Proteomic profiling of serum small extracellular vesicles reveals immune signatures of children with pneumonia
Introduction
Pneumonia contributes significantly to high mortality rates in children worldwide (1). The clinical presentation of viral, M. pneumonia, and other bacterial infections overlap significantly (2), and an understanding of how pathogen infection influences clinical outcomes in pediatric patients is needed. Besides respiratory tract diseases, many pathogens have been known to cause a wide variety of extrapulmonary complications in children (3). However, the causal relation between pathogen infection and the development of diseases is not yet completely understood. Due to differences in host responses induced by different pathogens, an understanding of these responses would allow for better interpretation of disease pathology. For example, it has been shown that host transcriptional profiles revealed immune signatures that distinguished between viral and bacterial infections (4,5).
Small extracellular vesicles (sEVs), with a size range of ~30 to 150 nm, harbor a diversity of functional molecules, including host-derived proteins, nucleic acids, and lipids. In particular, proteins loaded in sEVs exhibit unique characteristics. sEVs originate from multivesicular bodies (MVB) formed by inward invagination of the membrane of late endosomes, therefore endosome- and endocytic vesicle-related proteins and MVB biogenesis-related proteins, such as CD9, CD63, CD81, syntenin-1, ALIX and TSG101, are enriched in sEVs in comparison to cells or other extracellular vesicles (6). Previous proteomic analysis, however, has shown that nuclear, mitochondrial, endoplasmic reticulum and Golgi proteins rarely appeared in sEVs (7). Moreover, sEVs cargo has been demonstrated to include proteins involved in a wide variety of diseases such as cancer-related proteins glypican-1 (8) and integrins (9), and immune-related proteins PD-L1 (10) and viral receptor ACE2 (11). Given that proteins are critical bioactive components of sEVs cargo, sEVs proteins are regarded as a useful source of circulating biomarkers for diagnosing a wide range of diseases (12).
Intercellular communication through sEVs is involved in the pathogenesis of various inflammatory diseases (13). Depending upon the context, sEVs can stimulate or suppress the immune responses to viral and microbial infections (14). The function of sEVs in inflammation regulation maybe due to the transfer of peptide antigens to recipient cells. sEVs from dendritic cells or B cells carry major histocompatibility complex (MHC) molecules, and present peptide antigens to specific T cells to induce their activation (15-17). In addition, immune response can be regulated by sEVs cargos. Previous studies have shown that sEVs mediated the intercellular transfer of antiviral molecules. For example, anti-HIV-1 protein cytidine deaminase APOBEC3G (A3G) can be secreted in sEVs by T cells or liver nonparenchymal cells (LNPCs), and these A3G containing sEVs inhibited HIV-1 replication (18,19). Recently, accumulating evidence has suggested that sEVs play an essential role in pathogenesis of lung diseases with inflammation involvement, including chronic obstructive pulmonary disease, asthma, acute lung injury, and coronavirus disease 2019 (COVID-19) disease (20-24). For example, in chronic inflammatory lung disease, neutrophil-derived sEVs bind neutrophil elastase to promote pulmonary extracellular matrix (ECM) destruction (20).
The characteristics and role of sEVs from patients with pneumonia, especially pediatric patients, however, remain ill-defined. Jung et al. measured 40 membrane proteins in plasma sEVs by protein microarrays and showed that the protein composition of sEVs enabled differentiation between patients with community-acquired pneumonia (CAP) and those with acute exacerbation of chronic obstructive pulmonary disease (AECOPD), suggesting that the protein composition of circulating sEVs can be used as a potential marker for the diagnosis of pneumonia (25). Unfortunately, the above studies targeted the detection of certain known pneumonia-related proteins in sEVs, whereas studies using a high-throughput and unbiased way to analyze the composition of sEVs are very rare. In the present study, we hypothesized that serum sEVs proteome would provide insights into the pathogenesis of pediatric pneumonia. We isolated serum sEVs from children with pneumonia and healthy children using size-exclusion chromatography, which has been shown to effectively remove contaminated proteins from collected sEV fractions (26), and then performed label-free mass spectrometry proteomic analysis to identify differentially expressed proteins (DEPs) and conducted functional enrichment analysis of them. We present the following article in accordance with the MDAR reporting checklist (available at https://tp.amegroups.com/article/view/10.21037/tp-22-134/rc).
Methods
Patient and sample collection
A total of 6 pediatric patients (5 females and 1 male) aged 3–10 years treated in Shanghai Children’s Medical Center were included in the study. Pneumonia was diagnosed based on fever, cough, breathlessness, leukocytosis, and radiological features. Etiologies were routinely detected as described previously (27). Since the clinical presentation of viral and bacterial infection overlap significantly, we speculate that common molecular features are present in the two types of pneumonia. Patients with both types of infection were included together in this study. A total of 3 children with M. pneumoniae infection and 3 children with viral infection were included. None of the patients had any previous history of pneumonia or any other complications. Healthy children were identified through laboratory tests and excluded if they presented with fever or respiratory symptoms. A total of 3 healthy children (1 female and 2 males) aged 4–5 years were included as controls. All subjects belong to the ethnic Han population in China. The clinical characteristics of patients are shown in Table 1. There were no differences in age, sex, or white blood cell (WBC) counts in children with pneumonia compared with the healthy controls, but the pneumonia group had lower lymphocytes and a higher neutrophils proportion.
Table 1
Characteristics | Healthy control | Pneumonia | P value* | ||||||||||
---|---|---|---|---|---|---|---|---|---|---|---|---|---|
HC1 | HC2 | HC3 | Median | P1 | P2 | P3 | P4 | P5 | P6 | Median | |||
Age (years) | 5 | 4 | 5 | 5 | 3 | 3 | 8 | 4 | 10 | 8 | 6 | 0.896 | |
Sex | Male | Male | Female | Male | Female | Female | Female | Female | Female | 0.226 | |||
WBC count (109/L) | 8.12 | 8.88 | 7.90 | 8.12 | – | 12.11 | 6.43 | 7.24 | 8.83 | 9.04 | 8.83 | 0.882 | |
Lymphocyte (%) | 37.7 | 45.4 | 55.1 | 45.4 | – | 32.9 | 14.0 | 28.4 | 34.3 | 29.0 | 29.0 | 0.025 | |
Neutrophil (%) | 54.6 | 42.5 | 26.8 | 42.5 | – | 57.8 | 83.8 | 63.8 | 56.2 | 60.4 | 60.4 | 0.025 | |
CRP (mg/L) | – | <1 | <1 | – | – | <1 | 8 | 4 | 4 | 41 | 6 | – | |
Ferritin (ng/mL) | – | – | – | – | 36 | 26.7 | 417.3 | 83.7 | 164.7 | 165.4 | 124.2 | – | |
LDH (U/L) | – | – | – | – | 547 | 638 | 893 | 748 | 562 | 829 | 693 | – | |
PCT (ng/mL) | – | – | – | – | 0.05 | <0.02 | 0.20 | 0.43 | 0.07 | 0.09 | 0.09 | – |
*, P value for sex variable was estimated by Fisher’s exact test; P values for other continuous variables were estimated by Mann-Whitney U test. CRP, C-reactive protein; HC, healthy control; LDH, lactate dehydrogenase; P, pneumonia; PCT, procalcitonin; WBC, white blood cells.
Serum samples were collected according to clinical study protocols approved by the ethics committee of Shanghai Children’s Medical Center (No. W2021010). In general, serum was collected on the second day after patient admission. In brief, about 2–3 mL of peripheral blood was collected into the BD Vacutainer® venous blood collection tube (BD Biosciences, Franklin Lakes, NJ, USA). The tube was then gently inverted until thoroughly mixed and left at room temperature for approximately 60 minutes to allow the blood to coagulate. The upper serum was pipetted into a 1.5 mL Eppendorf tube. The supernatant was transferred to a 1.5 mL cryovial, centrifuged at 13,000 rpm for 10 minutes at 4 ℃, and then stored at −80 ℃ until used. The study was conducted in accordance with the Declaration of Helsinki (as revised in 2013). Informed consent was obtained from the parents of all children.
Isolation and characterization of serum sEVs
Frozen serum samples were thawed in a 25 ℃ water bath and then centrifuged at 1,500 g for 10 minutes at 4 ℃. The supernatant was transferred to a new tube and centrifuged at 10,000 g for 30 minutes at 4 ℃. The new supernatant was used for sEV extraction using the qEV size exclusion columns (qEVoriginal/70 nm Legacy, IZON Science, Christchurch, New Zealand) as described previously (26). In brief, the buffer at the top of the column was removed with a pipette, the serum sample was added, and the bottom slide cap of the column was immediately removed. Nine fractions, each with a volume of 0.5 mL, were collected consecutively. A total of 1.5 mL from fractions 7–9 mainly containing sEVs were then concentrated to 250 µL using an Amicon® Ultra 10K centrifugal filter (Millipore, Burlington, MA, USA). Particle and protein concentrations were determined with the qNano Gold tunable resistive pulse sensing (TRPS) instrument (IZON Science, Christchurch, New Zealand) and bicinchoninic acid (BCA) assay, respectively. The ultrastructure of sEVs was analyzed by transmission electron microscopy (TEM) (Tecnai G2 Spirit BioTwin, FEI, Oregon, USA) as described previously (28).
Liquid chromatography-tandem mass spectrometry (LC-MS/MS)
Purified sEVs were lysed in buffer containing 7 M urea, 2% sodium dodecyl sulphate (SDS), and 1× protease inhibitor cocktail and digested using the standard filter aided proteome preparation protocol as described previously (28). Briefly, proteins were reduced with 20 mM dithiothreitol for 60 minutes at 57 ℃, alkylated with 90 mM iodoacetamide for 40 minutes, and digested with trypsin overnight at 37 ℃. The peptides after centrifugation and concentration were desalted by MonoSpin column (5010-21701, GL Sciences, Tokyo, Japan). In brief, the MonoSpin column was first treated with 100% acetonitrile, then equilibrated with 0.1% trifluoroacetic acid (TFA). The peptides resuspended in 0.1% TFA were applied to the column and centrifuged. Afterwards the column was washed with 0.1% TFA, and finally 50% acetonitrile was added to elute the peptides by centrifugation. The eluted peptides were centrifuged and dried under vacuum to remove acetonitrile
LC-MS/MS was performed with a QE-HF-X mass spectrometer coupled to an EASY-nLC 1200 (Thermo Scientific, Waltham, MA, USA). The vacuum-dried samples mentioned above were reconstituted with 0.1% formic acid (FA), transferred to an analytical column (C18, 2 µm, 100 Å, 50 µm × 15 cm; Thermo Scientific, Waltham, MA, USA), and separated using EASY-nLC 1200 at a flow rate of 300 nL/minute. The tandem mass spectrometry detection adopted the data dependent acquisition (DDA) mode. MS/MS spectra was acquired with a scan resolution of 60,000 full width at half maximum (FWHM) and 350–2,000 m/z of mass-to-charge ratio. Peptides were fragmented using higher-energy collisional dissociation at 28% normalized collision energy.
Proteomic data processing
Raw data were processed with MaxQuant (v 1.5.8.3) using the settings against a human protein database UniProt/SwissProt (last modified 2019-6-20). Reverse and potential contaminant proteins were removed. Carbamidomethyl (C) was set as a fixed modification, whereas oxidation (M) and acetyl (protein N-term) were variable modifications. The minimal peptide length was set to 7 amino acids, and 2 missed cleavages were set as maximum. The results were filtered at 0.01 false discovery rate (FDR) (peptide) and 0.05 FDR (protein). Label free quantification (LFQ) values were normalized by log2 transformation and used for protein quantification by “linear models for microarray data” (LIMMA) method (29).
Bioinformatics analysis
T-distributed stochastic neighbor embedding (t-SNE) was used for exploring proteomic data using the R package “Rtsne” (30). Pearson correlation coefficients analysis was performed using R package “corrplot”. Gene Ontology (GO), Reactome pathway enrichment, and protein-protein network analysis were conducted using R package “clusterProfiler” (31) and 5 functional enrichment analysis web tools including DAVID (http://david.ncifcrf.gov/) (32), Metascape (https://metascape.org/) (33), WebGestalt (http://webgestalt.org/) (34), g:Profiler (http://biit.cs.ut.ee/gprofiler) (35), and Reactome (https://reactome.org/) (36). Only processes and pathways with at least P<0.05 were considered for analysis.
Statistical analysis
Statistical significance of clinical variables was determined with Fisher’s exact test and Mann-Whitney U test. Differences in number and size of sEVs were determined with unpaired two-tailed Student’s t-test.
Results
Characterization of serum sEVs
A total of 3 healthy children and 6 children with pneumonia (age ≤10 years) were included in this study. Collected particles presented a typical cup-like shape through TEM analysis (Figure 1A). The mean size in diameter was 135 nm (range, 62–300 nm; median 122 nm) through TRPS analysis (Figure 1B). The diameter of most particles distributed between 50 and 150 nm (Figure 1B). The number and size of sEVs were comparable between the healthy and pneumonia groups (Figure 1C). Altogether, these results demonstrated that sEVs were successfully isolated from serum.
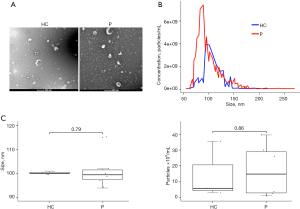
Global overview of the proteomic profiles in serum sEVs
We performed label-free proteomic analysis of serum sEVs by LC-MS/MS and obtained 5,024 peptides in total. By mapping these peptides to corresponding protein sequences, we found 534 proteins in total (table available at: https://cdn.amegroups.cn/static/application/6ea50a5bae62e5f087c95d231b359aa5/tp-22-134-1.pdf). The number of proteins were 266, 328, and 414 in the 3 healthy children, respectively, while the number of proteins were 419, 356, 395, 362, 436, and 383 in the 6 children with pneumonia, respectively (Figure S1A). The average spectral counts were calculated as 13.97 for all peptides (Figure S1B), indicating the proteomic results were highly reliable at the peptide level. A total of 528 proteins (98.9%) with ≥1 unique peptide were identified as high confidence proteins and subjected to subsequent analysis (table available at: https://cdn.amegroups.cn/static/application/6ea50a5bae62e5f087c95d231b359aa5/tp-22-134-1.pdf).
GO enrichment analysis of the cellular location of serum sEV proteins was performed through the DAVID web tool. The result showed that most proteins were located in the extracellular region, organelle, extracellular vesicles, and exosomes (Figure 2A), indicating the serum sEVs isolated had relatively good purity. Compared with more than 4,000 sEV proteins recorded in the Exocarta database (http://www.exocarta.org/), proteomic analysis of serum sEVs identified 316 of these reported proteins (Figure 2B). Further, the proteome of serum sEVs was compared with the 100 proteins most frequently identified in sEV proteomic studies and reported in the ExoCarta Top 100 proteins. A total of 38 of the top 100 proteins were identified in our analysis, and most had relatively high mean expression levels (Figure 2C), confirming the good quality of the serum sEV enrichment. In addition, sEV markers (CD81, CD9, GAPDH, GNB1, and LGALS3BP) were detected, and expression levels of these markers were comparable in the healthy group and the pneumonia group (Figure 2D), as expected.
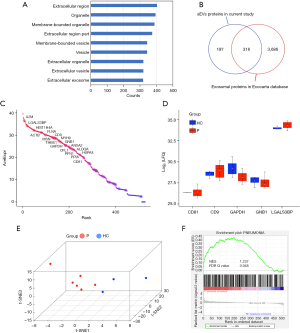
The non-linear dimensionality reduction algorithm t-SNE was then used for exploring proteomic data. T-SNE analysis of all samples was performed using 513 proteins with ≥1 unique peptide and valid LFQ values (LFQ >0) in at least 1 sample. The results showed that the pneumonia and healthy groups were clearly distinct (Figure 2E), suggesting a differential proteomic feature of sEVs between the pneumonia and healthy groups. To explain the difference between the pneumonia and healthy group, we analyzed whether serum sEVs derived from children with pneumonia was significantly enriched in those proteins related to pneumonia compared with that of healthy children using gene set enrichment analysis (GSEA). We first obtained a gene set related to pneumonia from the Comparative Toxicogenomics Database (CTD), ranked these genes according to the reference count, and selected the first 5,000 genes as the reference gene set for GSEA as described previously (37). The analysis showed that pneumonia-related genes in the reference gene set were positively correlated with the gene signature of sEVs in the pneumonia group, whereas they were negatively correlated with that of the healthy group (Figure 2F). These results indicated that proteomic data of serum sEVs provided mechanistic insights into the signature of children with pneumonia that could be leveraged to understand clinical outcomes.
Proteomic profiling of serum sEVs deciphers immune signatures of children with pneumonia
To eliminate the effect of contaminants on quantitative analysis of serum sEV proteome, we excluded the data of 14 apolipoproteins in our subsequent analysis. A total of 180 proteins with LFQ >0 in all samples in the healthy group and at least 4 samples in the pneumonia group were included (Table 2).
Table 2
Protein | LogFC | P value |
---|---|---|
HIST2H3A | 2.827 | 0.002 |
HIST1H4A | 2.354 | 0.005 |
IGLV6-57 | 2.200 | 0.045 |
SERPINA1 | 2.174 | 0.050 |
HIST1H2BL | 1.939 | 0.029 |
PROS1 | 1.853 | 0.142 |
C1R | 1.787 | 0.109 |
C4A | 1.695 | 0.085 |
C3 | 1.607 | 0.066 |
SERPINC1 | 1.580 | 0.078 |
C7 | 1.555 | 0.097 |
F2 | 1.539 | 0.054 |
CFH | 1.485 | 0.160 |
ITGB3 | 1.462 | 0.093 |
ITIH4 | 1.455 | 0.047 |
VTN | 1.305 | 0.075 |
IGHG3 | 1.301 | 0.210 |
IGHG1 | 1.241 | 0.075 |
SAA4 | 1.239 | 0.180 |
CLU | 1.237 | 0.077 |
IGHV5-51 | 1.209 | 0.220 |
HP | 1.155 | 0.155 |
C4BPB | 1.147 | 0.285 |
MMRN1 | 1.124 | 0.312 |
HPX | 1.112 | 0.196 |
IGLV3-10 | 1.090 | 0.167 |
IGLV3-25 | 1.085 | 0.169 |
ORM1 | 1.057 | 0.088 |
IGHG2 | 1.025 | 0.196 |
IGHV4-61 | 0.980 | 0.116 |
IGHV3-49 | 0.958 | 0.213 |
ORM2 | 0.920 | 0.180 |
C4BPA | 0.864 | 0.269 |
GSN | 0.847 | 0.199 |
IGKV1-27 | 0.842 | 0.272 |
F11 | 0.823 | 0.425 |
PF4 | 0.806 | 0.298 |
FGG | 0.769 | 0.599 |
AHSG | 0.769 | 0.293 |
FLNA | 0.758 | 0.321 |
IGHV1-2 | 0.748 | 0.309 |
IGHV3-74 | 0.088 | 0.889 |
IGHV3-15 | 0.081 | 0.907 |
IGLV10-54 | 0.066 | 0.942 |
IGLC7 | 0.063 | 0.935 |
IGKV4-1 | 0.062 | 0.943 |
LCN1P1 | 0.049 | 0.947 |
COL6A3 | 0.040 | 0.972 |
IGLV1-47 | 0.018 | 0.978 |
KRT6B | 0.011 | 0.991 |
IGKV3D-20 | 0.008 | 0.992 |
IGKV1D-16 | 0.003 | 0.996 |
IGHM | −0.013 | 0.985 |
IGLC3 | −0.015 | 0.981 |
IGKV2-24 | −0.022 | 0.976 |
FN1 | −0.027 | 0.963 |
KRTAP4-8 | −0.030 | 0.960 |
B2M | −0.057 | 0.940 |
HBA1 | −0.058 | 0.941 |
S100A8 | −0.074 | 0.927 |
A2M | −0.084 | 0.886 |
CD5L | −0.105 | 0.876 |
MYO1H | −0.116 | 0.864 |
IGJ | −0.118 | 0.872 |
SLC9A3R2 | −0.119 | 0.891 |
IGKV1-17 | −0.156 | 0.803 |
ECM1 | −0.158 | 0.794 |
IGLL5 | −0.163 | 0.815 |
MBL2 | −0.172 | 0.838 |
TNC | −0.187 | 0.762 |
IGKV1-16 | −0.197 | 0.766 |
GGT2 | −0.249 | 0.704 |
CAT | −0.266 | 0.732 |
SRGN | −0.282 | 0.812 |
IGLV3-21 | −0.289 | 0.667 |
FGA | −0.299 | 0.755 |
LYZ | −0.307 | 0.815 |
IGKV3D-11 | −0.319 | 0.653 |
PPBP | −0.320 | 0.719 |
COL6A1 | −0.321 | 0.640 |
HIST1H1C | −0.329 | 0.718 |
VWF | −0.362 | 0.622 |
TNXB | −1.305 | 0.237 |
FCN2 | −1.353 | 0.095 |
PANK4 | −1.425 | 0.207 |
ANXA2 | −1.547 | 0.039 |
PRDX2 | −1.562 | 0.059 |
COL1A2 | −1.604 | 0.063 |
IGLV4-60 | −1.611 | 0.089 |
COL1A1 | −1.642 | 0.025 |
IGHA1 | 0.696 | 0.238 |
LRP1 | 0.689 | 0.376 |
HBB | 0.647 | 0.429 |
TF | 0.642 | 0.351 |
IGHV1-46 | 0.639 | 0.338 |
IGLV3-27 | 0.627 | 0.501 |
MYH9 | 0.582 | 0.388 |
PIGR | 0.582 | 0.368 |
FBLN1 | 0.553 | 0.338 |
FCN3 | 0.545 | 0.399 |
FGB | 0.542 | 0.663 |
IGKC | 0.535 | 0.411 |
IGHV3-73 | 0.518 | 0.452 |
P0DOX2 | 0.449 | 0.474 |
HPR | 0.445 | 0.584 |
CD9 | 0.429 | 0.534 |
CFL1 | 0.397 | 0.616 |
C1S | 0.392 | 0.515 |
ACTB | 0.387 | 0.538 |
LPA | 0.368 | 0.647 |
IGLV1-40 | 0.337 | 0.623 |
IGHV3-72 | 0.333 | 0.599 |
PF4V1 | 0.329 | 0.646 |
FABP4 | 0.327 | 0.669 |
LGALS3BP | 0.322 | 0.574 |
PFN1 | 0.302 | 0.682 |
IGLV3-19 | 0.292 | 0.693 |
IGHV4-28 | 0.288 | 0.723 |
ALDOA | 0.288 | 0.716 |
IGHV3-43 | 0.287 | 0.703 |
S100A9 | 0.274 | 0.721 |
IGKV3-20 | 0.267 | 0.690 |
IGKV6D-21 | 0.213 | 0.721 |
PPIA | 0.178 | 0.779 |
IGHV3-9 | 0.163 | 0.796 |
ITIH1 | 0.134 | 0.887 |
IGHV3-7 | 0.133 | 0.830 |
P0DOX7 | 0.124 | 0.849 |
AMBP | 0.123 | 0.827 |
IGLV4-69 | 0.105 | 0.893 |
FABP5 | 0.092 | 0.935 |
IGKV A18 | −0.377 | 0.609 |
IGLV3-1 | −0.379 | 0.583 |
ERICH6B | −0.384 | 0.539 |
IGHV6-1 | −0.385 | 0.694 |
VCAN | −0.392 | 0.536 |
GNB1 | −0.413 | 0.591 |
HSPB1 | −0.419 | 0.624 |
IGKV3D-15 | −0.450 | 0.539 |
CSTA | −0.459 | 0.648 |
IGLV9-49 | −0.472 | 0.527 |
TYMP | −0.473 | 0.438 |
SSC5D | −0.486 | 0.491 |
HEG1 | −0.496 | 0.443 |
HRG | −0.512 | 0.404 |
FCGBP | −0.537 | 0.390 |
IGLV2-18 | −0.559 | 0.493 |
FMN2 | −0.575 | 0.418 |
COL6A2 | −0.603 | 0.331 |
PRG4 | −0.607 | 0.415 |
IGHV3-13 | −0.630 | 0.430 |
IGLV7-46 | −0.633 | 0.401 |
GLIPR2 | −0.660 | 0.345 |
MASP2 | −0.684 | 0.335 |
AZGP1 | −0.687 | 0.381 |
THBS4 | −0.690 | 0.318 |
IGLV1-51 | −0.700 | 0.341 |
MASP1 | −0.734 | 0.229 |
COL4A2 | −0.738 | 0.288 |
MSN | −0.745 | 0.424 |
UBA52 | −0.755 | 0.321 |
S100A7 | −0.777 | 0.469 |
CALM3 | −0.786 | 0.292 |
CALML5 | −0.882 | 0.400 |
IGLV5-45 | −1.054 | 0.245 |
DCD | −1.069 | 0.358 |
GAPDH | −1.081 | 0.138 |
COL4A1 | −1.113 | 0.188 |
IGLV8-61 | −1.121 | 0.239 |
CFP | −1.131 | 0.253 |
HMGB1 | −1.145 | 0.229 |
EP400 | −1.164 | 0.157 |
XP32 | −1.779 | 0.022 |
DSC1 | −1.911 | 0.022 |
COL2A1 | −2.206 | 0.021 |
DSG1 | −2.385 | 0.030 |
HAPLN1 | −2.452 | 0.004 |
CASP14 | −2.555 | 0.059 |
ACAN | −2.651 | 0.001 |
DSP | −3.957 | 0.000 |
FC = expression (pneumonia)/expression (healthy control). LogFC: log2 (FC). FC, fold change; sEV, small extracellular vesicle.
We first determined the Pearson correlation coefficients between the LFQ values of the 180 proteins and grouped proteins by similarity. Unsupervised hierarchical clustering of these correlation coefficients revealed 2 main groups of proteins, which were anticorrelated (Figure 3A). In group 1, there were more collagen proteins than group 2, whereas more complement proteins were detected in group 2. Histone proteins (HIST1H1C, HIST1H2BL, HIST1H4A, and HIST2H3A), desmosome components (DSD, DSC1, DSG1, DSP) and damage associated molecular pattern (DAMP) proteins HMGB1 and S100A7 were identified in group 1. In group 2, we detected acute phase proteins ITIH1, ITIH4, ORM1, ORM2, SAA4, SERPINA1, and SERPINC1. These indicated that serum sEV proteome was enriched in many kinds of immune molecules.
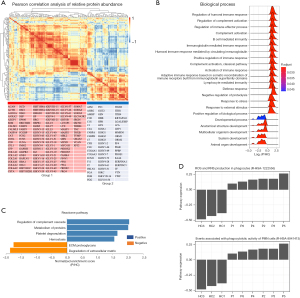
We then ranked these quantified proteins according to the fold change in the pneumonia group versus the healthy group (Table 2). GSEA was used to identify significantly enriched or depleted groups of proteins with respect to pneumonia in serum sEVs. First, GSEA analysis of GO biological processes showed that the pneumonia group was positively enriched in many processes associated with immunity such as regulation of humoral immune response, complement activation and immune effector process, lymphocyte mediated immunity, defense response, and response to stress, whereas it was negatively enriched in organ, system, and anatomical structure development (Figure 3B, Table S1).
To gain further insight, GSEA analysis of Reactome pathways was performed using the WebGestalt web tool. Reactome enrichment analysis showed that the pneumonia group was positively enriched in regulation of complement cascade, metabolism of proteins, platelet degranulation, and hemostasis, but it was negatively enriched in ECM proteoglycans and degradation of ECM (Figure 3C). Further, a single sample GSEA (ssGSEA) method was used to analyze proteomic data of each single sample using a Reactome web analysis tool. ssGSEA analysis of Reactome pathways showed that the production of reactive oxygen species (ROS) and reactive nitrogen species (RNS) in phagocytes as well as phagocytic activity of polymorphonuclear leukocytes (PMN) cells were positively enriched in each sample of the pneumonia group but were negatively enriched in each sample of the healthy group (Figure 3D). These results indicated that the proteomic data of serum sEVs reflected immune-related events of children with pneumonia, providing further insights into immune activation that could be used to guide precision treatment.
Identification of protein networks associated with immune response and tissue structure
We first determined which proteins were mapped to the GO terms positively and negatively enriched in the pneumonia group mentioned above and then carried out protein-protein interaction enrichment analysis of these proteins using the Metascape web tool. Meanwhile, the Molecular Complex Detection (MCODE) algorithm was applied to identify densely connected network components. Forty-five proteins contributed to the GO terms positively enriched in the pneumonia group (Figure 4A), most of which were associated with complement, platelet, and acute inflammatory response (Figure 4A), whereas 29 proteins mapped to the GO terms negatively enriched in the pneumonia group (Figure 4B) were relevant to tissue development and extracellular structure remodeling such as ECM proteoglycans, vessel development, epithelial cell differentiation, and formation of the cornified envelope (Figure 4B).
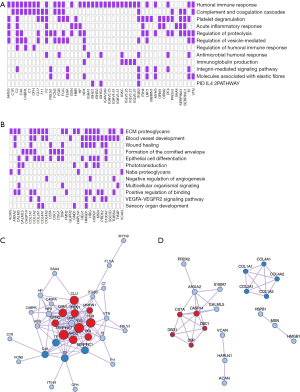
Protein network analysis showed that 33 of positive enriched proteins formed physical interactions, and 2 MCODE networks were identified (Figure 4C). One of the MCODE networks contained 10 proteins (AHSG, CLU, FGB, FGG, MMRN1, ORM1, ORM2, PF4, PROS1, and SERPINA1), and the functional enrichment term of the MCODE components was primarily associated with platelet degranulation and activation (Figure 4C). The second MCODE network was composed of C3, C4A, SERPINC1, and transferrin, which are linked to protein phosphorylation, regulation of insulin-like growth factor transport and uptake, and complement and coagulation cascades (Figure 4C). However, 20 of negative enriched proteins formed 4 independent interactions, and 2 MCODE networks were identified (Figure 4D). The first MCODE network consisted of CASP14, CSTA, DSC1, DSG1, and DSP, which are involved in cornified envelope formation and keratinization, whereas the second MCODE network was made up of 5 collagen proteins, COL1A1, COL1A2, COL2A1, COL4A1, and COL4A2 (Figure 4D). In sum, these results illustrated that the pneumonia group was positively enriched in platelet- and complement-related protein networks but negatively enriched in keratinization- and collagen-related protein networks.
Identification of DEPs in serum sEVs associated with immune response between children with pneumonia and healthy children
Among 180 quantified proteins, a total of 15 DEPs (logFC >1 or <−1, P<0.05) were identified with 6 upregulated proteins, including SERPINA1, ITIH4, IGLV6-57, HIST2H3A, HIST1H4, and HIST1H2BL, and 9 downregulated proteins, including COL1A1, ANXA2, COL2A1, ACAN, DSP, XP32, DSC1, HAPLN1, and DSG1 in the pneumonia group compared with the healthy group (Figure 5A, Table 2). These DEPs clearly distinguished the pneumonia from the healthy group (Figure 5B). GO enrichment analysis of DEPs was performed through the g:Profiler web tool (table available at: https://cdn.amegroups.cn/static/application/f37d5981d282d8dbbd472bf6b6a418a7/tp-22-134-2.pdf). Of upregulated proteins, 3 histone proteins, HIST2H3A, HIST1H4, and HIST1H2BL, are associated with nucleosome assembly. SERPINA1 and ITIH4 are acute phase proteins (Figure 5C). However, many downregulated proteins contributed to the following GO terms: collagen fibril and extracellular structure organization, cell adhesion, tissue development, and epidermal cell differentiation (Figure 5C). Of note, upregulated proteins SERPINA1, ITIH4, and IGLV6-57 and downregulated proteins COL1A1, ANXA2, COL2A1, DSP, DSC1, and DSG1 were linked to immune-related processes such as neutrophil activation, leukocyte-mediated immunity, and immune response in general (Figure 5C). We analyzed Pearson correlation coefficients between LFQ values of DEPs and clinical values of C-reactive protein (CRP), procalcitonin (PCT), and neutrophil count in the pneumonia group and found COL2A1 was negatively correlated with neutrophil count (R=−0.89, P=0.045; Figure 5D), indicating that a decrease in COL2A1 expression may lead to activation of neutrophils in children with pneumonia.
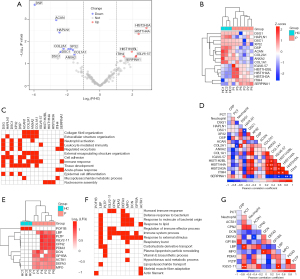
We then identified unique proteins which were exclusively detected in at least 4 samples in the pneumonia group or all samples in the healthy group with LFQ >0. A total of 10 unique proteins (MPO, DEFA3, ACTA1, GP1BA, DCN, PDIA3, CPN2, IGLV2-11, PLTP, and LBP) were identified in the pneumonia group, and only 1 unique protein (POF1B) was found in the healthy group (Figure 5E). We focused on analysis of the unique proteins in the pneumonia group and used the g:Profiler web tool to perform GO biological processes enrichment analysis (Table S2). The mapped biological processes containing the largest number of these unique proteins were immune system processes, which included 7 enriched proteins (MPO, DEFA3, GP1BA, PDIA3, CPN2, IGLV2-11, and LBP), and processes related to response to external stimulus, which included 7 enriched proteins (MPO, DEFA3, ACTA1, GP1BA, DCN, IGLV2-11, and LBP). Proteins with the greatest number of mapped GO terms were MPO, LBP and DEFA3, which contributed to immune-related GO terms such as humoral immune response, defense response to bacterium or molecules of bacterial origin, response to lipids, regulation of immune effector processes, and respiratory burst (Figure 5F). We also analyzed Pearson correlation coefficients between LFQ values of these unique sEV proteins and clinical values of CRP, PCT, and neutrophil count. As a result, we identified 2 proteins, LBP and IGLV2-11, positively correlated with PCT (P<0.05; Figure 5G). In particular, LBP and PCT have been reported as potential biomarkers for the diagnosis of virus and bacterial infections. This suggested that serum sEV proteins, once validated in a larger cohort, could be considered a potential source of biomarkers for diagnosing pediatric pneumonia.
Collectively, these results demonstrated that proteome of serum sEVs could shed light on human host responses to pathogen exposure and facilitate understanding of the fundamental biology of pediatric pneumonia.
Discussion
sEVs have been reported to be crucial messengers in the regulation of infection processes and are likely involved in the modulation of multiple signaling processes associated with immune response (38). In particular, sEVs play a key role in the development of lung inflammation in pneumonia (39). Moreover, sEVs are used as a superior biomarker for disease diagnosis which has attracted the most attention in clinical application. sEVs show significant superiority over other types of biomarkers (12,40,41). First, sEVs are present in almost all bodily fluids, making them easy to collect. Second, the cargoes in sEVs are encapsulated by lipid bilayers, which protects them from degradation by external enzymes and provides greater stability. Third, sEVs are directly synthesized and secreted by living cells, contain biological information from parent cells and can better reflect the state of lesion tissue. Fourth, sEVs show superiority to other types of biomarkers in diagnostic accuracy. For example, the diagnostic accuracy of PD-L1 located in plasma sEVs for pancreatic cancer is higher than that of total plasma PD-L1 and microvesicular PD-L1 (10).
We performed proteomic analysis of serum sEVs in children with pneumonia caused by M. pneumoniae and viral infections. Our results showed that sEV proteome in children with pneumonia was positively enriched in many immune processes and pathways, including humoral immune response, complement cascades, and platelet degranulation, suggesting that the proteome of sEVs could reveal immune signatures of children with pneumonia. Further, protein-protein network analysis showed that the most significantly connected network in the pneumonia group was constructed by platelet degranulation- and activation-related proteins (AHSG, CLU, FGB, FGG, MMRN1, ORM1, ORM2, PF4, PROS1, and SERPINA1), suggesting that serum sEVs may be at least partially derived from platelets and serum sEV proteome may have reflected the activity of platelets in children with pneumonia.
Neutrophils are the most important cells recruited to the lungs to participate in innate immunity in the process of lung infection and are closely related to the progression of pneumonia (42). Neutrophil activity proteins MPO and DEFA3 were significantly overrepresented in children with pneumonia, suggesting that neutrophils were activated in these pediatric pneumonia patients. We speculated that expression levels of neutrophil activity-related proteins loading into sEVs may have reflected the immune activity and progress of pneumonia. MPO is secreted by neutrophils to produce ROS and participate in the inflammatory response, and increased expression levels of MPO represent PMN cell activation and lung inflammation (20). Consistently, pathway analysis showed that the production of ROS was positively enriched in each sample of the pneumonia group, indicating that the proteome of sEVs may have reflected the phagocytic activity of PMN cells. In addition, neutrophilic inflammation can disrupt formation of the elastic fiber network, resulting in impairment of lung structure and function (43). However, several proteins with elastic fiber were upregulated in the pneumonia group, including VTN, TF, MYH9, LRP1, ITGB3, HPX, FLNA, and FBLN1, implying that tissue repair mechanism was activated in infected lung to protect lung structure.
PDIA3, a member of protein disulfide isomerases (PDI) family, was significantly overrepresented in children with pneumonia. Previous studies have shown that PDIA3 interacted with influenza A virus (IAV) hemagglutinin (HA) and was specifically upregulated in infected mouse or human lung epithelial cells (44). We speculated that PDIA3 may have been required for effective virus infection pathogenesis in children with pneumonia, and pharmacological inhibition of PDIs may represent a new promising therapeutic strategy for pediatric pneumonia.
One of the key observations was the enrichment of histone proteins in children with pneumonia. Histones are involved in numerous biological processes, largely through repressing transcription. Interestingly, previous studies have found that certain histones were highly expressed in activated neutrophils (45). Our proteomic data showed that expression levels of HIST2H3A, HIST1H4, and HIST1H2BL were increased significantly in the pneumonia group, indicating that histone-mediated regulation of gene expression may have played a key role in the pathogenesis of pneumonia.
In addition to upregulated proteins in children with pneumonia, many downregulated proteins were also involved in the process of pathogen infection. These downregulated proteins were related to lung epidermal cells and blood vessel adhesion and involved in the repair of damaged lung tissue. Among them, collagen proteins participate in ECM organization and play an important role in lung inflammation. It has been shown that pathogens utilized host ECM proteins such as collagen for adhesion and invasion of the host cells (46). We speculated that changes of these collagen-related proteins in serum sEVs, including COL1A1, COL2A1, and ACAN, indicated that ECM remodeling occurred under infections in children. Among the downregulated proteins, we also detected several desmosome proteins (DSC1, DSG1, and DSP). Desmosome is required for integrity of airway epidermal cells and participates in immunomodulatory actions (47,48). Notably, disruption of DSG1 has also been associated with the skin diseases palmoplantar keratoderma and erythroderma, and loss of DSG1 function induces immune activation in these disease states (49). However, the function of DSG1 in the pathogenesis of pneumonia remains unclear and needs further study.
GO analysis showed that blood vessel development was negatively enriched in children with pneumonia, consistent with the fact that pulmonary blood vessels play a critical role in the pathogenesis of lung injuries (50). Many downregulated proteins involved in blood vessel development also participate in ECM proteoglycans, including THBS4, SSC5D, MSN, HSBP1, HRG, HMGB1, COL4A2, COL4A1, COL1A1, COL1A2, and ANXA2, suggesting that ECM remodeling may lead to vascular destruction and increased permeability, further disrupting the epithelial-endothelial barrier. Therefore, we speculated that a reduction in the expression of these proteins could be a risk factor in children for developing pneumonia after infection. Collectively, these downregulated proteins could be regarded as molecule signatures to predict the remodeling of the extracellular microenvironment, tissue structure, and immune activity of the lungs in children with pneumonia.
An improved understanding of the pathogenesis of pediatric pneumonia and immunologic state could help identify patients at significant risk for complications, guiding precision therapeutic approaches. Proteomic data of serum sEVs provides mechanistic insights into the signatures of children with pneumonia that could be leveraged to understand the pediatric patient’s clinical outcomes. The value of our study is that this data may also help us understand and more effectively predict, prevent, and treat infection in children.
A limitation of this study was that as the sample size was small, there was insufficient statistical power to make strong statements on the associations between protein expression and pneumonia. The expression of potential DEPs needs to be verified in more samples. In addition, our study did not distinguish between children with pneumonia caused by different pathogens. The mechanisms of pneumonia caused by different pathogens should vary, and in-depth research is needed in the future.
Conclusions
We performed label-free proteomic analysis of serum sEVs derived from children with pneumonia and found that proteins associated with regulation of neutrophil and complement activity were upregulated and proteins involved in ECM and cell adhesion were downregulated in children with pneumonia. Collectively, the results of this study facilitated understanding of the pathogenesis of pediatric pneumonia.
Acknowledgments
We are grateful to all the children who took part in the study.
Funding: This work was supported by the Shanghai Municipal Health Commission (No. 202140111).
Footnote
Reporting Checklist: The authors have completed the MDAR reporting checklist. Available at https://tp.amegroups.com/article/view/10.21037/tp-22-134/rc
Data Sharing Statement: Available at https://tp.amegroups.com/article/view/10.21037/tp-22-134/dss
Conflicts of Interest: All authors have completed the ICMJE uniform disclosure form (available at https://tp.amegroups.com/article/view/10.21037/tp-22-134/coif). DJ, SW, KS, and QZ are employees of Wayen Biotechnologies (Shanghai), Inc. The other authors have no conflicts of interest to declare.
Ethical Statement: The authors are accountable for all aspects of the work in ensuring that questions related to the accuracy or integrity of any part of the work are appropriately investigated and resolved. The study was conducted in accordance with the Declaration of Helsinki (as revised in 2013). The study was approved by the ethics committee of Shanghai Children’s Medical Center (No. W2021010). Informed consent was obtained from the parents of all children.
Open Access Statement: This is an Open Access article distributed in accordance with the Creative Commons Attribution-NonCommercial-NoDerivs 4.0 International License (CC BY-NC-ND 4.0), which permits the non-commercial replication and distribution of the article with the strict proviso that no changes or edits are made and the original work is properly cited (including links to both the formal publication through the relevant DOI and the license). See: https://creativecommons.org/licenses/by-nc-nd/4.0/.
References
- File TM. Community-acquired pneumonia. Lancet 2003;362:1991-2001. [Crossref] [PubMed]
- Klompas M. Does this patient have ventilator-associated pneumonia? JAMA 2007;297:1583-93. [Crossref] [PubMed]
- Sellers SA, Hagan RS, Hayden FG, et al. The hidden burden of influenza: A review of the extra-pulmonary complications of influenza infection. Influenza Other Respir Viruses 2017;11:372-93. [Crossref] [PubMed]
- Zaas AK, Chen M, Varkey J, et al. Gene expression signatures diagnose influenza and other symptomatic respiratory viral infections in humans. Cell Host Microbe 2009;6:207-17. [Crossref] [PubMed]
- Andres-Terre M, McGuire HM, Pouliot Y, et al. Integrated, Multi-cohort Analysis Identifies Conserved Transcriptional Signatures across Multiple Respiratory Viruses. Immunity 2015;43:1199-211. [Crossref] [PubMed]
- Kowal J, Arras G, Colombo M, et al. Proteomic comparison defines novel markers to characterize heterogeneous populations of extracellular vesicle subtypes. Proc Natl Acad Sci U S A 2016;113:E968-77. [Crossref] [PubMed]
- Kugeratski FG, Hodge K, Lilla S, et al. Quantitative proteomics identifies the core proteome of exosomes with syntenin-1 as the highest abundant protein and a putative universal biomarker. Nat Cell Biol 2021;23:631-41. [Crossref] [PubMed]
- Melo SA, Luecke LB, Kahlert C, et al. Glypican-1 identifies cancer exosomes and detects early pancreatic cancer. Nature 2015;523:177-82. [Crossref] [PubMed]
- Hoshino A, Costa-Silva B, Shen TL, et al. Tumour exosome integrins determine organotropic metastasis. Nature 2015;527:329-35. [Crossref] [PubMed]
- Chen G, Huang AC, Zhang W, et al. Exosomal PD-L1 contributes to immunosuppression and is associated with anti-PD-1 response. Nature 2018;560:382-6. [Crossref] [PubMed]
- El-Shennawy L, Hoffmann AD, Dashzeveg NK, et al. Circulating ACE2-expressing extracellular vesicles block broad strains of SARS-CoV-2. Nat Commun 2022;13:405. [Crossref] [PubMed]
- Zhou B, Xu K, Zheng X, et al. Application of exosomes as liquid biopsy in clinical diagnosis. Signal Transduct Target Ther 2020;5:144. [Crossref] [PubMed]
- Kalluri R, LeBleu VS. The biology, function, and biomedical applications of exosomes. Science 2020;367:eaau6977. [Crossref] [PubMed]
- Robbins PD, Dorronsoro A, Booker CN. Regulation of chronic inflammatory and immune processes by extracellular vesicles. J Clin Invest 2016;126:1173-80. [Crossref] [PubMed]
- Muntasell A, Berger AC, Roche PA. T cell-induced secretion of MHC class II-peptide complexes on B cell exosomes. EMBO J 2007;26:4263-72. [Crossref] [PubMed]
- Montecalvo A, Shufesky WJ, Stolz DB, et al. Exosomes as a short-range mechanism to spread alloantigen between dendritic cells during T cell allorecognition. J Immunol 2008;180:3081-90. [Crossref] [PubMed]
- Nolte-'t Hoen EN, Buschow SI, Anderton SM, et al. Activated T cells recruit exosomes secreted by dendritic cells via LFA-1. Blood 2009;113:1977-81. [Crossref] [PubMed]
- Khatua AK, Taylor HE, Hildreth JE, et al. Exosomes packaging APOBEC3G confer human immunodeficiency virus resistance to recipient cells. J Virol 2009;83:512-21. [Crossref] [PubMed]
- Li J, Liu K, Liu Y, et al. Exosomes mediate the cell-to-cell transmission of IFN-α-induced antiviral activity. Nat Immunol 2013;14:793-803. [Crossref] [PubMed]
- Genschmer KR, Russell DW, Lal C, et al. Activated PMN Exosomes: Pathogenic Entities Causing Matrix Destruction and Disease in the Lung. Cell 2019;176:113-126.e15. [Crossref] [PubMed]
- Haj-Salem I, Plante S, Gounni AS, et al. Fibroblast-derived exosomes promote epithelial cell proliferation through TGF-β2 signalling pathway in severe asthma. Allergy 2018;73:178-86. [Crossref] [PubMed]
- Moon HG, Cao Y, Yang J, et al. Lung epithelial cell-derived extracellular vesicles activate macrophage-mediated inflammatory responses via ROCK1 pathway. Cell Death Dis 2015;6:e2016. [Crossref] [PubMed]
- Krishnamachary B, Cook C, Kumar A, et al. Extracellular vesicle-mediated endothelial apoptosis and EV-associated proteins correlate with COVID-19 disease severity. J Extracell Vesicles 2021;10:e12117. [Crossref] [PubMed]
- Raab-Traub N, Dittmer DP. Viral effects on the content and function of extracellular vesicles. Nat Rev Microbiol 2017;15:559-72. [Crossref] [PubMed]
- Jung AL, Møller Jørgensen M, Bæk R, et al. Surface Proteome of Plasma Extracellular Vesicles as Biomarkers for Pneumonia and Acute Exacerbation of Chronic Obstructive Pulmonary Disease. J Infect Dis 2020;221:325-35. [PubMed]
- Lobb RJ, Becker M, Wen SW, et al. Optimized exosome isolation protocol for cell culture supernatant and human plasma. J Extracell Vesicles 2015;4:27031. [Crossref] [PubMed]
- Yang L, Yang Z, Cheng L, et al. Lectin Microarray Combined with Mass Spectrometry Identifies Haptoglobin-Related Protein (HPR) as a Potential Serologic Biomarker for Separating Nonbacterial Pneumonia from Bacterial Pneumonia in Childhood. Proteomics Clin Appl 2018;12:e1800030. [Crossref] [PubMed]
- Bai X, Li J, Li L, et al. Extracellular Vesicles From Adipose Tissue-Derived Stem Cells Affect Notch-miR148a-3p Axis to Regulate Polarization of Macrophages and Alleviate Sepsis in Mice. Front Immunol 2020;11:1391. [Crossref] [PubMed]
- Jiang Y, Sun A, Zhao Y, et al. Proteomics identifies new therapeutic targets of early-stage hepatocellular carcinoma. Nature 2019;567:257-61. [Crossref] [PubMed]
- van der Maaten L, Hinton G. Visualizing Data using t-SNE. J Mach Learn Res 2008;9:2579-605.
- Wu T, Hu E, Xu S, et al. clusterProfiler 4.0: A universal enrichment tool for interpreting omics data. Innovation (Camb) 2021;2:100141. [Crossref] [PubMed]
- Huang da W. Sherman BT, Lempicki RA. Systematic and integrative analysis of large gene lists using DAVID bioinformatics resources. Nat Protoc 2009;4:44-57. [Crossref] [PubMed]
- Zhou Y, Zhou B, Pache L, et al. Metascape provides a biologist-oriented resource for the analysis of systems-level datasets. Nat Commun 2019;10:1523. [Crossref] [PubMed]
- Liao Y, Wang J, Jaehnig EJ, et al. WebGestalt 2019: gene set analysis toolkit with revamped UIs and APIs. Nucleic Acids Res 2019;47:W199-205. [Crossref] [PubMed]
- Raudvere U, Kolberg L, Kuzmin I, et al. g:Profiler: a web server for functional enrichment analysis and conversions of gene lists (2019 update). Nucleic Acids Res 2019;47:W191-8. [Crossref] [PubMed]
- Jassal B, Matthews L, Viteri G, et al. The reactome pathway knowledgebase. Nucleic Acids Res 2020;48:D498-503. [PubMed]
- Walter JM, Ren Z, Yacoub T, et al. Multidimensional Assessment of the Host Response in Mechanically Ventilated Patients with Suspected Pneumonia. Am J Respir Crit Care Med 2019;199:1225-37. [Crossref] [PubMed]
- Schorey JS, Cheng Y, Singh PP, et al. Exosomes and other extracellular vesicles in host-pathogen interactions. EMBO Rep 2015;16:24-43. [Crossref] [PubMed]
- Murphy CA, O'Reilly DP, Neary E, et al. A review of the role of extracellular vesicles in neonatal physiology and pathology. Pediatr Res 2021;90:289-99. [Crossref] [PubMed]
- Yu W, Hurley J, Roberts D, et al. Exosome-based liquid biopsies in cancer: opportunities and challenges. Ann Oncol 2021;32:466-77. [Crossref] [PubMed]
- LeBleu VS, Kalluri R. Exosomes as a Multicomponent Biomarker Platform in Cancer. Trends Cancer 2020;6:767-74. [Crossref] [PubMed]
- Grudzinska FS, Brodlie M, Scholefield BR, et al. Neutrophils in community-acquired pneumonia: parallels in dysfunction at the extremes of age. Thorax 2020;75:164-71. [Crossref] [PubMed]
- Benjamin JT, Plosa EJ, Sucre JM, et al. Neutrophilic inflammation during lung development disrupts elastin assembly and predisposes adult mice to COPD. J Clin Invest 2021;131:139481. [Crossref] [PubMed]
- Chamberlain N, Korwin-Mihavics BR, Nakada EM, et al. Lung epithelial protein disulfide isomerase A3 (PDIA3) plays an important role in influenza infection, inflammation, and airway mechanics. Redox Biol 2019;22:101129. [Crossref] [PubMed]
- Shankar R, Leimanis ML, Newbury PA, et al. Gene expression signatures identify paediatric patients with multiple organ dysfunction who require advanced life support in the intensive care unit. EBioMedicine 2020;62:103122. [Crossref] [PubMed]
- Singh B, Fleury C, Jalalvand F, et al. Human pathogens utilize host extracellular matrix proteins laminin and collagen for adhesion and invasion of the host. FEMS Microbiol Rev 2012;36:1122-80. [Crossref] [PubMed]
- van Moorsel CHM. Desmoplakin: An Important Player in Aging Lung Disease. Am J Respir Crit Care Med 2020;202:1201-2. [Crossref] [PubMed]
- Heijink IH, Kuchibhotla VNS, Roffel MP, et al. Epithelial cell dysfunction, a major driver of asthma development. Allergy 2020;75:1902-17. [Crossref] [PubMed]
- Samuelov L, Sarig O, Harmon RM, et al. Desmoglein 1 deficiency results in severe dermatitis, multiple allergies and metabolic wasting. Nat Genet 2013;45:1244-8. [Crossref] [PubMed]
- Armstrong SM, Darwish I, Lee WL. Endothelial activation and dysfunction in the pathogenesis of influenza A virus infection. Virulence 2013;4:537-42. [Crossref] [PubMed]