Derivation and validation of a prediction model for neonate unplanned rehospitalization in a tertiary center in China
Introduction
Recently, rehospitalization rates have been used as an indicator of the quality of care that patients received during hospital admission and after discharge (1-3). The definition of rehospitalization varies across studies. The most commonly used definition of rehospitalization is rehospitalization between 28 and 31 days after discharge for unplanned reasons (4-8). Rehospitalization rates for children aged 0–18 years range from 3.4–28.6% (5,9-12). There is a paucity of studies addressing neonate rehospitalizations. Gay et al. found that 11.4% of the rehospitalizations in the Monroe Carell Jr Children’s Hospital in Vanderbilt involved neonates, second to patients aged >10 years (13).
Unplanned rehospitalization within a short period of time is potentially preventable, and studies have indicated that the proportion of preventable rehospitalizations among all rehospitalizations ranges from 9% to 59% (5,9-12,14). Preventable rehospitalizations result in unnecessary hospitalization, an increased risk of nosocomial infections, additional costs, the waste of medical resources, increased stress in both neonates and mothers, delayed breast feeding, etc. (11,12,15-18). By analyzing possible factors affecting rehospitalization, additional support and medication could be given to neonates at a higher risk of rehospitalization, reducing the likelihood that those neonates would be rehospitalized.
Several studies have investigated the epidemiology of and risk factors for neonate rehospitalization, including sex, race, ethnicity, gestational age (GA), birth weight (BW), duration of index hospitalization, whether they are covered by Medicaid or private insurance, and maternal conditions (18-22). However, few researchers have studied the risk factors for neonate unplanned rehospitalization within a tertiary center, so these factors have not been included in clinical prediction models. To reduce the rehospitalization of neonates, we sought to build a neonate rehospitalization model. The findings will provide a basis for the assessment, development and improvement of neonatal clinical management, hopefully reducing the rate of neonate rehospitalization.
We present the following article in accordance with the TRIPOD reporting checklist (available at http://dx.doi.org/10.21037tp-20-184).
Methods
Study design, setting, and population
We developed a binominal regression model with unplanned rehospitalization as an outcome. Data were collected from the inpatient medical records from the Children’s Hospital of Fudan University (CHOF). Neonates who were admitted to the Department of Neonatology of CHOF between 11/1/2016 and 12/31/2018 were included. The dataset was divided into the model construction dataset and the validation dataset according to admission date. The subjects in the model construction dataset were neonates hospitalized from November 2016 to January 2018, while the validation dataset subjects were neonates hospitalized from February 2018 to December 2018. Both the model construction dataset and the validation dataset were divided into the rehospitalization group and the control group.
The inclusion criterion for the rehospitalization group was unplanned rehospitalization. Unplanned rehospitalization was defined as a second admission of a neonate to the Department of Neonatology of CHOF within 31 days after the discharge date of an index admission for unplanned reasons. The exclusion criteria included planned rehospitalization; hospitalization in a department other than the Department of Neonatology; transfer to a ward other than the Department of Neonatology; death; rehospitalization more than 31 days after the discharge date; and more than two hospitalizations.
Neonates who were admitted to the Department of Neonatology of CHOF between 11/1/2016 and 12/31/2018 were included as the control group, excluding neonates who were rehospitalized in either the neonatal inpatient ward or other pediatric inpatient wards within 31 days after discharge, neonates with more than one inpatient event, and those who died before discharge. Random coding was applied for the selection of the control group. The number of neonates chosen as the control group for the model construction dataset was 1.5 times the number of neonates with unplanned rehospitalizations, and 150 neonates were randomly selected for the validation dataset. A flow chart of the subjects is represented in Figure 1.
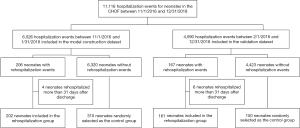
Main outcome measures
Unplanned rehospitalizations for any reason were measured because patients could be readmitted for related conditions even if the index admission and rehospitalization diagnoses were different.
Factors inclusion
The literature on neonatal risk factors associated with rehospitalization was examined. A total of 37 risk factors were identified. Based on their availability and clinical adaptability, 21 risk factors were selected for the initial stage of model development. The 21 risk factors were as follows: sex, GA, BW, blood culture positivity, the maximum total serum bilirubin (TSB) level during hospitalization, the procalcitonin (PCT) level, the use of antibiotics during hospitalization, antibiotic use duration, respiratory support duration, respiratory support during hospitalization, blood transfusion during hospitalization, invasive operation during hospitalization, neonatal intensive care unit (NICU) length of stay (LOS), nonmedical order discharge, hospital LOS, 5-minute Apgar score, maternal age, socioeconomic status and hospitalization fees. Risk factors with a more than 20% missing data were excluded. Data for included risk factors were collected from the inpatient medical records from the CHOF.
Sample size
The adequacy of the sample size (n) for the development of the predictive model was ensured by employing the required sample size equation (Equation 1). Overfitting was minimized with the combination of the predictor parameters (P), the coefficient of determination and the Van Houwelingen shrinkage factor (SVH). The coefficient of determination was set as 0.1, while the SVH was set as 0.9.
[1]
Statistical analyses
Continuous variables are expressed as the means (SDs) and were compared using an unpaired, 2-tailed t-test or the Mann-Whitney test. Categorical variables were compared using the χ2 test. P<0.05 was considered statistically significant for the analyses. The significance of each variable in the model construction dataset was assessed with a binominal regression model. Univariate regression analysis was used to evaluate the predictive accuracy of each variable in the prediction model.
A nomogram was formulated for use in clinical practice based on the results of binominal regression with R version 3.5.2. Each regression coefficient in binominal logistic regression was converted proportionally to a 0- to 100-point scale. The effect of the variable with the highest β coefficient (absolute value) is assigned 100 points. The points are added across independent variables to derive the total points, which are converted to the predicted probability. The predictive performance of the nomogram was measured by the concordance index (C-index) and calibration with bootstrap samples was performed to decrease the overfit bias. Receiver operating characteristic (ROC) curve analysis was used to calculate the optimal cutoff values that maximized the Youden index.
Validation
To validate the model derived from the model construction dataset, we applied the scoring method to the validation dataset (N=311). C-statistics were used for validation.
Ethical considerations
The study involving human participants were reviewed and approved by the Ethical Committee of Children’s Hospital, Fudan University [No. (2018)213]. Informed consents for participation were obtained from parents or legal guardians of the patients in this study. The study was conducted in accordance with the Declaration of Helsinki (as revised in 2013).
Results
Study population and sample
There were 11,116 hospitalization events for neonates in the CHOF between 11/1/2016 and 12/31/2018. The model construction dataset included 6,526 hospitalization events, with 206 rehospitalization events. The validation dataset included 4,590 hospitalization events, with 167 rehospitalization events. Four rehospitalization events from the model construction dataset and 6 rehospitalization events from the validation dataset were excluded according to the exclusion criteria. The unplanned rehospitalization rates in the model construction dataset and the validation dataset were 3.10% vs. 3.51%, respectively, and the overall unplanned rehospitalization rate was 3.27%.
In the model construction dataset, the rehospitalization group and the control group had a median GA of 35.8 vs. 37.49 weeks (P<0.001) and a median BW of 2,578.72 vs. 2,923.65 grams (P<0.001). The proportions of males in the rehospitalization group (n=202) and the control group (n=310) were 61.65% and 56.13% (P=0.271), respectively. The median LOS for the two groups was 8.5 days (P=0.558). The median NICU LOS for the rehospitalization group was 13 days (IQR: 4–44.75), while it was 6 days (IQR: 3–15.5) for the control group (P<0.001). The rehospitalization group and the control group median maternal ages of 29.59 and 29.83 years (P=0.349) and nonmedical discharge rates of 44% vs. 39% (P=0.018), respectively.
In contrast with the model construction dataset, the validation dataset had a median GA of 36 weeks (P=0.557) and a median BW of 2,780 grams (P=0.866). The median LOS was 7.5 days (IQR: 4.5–15) in the neonatal inpatient ward (P=0.082) and 9 days (IQR: 3–29) in the NICU (P=0.675). The median maternal age for the validation set was 29.87 years (P=0.483), with a nonmedical discharge rate of 66% (P=0.032) (Table 1).
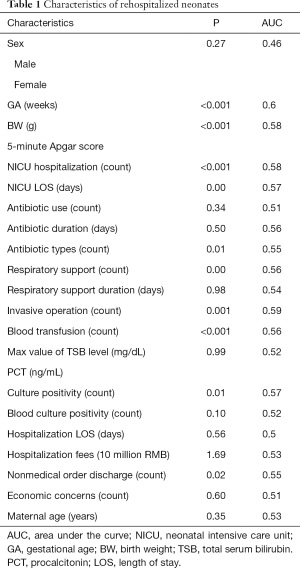
Full table
The nomogram and its predictive performance
Univariate logistic analysis of risk factors for infant rehospitalization identified 17 variables with AUCs >0.52 (Table 2). PCT and the 5-minute Apgar score were excluded due to missing data. Four risk factors were selected in the variable selection process, and risk factors associated with neonate unplanned rehospitalization according to the binominal regression model are shown in Table 3. Strong associations were observed for GA (OR: 0.99; 95% CI: 0.98–1.00), NICU LOS (OR: 1.02; 95% CI: 1.01–1.04), nonmedical order discharge (OR: 2.22; 95% CI: 1.34–3.68) and maternal age (OR: 0.96; 95% CI: 0.92–1.00). These strongly associated risk factors were used to generate the prediction model for neonate unplanned rehospitalization.
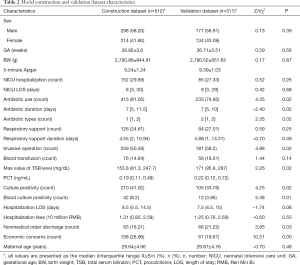
Full table
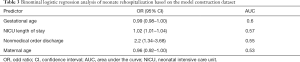
Full table
The regression coefficients (β) from the binominal logistic model were used to construct the prediction model for neonate unplanned rehospitalization. The scoring system was as follows: Log [P/1-P] = 3.7535- 0.0132* (GA) + 0.0225* (NICU LOS) + 0.7976* (nonmedical order discharge) - 0.0132* (maternal age). The performance of the nomogram was measured by ROC curve analysis, and the AUC was 0.68 in the model construction dataset. A nomogram was generated to summarize the results of the scoring system for clinical use (Figure 2).
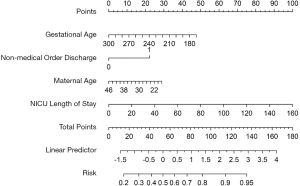
Validation
The model developed using the model construction dataset was tested for validity in the validation dataset. The predictive accuracy of the nomogram was measured by the bootstrap method. The C-index for the validation dataset was 0.65, and the risk estimation for the calibration curve was good (Figure 3).
The actual result and the result predicted by the neonate unplanned rehospitalization model were analyzed by the Youden index. The model had the best performance when the Youden index was maximized at 1.22. The cutoff score that maximized the Youden index was 0.55, with a sensitivity of 63% and a specificity of 59%. The probability of the cutoff point was 37%, corresponding to a total of 39 points on the nomogram.
Discussion
In this study, a prognostic model for neonate rehospitalization was created by combining neonatal clinical data and demographic data based on 6,526 hospital admission hospital events. External validation was performed based on 4,590 admission events. The overall unplanned rehospitalization rate for neonates was 3.27%. Analysis of 21 risk factors, including 15 clinical factors and 6 demographic factors, produced a simple model that included 4 risk factors. Based on the results, lower GA, a longer NICU LOS, nonmedical order discharge, and a lower maternal age were confirmed to be strong risk factors for neonate unplanned rehospitalizations. The nomogram derived from the prediction model can be used by clinicians on paper or as an online risk calculator.
Previous studies indicated that GA, BW, sex, race, LOS, diagnosis, Apgar score, maternal age, etc. were risk factors for neonate rehospitalization, but the studies were mostly based on maternity hospitals with neonates who were relatively healthy (4,23). Few studies have constructed prediction models based on neonates in the NICU (4,24). One study constructed a model for infants that incorporated 7 binary factors (Apgar score, BW, congenital abnormalities, maternal age, maternal smoking during pregnancy, maternal substance and alcohol use during pregnancy, and previous pregnancy) that predict infant mortality (25). However, risk factors for rehospitalization differ slightly from risk factors for mortality in neonates and there has been no research established a prediction model for neonate unplanned rehospitalization. Our present study focused on disease-based neonate rehospitalization in a specialized hospital and incorporated 4 comprehensive and easily available predischarge variables. The model performed well, with an AUC of 0.68 and a C-index value of 0.65 in the training and validation datasets.
This prognostic model has clinical relevance for neonatologists, enabling them to better identify neonates who are at high risk of rehospitalization. According to our neonate rehospitalization prediction model, 61.1% of the outcomes for discharged neonates could be predicted well (including both safe discharge and rehospitalization). The outcomes of 19.3% of the discharged neonates might be predicted incorrectly (predicted to have a safe discharge but eventually readmitted), putting the discharged infants at risk for health-related uncertainties. Therefore, follow-up for high-risk neonates could be achieved by short-term outpatient visits or maternal education with regard to adequate postnatal care and follow-up should be enhanced for neonates with total scores greater than 39 points.
Limitations
The study has several limitations. First, the datasets did not include information about rehospitalization in a different hospital nor the data from the hospital previously attended, which likely led to an underestimation of rehospitalization. Second, the patients were all consisted of the Han nationality and the data were collected from a single city, thus the application of the model in different races and regions should be assessed. Third, prospective studies are needed to further confirm the reliability of the nomogram.
Conclusions
By including 4 main risk factors for neonate rehospitalization, a nomogram for neonatal clinicians was generated. The model is a good predictor of neonate unplanned rehospitalization, and it performed well after validation. A total score that greater than 39 indicated the need for further follow-up.
Acknowledgments
We want to express out most sincere gratitude to all the infants who participated in our study as well as their parents and guardians for their commitment to the project.
Funding: None.
Footnote
Reporting Checklist: The authors have completed the TRIPOD reporting checklist. Available at http://dx.doi.org/10.21037/tp-20-184
Data Sharing Statement: Available at http://dx.doi.org/10.21037/tp-20-184
Conflicts of Interest: All authors have completed the ICMJE uniform disclosure form (available at http://dx.doi.org/10.21037/tp-20-184). The authors have no conflicts of interest to declare.
Ethical Statement: The authors are accountable for all aspects of the work in ensuring that questions related to the accuracy or integrity of any part of the work are appropriately investigated and resolved. The study was conducted in accordance with the Declaration of Helsinki (as revised in 2013). The study involving human participants were reviewed and approved by the Ethical Committee of Children’s Hospital, Fudan University board [No. (2018)213]. Informed consents for participation were obtained from parents or legal guardians of the patients in this study.
Open Access Statement: This is an Open Access article distributed in accordance with the Creative Commons Attribution-NonCommercial-NoDerivs 4.0 International License (CC BY-NC-ND 4.0), which permits the non-commercial replication and distribution of the article with the strict proviso that no changes or edits are made and the original work is properly cited (including links to both the formal publication through the relevant DOI and the license). See: https://creativecommons.org/licenses/by-nc-nd/4.0/.
References
- Berry JG, Hall DE, Kuo DZ, et al. Hospital utilization and characteristics of patients experiencing recurrent readmissions within children's hospitals. JAMA 2011;305:682-90. [Crossref] [PubMed]
- Kristensen SR, Bech M, Quentin W. A roadmap for comparing readmission policies with application to Denmark, England, Germany and the United States. Health Policy 2015;119:264-73. [Crossref] [PubMed]
- Nakamura MM, Toomey SL, Zaslavsky AM, et al. Measuring pediatric hospital readmission rates to drive quality improvement. Acad Pediatr 2014;14:S39-46. [Crossref] [PubMed]
- Zhou H, Della PR, Roberts P, et al. Utility of models to predict 28-day or 30-day unplanned hospital readmissions: an updated systematic review. BMJ Open 2016;6:e011060. [Crossref] [PubMed]
- Considine J, Berry D, Newnham E, et al. Factors associated with unplanned readmissions within 1 day of acute care discharge: a retrospective cohort study. BMC Health Serv Res 2018;18:713. [Crossref] [PubMed]
- Markham JL, Hall M, Gay JC, et al. Length of Stay and Cost of Pediatric Readmissions. Pediatrics 2018;141:e20172934. [Crossref] [PubMed]
- Chung HS, Hathaway DK, Lew DB. Risk factors associated with hospital readmission in pediatric asthma. J Pediatr Nurs 2015;30:364-84. [Crossref] [PubMed]
- Zhou H, Roberts PA, Dhaliwal SS, et al. Risk factors associated with paediatric unplanned hospital readmissions: a systematic review. BMJ Open 2019;9:e020554. [Crossref] [PubMed]
- Auger KA, Mueller EL, Weinberg SH, et al. A Validated Method for Identifying Unplanned Pediatric Readmission. J Pediatr 2016;170:105-12.e1-2.
- Berry JG, Toomey SL, Zaslavsky AM, et al. Pediatric readmission prevalence and variability across hospitals. JAMA 2013;309:372-80. [Crossref] [PubMed]
- Young PC, Korgenski K, Buchi KF. Early readmission of newborns in a large health care system. Pediatrics 2013;131:e1538-44. [Crossref] [PubMed]
- Metcalfe A, Mathai M, Liu S, et al. Proportion of neonatal readmission attributed to length of stay for childbirth: a population-based cohort study. BMJ Open 2016;6:e012007. [Crossref] [PubMed]
- Gay JC, Hain PD, Grantham JA, et al. Epidemiology of 15-Day Readmissions to a Children's Hospital. Pediatrics 2011;127:e1505-12. [Crossref] [PubMed]
- Yam CH, Wong EL, Chan FW, et al. Avoidable readmission in Hong Kong--system, clinician, patient or social factor? BMC Health Serv Res 2010;10:311. [Crossref] [PubMed]
- Bucholz EM, Gay JC, Hall M, et al. Timing and Causes of Common Pediatric Readmissions. J Pediatr 2018;200:240-8.e1. [Crossref] [PubMed]
- Seaton SE, Barker L, Jenkins D, et al. What factors predict length of stay in a neonatal unit: a systematic review. BMJ Open 2016;6:e010466. [Crossref] [PubMed]
- Burgos AE, Schmitt SK, Stevenson DK, et al. Readmission for neonatal jaundice in California, 1991-2000: trends and implications. Pediatrics 2008;121:e864-9. [Crossref] [PubMed]
- Paul DA, Agiro A, Hoffman M, et al. Hospital Admission and Emergency Department Utilization in an Infant Medicaid Population. Hosp Pediatr 2016;6:587-594. [Crossref] [PubMed]
- Underwood MA, Danielsen B, Gilbert WM. Cost, causes and rates of rehospitalization of preterm infants. J Perinatol 2007;27:614-9. [Crossref] [PubMed]
- Varvarigou A, Fouzas S, Skylogianni E, et al. Transcutaneous bilirubin nomogram for prediction of significant neonatal hyperbilirubinemia. Pediatrics 2009;124:1052-9. [Crossref] [PubMed]
- Lain SJ, Roberts CL, Bowen JR, et al. Early discharge of infants and risk of readmission for jaundice. Pediatrics 2015;135:314-21. [Crossref] [PubMed]
- Reed RA, Morgan AS, Zeitlin J, et al. Assessing the risk of early unplanned rehospitalisation in preterm babies: EPIPAGE 2 study. BMC Pediatr 2019;19:451. [Crossref] [PubMed]
- Zhou H, Roberts PA, Dhaliwal SS, et al. Risk factors associated with paediatric unplanned hospital readmissions: a systematic review. BMJ Open 2019;9:e020554. [Crossref] [PubMed]
- Kansagara D, Englander H, Salanitro A, et al. Risk prediction models for hospital readmission: a systematic review. JAMA 2011;306:1688-98. [Crossref] [PubMed]
- Piris Borregas S, Torres Valdivieso MJ, Martín-Arriscado C, et al. Model that predicted death or disabilities in premature infants was valid at seven years of age. Acta Paediatr 2019;108:1245-9. [Crossref] [PubMed]