Postnatal age is strongly correlated with the early development of the gut microbiome in preterm infants
Introduction
Overwhelming evidence suggests that the gut microbiome plays an integral role in both maternal and newborn health (1,2). The composition of the maternal microbiome, from both human and mice models, is linked with an infant’s risk of developing adverse events such as autism, allergies, and autoimmune and metabolic diseases (3-5). The infant microbiome impacts neonatal development in multiple aspects, including the development of metabolism, nerve and immune responses (4,6). Further, disturbance of gut microbial composition in early life can elevate the risk of a variety of clinical events, including feeding intolerance, necrotizing enterocolitis, and sepsis (6-8).
The early postpartum period, which refers to the period of early life in which breastfeeding predominates, is a critical period of time for the establishment of the gut microbiota (6,9). Studies have found the gut microbiome profile of infants to be time dependent, with specific types of microbes enriched at different stages of early-life development (6,10,11). Further, many events in early life, such as delivery by caesarean section, formula feeding, and exposure to antibiotics, can perturb the composition of the gut microbiota (12-15). For instance, Bifidobacterium and Lactobacillus are enriched in breast-fed infants compared to those who are fed exclusively with formula. However, at 1 week of age, the gut microbiota of neonates delivered by cesarean section is characterized by high levels of Clostridium, but not Bifidobacterium and Bacteroides, compared with that of infants delivered by spontaneous vaginal delivery (13,16). Meanwhile, antimicrobial agents often reduce gut bacterial diversity and disrupt the ecological balance of the neonatal microbiome (12,17-19).
Preterm infants have a distinct gut microbial community compared to full-term infants, due to their congenital developmental immaturity and exposure to complex clinical interventions. Multiple factors may influence the initial colonization, development, and function of preterm infants’ gut microbiota, review of the literature revealed that evidence converged on the effect of age, mode of delivery, use of antibiotics, and consumption of human milk in the composition of gut microbiota of preterm infants (6,17,20). The colonization and dynamic changes in the gut microbiome of preterm infants have attracted much attention (7,12,17,21-23). However, large-scale cross-sectional and longitudinal studies yielded inconsistent results regarding the principle of the development of the gut microbiome in preterm infants. In particular, time-related factors including postnatal age (Age), gestational age (GA) at birth, and postmenstrual age (PMA; the combination of GA at birth and postnatal age), showed varied patterns in their relationships with gut microbiome development in preterm infants (24-28). These three different time scales reflect impacts either from the congenital development or from external interventions after birth. A clear understanding of the early-life developmental trajectories of the gut microbiome of premature infants has yet to be obtained. Therefore, in the present study, we examined the relationships of gut microbiome dynamics with Age, PMA, and GA in preterm infants utilizing dense sampling times in a relatively large-scale prospective cohort.
We present the following article in accordance with the MDAR reporting checklist (available at https://dx.doi.org/10.21037/tp-21-367).
Methods
Study participants
A cohort of preterm infants was prospectively enrolled from Nanfang Hospital, Southern Medical University. The basic demographic information of the infants, including sex, GA, delivery method, and medication, was collected from the medical record system by trained researchers.
All participating infants included in this study had a GA of less than 37 weeks and their parents were willing to consent to all aspects of the study protocol. Participants were excluded if they had known chromosomal abnormalities or genetic metabolic diseases.
All samples were obtained from infants hospitalized in the neonatal intensive care unit (NICU) at Nanfang Hospital, Southern Medical University. The study was conducted in accordance with the Declaration of Helsinki (as revised in 2013). The study was approved from the Medical Ethics Committee of Nanfang Hospital (approval No. NFEC-2021-054) and informed consent was signed from the parents of all registered infants. The study was enrolled in the Chinese Clinical Trial Register (trial registration No. ChiCTR2100044469).
Sample collection and processing
Fecal samples were collected from the infants from birth until discharge. After being collected from diapers, the fresh fecal samples were promptly stored at –40 °C and were transported to a laboratory within 3 days, where they were stored at –80 °C until DNA extraction.
DNA extraction and 16S ribosomal RNA gene sequencing
The QIAamp Mini Kit was used for DNA extraction in line with the manufacturer’s instructions. The V4 hypervariable region of the 16S ribosomal RNA (rRNA) gene was amplified and sequenced using an Illumina ISEQ 100 platform.
Bioinformatics
Raw fastq files were split into single-sample paired-end fastq files according to their barcode sequences using laboratory-developed scripts. Each sample sequencing read was denoised with DADA2 (version 1.6.0), to generate amplicon sequence variants (ASVs). The representative sequences were aligned using PyNAST algorithms and a phylogenetics tree was built with FastTree. Taxonomy was assigned to each ASV using the Ribosomal Database Project classifier with default parameters and the GreenGenes (version 13.8) database in QIIME (version 1.9.1). The ASVs from reagent controls were excluded from downstream analysis. To avoid possible bias caused by variation in different samples’ sequencing depth, each sample was rarefied to 3,180 sequences, and those with fewer than 3,180 sequences were excluded from downstream analysis.
Statistical analysis
All subsequent biostatistical analyses were performed using QIIME (version 1.9.1) software or R language (version 4.0.5). Alpha diversity [Shannon index and phylogenetic diversity (PD) whole-tree index] and beta diversity dissimilarity matrices (unweighted and weighted UniFrac distance) were calculated based on ASV level abundance. Principal coordinate analysis (PCoA) was used to display differences among samples based on beta diversity dissimilarity matrices, and permutational multivariate analysis of variance (PERMANOVA) with 999 permutations was used to estimate the statistical significance of group-wise beta diversity. Linear discriminant analysis Effect Size (LEfSe) was applied to compare the discriminative data and to identify bacteria displaying alterations in abundance between different Age groups. Only bacteria with a linear discriminant analysis (LDA) value of >4 (representing the difference in the feature between groups) and those in the top 10 for abundances were displayed.
Random forest modeling was adopted to regress the relative abundance of bacterial genus present in at least 10% samples against Age, GA, and PMA, using the R packages ‘randomForest’ (version 4.6.12) and ‘caret’ (version 6.0.68) to tune hyperparameters and perform 10-fold cross-validation. For each model trained, we evaluated the performance of the regression by calculating the Pearson correlation coefficient between the actual and predicted values. To explore the longitudinal change in each bacteria, using the R package ‘lmerTest’ (version 3.1.3), we used a linear mixed-effects (LME) model to fit the relative abundance of each bacteria at the genus level by taking the Age and GA as fixed effects and the participant as a random effect.
Between-group comparisons were performed using the Wilcoxon’s rank-sum test for two groups and the Kruskal-Wallis test for multiple groups. Benjamini-Hochberg’s false discovery rate (FDR) method was adopted to adjust for multiple comparisons. A P value or FDR <0.05 was considered statistically significant.
Results
Characterization of the study participants and sequencing
The present study enrolled 151 preterm infants, with GAs ranging from 26 to 36 weeks (29.9±2.2 weeks) (Table 1). Fecal samples were collected from the infants from birth until discharge (Figure 1). A total of 604 samples were obtained for sequencing to obtain their microbiome profiles. The mean birth weight was 1.35±0.37 kg, suggesting that most of the infants had a low weight at birth. A total of 7,562,303 high-quality sequences were obtained, and the median read count of each sample was 11,650 (range, 756–43,762). After denoising with DADA2, 9,596 ASVs were obtained. After rarefying the samples to 3,180 sequences, 604 samples and 8,826 ASVs were retained for downstream analysis.
Table 1
Variables (n=604) | Values [mean ± SD or n (%)] |
---|---|
Gestational age (weeks) | 29.9±2.2 |
Postmenstrual age (weeks) | 32.0±2.6 |
Birth weight (kg) | 1.35±0.37 |
Sex, n (%) | |
Female | 165 (27.32) |
Male | 439 (72.68) |
Delivery mode, n (%) | |
Cesarean section | 426 (70.53) |
Vaginal delivery | 178 (29.47) |
Received antibiotics, n (%) | |
Yes | 575 (95.20) |
No | 19 (3.15) |
NA | 10 (1.65) |
Age† group, n (%) | |
T1 (days 1–4)‡ | 158 (26.16) |
T2 (days 5–15) | 222 (36.75) |
T3 (days 16–22) | 92 (15.23) |
T4 (after day 23) | 132 (21.86) |
PMA§ group, n (%) | |
26–27 weeks | 20 (3.31) |
28–29 weeks | 81 (13.41) |
30–31 weeks | 140 (23.18) |
32–33 weeks | 209 (34.60) |
After 34 weeks | 154 (25.50) |
GA¶ group, n (%) | |
26–27 weeks | 105 (17.38) |
28–29 weeks | 152 (25.17) |
30–31 weeks | 202 (33.44) |
32–33 weeks | 108 (17.88) |
After 34 weeks | 37 (6.13) |
†, Age, postnatal age; ‡, T1: the first group of Age is defined as D1 to D4 to include samples within 72 hours after birth; §, PMA: postmenstrual age; ¶, GA: gestational age.
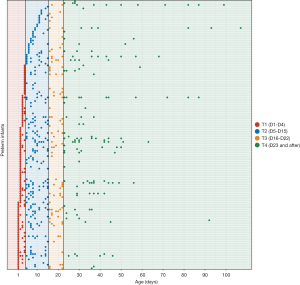
The gut microbiome of preterm infants is most closely related to Age
According to the PCoA with unweighted UniFrac distance, Age showed the most obvious correlation with dynamic changes in the gut microbiome (Figure 2A-2C). In comparison, the microbiome was distributed evenly in relation to GA, with no obvious clustering patterns (Figure 2C). For PMA, a clustering pattern was also visible, although it was less obvious than that of Age (Figure 2B). Multivariate PERMANOVA analysis indicated Age to be a significant contributor to microbiome variation; importantly, its influence was more significant than that of GA and PMA, and far more significant than that of delivery method and the duration of antibiotics.

According to the alteration pattern of the gut microbiome, we divided Age into 4 subgroups (T1–T4), and PMA and GA into 5 subgroups (Table 1, Figure 2D-2F). We observed that the samples collected on day 1 to day 4 were clustered together, reflecting a unique composition of the meconium microbiome (T1, Figure 2D). The most drastic periods of change occurred between day 5 and day 15 (T2), followed by day 16 to day 22 (T3). The gut microbiome post day 23 (T4) was relatively stable compared to that during T2 and T3, and clustered together in the PCoA plot (Figure 2D). The ordination of the microbiome composition showed a clear Age-related pattern, with a negative correlation between Age and the PCo1 axis (Figure 2A,2G). The Shannon index, which represents the richness and evenness of microbiome composition, was highest during T1, decreased rapidly during T2, and stabilized at T3–T4 (Figure 2H). The significant correlations of microbiome signatures with Age, PMA, and GA were also manifested in the Adonis analysis, with Age showing the highest R2 value and GA the lowest (Figure 2I).
Signatures of the gut microbiome at each Age stage
Since the gut microbiome of the preterm infants changed throughout the T1 to T4 stages, we further analyzed the representative microbes in these different time windows. As shown by the class-level histogram in Figure 3A, Enterobacteriaceae, Enterococcaceae, Streptococcaceae, Staphylococcaceae, and Clostridiaceae were the major classes at the late stage of development (with increases from T2 to T4), while Moraxellaceae, Comamonadaceae, Xanthomonadaceae, Sphingomonadaceae, and Mycoplasmataceae were more abundant in the T1 samples. LEfSe analysis was performed to identify bacteria specifically enriched at each stage (Figure 3B). Many environmental and delivery-related microbes, including Sphingomonas, Burkholderia, Acinetobacter, and Stenotrophomonas, were enriched in the meconium stage. During the T2 stage, Bifidobacterium was slightly enriched. In comparison, Streptococcus and Enterococcus were elevated through the T2 to T4 stages, being most abundant at the T3 stage, while Enterobacteriaceae was predominate in T4 samples.
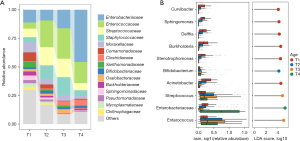
Machine learning model to predict Age, PMA, and GA based on the gut microbiome composition
We next sought to determine whether Age, PMA, and GA can be predicted based on the gut microbiome composition. The prediction may reflect both external and internal selective pressures on the gut microbiome. We established a random forest regression model to correlate the relative abundance of gut microbiota with Age, PMA, and GA in the preterm infants (Figure 4). The correlation coefficient between the predicted and actual Age was 0.6837 (P<0.001, Figure 4A), while those for PMA and GA were 0.5282 (P<0.001) (Figure 4C) and 0.3810 (P<0.001) (Figure 4E), respectively. The top microbial contributors in the three time scales differed across their respective random forest models. Three taxa among the top 10 microbial contributors were shared between the models for Age and GA, namely Enterobacteriaceae, Klebsiella, and Streptococcus. Multiple taxa such as Enterococcus (100.00), Burkholderia (72.61), Clostridium (65.60), Stenotrophomonas (62.30), Delftia (50.81), Caulobacteraceae (46.49), and Staphylococcus (45.82), specifically contributed to Age but had no significant predictive value for GA (Figure 4B). However, Rothia (62.80), Sphingomonas (61.16), Sediminibacterium (53.12), Lactobacillales (46.96), Curvibacter (44.97), Lactobacillus (36.67), and Acinetobacter (36.41) specifically contributed to GA (Figure 4F). The model for PMA shared many taxa with both models, suggesting that the correlation of PMA with gut microbiome could be a combination of effects from Age and GA. Two taxa, Bifidobacterium (77.38) and Escherichia (68.76), specifically contributed to PMA (Figure 4D).
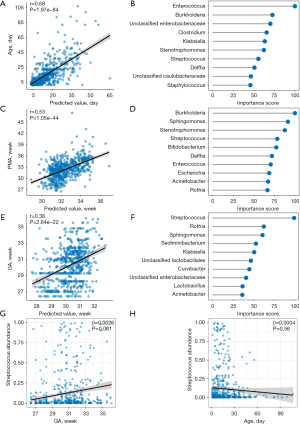
Based on our observation that Age had a remarkable influence on the microbiome, we further evaluated the correlation between the microbiome and GA using an LME model, with Age as a confounding factor (Figure 4G,4H). Streptococcus, the top microbial contributor to GA in the original model, remained significantly associated with GA after adjustment for Age (r=0.0026, P=0.0015), indicating that its association with GA was independent of Age.
Discussion
The immaturity of the intestine and immune system is a physiological feature of preterm infants that has an innate influence on the development of their gut microbiome. Such immaturity is gradually ameliorated as the GA increases. Preterm infants experience many physiological, medical, dietary, and environmental challenges after birth. Meanwhile, both innate and acquired factors simultaneously affect gut microbial colonization in preterm infants (7,29), which is related to the occurrence and development of diseases (30,31). Therefore, understanding the dynamic pattern of the gut microbiome in early life, particularly in relation to different time scales, namely Age, GA, and PMA, can provide insights into the importance of internal and external factors to infants’ early development.
The present study suggests that among the three time scales, Age has the strongest influence on dynamic structural changes of the gut microbiome, while GA has the weakest. In their study of 82 preterm infants, Grier et al. found that the gut microbiome of the infants could be grouped into different community state types (32), which were correlated with different infant maturity measurement indicators (birth GA, week age, and PMA). Chernikova et al. analyzed the alpha diversity of the gut microbiome of 30 preterm infants from an NICU and concluded that there were significant associations between the microbiome and different GAs (26). Based on fecal samples, Yee et al. studied 83 preterm infants’ microbiota in the first 6 weeks after birth and found that the alpha diversity of the gut microbiome was positively correlated with PMA (33). In agreement with these findings, the present study demonstrated that GA and PMA were significantly correlated with the development of the gut microbiome in the early life of preterm infants (Figure 2B,2C). However, both GA and PMA exhibited a weaker association with the microbiome than did Age, and time-dependent changes in the gut microbiome were not affected by sex, delivery method, or even the course of antibiotic medication. Our results revealed a dynamic gut microbiome pattern that constantly changes with time after birth. The finding that the microbiome exhibited a much weaker correlation with GA than Age indicates that the various clinical interventions prescribed to preterm infants could predominate over the characteristics of innate development. Our results are consistent with the view of Rao et al. that the gut microbiota of preterm infants develops in a predicable manner (28), and provide further evidence for the time-dependent pattern of microbiome development.
Based on the dynamic gut microbiome patterns, we further divided the early life of the preterm infants into four stages. Importantly, the microbiome showed distinct characteristics at each of the four stages. During the T1 (meconium) stage, gut microbiome diversity was relatively high, with the community predominated by Proteobacteria. However, the alpha-diversity rapidly declined during the T2 stage (D5–D15), with taxa such as Enterococcus and Streptococcus significantly enriched. The microbial diversity was further reduced at the T3 (D16–D22) and T4 (D23 and after) stages, with Streptococcus and Enterobacteriaceae becoming the signature taxa, respectively. This transition pattern is similar to several previous reports (25,28), and suggests that the gut microbiome follows certain development and evolutionary trajectories in the early life of preterm infants (25,28,34). Interestingly, in our cohort, the gut microbiome showed relatively high diversity throughout the entire T1 period, despite almost all of the infants commencing broad-spectrum antibiotics within one hour after birth. While looking into the details of the microbiome composition at the T1 stage, many of the microbes have been reported to be correlated with perinatal period events. For instance, elevated levels of Ureaplasma, Streptococcus, Proteus, or Stenotrophomonas are often associated with increased pregnancy risks (5,35). As nearly 25% of preterm infants are born to mothers with an intrauterine infection and occult microbial invasion of the amniotic cavity (36), the presence of these genera in infants’ microbiomes, as observed in our results, could possibly reflect intrauterine colonization from their mothers. Furthermore, most preterm infants delivered by cesarean section have a higher abundance of Proteobacteria than do full-term infants (12,17,25,34), which is consistent with our finding that Proteobacteria was predominant during the T1 stage. It should be noted that our results differ from previous reports that the gut microbiome of newborns during early life had low diversity or was sterile (12,27,37-39). Also, DNA extraction methods may affect the determination of microbiome diversity in low biomass samples, which should be further studied in future. A standardized protocol for DNA extraction from fecal samples may be ideal to decrease variability due to DNA extraction, but using standardized protocols will still not prevent other inter-laboratory differences.
The T2 time window witnessed marked alterations of the gut microbiome. The α-diversity dropped significantly, and Bifidobacterium was slightly enriched, implying a trend of the natural development of the newborn gut microbiome being rapidly interrupted by environmental factors, including medical treatments. Importantly, clinically, this period is key for the development of many complications in preterm infants, such as infection, feeding intolerance, and white matter damage, suggesting that possible relationships exist between gut microbiome dysbiosis and health conditions and their prognosis. The dramatic alteration of the gut microbiome at the T2 stage supports the idea that this stage could be a crucial developmental window which likely has a long-term impact on an individual’s health. Microbial diversity continued to decrease during the T3 and T4 stages, with the replacement of commensal bacterial colonization by opportunistic pathogens such as Enterococcus, Streptococcus, and Enterobacteriaceae.
The random forest regression models to predict Age, PMA, and GA using the microbiome further reinforced our finding that the development of preterm infants’ gut microbiome in early life may follow specific, Age-dependent trajectories. Our results are congruent with those of Hill et al., who found that the microbiome was similar at 1 week of age, regardless of GA (12). Innate development (which is related to GA) certainly affects the gut microbiome, but we found the influence of GA to be much weaker than that of Age. The finding that PMA has a far stronger association than GA with the microbiome is likely due to its incorporation of Age-related factors. Therefore, we reason that postnatal clinical interventions, rather than the natural development of the gut, has major impact on the early-stage development of the gut microbiome in preterm infants. In clinical practice, GA can be used to assess the maturity of congenital development in preterm infants, which may impact prognosis (26,30). We found that Streptococcus was highly correlated with GA, and importantly, this correlation was independent of Age. The relative abundance of Streptococcus increased with the increase of GA at birth. The relationship between GA and microbiota could be helpful in evaluating the postnatal development of preterm infants after birth in clinic, and needs to be validated in a larger population cohort.
There are several limitations to this study. First, we divided Age into four stages according to the characteristics of the gut microbiome. Although signature taxa were detected for each stage, whether such division is optimal in our cohort, and in other independent cohorts of preterm infants, warrants further investigation. It is also be important to further explore the relevance of each of the four stages with key clinical events, such as development, disease, and prognosis. Second, due to the observational nature of this cohort study, almost all of the participants had used antibiotics for more than 7 days. Even though our study enrolled 151 preterm infants, we may still be underpowered to precisely assess the various confounding effects (such as antibiotic usage) on our observations due to the extreme complexity of the diseases and treatments of preterm infants. It should, however, be noted that Kim et al. found that the gut microbiome of preterm infants treated with antibiotics did not differ from that in the non-treated group (40). Larger longitudinal cohorts with a prospective, interventional study design are needed to better address these issues.
Conclusions
The present study showed that the composition of the gut microbiome in preterm infants in early life is Age dependent, with the microbiome-Age correlations being significantly greater than those between the microbiome and GA or PMA. The early-life development of the gut microbiome in preterm infants can be divided into four stages: the gut microbiome is highly diverse in the T1 stage, alters rapidly in the T2 stage as its diversity declines, and transits to dysbiotic states in the T3 and T4 stages, with opportunistic pathogens becoming predominate. Our results suggest that clinical interventions, rather than natural gut development, contributes most to the early development of the gut microbiome in preterm infants. Further large-scale cohort studies would be useful to clarifying the prognostic value of the early dynamics of the gut microbiome for clinical outcomes and lateral developments.
Acknowledgments
Funding: The work was supported by the National Key Research and Development Program of China (grant No. 2019YFA0802300 to YH) and the National Natural Science Foundation of China (grant No. 81925062 to HZ). The funders had no role in the study design, data collection and analysis, decision to publish, or preparation of the manuscript.
Footnote
Reporting Checklist: The authors have completed the MDAR reporting checklist. Available at https://dx.doi.org/10.21037/tp-21-367
Data Sharing Statement: Available at https://dx.doi.org/10.21037/tp-21-367
Conflicts of Interest: All authors have completed the ICMJE uniform disclosure form (available at https://dx.doi.org/10.21037/tp-21-367). The authors have no conflicts of interest to declare.
Ethical Statement: The authors are accountable for all aspects of the work in ensuring that questions related to the accuracy or integrity of any part of the work are appropriately investigated and resolved. The study was conducted in accordance with the Declaration of Helsinki (as revised in 2013). The study was approved from the Medical Ethics Committee of Nanfang Hospital (approval No. NFEC-2021-054) and informed consent was signed from the parents of all registered infants. The study was enrolled in the Chinese Clinical Trial Register (trial registration No. ChiCTR2100044469).
Open Access Statement: This is an Open Access article distributed in accordance with the Creative Commons Attribution-NonCommercial-NoDerivs 4.0 International License (CC BY-NC-ND 4.0), which permits the non-commercial replication and distribution of the article with the strict proviso that no changes or edits are made and the original work is properly cited (including links to both the formal publication through the relevant DOI and the license). See: https://creativecommons.org/licenses/by-nc-nd/4.0/.
References
- McDonald B, McCoy KD. Maternal microbiota in pregnancy and early life. Science 2019;365:984-5. [Crossref] [PubMed]
- Wang J, Zheng J, Shi W, et al. Dysbiosis of maternal and neonatal microbiota associated with gestational diabetes mellitus. Gut 2018;67:1614-25. [Crossref] [PubMed]
- Lammert CR, Frost EL, Bolte AC, et al. Cutting Edge: Critical Roles for Microbiota-Mediated Regulation of the Immune System in a Prenatal Immune Activation Model of Autism. J Immunol 2018;201:845-50. [Crossref] [PubMed]
- Vuong HE, Pronovost GN, Williams DW, et al. The maternal microbiome modulates fetal neurodevelopment in mice. Nature 2020;586:281-6. [Crossref] [PubMed]
- Wang S, Ryan CA, Boyaval P, et al. Maternal Vertical Transmission Affecting Early-life Microbiota Development. Trends Microbiol 2020;28:28-45. [Crossref] [PubMed]
- Dominguez-Bello MG, Godoy-Vitorino F, Knight R, et al. Role of the microbiome in human development. Gut 2019;68:1108-14. [Crossref] [PubMed]
- Rozé JC, Ancel PY, Marchand-Martin L, et al. Assessment of Neonatal Intensive Care Unit Practices and Preterm Newborn Gut Microbiota and 2-Year Neurodevelopmental Outcomes. JAMA Netw Open 2020;3:e2018119 [Crossref] [PubMed]
- Warner BB, Deych E, Zhou Y, et al. Gut bacteria dysbiosis and necrotising enterocolitis in very low birthweight infants: a prospective case-control study. Lancet 2016;387:1928-36. [Crossref] [PubMed]
- Hill JH, Round JL. SnapShot: Microbiota effects on host physiology. Cell 2021;184:2796-2796.e1. [Crossref] [PubMed]
- Stanislawski MA, Dabelea D, Wagner BD, et al. Gut Microbiota in the First 2 Years of Life and the Association with Body Mass Index at Age 12 in a Norwegian Birth Cohort. mBio 2018;9:01751-18. [Crossref] [PubMed]
- Odamaki T, Kato K, Sugahara H, et al. Age-related changes in gut microbiota composition from newborn to centenarian: a cross-sectional study. BMC Microbiol 2016;16:90. [Crossref] [PubMed]
- Hill CJ, Lynch DB, Murphy K, et al. Evolution of gut microbiota composition from birth to 24 weeks in the INFANTMET Cohort. Microbiome 2017;5:4. [Crossref] [PubMed]
- Shao Y, Forster SC, Tsaliki E, et al. Stunted microbiota and opportunistic pathogen colonization in caesarean-section birth. Nature 2019;574:117-21. [Crossref] [PubMed]
- D'Agata AL, Wu J, Welandawe MKV, et al. Effects of early life NICU stress on the developing gut microbiome. Dev Psychobiol 2019;61:650-60. [Crossref] [PubMed]
- Vandenplas Y, Carnielli VP, Ksiazyk J, et al. Factors affecting early-life intestinal microbiota development. Nutrition 2020;78:110812 [Crossref] [PubMed]
- Chernikova DA, Koestler DC, Hoen AG, et al. Fetal exposures and perinatal influences on the stool microbiota of premature infants. J Matern Fetal Neonatal Med 2016;29:99-105. [Crossref] [PubMed]
- Aguilar-Lopez M, Dinsmoor AM, Ho TTB, et al. A systematic review of the factors influencing microbial colonization of the preterm infant gut. Gut Microbes 2021;13:1-33. [Crossref] [PubMed]
- Gibson MK, Wang B, Ahmadi S, et al. Developmental dynamics of the preterm infant gut microbiota and antibiotic resistome. Nat Microbiol 2016;1:16024. [Crossref] [PubMed]
- Li X, Stokholm J, Brejnrod A, et al. The infant gut resistome associates with E. coli, environmental exposures, gut microbiome maturity, and asthma-associated bacterial composition. Cell Host Microbe 2021;29:975-987.e4. [Crossref] [PubMed]
- Grier A, Qiu X, Bandyopadhyay S, et al. Impact of prematurity and nutrition on the developing gut microbiome and preterm infant growth. Microbiome 2017;5:158. [Crossref] [PubMed]
- Gasparrini AJ, Wang B, Sun X, et al. Persistent metagenomic signatures of early-life hospitalization and antibiotic treatment in the infant gut microbiota and resistome. Nat Microbiol 2019;4:2285-97. [Crossref] [PubMed]
- Olm MR, Crits-Christoph A, Bouma-Gregson K, et al. inStrain profiles population microdiversity from metagenomic data and sensitively detects shared microbial strains. Nat Biotechnol 2021;39:727-36. [Crossref] [PubMed]
- Hesla HM, Stenius F, Jäderlund L, et al. Impact of lifestyle on the gut microbiota of healthy infants and their mothers—the ALADDIN birth cohort. FEMS Microbiol Ecol 2014;90:791-801. [Crossref] [PubMed]
- Korpela K, Blakstad EW, Moltu SJ, et al. Intestinal microbiota development and gestational age in preterm neonates. Sci Rep 2018;8:2453. [Crossref] [PubMed]
- Ho TTB, Groer MW, Kane B, et al. Dichotomous development of the gut microbiome in preterm infants. Microbiome 2018;6:157. [Crossref] [PubMed]
- Chernikova DA, Madan JC, Housman ML, et al. The premature infant gut microbiome during the first 6 weeks of life differs based on gestational maturity at birth. Pediatr Res 2018;84:71-9. [Crossref] [PubMed]
- Liu CJ, Liang X, Niu ZY, et al. Is the delivery mode a critical factor for the microbial communities in the meconium? EBioMedicine 2019;49:354-63. [Crossref] [PubMed]
- Rao C, Coyte KZ, Bainter W, et al. Multi-kingdom ecological drivers of microbiota assembly in preterm infants. Nature 2021;591:633-8. [Crossref] [PubMed]
- Olin A, Henckel E, Chen Y, et al. Stereotypic Immune System Development in Newborn Children. Cell 2018;174:1277-1292.e14. [Crossref] [PubMed]
- Pierrat V, Marchand-Martin L, Marret S, et al. Neurodevelopmental outcomes at age 5 among children born preterm: EPIPAGE-2 cohort study. BMJ 2021;373: [PubMed]
- Agirman G, Hsiao EY. SnapShot: The microbiota-gut-brain axis. Cell 2021;184:2524-2524.e1. [Crossref] [PubMed]
- Grier A, McDavid A, Wang B, et al. Neonatal gut and respiratory microbiota: coordinated development through time and space. Microbiome 2018;6:193. [Crossref] [PubMed]
- Yee AL, Miller E, Dishaw LJ, et al. Longitudinal Microbiome Composition and Stability Correlate with Increased Weight and Length of Very-Low-Birth-Weight Infants. mSystems 2019; [Crossref] [PubMed]
- Chu DM, Ma J, Prince AL, et al. Maturation of the infant microbiome community structure and function across multiple body sites and in relation to mode of delivery. Nat Med 2017;23:314-26. [Crossref] [PubMed]
- Ma C, Du J, Dou Y, et al. The Associations of Genital Mycoplasmas with Female Infertility and Adverse Pregnancy Outcomes: a Systematic Review and Meta-analysis. Reprod Sci 2021; Epub ahead of print. [Crossref] [PubMed]
- Tamburini S, Shen N, Wu HC, et al. The microbiome in early life: implications for health outcomes. Nat Med 2016;22:713-22. [Crossref] [PubMed]
- Kennedy KM, Gerlach MJ, Adam T, et al. Fetal meconium does not have a detectable microbiota before birth. Nat Microbiol 2021;6:865-73. [Crossref] [PubMed]
- Yang J, Yao S, Cheng K, et al. Comparison of Meconium Microbiome in Dizygotic and Monozygotic Twins Born by Caesarean Section (CS). Front Microbiol 2020;11:1139. [Crossref] [PubMed]
- de Goffau MC, Lager S, Sovio U, et al. Human placenta has no microbiome but can contain potential pathogens. Nature 2019;572:329-34. [Crossref] [PubMed]
- Kim CS, Grady N, Derrick M, et al. Effect of Antibiotic Use Within First 48 Hours of Life on the Preterm Infant Microbiome: A Randomized Clinical Trial. JAMA Pediatr 2021;175:303-5. [Crossref] [PubMed]
(English Language Editor: J. Reynolds)