Examining impulsivity and risky decision making among school youth in balloon analogue risk task
Introduction
From 15 to 25 years of age is a period of increasing risky behavior, such as smoking, binge drinking, physical violence, and self-harm. Risk taking is even more likely to increase between 13 and 17 years (1,2). Impulsivity trait defined as responding with a deficient assessment of the context, which referred to inability to wait for a delayed potential reward, or the rapid response model (3). In behavioral measured evidence, impulsivity is mainly caused by inability to wait for a delayed reward but inclination to rapid response without adequate assessment of potential risk. Advances in cognitive neuroscience have shown that the interaction of emotion and executive control helps to explain the phenomenon of risk taking in young adulthood. Risk taking tendencies are emphasized in the individual pursuit of potential rewarding stimuli and can be motivated by novelty-seeking (4,5). In line with this suggestion, cognitive theory has increasingly shown that risky behavior is linked to change in the prefrontal cortex (PFC) and some limbic brain areas, and dopamine (DA) input to these areas (6,7). In particular, research shows that the PFC is developed during adolescence, when about fifty percent of all synapses are lost (8), different receptors (including DA receptors) are overproduced and pruned (9), fiber density in the PFC becomes higher than both before and after 15–20 years (10), and the mesocortical DA input is higher, while subcortical DA activity is lower (11). Based on these suggestions that rewarding stimuli and novelty-seeking are drivers of risk taking behavior for adolescence, their prone to risk behavior mainly attribute to the brain’s biological mechanisms that DA system plays an important function of adjust the balances between take-risk and reward stimuli. Theoretically, the dual-process model theory provides two neural systems to explain an individual making a risky decision (12). The model considers the affective system to be automatic and spontaneous and driven by the midbrain dopaminergic center, however, the analytic decision system depends on high order brain structures that are involved in anterior cingulate cortex (ACC) and prefrontal cortex (PFC) (13). The models hypothesize a “hot” experiential system that refers to when the affective system is inclined by arousal toward risk taking and stimulates an individual to make an impulsive or emotional decision (14-16). The model also hypothesizes a “cold” system that refers to the analytic decision system which is involved in rational decision making due to its promise of short-term rewards (17,18). Many studies have found that activation of the fronto-limbic circuits is a brain area related to reward, which involves the ventromedial prefrontal cortex (vmPFC), the amygdala, and the insula (19,20). The hot impulsive system mentioned above is significantly driven by the urge to seek novelty and reward. These findings are in line with the suggestion that an unbalanced DA reward system may lead to risk-prone behavior in adolescence and that the imbalance can be modulated in late adolescence and adulthood (21,22). This prolonged maturation provides an extended window for external environment factors to exert influence on the development of an individual’s cognitive control ability, which has downstream consequences for the adolescent involved in risky behaviors (23,24).
Our study combines used event-related potential (ERP) and BART to explore the cognitive mechanism of risk taking decision in school youth. ERP are induced exogenous by environmental stimuli (such scenario events or sensory stimuli) or endogenous by cognitive processes (such as decision making or conscious attention), and also ERP is a real-time record tool the underlying neural process during a psychological experiment task. Impulsivity can be measured by personality questionnaire, while laboratory trail in rewarding discounting model can provide behavioral and cognitive manifestation. BART is a computer-based tool, which designed the subjects engage in risk-taking pump balloon and would respond an index to reflect the degree of risk-taking. Convincingly, Lejuez et al. (25) evaluated the task experimental validity, the evidence shown that behavioral BART score is closely correlated with risky related trait and also with risky taking behaviors in real world. These similar results also examined in other research especially in young sample with sensation seeking (26) impulsivity (27), and alcoholism (28).
Two ERP components: P300 and feedback-related negativity (FRN, were elicit in BART task, which have been found to be related to risk taking and risky decision making. P300 amplitude is feedback related reward processing and positivity occurring at 300–400 ms, and which is thought to reflect noradrenergic system and locus coeruleus activity when the evaluation immediate stimulus and resulting decision making (29). Several researchers revealed (30,31) that P300 is sensitive to the magnitude of reward, that means a more positive-going P300 linked to a larger reward. FRN is a typical ERP response to the loss or gain outcome in 250–350 ms time window, it has been found to be sensitive to outcome valence, that means the more violation of expectation will elicit more negative FRN (32). FRN is identified to originate from the anterior cingulated cortex (ACC), so it may reflect the mechanisms of stimuli monitoring and controlling behavior (33,34).
In light of these considerations, our research is a laboratory-based ERP experiment. BART task was used to examine the neural processes related to risk taking decision making in a sample of 15 to 25 years old. RS was investigated using a Chinese version-risk behavior questionnaire for school youth (RBQ-SY). Our samples were recruited from one public high school and one university in Hubei province. The classic BART task was used to explore the role of impulsivity and underlying neural processes involved in risky decision making. The findings are comparison between experiment sample with risk behavior and non-risk behavior and RS sample be more prone to make risky decisions than non-RS sample is expected, to indicate impulsivity trait as a risk factor that led to behavioral and cognitive risk taking in adolescent.
We present the following article in accordance with the MDAR reporting checklist (available at https://tp.amegroups.com/article/view/10.21037/tp-21-594/rc).
Methods
Subjects
All subjects were students enrolled in the public high school and university in Hubei province. They were recruited during a physical and mental health survey for school youth. The sample included 15 males and 16 females who were right-handed adults and ranged in age from 15 to 25 (mean age 21.35±3.16 years). We used an experiment vs. control design. The RSQ-SY questionnaire were used to investigate the happen of risk behavior in school youth. The total sample were divided into two groups according to whether happened risk behavior at least three times in a month in the past 1 year. The double-blind method was used to ensure each group subject to displayed authenticity in experiment. The experimental subjects (RS) refer to happened one or more risk behaviors at least three times in a month in the past 1 year. The health control subjects (HC) refer to non-risk behavior happened reach to above criteria. All subjects had normal visual acuity or corrected visual acuity, no color blindness or color weakness, no neurological disorders, no history of mental and brain diseases, no history of drug abuse, and has never participated in a class experiment.
All procedures performed in this study involving human participants were in accordance with the Declaration of Helsinki (as revised in 2013). The study was approved by Third Xiangya Hospital Ethics Committee (Authorization number: 2020-S512). All the participants received oral and written information, and written consent forms were signed by all participants before participating, and for those under age of 18, the informed consents were obtained from their parents/guardians.
Measures
Psychometric measures
The Barratt impulsiveness scale (BIS-11) was used (35). The revised Chinese scale was adopted as a self-report tool to measure impulsiveness trait, and the scale contain 30 items in three dimensions. Cronbach’s ɑ coefficient for the total scale was 0.785.
The Chinese version-risk behavior questionnaire for school youth (RBQ-SY) was compiled items from the Adolescent Health Risk Behavior Monitoring System (YRBSS) of the United States of America, and the Canadian version of the Risk Behavior Questionnaire (RSQ-A), interviews of the surveyed population. The RSQ-SY was self-report and contain 18 items in five dimensions: (I) aggressive violence; (II) substance dependence; (III) intentional injury; (IV) unsafe sex; and (V) illegal behavior. Cronbach alpha value of RBQ-SY total scale was 0.816.
Balloon analogue risk task (BART)
BART task was proposed by Lejuez et al. (2002) and is a well-established and validated metric of risk-taking propensity (36). BART task included 60 balloons that were classified into three blocks, there was a certain probability that the balloon would burst. Two boxes presented on screen, one is titled “current account” and the other titled “total earned”, which used to notify the participant of whether their accumulated decision was gain or deficit. During the task, the computer screen presented a game with a small, simulated balloon. The participants were instructed to press a button to simulate inflation. Each pump air into the balloon was accompanied a sound to hint the subjects whether decide to stop pumping, if the balloon was not burst, the temporary earnings would be transferred to permanent bank; if the balloon was pumped explosion, the temporary earning would be lost. With the experiment advancing the participant could adjust their decision strategies according to the current account is gain (positive feedback) or lost (negative feedback). Finally, each participant’s BART score was used as their behavior data to examine their risk seeking tendency. The BART score referred to the number of un-burst balloons (UB) divided by the total number of times the participant pressed to inflate the balloon (TP). The higher of BART score means the more inclination to engage in risk-taking.
ERP experiment
The participants were seated 1 m in front of a computer. Each wore a cap with a 64-channel array of electrodes. They were asked to press the left or right button on a keyboard. The task feedback appeared on the screen to report the trial outcome. The feedback screen stayed on until the task was completed. After we acquired each participant’s written informed consent, the experiment started with a direct questionnaire, followed by the placement of an electrode cap. Electroencephalo-graph (EEG) were recorded during in BART trial. All subjects received a small financial remuneration depend on their BART score when finished the experiment.
ERP was recorded was from the 64-channel amplifier and acquisition software (NEUROSCAN 4.5) positioned according to the “10/20 International System”. An offline common reference was computed to limit the problem of signal-to-noise ratio. A ground electrode was located at each subject’s forehead, and a vertical electro-oculogram (VEOG) and a horizontal electro-oculogram (HEOG) were located on the outer side of the participant’s eyes. Continuous EEG readings were recorded using a sampling rate of 1,000 Hz and a digital bandpass filtered from 0.1 to 30 Hz. The impedance of all electrodes was kept below 5 Ω. EEG epochs were extracted from 200 ms before to 1,000 ms after presentation of the outcome stimulus, then were corrected based on a baseline. the EEG in different experimental conditions were averaged as ERPs results.
We analyzed two early components: P300 and FRN. These typically emerged in risk taking tasks and have been shown to be highly sensitive to manipulations of reward parameters (37,38). EEG recorded at scalp electrodes as follows: P300 corresponding time window is 300–500 ms and located at Pz; FRN corresponding time window is 200–280 ms and located at Fz.
Statistical analysis
We used SPSS 20.0 (IBM Corp, Armonk, NY, USA) for Windows for statistical analysis and set the significant level P values at 0.05. Impulsiveness of BIS score and the BART score were compared between the experimental and control subjects, the significant differences within-group are expected. The RS data were extracted to analyze the correlation among BIS, BART, and ERP components, which were assessed by using Pearson’s correlations coefficient. For ERPs, three-way repeated Analysis of Variance (ANOVA was used to examine the effects of within three factors (group, feedback, block) in a mixed design: 2 groups (RS/HC) ×3 blocks (1/2/3) ×2 feedback (P/N). Simple contrasts were computed to indicate the significant main and interaction effects. We carried out Mauchly’s test for the assumption of sphericity and Levene’s test for the assumption of homogeneity of variances.
Results
Questionnaire findings
The questionnaire data are summarized in Table 1. The mean score of the BART and BIS conducted independent t-tests is also shown. Comparison of mean score between RS and HC subjects, the results demonstrated a significant difference in BART score (t=9.36, P=0.031), and in BIS (t=12.03, P=0.024).
Table 1
Total score | RS (n=16) | HC (n=15) | Test statistic | P value |
---|---|---|---|---|
BART | 21.32±2.10 | 17.55±3.14 | 9.36 | 0.031 |
BIS | 25.31±5.71 | 17.63±6.52 | 12.03 | 0.024 |
BART, balloon analogue risk task; BIS, Barratt impulsiveness scale; RS, experimental subjects with risk behavior; HC, health control subjects without risk behavior.
Pearson’s correlation test was conducted among variables of BART, BIS, and EEG components. Results are shown in Table 2. There was significantly positive relation between BART and BIS (r=0.711, P<0.01), which suggests that greater impulsivity was correlated with greater risk-taking behavioral inclination. BIS was significantly negative correlated with two EEG components of P300 (r=−0.533, P<0.05) and FRN (r=−0.556, P<0.01), and the significantly negative correlation also occurred between behavioral BART score and FRN (r=−0.617, P<0.01).
Table 2
Factors | BART | BIS | P300 | FRN |
---|---|---|---|---|
BART | – | – | – | – |
BIS | 0.711** | – | – | – |
P300 | −0.395 | −0.533* | – | – |
FRN | −0.617** | −0.556* | −0.187 | – |
P300 and FRN indicates the average amplitude (μV) of two EEG components that were elicited in the BART. BART, balloon analogue risk task; BIS, Barratt impulsiveness scale; EEG, electroencephalo-graph; FRN, feedback-related negativity. *, P<0.05; **, P<0.01.
ERP findings
The study featured a 2 (group: RS/HC) ×2 (feedback: gain/lost) ×3 (block: 1/2/3), the EEG and behavioral data were designed to compare between experimental subjects (RS) and health control subjects (HC). The average amplitude of P300 and FRN are summarized in Table 3.
Table 3
Block-feedback | P300 | FRN | |||||||||
---|---|---|---|---|---|---|---|---|---|---|---|
HC | RS | HC | RS | ||||||||
Mean | SD | Mean | SD | Mean | SD | Mean | SD | ||||
1-P | 5.20 | 0.25 | 3.82 | 0.23 | 4.34 | 0.16 | 4.34 | 0.16 | |||
1-N | 5.74 | 0.24 | 3.02 | 0.21 | 4.84 | 0.13 | 4.84 | 0.13 | |||
2-P | 4.88 | 0.56 | 2.42 | 0.22 | 4.04 | 0.50 | 4.04 | 0.50 | |||
2-N | 4.32 | 1.13 | 2.76 | 0.26 | 4.22 | 0.18 | 4.22 | 0.18 | |||
3-P | 4.67 | 0.23 | 2.68 | 0.10 | 3.93 | 0.76 | 3.93 | 0.76 | |||
3-N | 4.91 | 0.27 | 3.95 | 0.39 | 4.10 | 0.30 | 4.10 | 0.30 | |||
Total | 4.95 | 0.69 | 3.11 | 0.63 | 4.24 | 0.49 | 4.24 | 0.49 |
P300 and FRN indicates the average amplitude (μV) of two EEG components that were elicited in the BART. RS, experimental subjects with risk behavior; HC, health control subjects without risk behavior; BART, balloon analogue risk task; EEG, electroencephalo-graph.
P300
A repeated ANOVA test revealed a significant main effect and interactive effect. Results are shown in Table 4. The main effect of within-group was {F[1,29] =35.76, P<0.01}. The main effect of within-feedback was {F[1,29] =16.37, P<0.05}. There were significant interaction effect between block and feedback, the result was {F[2,29] =23.69, P<0.01}, and the significant interaction also found in group × block × feedback, the results was {F[2,29] =5.62, P<0.05}. the mean amplitude of P300 were compared between RS and HC, Figure 1 shows that there were totally four blocks demonstrate HC > RS. Figure 2 shows that the EEG waveform for the average amplitude of P300 in HC (indicated by the blue line) displayed a more positive trend than the trend shown by RS (indicated by the red line) under both conditions of positive and negative feedback.
Table 4
Variance factor | P300 | FRN | |||||||
---|---|---|---|---|---|---|---|---|---|
SS | df | MS | F | SS | df | MS | F | ||
a | 85.52 | 1.00 | 85.52 | 35.76** | 41.14 | 1.00 | 41.14 | 16.10** | |
b | 1.55 | 1.00 | 1.55 | 6.37* | 1.50 | 1.00 | 1.50 | 2.00 | |
c | 0.61 | 2.00 | 0.31 | 1.22 | 6.71 | 2.00 | 3.36 | 7.52** | |
a×b | 5.13 | 1.00 | 5.13 | 16.37** | 0.09 | 1.00 | 0.09 | 0.11 | |
a×c | 1.64 | 2.00 | 0.82 | 2.77 | 0.03 | 2.00 | 0.02 | 0.03 | |
b×c | 13.54 | 2.00 | 6.77 | 23.69** | 31.12 | 2.00 | 15.56 | 16.16** | |
a×b×c | 3.83 | 2.00 | 1.92 | 5.62* | 3.88 | 2.00 | 1.94 | 5.50* |
a, group; b, feedback; c, block; *, P<0.05; **, P<0.01. SS, sum of squares from mean; df, degree of freedom; MS, mean square; ANOVA, analysis of variance; P300, positive amplitude at 300 ms; FRN, feedback-related negativity
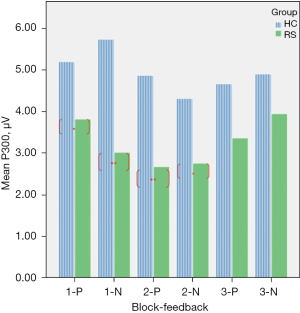
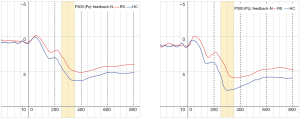
FRN
Table 4 shows that there were significant main effects of within-group: {F[1,29] =16.10, P<0.01}, and main effects of within-block {F[1,29] =7.52, P<0.01}. The significant interaction effect in block × feedback was {F[2,29] =16.16, P<0.01} and in group × block × feedback was {F[2,29] =5.50, P<0.05}. The comparison of the mean amplitude of FRN between RS and HC (Figure 3) shows a significant difference in block 2-N and block 3-N. Figure 4 shows that the EEG waveform for the average amplitude of FRN in HC (indicated by the blue line) was more negative-going than it in RS (indicated by the red line) under negative feedback condition, while RS and HC responded to similarly in positive feedback condition.
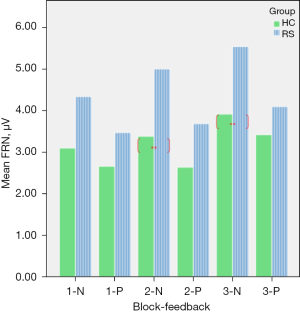
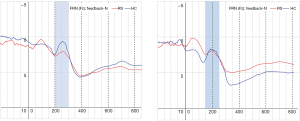
Discussion
Our study firstly investigated the occurrence of risk behavior in school youth which students aged 15 to 25 years, and individuals with risk behavior and non-risk behavior were compared by laboratory risk-taking decision task. Behavioral data and psychological measures were obtained, as such, the evidence was expected to reveal the differences between experiment subjects (RS) and health control subjects (HC) is related to potential impulsivity trait, which overt risk-taking behavior and ERP responses give convincing explanation.
P300 value can be seen as a reliable index of cognitive capability and personality. The current study, when subjects engaged in a series of risk evaluations in BART task, the RS subjects had a higher BIS score than the HC sample, and the RS sample presented a higher BART behavioral score, that the HC sample. We found that the RS sample displayed reduced P300 amplitude. According to the existing research suggestion that a lower P300 is associated with dysfunction of cognitive control. Thus, P300 reduction can be considered a risk factor that reflects disinhibition. Our results of P300 declination were in line with Moeller FG viewpoint (39) that a lower P300 amplitude was correlated with behavioral laboratory impulsivity score. This point can also be verified by the significant positive relationship between BIS and BART (r=0.711). Our results illustrate that impulsivity is a risk factor for risk-taking behavior and that P300 response to risk taking behavior in RS individuals was associated with the reason for impaired cognitive control. Conversely, the correlation between P300 and the impulsivity-related trait can have a zero relation (40), and the positive relation (41). as the positive amplified P300 effect represent the attention and motivational maker of events perceived as significant, and reflect a post feedback evaluation to proactive control the future behavior and increase rewards. Conversely, the lowered P300 amplitudes in our RS sample indicated that individual insensitive to negative feedback and dysfunction to monitor and adjust in process, which in line with higher BART score of RS sample that means higher inclination to risk decision.
The significant negative correlation (r=−0.533) between P300 and impulsiveness trait of BIS score were also analyzed in our study, this evidence is convincing to explain individuals with high impulsivity would have more risky behavior. The negative relation was also observed between P300 and BART (r=−0.395), but without statistic significantly. The negative correlation that emerged between impulsivity and P300 amplitude was consistent with previous studies that sampled participants with psychopathology, for instance, clinical depression (42), conduct disorder (43) and schizophrenia (44). In addition, existing studies have also examined healthy sample with substance use disorders (45), and alcoholism (46), which also found a reduced P300 amplitude. Although these studies support the contention that executive function in the anterior right ventral (ARV) brain region was impaired when an impulsive individual is in a risk taking context, other studies presented converse findings in impulsivity related trait measures, such as sensation seeking (47), novelty seeking (48), indicating that more studies are needed to further explore this issue.
FRN shows a negative deflection at 200–300 ms post-feedback onset (49), and it is likely to be from the neural source of the dorsal anterior cingulate cortex (dACC) (50), which is a region proposed to play an important role in cognitive control for risk taking (51). FRN was typically taken as a component to represent a reward-prediction error which was more positive when outcomes met a person’s expectation and more negative when the outcome was worse than the participant’s expectation (52). Sambrook and Goslin (53) reviewed more than 25 studies in a meta-analysis which shows FRN will be more positive for unexpected rather than expected positive outcomes. Meta-analysis revealed that FRN was a predicted index for the outcome of expectations, for instance, the amplitude of FRN was more positive when outcomes exceeded expectations and more negative when outcomes were worse than expectations (54). FRN depends on both of outcome valence and expectedness (55). Based on this work, we hypothesized that the RS sample would have a more negative FRN than HC sample, because the RS sample would be more sensitive to unexpected outcomes. This hypothesis was supported in our findings, which showed that a significant negative correlation existed between BIS and FRN (r=−0.556) and also between BART behavioral score and FRN (r=−0.617). These results are consistent with Yamaguchi finding (56) that the non-planning score of BIS was negatively correlated with FRN amplitude (r=−0.60) which means individual with higher in impulsivity is more sensitive to unexpected outcome that led to FRN negative-going. making risky choices. In addition, our EEG (Figure 4) showed that the RS sample has a more descending FRN waveform than the HC sample. According to the interpretation of the FRN as a reward prediction error component, it is possible that the RS sample in our research with higher impulsivity, so their risk taking choice could be accounted for maladaptive feedback processing which less evaluate the negative feedback. Similar findings were also reported in neurological and psychiatric clinical samples in which the FRN amplitude was decreased in participants with schizophrenia (57) and alcoholism (58), while the increased FRN amplitude also reported in hyperactivity disorder (59) and depression (60). therefore, FRN can be seen as an indicator for monitor information received from our internal or external cues, and give matched feedback between attended behavior and the error-feedback signal.
Taken together, one of the main findings of current study was that the RS subjects with more risk behaviors that not only reflected in higher score of psychological measurement of the BIS questionnaire, and also obviously in behavioral task (BART score) and EEG components (P300 and FRN). This RS subjects' characteristic was more inclination to risk taking which related to impulsiveness, and impulsivity-related EEG components also demonstrated that activity patterns of P300 is more positive-going than HC subjects, and FRN was more negative-going than HC subjects. The negative correlation between personal impulsivity (BIS, BART) score and cognitive ERP components (P300; FRN) were in line in with existing studies, that provide more evidence to explain that impulsivity trait would put individuals into poor display cognitive control and less sensitive to negative consequence, which lead to be more involve in risk behaviors. The impulsiveness can be seen as age-related trait in youth people, which characteristic involved acting without thinking or non-planning, this problem should be prevented by promote youth awareness of potential risk and reduce environmental lure such as (pornography advertising or violent online).
The limitations and strengths in our study as follow: first, the school-aged youth sample is a small part of total youth people, therefore our conclusion only partially represented a wide spectrum. second, we investigated the risk behavior by RSQ-SY questionnaire that typically contain items from school youth, so the certain age group and campus environment is limitation to predict the whole epidemic of risk behavior in total young people, so the larger samples that include in a variety of sample should be considered to enrich the conclusion in risk behavior in different sample and related different personality. As to neuroscience study, except typically impulsiveness-related components P300 and FRN, other EEG components should be further explored to provide an abundant illustration of this issue. The strength of this study is that BART results have been shown to powerfully predict risk-taking behavior in the real world for multiple populations, behavioral experiment and ERP method can provided convincing evidence for related research, as a basis material to enlighten further research reveal the neural mechanism of risk behavior in real society. Therefor the current study can enlighten the psychological intervention to promote the balanced awareness between potential risk and the immediate reward, the evidence that balanced awareness accompanied with the reduce of risky behavior can be expected in future study.
Conclusions
Our study provides psychological and cognitive evidence that risky decision can be partially attributed to impulsive trait, which lead individual to immediate rewards and relatively deficient in evaluation of long-term consequences. the inclination can display in P300 and FRN reduction, the results can shed light on the future research to explore other EEG components to explain the cognitive mechanism in risk decision.
Acknowledgments
Funding: This work was supported by the Scientific Research Project for young and middle-aged Talents of Hubei Provincial Department of Education under grant number Q20192803.
Footnote
Reporting Checklist: The authors have completed the MDAR reporting checklist. Available at https://tp.amegroups.com/article/view/10.21037/tp-21-594/rc
Data Sharing Statement: Available at https://tp.amegroups.com/article/view/10.21037/tp-21-594/dss
Conflicts of Interest: All authors have completed the ICMJE uniform disclosure form (available at https://tp.amegroups.com/article/view/10.21037/tp-21-594/coif). The authors have no conflicts of interest to declare.
Ethical Statement: The authors are accountable for all aspects of the work in ensuring that questions related to the accuracy or integrity of any part of the work are appropriately investigated and resolved. All procedures performed in this study involving human participants were in accordance with the Declaration of Helsinki (as revised in 2013). The study was approved by Third Xiangya Hospital Ethics Committee (authorization number: 2020-S512). All the participants received oral and written information, and written consent forms were signed by all participants before participating, and for those under age of 18, the informed consents were obtained from their parents/guardians.
Open Access Statement: This is an Open Access article distributed in accordance with the Creative Commons Attribution-NonCommercial-NoDerivs 4.0 International License (CC BY-NC-ND 4.0), which permits the non-commercial replication and distribution of the article with the strict proviso that no changes or edits are made and the original work is properly cited (including links to both the formal publication through the relevant DOI and the license). See: https://creativecommons.org/licenses/by-nc-nd/4.0/.
References
- Ryan BL, Stewart M, Campbell MK, et al. Understanding adolescent and young adult use of family physician services: a cross-sectional analysis of the Canadian Community Health Survey. BMC Fam Pract 2011;12:118. [Crossref] [PubMed]
- Viner RM, Ozer EM, Denny S, et al. Adolescence and the social determinants of health. Lancet 2012;379:1641-52. [Crossref] [PubMed]
- Mungo A, Hein M, Hubain P, et al. Impulsivity and its Therapeutic Management in Borderline Personality Disorder: a Systematic Review. Psychiatr Q 2020;91:1333-62. [Crossref] [PubMed]
- Forbes EE, Dahl RE. Pubertal development and behavior: hormonal activation of social and motivational tendencies. Brain Cogn 2010;72:66-72. [Crossref] [PubMed]
- Slavny RJM, Sebastian CL, Pote H. Age-related changes in cognitive biases during adolescence. J Adolesc 2019;74:63-70. [Crossref] [PubMed]
- Luciana M, Collins PF. Incentive Motivation, Cognitive Control, and the Adolescent Brain: Is It Time for a Paradigm Shift? Child Dev Perspect 2012;6:392-9. [Crossref] [PubMed]
- Wahlstrom D, White T, Luciana M. Neurobehavioral evidence for changes in dopamine system activity during adolescence. Neurosci Biobehav Rev 2010;34:631-48. [Crossref] [PubMed]
- Rakic P, Bourgeois JP, Goldman-Rakic PS. Synaptic development of the cerebral cortex: implications for learning, memory, and mental illness. Prog Brain Res 1994;102:227-43. [Crossref] [PubMed]
- Lidow MS, Goldman-Rakic PS, Rakic P. Synchronized overproduction of neurotransmitter receptors in diverse regions of the primate cerebral cortex. Proc Natl Acad Sci U S A 1991;88:10218-21. [Crossref] [PubMed]
- Vijayakumar N, Op de Macks Z, Shirtcliff EA, et al. Puberty and the human brain: Insights into adolescent development. Neurosci Biobehav Rev 2018;92:417-36. [Crossref] [PubMed]
- Sedgwick O, Young S, Baumeister D, et al. Neuropsychology and emotion processing in violent individuals with antisocial personality disorder or schizophrenia: the same or different? A systematic review and meta-analysis. Aust N Z J Psychiatry 2017;51:1178-97. [Crossref] [PubMed]
- Fried CS, Reppucci ND. Criminal decision making: the development of adolescent judgment, criminal responsibility, and culpability. Law Hum Behav 2001;25:45-61. [Crossref] [PubMed]
- Ruchsow M, Reuter K, Hermle L, et al. Executive control in obsessive-compulsive disorder: event-related potentials in a Go/Nogo task. J Neural Transm (Vienna) 2007;114:1595-601. [Crossref] [PubMed]
- Nelson CA, Bloom FE, Cameron JL, et al. An integrative, multidisciplinary approach to the study of brain-behavior relations in the context of typical and atypical development. Dev Psychopathol 2002;14:499-520. [Crossref] [PubMed]
- Romer D, Hennessy M. A biosocial-affect model of adolescent sensation seeking: the role of affect evaluation and peer-group influence in adolescent drug use. Prev Sci 2007;8:89-101. [Crossref] [PubMed]
- Paulus MP, Rogalsky C, Simmons A, et al. Increased activation in the right insula during risk-taking decision making is related to harm avoidance and neuroticism. Neuroimage 2003;19:1439-48. [Crossref] [PubMed]
- Knoch D, Gianotti LR, Pascual-Leone A, et al. Disruption of right prefrontal cortex by low-frequency repetitive transcranial magnetic stimulation induces risk-taking behavior. J Neurosci 2006;26:6469-72. [Crossref] [PubMed]
- Tom SM, Fox CR, Trepel C, et al. The neural basis of loss aversion in decision-making under risk. Science 2007;315:515-8. [Crossref] [PubMed]
- Li CS, Chao HH, Lee TW. Neural correlates of speeded as compared with delayed responses in a stop signal task: an indirect analog of risk taking and association with an anxiety trait. Cereb Cortex 2009;19:839-48. [Crossref] [PubMed]
- Rao H, Korczykowski M, Pluta J, et al. Neural correlates of voluntary and involuntary risk taking in the human brain: an fMRI Study of the Balloon Analog Risk Task (BART). Neuroimage 2008;42:902-10. [Crossref] [PubMed]
- Crone EA, Dahl RE. Understanding adolescence as a period of social-affective engagement and goal flexibility. Nat Rev Neurosci 2012;13:636-50. [Crossref] [PubMed]
- Blakemore SJ, Mills KL. Is adolescence a sensitive period for sociocultural processing? Annu Rev Psychol 2014;65:187-207. [Crossref] [PubMed]
- Nelson EE, Leibenluft E, McClure EB, et al. The social re-orientation of adolescence: a neuroscience perspective on the process and its relation to psychopathology. Psychol Med 2005;35:163-74. [Crossref] [PubMed]
- Spear LP. The adolescent brain and age-related behavioral manifestations. Neurosci Biobehav Rev 2000;24:417-63. [Crossref] [PubMed]
- Lejuez CW, Read JP, Kahler CW, et al. Evaluation of a behavioral measure of risk taking: the Balloon Analogue Risk Task (BART). J Exp Psychol Appl 2002;8:75-84. [Crossref] [PubMed]
- Paydary K, Mahin Torabi S. Impulsivity, Sensation Seeking, and Risk-Taking Behaviors among HIV-Positive and HIV-Negative Heroin Dependent Persons. AIDS Res Treat 2016;2016:5323256. [Crossref] [PubMed]
- Hunt MK, Hopko DR, Bare R, et al. Construct validity of the Balloon Analog Risk Task (BART): associations with psychopathy and impulsivity. Assessment 2005;12:416-28. [Crossref] [PubMed]
- Rose AK, Jones A, Clarke N, et al. Alcohol-induced risk taking on the BART mediates alcohol priming. Psychopharmacology (Berl) 2014;231:2273-80. [Crossref] [PubMed]
- Ma Q, Wang X, Shu L, et al. P300 and categorization in brand extension. Neurosci Lett 2008;431:57-61. [Crossref] [PubMed]
- Yeung N, Sanfey AG. Independent coding of reward magnitude and valence in the human brain. J Neurosci 2004;24:6258-64. [Crossref] [PubMed]
- Nieuwenhuis S, Aston-Jones G, Cohen JD. Decision making, the P3, and the locus coeruleus-norepinephrine system. Psychol Bull 2005;131:510-32. [Crossref] [PubMed]
- Wu Y, Zhou X. The P300 and reward valence, magnitude, and expectancy in outcome evaluation. Brain Res 2009;1286:114-22. [Crossref] [PubMed]
- Debener S, Ullsperger M, Siegel M, et al. Trial-by-trial coupling of concurrent electroencephalogram and functional magnetic resonance imaging identifies the dynamics of performance monitoring. J Neurosci 2005;25:11730-7. [Crossref] [PubMed]
- Gehring WJ, Willoughby AR. The medial frontal cortex and the rapid processing of monetary gains and losses. Science 2002;295:2279-82. [Crossref] [PubMed]
- Barratt ES, Stanford MS, Kent TA, et al. Neuropsychological and cognitive psychophysiological substrates of impulsive aggression. Biol Psychiatry 1997;41:1045-61. [Crossref] [PubMed]
- Lejuez CW, Aklin WM, Zvolensky MJ, et al. Evaluation of the Balloon Analogue Risk Task (BART) as a predictor of adolescent real-world risk-taking behaviours. J Adolesc 2003;26:475-9. [Crossref] [PubMed]
- Hajcak G, Holroyd CB, Moser JS, et al. Brain potentials associated with expected and unexpected good and bad outcomes. Psychophysiology 2005;42:161-70. [Crossref] [PubMed]
- Martin LE, Potts GF. Impulsivity in Decision-Making: An Event-Related Potential Investigation. Pers Individ Dif 2009;46:303. [Crossref] [PubMed]
- Moeller FG, Barratt ES, Fischer CJ, et al. P300 event-related potential amplitude and impulsivity in cocaine-dependent subjects. Neuropsychobiology 2004;50:167-73. [Crossref] [PubMed]
- Vedeniapin AB, Anokhin AP, Sirevaag E, et al. Visual P300 and the self-directedness scale of the Temperament and Character Inventory. Psychiatry Res 2001;101:145-56. [Crossref] [PubMed]
- Pierson A, Le Houezec J, Fossaert A, et al. Frontal reactivity and sensation seeking an ERP study in skydivers. Prog Neuropsychopharmacol Biol Psychiatry 1999;23:447-63. [Crossref] [PubMed]
- Röschke J, Wagner P. A confirmatory study on the mechanisms behind reduced P300 waves in depression. Neuropsychopharmacology 2003;28:S9-12. [Crossref] [PubMed]
- Bauer LO, Hesselbrock VM. P300 decrements in teenagers with conduct problems: implications for substance abuse risk and brain development. Biol Psychiatry 1999;46:263-72. [Crossref] [PubMed]
- Mathalon DH, Ford JM, Pfefferbaum A. Trait and state aspects of P300 amplitude reduction in schizophrenia: a retrospective longitudinal study. Biol Psychiatry 2000;47:434-49. [Crossref] [PubMed]
- Bauer LO. CNS recovery from cocaine, cocaine and alcohol, or opioid dependence: a P300 study. Clin Neurophysiol 2001;112:1508-15. [Crossref] [PubMed]
- Prabhu VR, Porjesz B, Chorlian DB, et al. Visual p3 in female alcoholics. Alcohol Clin Exp Res 2001;25:531-9. [Crossref] [PubMed]
- Ratsma JE, van der Stelt O, Schoffelmeer AN, et al. P3 event-related potential, dopamine D2 receptor A1 allele, and sensation-seeking in adult children of alcoholics. Alcohol Clin Exp Res 2001;25:960-7. [Crossref] [PubMed]
- Hansenne M. P300 and personality: an investigation with the Cloninger's model. Biol Psychol 1999;50:143-55. [Crossref] [PubMed]
- Miltner WH, Braun CH, Coles MG. Event-related brain potentials following incorrect feedback in a time-estimation task: evidence for a "generic" neural system for error detection. J Cogn Neurosci 1997;9:788-98. [Crossref] [PubMed]
- Hauser TU, Iannaccone R, Stämpfli P, et al. The feedback-related negativity (FRN) revisited: new insights into the localization, meaning and network organization. Neuroimage 2014;84:159-68. [Crossref] [PubMed]
- Steinberg L. A Social Neuroscience Perspective on Adolescent Risk-Taking. Dev Rev 2008;28:78-106. [Crossref] [PubMed]
- Holroyd CB, Coles MGH. The neural basis of human error processing: reinforcement learning, dopamine, and the error-related negativity. Psychol Rev 2002;109:679-709. [Crossref] [PubMed]
- Sambrook TD, Goslin J. A neural reward prediction error revealed by a meta-analysis of ERPs using great grand averages. Psychol Bull 2015;141:213-35. [Crossref] [PubMed]
- Chase HW, Swainson R, Durham L, et al. Feedback-related negativity codes prediction error but not behavioral adjustment during probabilistic reversal learning. J Cogn Neurosci 2011;23:936-46. [Crossref] [PubMed]
- Nieuwenhuis S, Holroyd CB, Mol N, et al. Reinforcement-related brain potentials from medial frontal cortex: origins and functional significance. Neurosci Biobehav Rev 2004;28:441-8. [Crossref] [PubMed]
- Onoda K, Abe S, Yamaguchi S. Feedback-related negativity is correlated with unplanned impulsivity. Neuroreport 2010;21:736-9. [Crossref] [PubMed]
- Morris SE, Heerey EA, Gold JM, et al. Learning-related changes in brain activity following errors and performance feedback in schizophrenia. Schizophr Res 2008;99:274-85. [Crossref] [PubMed]
- Kamarajan C, Rangaswamy M, Tang Y, et al. Dysfunctional reward processing in male alcoholics: an ERP study during a gambling task. J Psychiatr Res 2010;44:576-90. [Crossref] [PubMed]
- Groen Y, Wijers AA, Mulder LJ, et al. Error and feedback processing in children with ADHD and children with Autistic Spectrum Disorder: an EEG event-related potential study. Clin Neurophysiol 2008;119:2476-93. [Crossref] [PubMed]
- Foti D, Hajcak G. Depression and reduced sensitivity to non-rewards versus rewards: Evidence from event-related potentials. Biol Psychol 2009;81:1-8. [Crossref] [PubMed]