes at different resolutions. Finally, the smaller proportions of first-order (11.1%) and shape features (5.6%) indicate that while basic intensity statistics and morphological characteristics contribute to the analysis, they play a supplementary role compared to the more sophisticated texture and wavelet-based features in NEC assessment (8,21). In summary, texture and wavelet features comprise 83.4% of all features, indicating that the complex patterns and multi-scale characteristics of radiographs in NEC can be captured by these advanced image analysis methods (22). Notably, the wavelet.LH_firstorder_Skewness feature has the largest coefficient (−0.541) in the model, indicating its significant role in the model’s performance as the most important and specific sign. Skewness quantifies the asymmetry of voxel intensity distributions within medical images. The wavelet.LH_firstorder_Skewness feature may be sensitive to these changes and can help capture the complex patterns and multi-scale characteristics of radiographic images in NEC. In the context of radiomics, it can reflect the asymmetry of the gray level distribution in the image. In the radiological manifestations of NEC, the presence of pneumoperitoneum, pneumatosis intestinalis, and portal venous gas, which appear as low-density areas, mixed with the soft tissue density from thickened bowel loops, results in heterogeneous imaging findings. We hypothesized that these radiological features may be captured by these radiomic features. Previous researchers have hypothesized that the transform-filtered statistics may have the potential to suppress noise and highlight details in the original image, so that they can extract regions featuring progressively coarser texture patterns in a more adaptable way (10). However, current studies do not well integrate these physiology and pathology of the disease with radiomic features.
Our study demonstrated that parallel diagnosis combining radiologists and radiomics models improved the sensitivity of NEC diagnosis. For severe neonatal conditions like NEC that progress rapidly and have high mortality rates, timely detection and early intervention are crucial. Studies have shown that delayed diagnosis can lead to expanded intestinal necrosis, increasing surgical risks and mortality rates (23). Therefore, improving sensitivity in NEC diagnosis has special clinical significance, and the trade-off with slightly reduced specificity may be worthwhile. The advantages of this parallel diagnostic approach have been validated in multiple studies. A systematic review found that combining AI with clinicians significantly improved disease detection rates while maintaining high specificity (24). This advantage stems from the complementarity between AI systems and clinicians: radiomics models can capture subtle features difficult for human eyes to identify, while clinicians possess rich experience and comprehensive clinical thinking. One research confirmed that the combination of AI and radiologists could reduce missed diagnoses in breast cancer screening, effectively creating a double-check mechanism (25). Additionally, other research reported that AI-assisted diagnosis could improve radiologists’ work efficiency by helping them focus on suspicious cases (26). Our study also validates this point, as the parallel diagnostic strategy helps overcome potential subjective judgment differences and fatigue-related errors that may occur when relying solely on radiologists. AI should not be viewed as a tool to replace doctors but as a powerful aid to clinical decision-making (27). This aligns with our research philosophy of pursuing human-machine collaboration and complementary advantages to jointly improve diagnostic accuracy. Future research could further explore how to optimize the parallel diagnostic process and better integrate AI into clinical practice.
Nonetheless, there are certain limitations to this study. First, this is a single-center study, and although a temporal validation set was used to simulate the real situation, data from external centers are still needed for further validation. Second, our research primarily depends on single-source medical imaging data. However, clinical diagnosis of NEC often involves a combination of clinical symptoms, such as vomiting, abdominal distension, and bloody stools, along with radiological signs like pneumoperitoneum, pneumatosis intestinalis, and portal venous gas (3,14), and other studies have included clinical features, genetic markers, metabolomics, and fecal microbiota (11,28,29), therefore multimodal data such as ultrasound, and electronic medical record data can be combined with radiomics in the future. Third, while our results from the temporal validation set show no signs of overfitting, the number of features in the model increases concerns about overly optimistic results when the number of features in the model is high relative to the number of cases in a category. Augmenting the data may help in the training of the model. Fourth, this study utilized only the images associated with the initial diagnosis or suspected diagnosis of NEC from the radiological diagnostic reports. Further analysis of follow-up imaging from the case group may contribute to enhancing the effectiveness of radiomics in NEC diagnosis.
Conclusions
Radiomics shows promise as a valuable tool to complement traditional NEC diagnostics. By providing quantitative, reproducible data, it reduces subjectivity and enhances diagnostic precision, particularly by improving sensitivity and reducing missed diagnoses in equivocal cases. Future multi-center studies should validate these findings and explore combining radiomics with other diagnostic modalities.
Acknowledgments
None.
Footnote
Reporting Checklist: The authors have completed the TRIPOD reporting checklist. Available at https://tp.amegroups.com/article/view/10.21037/tp-2024-496/rc
Data Sharing Statement: Available at https://tp.amegroups.com/article/view/10.21037/tp-2024-496/dss
Peer Review File: Available at https://tp.amegroups.com/article/view/10.21037/tp-2024-496/prf
Funding: None.
Conflicts of Interest: All authors have completed the ICMJE uniform disclosure form (available at https://tp.amegroups.com/article/view/10.21037/tp-2024-496/coif). The authors have no conflicts of interest to declare.
Ethical Statement: The authors are accountable for all aspects of the work in ensuring that questions related to the accuracy or integrity of any part of the work are appropriately investigated and resolved. The study was conducted in accordance with the Declaration of Helsinki and its subsequent amendments. The study was approved by the institutional ethics committee of the Children’s Hospital of Fudan University (No. 2023-196). Informed consent was obtained from all the patients’ legal guardians, and the data were anonymized to ensure confidentiality.
Open Access Statement: This is an Open Access article distributed in accordance with the Creative Commons Attribution-NonCommercial-NoDerivs 4.0 International License (CC BY-NC-ND 4.0), which permits the non-commercial replication and distribution of the article with the strict proviso that no changes or edits are made and the original work is properly cited (including links to both the formal publication through the relevant DOI and the license). See: https://creativecommons.org/licenses/by-nc-nd/4.0/.
References
- Bell EF, Hintz SR, Hansen NI, et al. Mortality, In-Hospital Morbidity, Care Practices, and 2-Year Outcomes for Extremely Preterm Infants in the US, 2013-2018. JAMA 2022;327:248-63. [Crossref] [PubMed]
- Lueschow SR, Boly TJ, Jasper E, et al. A critical evaluation of current definitions of necrotizing enterocolitis. Pediatr Res 2022;91:590-7. Erratum in: Pediatr Res 2022;91:711. [Crossref] [PubMed]
- Hu X, Liang H, Li F, et al. Necrotizing enterocolitis: current understanding of the prevention and management. Pediatr Surg Int 2024;40:32. [Crossref] [PubMed]
- Hwang M, Tierradentro-García LO, Dennis RA, et al. The role of ultrasound in necrotizing enterocolitis. Pediatr Radiol 2022;52:702-15. [Crossref] [PubMed]
- Kim JH. Role of Abdominal US in Diagnosis of NEC. Clin Perinatol 2019;46:119-27. [Crossref] [PubMed]
- Ahle M, Ringertz HG, Rubesova E. The role of imaging in the management of necrotising enterocolitis: a multispecialist survey and a review of the literature. Eur Radiol 2018;28:3621-31. [Crossref] [PubMed]
- Gephart SM, Gordon PV, Penn AH, et al. Changing the paradigm of defining, detecting, and diagnosing NEC: Perspectives on Bell’s stages and biomarkers for NEC. Semin Pediatr Surg 2018;27:3-10. [Crossref] [PubMed]
- Gillies RJ, Kinahan PE, Hricak H. Radiomics: Images Are More than Pictures, They Are Data. Radiology 2016;278:563-77. [Crossref] [PubMed]
- Tomaszewski MR, Gillies RJ. The Biological Meaning of Radiomic Features. Radiology 2021;298:505-16. [Crossref] [PubMed]
- Li Y, Wu K, Yang H, et al. Surgical prediction of neonatal necrotizing enterocolitis based on radiomics and clinical information. Abdom Radiol (NY) 2024;49:1020-30. [Crossref] [PubMed]
- Gao W, Pei Y, Liang H, et al. Multimodal AI System for the Rapid Diagnosis and Surgical Prediction of Necrotizing Enterocolitis. IEEE Access 2021;9:51050-64.
- Coursey CA, Hollingsworth CL, Gaca AM, et al. Radiologists’ agreement when using a 10-point scale to report abdominal radiographic findings of necrotizing enterocolitis in neonates and infants. AJR Am J Roentgenol 2008;191:190-7. Erratum in: AJR Am J Roentgenol 2008;191:931. [Crossref] [PubMed]
- Chen Y, Duan Y, Wei B, et al. Value of portal venous gas and a nomogram for predicting severe neonatal necrotizing enterocolitis. Pediatr Res 2024; Epub ahead of print. [Crossref] [PubMed]
- Patel RM, Ferguson J, McElroy SJ, et al. Defining necrotizing enterocolitis: current difficulties and future opportunities. Pediatr Res 2020;88:10-5. [Crossref] [PubMed]
- Fedorov A, Beichel R, Kalpathy-Cramer J, et al. 3D Slicer as an image computing platform for the Quantitative Imaging Network. Magn Reson Imaging 2012;30:1323-41. [Crossref] [PubMed]
- Song Y, Zhang J, Zhang YD, et al. FeAture Explorer (FAE): A tool for developing and comparing radiomics models. PLoS One 2020;15:e0237587. [Crossref] [PubMed]
- Xia TY, Zhou ZH, Meng XP, et al. Predicting Microvascular Invasion in Hepatocellular Carcinoma Using CT-based Radiomics Model. Radiology 2023;307:e222729. [Crossref] [PubMed]
- Jia H, Li R, Liu Y, et al. Preoperative Prediction of Perineural Invasion and Prognosis in Gastric Cancer Based on Machine Learning through a Radiomics-Clinicopathological Nomogram. Cancers (Basel) 2024;16:614. [Crossref] [PubMed]
- Aerts HJ, Velazquez ER, Leijenaar RT, et al. Decoding tumour phenotype by noninvasive imaging using a quantitative radiomics approach. Nat Commun 2014;5:4006. [Crossref] [PubMed]
- Yip SS, Aerts HJ. Applications and limitations of radiomics. Phys Med Biol 2016;61:R150-66. [Crossref] [PubMed]
- Lambin P, Rios-Velazquez E, Leijenaar R, et al. Radiomics: extracting more information from medical images using advanced feature analysis. Eur J Cancer 2012;48:441-6. [Crossref] [PubMed]
- van Griethuysen JJM, Fedorov A, Parmar C, et al. Computational Radiomics System to Decode the Radiographic Phenotype. Cancer Res 2017;77:e104-7. [Crossref] [PubMed]
- Patel EU, Wilson DA, Brennan EA, et al. Earlier re-initiation of enteral feeding after necrotizing enterocolitis decreases recurrence or stricture: a systematic review and meta-analysis. J Perinatol 2020;40:1679-87. [Crossref] [PubMed]
- Liu X, Faes L, Kale AU, et al. A comparison of deep learning performance against health-care professionals in detecting diseases from medical imaging: a systematic review and meta-analysis. Lancet Digit Health 2019;1:e271-97. [Crossref] [PubMed]
- Rodriguez-Ruiz A, Lång K, Gubern-Merida A, et al. Stand-Alone Artificial Intelligence for Breast Cancer Detection in Mammography: Comparison With 101 Radiologists. J Natl Cancer Inst 2019;111:916-22. [Crossref] [PubMed]
- McKinney SM, Sieniek M, Godbole V, et al. International evaluation of an AI system for breast cancer screening. Nature 2020;577:89-94. Erratum in: Nature 2020;586:E19. [Crossref] [PubMed]
- Göndöcs D, Dörfler V. AI in medical diagnosis: AI prediction & human judgment. Artif Intell Med 2024;149:102769. [Crossref] [PubMed]
- Hooven TA, Lin AYC, Salleb-Aouissi A. Multiple Instance Learning for Predicting Necrotizing Enterocolitis in Premature Infants Using Microbiome Data. Proc ACM Conf Health Inference Learn (2020) 2020;2020:99-109.
- Lin YC, Salleb-Aouissi A, Hooven TA. Interpretable prediction of necrotizing enterocolitis from machine learning analysis of premature infant stool microbiota. BMC Bioinformatics 2022;23:104. [Crossref] [PubMed]