Bioinformatics analysis of lncRNAs in the occurrence and development of osteosarcoma
Introduction
Osteosarcoma (OS) is a primary malignant bone tumor that most commonly affects children, adolescents, and young adults (1-3). OS occurs in the epiphysis of long bones, most commonly in the distal femur (43%), proximal tibia (23%), and humerus (10%) (4,5). Patients with advanced metastatic OS have a poor prognosis. OS patients often develop a resistance to standard therapies; thus, treatment regimens need to be improved and novel therapeutic targets need to identified (6). At present, the underlying molecular mechanism of OS is still unclear, which hinders the development of prognosis and treatment strategies. It is urgent to identify new prognostic and diagnostic biomarkers in OS.
Numerous studies have been conducted upon this issue. With advancement of sequencing technologies, several kinds of non-coding RNAs (ncRNAs) have been discovered, such as the microRNAs, lncRNAs and circleRNAs (7-9). Recently microRNAs (i.e., miR-9, miR-21, miR-29 and miR-195) have been presented play a potential biological role in osteosarcoma and can be used as diagnostic and prognostic markers and therapeutic targets (7,8). LncRNAs play an important role in the occurrence and development of tumors, which show significant potential in osteosarcoma prognosis (10). However, roles of lncRNAs in the progress, prognosis and metastasis of osteosarcoma OS has not been widely explored (11). There is experimental evidence that many lncRNAs are involved in the pathogenesis and development of OS; For example, Ankyrin Repeat and SOCS Box Containing 16 Antisense RNA 1 (ASB16-AS1) has been identified as a novel oncogenic lncRNA in OS cells. ASB16-AS1 increases the level of hepatoma-derived growth factor expression by sponging Mir-760, which play a promoting role in OS (12). Potassium Voltage-Gated Channel Subfamily Q Member 1 Opposite Strand/Antisense Transcript 1 (KCNQ1OT1) expression has been shown to be increased in the tissues of patients with OS and is associated with OS progression and decreased overall survival (13). KCNQ1OT1 may be a drug-resistant lncRNA and a promising target for avoiding chemical resistance (13). LINC01116 targets miR-520a-3p and affects interleukin-6 receptor (IL6R), promoting the proliferation and migration of OS cells through the Janus-activated kinase/signal transducer and activator of transcription (JAK/STAT) signaling pathway (14). The high expression of lncRNA prostate cancer-associated transcript 6 (PCAT6) has been shown to be positively correlated with an advanced stage and the metastatic state of OS, and a survival analysis showed that the upregulation of PCAT6 is associated with a poor prognosis (15). The down syndrome cell adhesion molecule antisense 1 (DSCAM-AS1) silencing significantly inhibited the viability and invasion characteristics of OS cells, while DSCAM-AS1 upregulation had the opposite effect (16). Long Intergenic Non-Protein Coding RNA 1614 (LINC01614) can serve as a competing endogenous RNA and promote the proliferation and invasion of OS cells through the miR-520a-3p/sorting nexin 3 (SNX3) axis, thus serving as a novel prognostic marker for clinical OS (17). LncRNA small nucleolar RNA host gene 4 (SNHG4) is highly expressed in OS tissues and cell lines. In addition, SNHG4 expression has been shown to be associated with distant metastasis, tumor-node-metastasis staging, and survival in patients with OS (18). Melanotransferrin Antisense RNA 1 (MELTF-AS1) acts as a metastasis-promoting gene in OS via the upregulation of Matrix metalloproteinase 14 (MMP14), and may be a potential therapeutic and diagnostic target for OS (19). LncRNA LEF1-AS1 binds to heterogeneous nuclear ribonucleoprotein L, and promotes the proliferation, migration, and invasion of OS by enhancing the mRNA stability of LEF1 (20). LncRNA antisense noncoding RNA from the RNA binding motive 5 (RBM5-AS1) promotes OS cell proliferation, migration, and invasion in vitro and tumor growth in vivo. LncRNA RBM5-AS1 targets RBM5 in OS cells (21). LncRNA PCAT-1 promotes the progression of OS, and the mir-508-3p/ Zinc finger E-box binding homeobox 1 (ZEB1) axis has been shown to be associated with the functional role of PCAT-1 in OS, which suggests that PCAT-1/Mir-508-3p/ZEB1 may be a therapeutic target for OS patients (22). LncRNA titinantisense RNA1 (TTN-AS1) is highly expressed in OS and is associated with a poor prognosis (23). It regulates OS cell apoptosis and drug resistance through the Mir-134-5p/MBTD1 axis (23). A Kaplan-Meier analysis showed that the overexpression of mir-100-let-7a-2-mir-125b-1 cluster host gene (MIR100HG), HOXD cluster antisense RNA 1 (HOXD-AS1), Ewing sarcoma-associated transcript 1 (EWSAT1), LIM and Cysteine Rich Domains 1 Antisense RNA 1 (LMCD1-AS1), and many other lncRNAs predicts a poor prognosis in patients with OS (24). Thus, lncRNAs are very important in OS. Th early detection and screening of lncRNA expression and possible interventions may be of great help in reducing the mortality and improving the prognosis of OS patients.
In this study, a bioinformatics analysis demonstrated the role of lncRNAs in the occurrence and development of OS, and their clinical prognostic value was explored via univariate and multivariate regression analyses and receiver operating characteristic (ROC) curve analyses. More importantly, we conducted a Gene Ontology (GO) analysis, Kyoto Encyclopedia of Genes and Genomes (KEGG) analysis, Gene Set Enrichment Analysis (GSEA), and immune infiltration analysis to determine the biological functions of differentially expressed genes (DEGs) in high- and low-risk patients. Our results suggest that lncRNA is a possible future therapeutic target for OS. We present the following article in accordance with the TRIPOD reporting checklist (available at https://tp.amegroups.com/article/view/10.21037/tp-22-253/rc).
Methods
Data collection
Clinical data and RNA-sequencing data were downloaded from The Cancer Genome Atlas (TCGA) data set for OS (labeled x TARGET-OS; sample size n=86, one sample is excluded). Clinical data and RNA-sequencing data for cutaneous melanoma (labeled TCGA-SKCM; n=457) and IMvigor210 (n=348, http://research-pub.gene.com/IMvigor210CoreBiologies/) were also downloaded. A lncRNA data set of immune cells were defined as IM-lncRNAs. The chip data related to the following immune cells were collected: GSE13906, GSE23371, GSE25320, GSE27291, GSE27838, GSE28490, GSE28698, GSE28726, GSE37750, GSE39889, GSE42058, GSE49910, GSE51540, GSE59237, GSE6863, and GSE8059. The chip platform used was the Affymetrix HG-U133_Plus 2.0 platform. The robust multiarray analysis (RMA) method was used to standardize the data. The annotation information of lncRNA was extracted by NetAffx annotation files (HG-U133 Plus 2.0 Annotations, CSV format, release 36, July 12, 2016). As the data includes batch information, the comBat package was used to remove the batch effect. The pre-processed data were used for the subsequent analyses. High expression lncRNA in each immune cell type was screened, and the expression of lncRNA in each immune cell was determined to be normally distributed by a normal distribution test. Thus, the value at the 0.95 quantile of normal distribution was taken as the cutoff for whether a cell type was highly expressed. If the expression level of the lncRNA was higher than the cutoff, it was considered to be highly expressed lncRNA. A collection of highly expressed lncRNAs in all the immune cells was used for this analysis. The study was conducted in accordance with the Declaration of Helsinki (as revised in 2013).
Survival analysis and risk modeling
In the TARGET-OS data set, according to the immune score value obtained by the Estimation of Stromal and Immune cells in Malignant Tumour tissues using Expression data (ESTIMATE), the samples were divided into high and low immune score group by the median score. Then, differential expressed lncRNAs (DE-lncRNAs) were detected with a false discovery rate (FDR) <0.05 and |log2 (fold change)| ≥0.58 (21). Then, DE-lncRNAs intersect with IM-lncRNAs were used for univariate Cox regression analysis. lncRNAs with a P value <0.05 were kept for constructing risk model by the multivariate Cox regression and Stepwise screening analysis. Next, we built a risk-proportional regression model to calculate the risk score. Based on their risk scores, the samples were divided into high and low groups, and survival curves were drawn to compare the survival status of the 2 groups.
Bioinformatics analysis
We conducted a functional analysis of the DEGs in the samples of two risk-score groups, including a GO analysis, KEGG analysis, and GSEA analysis. To evaluate the relationship between the risk-score and tumor immune invasion, we firstly calculate the percentage of various immune cells in the sample of two risk-score groups by CIBERSORT (https://cibersort.stanford.edu/), TIMER (tumor immune estimation resource; https://cistrome.shinyapps.io/timer), XCELL (xcell.ucsf.edu/), and MCP-counter (https://github.com/ebecht/MCPcounter). Besides, 3 immune related gene signatures’ activity were calculated by GSVA. Then, checked this scores’ distribution between high and low risk group. We used 4 gene sets: comprising chemokines, immune checkpoint blockades (ICBs), immune activity-related genes, and immune cells to evaluate the relationship between the risk-score and tumor immune invasion.
Statistical analysis
To evaluate the prediction accuracy of the risk-score model, we plotted the ROC curves and calculated the areas under the curve (AUCs). Age, gender, risk score, and metastasis in the TARGET-OS data sets were analyzed by univariate and multivariate Cox regression analysis. All the statistic analysis were performed by R4.0 software.
Results
Differentially expressed lncRNAs in samples with high and low immune scores were screened
The flowchart of study was presented (Figure S1). To explore the relationship between the differentially expressed lncRNAs in the immune score samples and survival, we first conducted a differential analysis and screened DE-lncRNAs in the TARGET-OS data set. The intersection of these DE-lncRNAs and IM-lncRNAs (lncRNAs expressed by the immune cells) yielded the following 29 lncRNAs: Inositol-Tetrakisphosphate 1-Kinase antisense RNA 1 (ITPK1-AS1), Long Intergenic Non-Protein Coding RNA 599 (LINC00599), Glycerol kinase 3 pseudogene (GK3P), Ankyrin Repeat Domain 36B Pseudogene 2 (ANKRD36BP2), NPTN-IT1, RPARP-AS1, OLMALINC, Polycystin 1, Transient Receptor Potential Channel Interacting Pseudogene 6 (PKD1P6), Ectonucleoside Triphosphate Diphosphohydrolase 1 Antisense RNA 1 (ENTPD1-AS1), FTX, CH17-340M24.3, CARD8-AS1, PC-Esterase Domain Containing 1B Antisense RNA 1 (PCED1B-AS1), Napsin B Aspartic Peptidase Pseudogene (NAPSB), Glycoprotein Alpha-Galactosyltransferase 1 Pseudogene (GGTA1P), Proteasome 20S Subunit Beta 8 Antisense RNA 1 (PSMB8-AS1), Major Histocompatibility Complex, Class II, DR Beta 6 (HLA-DRB6), GTPase, Very Large Interferon Inducible Pseudogene 1 (GVINP1), HOXA Transcript Antisense RNA, Myeloid-Specific 1 (HOTAIRM1), Long Intergenic Non-Protein Coding RNA 996 (LINC00996), Small Nucleolar RNA Host Gene 9 (SNHG9), FLJ20021, Zinc Finger BED-Type Containing 5 Antisense RNA 1 (ZBED5-AS1), KANSL1-AS1, Integrin Subunit Beta 2 Antisense RNA 1 (ITGB2-AS1), T Cell Receptor Gamma Locus Antisense RNA 1 (TRG-AS1), Major Histocompatibility Complex, Class I, J (HLA-J), Trinucleotide Repeat Containing Adaptor 6C Antisense RNA 1 (TNRC6C-AS1), and PRR34 Antisense RNA 1 (PRR34-AS1) (Figure 1).
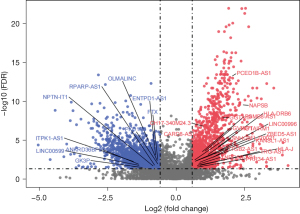
Prognostic value of the OS markers
To examine the prognostic value of these lncRNAs for OS patients, 15 lncRNAs were obtained by a univariate Cox regression analysis of the 29 lncRNAs (P<0.05; Table 1). Next, 15 lncRNAs were selected by a multivariate Cox regression analysis and stepwise screening. Ultimately, 6 lncRNAs were obtained (i.e., CARD8-AS1, FTX, KANSL1-AS1, NPTN-IT1, OLMALINC, and RPARP-AS1) (Table 2). The following formula was used: risk score = CARD8-AS1 * (–0.816784749) + FTX * (–1.167412412) + OLMALINC * (0.622776568) + KANSL1-AS1 * (–0.588179333) + NPTN-IT1 * (0.863758704) + RPARP-AS1 * (0.500369245). Next, the samples were divided into high- and low-risk groups according to their risk scores, and survival curves were drawn (Figure 2A). We found that samples with high-risk scores had poor survival. Next, we conducted a survival analysis in relation to age, gender, risk score, and metastasis in the TARGET-OS data set using univariate and multivariate regression analysis (Tables 3,4). The results showed that both risk score and metastasis were associated with survival regardless of the univariate or multivariate outcomes, and that these may be important factors affecting the prognosis of patients with OS.
Table 1
lncRNAs | Beta | HR | HR (95% CI for HR) | P value | High nums | Low nums |
---|---|---|---|---|---|---|
OLMALINC | 1.9 | 6.6 | 6.6 (3.1–14) | 1.50E-06 | 13 | 72 |
GK3P | 1.4 | 3.9 | 3.9 (1.8–8.4) | 0.00048 | 18 | 67 |
ANKRD36BP2 | 1.3 | 3.7 | 3.7 (1.6–8.4) | 0.0019 | 15 | 70 |
CARD8-AS1 | –1.4 | 0.25 | 0.25 (0.1–0.62) | 0.0028 | 40 | 45 |
PKD1P6 | 1.2 | 3.3 | 3.3 (1.3–8.4) | 0.011 | 9 | 76 |
KANSL1-AS1 | –0.95 | 0.38 | 0.38 (0.18–0.82) | 0.013 | 56 | 29 |
FTX | 0.96 | 2.6 | 2.6 (1.2–5.7) | 0.015 | 22 | 63 |
PCED1B-AS1 | –1.1 | 0.35 | 0.35 (0.15–0.82) | 0.016 | 37 | 48 |
RPARP-AS1 | 1.8 | 5.8 | 5.8 (1.4–25) | 0.018 | 65 | 20 |
NPTN-IT1 | 1.2 | 3.3 | 3.3 (1.2–9) | 0.02 | 9 | 76 |
TNRC6C-AS1 | –1 | 0.36 | 0.36 (0.15–0.91) | 0.031 | 75 | 10 |
GGTA1P | –1.3 | 0.28 | 0.28 (0.084–0.93) | 0.038 | 25 | 60 |
CH17-340M24.3 | –0.9 | 0.41 | 0.41 (0.17–0.96) | 0.041 | 36 | 49 |
LINC00599 | 0.79 | 2.2 | 2.2 (1–4.7) | 0.041 | 25 | 60 |
ZBED5-AS1 | –0.89 | 0.41 | 0.41 (0.17–0.97) | 0.043 | 33 | 52 |
Beta, regression coefficient beta; HR, hazard ratio; CI, confidence interval.
Table 2
Term | HR | Lower | Upper | Coef | Se (coef) | Z value | P value |
---|---|---|---|---|---|---|---|
CARD8-AS1 | 0.441850032 | 0.214477992 | 0.91026333 | –0.816784749 | 0.368763613 | –2.214927719 | 0.026765026 |
FTX | 0.311171083 | 0.110473154 | 0.87647939 | –1.167412412 | 0.528361914 | –2.209493872 | 0.027140308 |
KANSL1-AS1 | 0.55533745 | 0.32037526 | 0.962620156 | –0.588179333 | 0.280659723 | –2.095702673 | 0.036108575 |
NPTN-IT1 | 2.372059828 | 1.16966068 | 4.810512936 | 0.863758704 | 0.360743881 | 2.394382134 | 0.016648393 |
OLMALINC | 1.864096654 | 1.09016925 | 3.187446659 | 0.622776568 | 0.273700748 | 2.275392278 | 0.022882408 |
RPARP-AS1 | 1.649330165 | 1.006990703 | 2.701405273 | 0.500369245 | 0.251740781 | 1.98763682 | 0.046851871 |
HR, hazard ratio; Coef, coefficient, regression coefficient beta; Se (coef), standard error; z value = coef/se (coef).
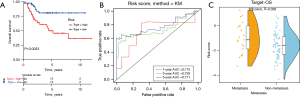
Table 3
Term | Beta | HR | HR (95% CI) | Lower | Upper | Wald.test | P value |
---|---|---|---|---|---|---|---|
Age | –0.056 | 0.95 | 0.95 (0.43–2.1) | 0.43 | 2.1 | 0.02 | 0.89 |
Gender | –0.34 | 0.71 | 0.71 (0.33–1.5) | 0.33 | 1.5 | 0.78 | 0.38 |
Type | 1.3 | 3.5 | 3.5 (1.5–8.3) | 1.5 | 8.3 | 8.2 | 0.0043 |
Meta. or non-metastatic | 1.6 | 4.7 | 4.7 (2.2–10) | 2.2 | 10 | 16 | 6.60E-05 |
Beta, regression coefficient beta; CI, confidence interval.
Table 4
Term | HR | Lower | Upper | Coef | Se (coef) | Z value | P value |
---|---|---|---|---|---|---|---|
Age >16 | 1.005184606 | 0.426658567 | 2.368160794 | 0.005171212 | 0.437223547 | 0.011827387 | 0.990563331 |
Gender (male) | 0.835465251 | 0.364224958 | 1.916404051 | –0.179766523 | 0.423587918 | –0.424390109 | 0.671281333 |
Type (high) | 3.691673791 | 1.544561948 | 8.823508437 | 1.306079957 | 0.444569198 | 2.937855263 | 0.003304913 |
Meta. or non-metastatic | 4.8087353 | 2.199025527 | 10.51553741 | 1.570434118 | 0.39920111 | 3.933942263 | 8.36E-05 |
HR, hazard ratio; Coef, coefficient, regression coefficient beta; Se(coef), standard error; z value = coef/se(coef).
To analyze the predictive value of the risk score in the prognosis of OS, time-dependent ROC curves were drawn based on the risk scores of the 6 genes and the AUCs were calculated (Figure 2B). The results showed that the AUCs of the 1-, 3-, and 5-year ROC curves of the prognostic model were 0.715, 0.729, and 0.771, respectively, indicating that the prediction accuracy of the risk-score model was relatively high. Finally, we analyzed the relationship between the risk score and metastasis. The risk scores were higher in the metastatic samples than those in the non- metastatic samples (Figure 2C).
The risk-score model was verified
First, we divided the samples into high- and low-risk groups according to the risk scores in the IMvigor210 data set (the urothelial carcinoma data set) and drew survival curves. The high-risk patients were found to have poor survival (Figure S2A). We analyzed the ROC curves of the risk scores and found that the AUCs of the ROC curves of the prognostic model at 1, 3, and 5 years were 0.466, 0.52, and 0.537, respectively (Figure S2B). We also verified these results in the TCGA-SKCM data set (the cutaneous melanoma data set). The data set comprised 42 samples with immunotherapy data and 415 samples without immunotherapy data. Based on whether the immunotherapy data were available or not, the samples were divided into high- and low-risk groups according to the risk scores, and survival curves were drawn. The high-risk patients with immunotherapy were found to have poor survival (Figure S2C). Then, we analyzed the ROC curves of risk score. We found that the AUC of the 1-, 3-, and 5-year ROC curves for the sample prognostic models with immunotherapy data were 0.86, 0.916, and 0.711, respectively (Figure S2D). The high-risk patients without immunotherapy were found to have poor survival (Figure S2E). The AUCs of the 1-, 3-, and 5-year ROC curves for the sample prognostic models without immunotherapy data were 0.522, 0.562, and 0.599, respectively (Figure S2F). These results are consistent with the above results and suggest that the prediction value of the OS risk-score model is relatively high.
Next, we verified the model by conducting a functional analysis of the DEGs between two risk group samples. Through a GO analysis of the biological processes, we found that the functions of these DEGs were mainly related to the immune response, defense response, positive regulation of immune system process, and cytokine response. Additionally, most of these DEGs were downregulated in the high-risk group (Figure 3A). Through a GO analysis of the cellular components, we found that the functions of these DEGs were mainly related to the intrinsic components of the plasma membrane, extracellular exosome, and cell surface. Additionally, most of these DEGs were downregulated in the high-risk group (Figure 3B). We conducted a GO analysis of the molecular function, and found that the functions of these DEGs were mainly related to signaling receptor binding, identical protein binding, and signaling receptor activity. Additionally, most of these DEGs were downregulated in the high-risk group (Figure 3C).
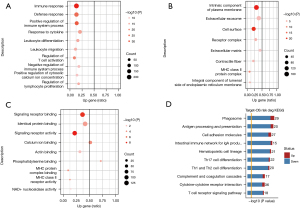
The KEGG analysis revealed that these DEGs were mainly involved in the phagosomes, cell adhesion molecules, and the cytokine-cytokine signaling pathway. Most of the DEGs in these pathways were downregulated (Figure 3D). For the GSEA analysis, we used immune-related gene sets and found that the B cell receptor (BCR) signaling pathway, T cell receptor (TCR) signaling pathway, natural killer (NK) cell cytotoxicity, antigen processing and presentation, chemokine receptors, and other immune-related signaling pathways were downregulated in the high-risk group. No upregulated immune-related signaling pathway was found (Figure 4A). We used gene sets from the KEGG database for the analysis and found antigen processing and presentation, NK cell-mediated cytotoxicity, Th17 cell differentiation, the T cell receptor signaling pathway, the Toll-like receptor signaling pathway, the chemokine signaling pathway, and other immune-related signaling pathways were downregulated in the high-risk group. The ribosome pathway was upregulated in the high-risk group (Figure 4B). In conclusion, the function of the DEGs in the low-risk group was mainly related to immune inflammation.
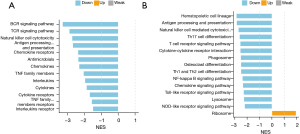
Immuno-infiltration analysis
First, we used a chemokine (Figure 5A), ICB (Figures 5B-5E), immune-activity-related gene (Figure 6A), and immune cell (Figure 6B) data set to evaluate whether the model was related to tumor immune invasion. The results showed that no matter the data set, almost all of the genes were more highly expressed in the low-risk group than the high-risk group. Next, we analyzed the relationship between the immune infiltration results and survival in the TARGET-OS data set (Table 5). The immune score was significantly associated with survival in the target-OS data sets, and cytotoxic_T cells, monocytic lineage, and cluster of differentiation (CD) 8 T cells were also associated with survival.
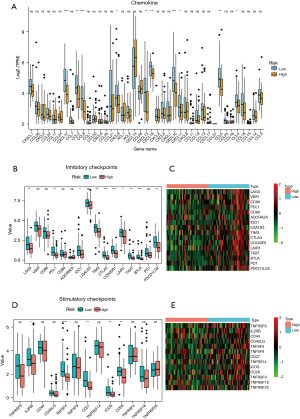
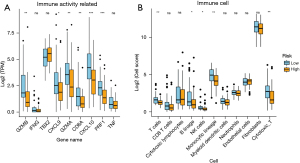
Table 5
Cell type | Survivorship curve P value (TARGET-OS Data set ) |
---|---|
Immune Score | 0.0011 |
Cytotoxic T cells | 0.045 |
Myeloid dendritic cells | 0.83 |
Monocytic lineage | 0.019 |
Cytotoxic lymphocytes | 0.71 |
Fibroblasts | 0.96 |
Endothelial cells | 0.26 |
Neutrophils | 0.2 |
NK cells | 0.14 |
B lineage | 0.05 |
CD8 T cells | 0.045 |
T cells | 0.31 |
Next, we examined the TARGET-OS data through CIBERSORT, TIMER, XCELL, MCP analyze the relation between risk score and immunity infiltration results. The CIBERSORT results showed that activated dendritic cells were richer in the low-risk samples (Figure 7A). According to the results of TIMER, macrophages and mDC cells were richer in the low-risk samples (Figure 7B). The XCELL results showed that B plasma was enriched in the high-risk samples. Hemastem cells, immune score, macrophage M1, macrophage, mDC activated, microenvironment scores, monocytes, stroma score, and T CD8+ central memory cells were enriched in the low-risk sample (Figure 8A). From the MCP results, B lineage, cytotoxic_T cells, monocytic lineage, NK cells and T cell expression were richer in the low-risk samples (Figure 8B). Due to the high proportion of immune cells with significant differences in the MCP results, we analyzed the correlation between the results of the immune infiltration calculated by MCP and risk scores. We found that monocytic lineage, fibroblasts, T cells, and other cells were highly negatively correlated with risk score (correlation coefficient value <0, P value <0.05) (Figure 8C and Table 6).
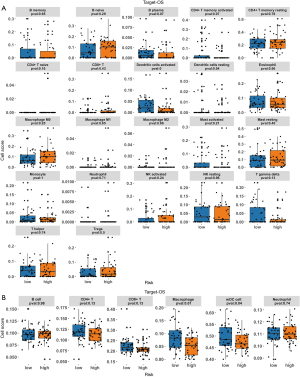
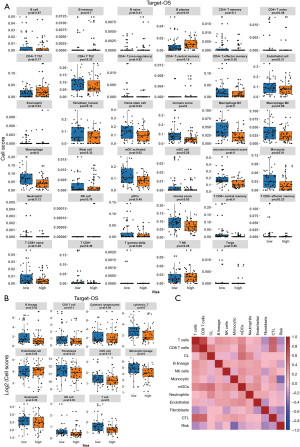
Table 6
Cell type | Cor_value | P value |
---|---|---|
Risk | 1 | 0 |
T cells | –0.263464746 | 0.014839614 |
CD8 T cells | –0.145846871 | 0.182904083 |
Cytotoxic lymphocytes | 0.005366495 | 0.96112298 |
B lineage | –0.154100059 | 0.159094681 |
NK cells | –0.009482383 | 0.931362481 |
Monocytic lineage | –0.438292802 | 2.72E-05 |
Myeloid dendritic cells | –0.154471182 | 0.158080916 |
Neutrophils | –0.074284273 | 0.499255801 |
Endothelial cells | –0.058055816 | 0.597659975 |
Fibroblasts | –0.297774076 | 0.005644012 |
Cytotoxic T cells | –0.208816999 | 0.055124599 |
Cor_value, correlation coefficient value.
Discussion
OS is a malignant bone tumor commonly seen in children and adolescents, and its incidence is increasing year by year (4). At present, its pathogenesis is very complicated and unclear. The circular RNA circTADA2A promotes the progression and metastasis of OS by sponging miR-203A-3p and regulating CREB3 expression (25). Circ_001422 accelerates OS oncogenesis and metastasis by regulating the miR-195-5p/FGF2/PI3K/Akt axis, which suggests that Circ_001422 can be the target for OS therapy (26). A comprehensive bioinformatics analysis of micro RNA (miRNA) and mRNA in OS showed that miR-30D-5p, miR-17-5p, miR-98-5p, miR-301A-3p, and miR-30E-5p are the hub miRNAs (27). A protein-protein interaction network analysis indicated COL1A1 COL1A2, MMP2, CDH11, and COL4A1 may be the core regulatory molecules in the occurrence and development of OS (27). MiRNA-218 inhibits cell proliferation, migration, and invasion by targeting runt-related transcription factor 2 in human OS cells (28). The overexpression of C3AR1 mRNA was confirmed to inhibit the proliferation, migration, and invasion of OS cells and induce apoptosis. It may be a promising therapeutic target for OS, and it may be related to prognosis and the tumor microenvironment (29). M6A regulators (i.e., FTO and IGF2BP2) may play an important role in the metastasis of OS, which is of great value to the prognosis and treatment strategy for OS patients (30). In this study, we focused on the relationship between 6 lncRNAs and the occurrence and development of OS, and our findings provide further insights into the potential molecular regulatory mechanism of the occurrence and development of OS.
The lncRNAs examined in this study not only play a very important role in OS, but have also been reported in the study to have related roles in other tumors (31). In this study, CARD8-AS1 was highly expressed in the samples with high immune scores. It is suggested that immune cells expressing CARD8-AS1 had a high degree of infiltration in high immune score samples. Additionally, the expression of CARD8-AS1 was negatively correlated with the risk score, indicating that the higher the expression of CARD8-AS1, the lower the risk score and the better the prognosis of patients. These results suggest that CARD8-AS1 has a protective effect in patients with OS. In ovarian cancer, card8-AS1 (HR =1.31, P=9.3E−03) was found to be significantly associated with the overall survival rate of ovarian cancer patients through a weighted gene co-expression network analysis (31). In relation to glioma, a study has found that CARD8-AS1 regulates the metastasis potential of glioma cell lines in vitro, and combined with clinical information, CARD8-AS1 has been identified as a risk lncRNA of glioma (32). Thus, the effects of the same gene in different tumors may be opposite, and the specific mechanism needs to be further explored.
In this study, the low expression of FTX in high immune score sample indicated that the immune cell expressing FTX infiltration degree in high immune score samples was low. However, by constructing a risk model construction, we found a negative correlation between FTX expression and risk score, which suggests that FTX plays a risk role in patients with OS. Research has found that FTX promotes gastric cancer progression by targeting miR-215 (33). In pancreatic cancer, FTX silencing inhibits pancreatic cancer cell proliferation and invasion via the upregulation of miR-513B-5p (34). In colorectal cancer, FTX promotes colorectal cancer cell migration and invasion through the miR-590-5P/RBPJ axis (35). Additionally, it has been found that FTX knockdown inhibits the proliferation and migration of OS by regulating miR-320A/TXNRD1 (36). These findings are consistent with our results, but the risk model we constructed still needs to be verified by further experiments.
In this study, the low expression of OLMALINC and RPARP-AS1 in the high immune score samples indicated that the infiltration degree of immune cell expressing OLMALINC and RPARP-AS1in high immune score samples was low. Additionally, the expression levels of OLMALINC and RPARP-AS1 were positively correlated with risk score, suggesting that OLMALINC and RPARP-AS1 play risk roles in patients with OS. Long intervening non-coding RNA (lincRNA) is a subclass of non-coding RNA (37). OLMALINC (LINC00263) and RPARP-AS1 have been identified as important regulators of the pathogenesis of non-alcoholic fatty liver disease (38,39). Studies have shown that OLMALINC (LINC00263), regulated by heterogeneous nuclear ribonucleoprotein K, upregulates CAPN2 through miR-147a, and thus plays an important role in cancer and malignant tumors (40). Additionally, Wnt-regulated OLMALINC (LINC00263) was found to modify cancer cell growth (41). This is consistent with our results, but the specific mechanism of action needs to be further studied through a large number of animal experiments. In colon cancer, a study has shown that the RPARP-AS1/miR-125A-5p axis plays an active role in promoting the proliferation, migration, and invasion of colon cancer cells (42). A bioinformatics analysis has previously shown that the high expression of RPARP-AS1 is associated with low survival in patients with OS (43). However, in lung adenocarcinoma and triple negative breast cancer, the high expression of RPARP-AS1 is associated with higher patient survival (44,45). This also suggests that the role of lncRNA in tumors is extremely complex and needs to be further studied. In summary, the prognostic effect between the lncRNAs discussed in our study in tumor development is obvious, and the model we established may be applicable to clinical practice. However, we have not yet verified it and hope that future studies will conduct molecular biology experiments to verify our conclusions.
We also used gene sets to evaluate whether the model was associated with tumor immune invasion. Chemokine is a large class of small cytokines that can be divided into 4 distinct subgroups (i.e., XC, CC, CXC, and CX3C) based on cysteine residues. These molecules are responsible for immune cell transportation and shaping the immune system, are expressed by cancer cells, and play an important role in cancer progression and treatment outcomes (46). XCL1, also known as lymphotactin, is produced by T cells, NK cells, and Natural killer T (NKT) cells during infection and inflammatory responses (47). CX3CL1 is a chemokine involved in the anti-cancer function of lymphocytes (mainly NK cells, T cells, and dendritic cells), and its increased expression in tumors can improve the prognosis of cancer patients (48). In gliomas, the CXCL family contributes to the immunosuppressive microenvironment in gliomas and enhances the sensitivity of gliomas to chemotherapy (49). The CXCL12/CXCR4 axis has become a new target for cancer therapy, and many CXCL12/CXCR4 antagonists have been developed and validated and shown promising anti-cancer activity in tumor cells (50). In breast cancer, CCL8 and CCL21 are critical in targeting cancer cells, particularly by modifying stroma and immune cells through tumor suppressor mechanisms (51). In hepatocellular carcinoma, targeting tumor-infiltrating macrophages through the CCL2/CCR2 signaling pathway can be a therapeutic strategy (52). In gastric cancer, MiR484 inhibits proliferation, migration, invasion, and induces apoptosis by targeting CCL18 (53).
In ICB gene sets, lymphocyte activation gene-3, CD233 (LAG3) is an activation marker of CD4+ and CD8+ T cells and can be used as a target for cancer immunotherapy (54). Some studies have shown that IDO1 inhibits the CD8+T cell response in colon cancer (55). Mir-448, as a tumor suppressor miRNA, enhances the CD8+T cell response by inhibiting IDO1 expression (55). CD200-CD200r1 signaling can reduce imiquimod-induced psoriatic skin inflammation by inhibiting macrophage activation (56). TNFSF4 is closely associated with therapies that induce anti-tumor immunity and may help induce a promising immune response in breast cancer (57). CTLA-4 has sequence homology with CD28 and is expressed on T cells after activation. They both regulate T cell proliferation and differentiation and play important roles in immune response pathways in vivo (58). In the immune activity-related gene set, CXCL9, also known as interferon-γ induced monocyte factor, is induced by interferon-gamma (IFN-γ) and mainly mediates lymphocyte infiltration into the lesion site and inhibits tumor growth (59). CXCL10 is a driver chemokine that affects CD4+ and CD8+ T cells, promoting anti-tumor immunity, and regulating autoimmunity (60).
Granzymes (GZMs) have been recognized as key cell death executors of cytotoxic T and NK cells during cancer immunosurveillance. In immune surveillance, the cytotoxic molecules (i.e., perforin and Granzyme B) exert anti-tumor and anti-infective effect (61). In this study, we found that no matter what the gene concentration, almost all the genes were more highly expressed in the low-risk group than the high-risk group. Through literature reports, we also found that most genes have anti-inflammatory and anti-cancer effects, which is consistent with our conclusion.
Conclusions
In this study, we comprehensively analyzed the relationship between the expression of lncRNA in OS and the occurrence, development, and prognosis of OS. The abnormal expression of 6 lncRNAs (i.e., CARD8-AS1, FTX, KANSL1-AS1, NPTN-IT1, OLMALINC, and RPARP-AS1) was significantly associated with the progression of OS, and these lncRNAs could be used as independent markers of OS to assess the prognosis of patients with OS. In conclusion, our study identified a novel marker for assessing the prognosis of OS patients and provides important evidence for further studies on the role of OS-related genes in OS.
Acknowledgments
Funding: This work was supported by the Nantong Municipal Science and technology plan project (Grant No. YYZ17012) and the Nantong Fifth Phase “226 project” Scientific Research Project in 2018.
Footnote
Reporting Checklist: The authors have completed the TRIPOD reporting checklist. Available at https://tp.amegroups.com/article/view/10.21037/tp-22-253/rc
Conflicts of Interest: All authors have completed the ICMJE uniform disclosure form (available at https://tp.amegroups.com/article/view/10.21037/tp-22-253/coif). The authors have no conflicts of interest to declare.
Ethical Statement: The authors are accountable for all aspects of the work in ensuring that questions related to the accuracy or integrity of any part of the work are appropriately investigated and resolved. The study was conducted in accordance with the Declaration of Helsinki (as revised in 2013).
Open Access Statement: This is an Open Access article distributed in accordance with the Creative Commons Attribution-NonCommercial-NoDerivs 4.0 International License (CC BY-NC-ND 4.0), which permits the non-commercial replication and distribution of the article with the strict proviso that no changes or edits are made and the original work is properly cited (including links to both the formal publication through the relevant DOI and the license). See: https://creativecommons.org/licenses/by-nc-nd/4.0/.
References
- Li Y, Zou J, Li B, et al. Anticancer effects of melatonin via regulating lncRNA JPX-Wnt/β-catenin signalling pathway in human osteosarcoma cells. J Cell Mol Med 2021;25:9543-56. [Crossref] [PubMed]
- Park HJ, Bae JS, Kim KM, et al. The PARP inhibitor olaparib potentiates the effect of the DNA damaging agent doxorubicin in osteosarcoma. J Exp Clin Cancer Res 2018;37:107. [Crossref] [PubMed]
- Zhang Z, Ha SH, Moon YJ, et al. Inhibition of SIRT6 potentiates the anti-tumor effect of doxorubicin through suppression of the DNA damage repair pathway in osteosarcoma. J Exp Clin Cancer Res 2020;39:247. [Crossref] [PubMed]
- Isakoff MS, Bielack SS, Meltzer P, et al. Osteosarcoma: Current Treatment and a Collaborative Pathway to Success. J Clin Oncol 2015;33:3029-35. [Crossref] [PubMed]
- Kim KM, Hussein UK, Park SH, et al. FAM83H is involved in stabilization of β-catenin and progression of osteosarcomas. J Exp Clin Cancer Res 2019;38:267. [Crossref] [PubMed]
- Brown HK, Tellez-Gabriel M, Heymann D. Cancer stem cells in osteosarcoma. Cancer Lett 2017;386:189-95. [Crossref] [PubMed]
- Llobat L, Gourbault O. Role of MicroRNAs in Human Osteosarcoma: Future Perspectives. Biomedicines 2021;9:463. [Crossref] [PubMed]
- Bottani M, Banfi G, Lombardi G. Circulating miRNAs as Diagnostic and Prognostic Biomarkers in Common Solid Tumors: Focus on Lung, Breast, Prostate Cancers, and Osteosarcoma. J Clin Med 2019;8:1661. [Crossref] [PubMed]
- Zhang W, Ren X, Qi L, et al. The value of lncRNAs as prognostic biomarkers on clinical outcomes in osteosarcoma: a meta-analysis. BMC Cancer 2021;21:202. [Crossref] [PubMed]
- Botti G, Giordano A, Feroce F, et al. Noncoding RNAs as circulating biomarkers in osteosarcoma patients. J Cell Physiol 2019;234:19249-55. [Crossref] [PubMed]
- Ju C, Zhou R, Sun J, et al. LncRNA SNHG5 promotes the progression of osteosarcoma by sponging the miR-212-3p/SGK3 axis. Cancer Cell Int 2018;18:141. [Crossref] [PubMed]
- Yin R, Liu J, Zhao D, et al. Long Non-Coding RNA ASB16-AS1 Functions as a miR-760 Sponge to Facilitate the Malignant Phenotype of Osteosarcoma by Increasing HDGF Expression. Onco Targets Ther 2020;13:2261-74. [Crossref] [PubMed]
- Cagle P, Qi Q, Niture S, et al. KCNQ1OT1: An Oncogenic Long Noncoding RNA. Biomolecules 2021;11:1602. [Crossref] [PubMed]
- Zhang B, Yu L, Han N, et al. LINC01116 targets miR-520a-3p and affects IL6R to promote the proliferation and migration of osteosarcoma cells through the Jak-stat signaling pathway. Biomed Pharmacother 2018;107:270-82. [Crossref] [PubMed]
- Wang S, Chen Z, Gu J, et al. The Role of lncRNA PCAT6 in Cancers. Front Oncol 2021;11:701495. [Crossref] [PubMed]
- Ghafouri-Fard S, Khoshbakht T, Taheri M, et al. A Review on the Carcinogenic Roles of DSCAM-AS1. Front Cell Dev Biol 2021;9:758513. [Crossref] [PubMed]
- Cai Q, Zhao X, Wang Y, et al. LINC01614 promotes osteosarcoma progression via miR-520a-3p/SNX3 axis. Cell Signal 2021;83:109985. [Crossref] [PubMed]
- Huang YF, Lu L, Shen HL, et al. LncRNA SNHG4 promotes osteosarcoma proliferation and migration by sponging miR-377-3p. Mol Genet Genomic Med 2020;8:e1349. [Crossref] [PubMed]
- Ding L, Liu T, Qu Y, et al. lncRNA MELTF-AS1 facilitates osteosarcoma metastasis by modulating MMP14 expression. Mol Ther Nucleic Acids 2021;26:787-97. [Crossref] [PubMed]
- Lu X, Qiao L, Liu Y. Long noncoding RNA LEF1-AS1 binds with HNRNPL to boost the proliferation, migration, and invasion in osteosarcoma by enhancing the mRNA stability of LEF1. J Cell Biochem 2020;121:4064-73. [Crossref] [PubMed]
- Deng B, Pan R, Ou X, et al. LncRNA RBM5-AS1 Promotes Osteosarcoma Cell Proliferation, Migration, and Invasion. Biomed Res Int 2021;2021:5271291. [Crossref] [PubMed]
- Chang L, Jia DL, Cao CS, et al. LncRNA PCAT-1 promotes the progression of osteosarcoma via miR-508-3p/ZEB1 axis. Eur Rev Med Pharmacol Sci 2021;25:2517-27. [PubMed]
- Fu D, Lu C, Qu X, et al. LncRNA TTN-AS1 regulates osteosarcoma cell apoptosis and drug resistance via the miR-134-5p/MBTD1 axis. Aging (Albany NY) 2019;11:8374-85. [Crossref] [PubMed]
- Ghafouri-Fard S, Shirvani-Farsani Z, Hussen BM, et al. The critical roles of lncRNAs in the development of osteosarcoma. Biomed Pharmacother 2021;135:111217. [Crossref] [PubMed]
- Wu Y, Xie Z, Chen J, et al. Circular RNA circTADA2A promotes osteosarcoma progression and metastasis by sponging miR-203a-3p and regulating CREB3 expression. Mol Cancer 2019;18:73. [Crossref] [PubMed]
- Yang B, Li L, Tong G, et al. Circular RNA circ_001422 promotes the progression and metastasis of osteosarcoma via the miR-195-5p/FGF2/PI3K/Akt axis. J Exp Clin Cancer Res 2021;40:235. [Crossref] [PubMed]
- Xu K, Zhang P, Zhang J, et al. Identification of potential micro-messenger RNAs (miRNA-mRNA) interaction network of osteosarcoma. Bioengineered 2021;12:3275-93. [Crossref] [PubMed]
- Guo Q, Ma J, Wu J. MiRNA-218 inhibits cell proliferation, migration and invasion by targeting Runt-related transcription factor 2 (Runx2) in human osteosarcoma cells. Regen Ther 2021;18:508-15. [Crossref] [PubMed]
- Zou T, Liu W, Wang Z, et al. C3AR1 mRNA as a Potential Therapeutic Target Associates With Clinical Outcomes and Tumor Microenvironment in Osteosarcoma. Front Med (Lausanne) 2021;8:642615. [Crossref] [PubMed]
- Liu H, Qin G, Ji Y, et al. Potential role of m6A RNA methylation regulators in osteosarcoma and its clinical prognostic value. J Orthop Surg Res 2021;16:294. [Crossref] [PubMed]
- Li N, Zhan X. Identification of clinical trait-related lncRNA and mRNA biomarkers with weighted gene co-expression network analysis as useful tool for personalized medicine in ovarian cancer. EPMA J 2019;10:273-90. [Crossref] [PubMed]
- Lin X, Jiang T, Bai J, et al. Characterization of Transcriptome Transition Associates Long Noncoding RNAs with Glioma Progression. Mol Ther Nucleic Acids 2018;13:620-32. [Crossref] [PubMed]
- Zhang F, Wang XS, Tang B, et al. Long non-coding RNA FTX promotes gastric cancer progression by targeting miR-215. Eur Rev Med Pharmacol Sci 2020;24:3037-48. [PubMed]
- Li S, Zhang Q, Liu W, et al. Silencing of FTX suppresses pancreatic cancer cell proliferation and invasion by upregulating miR-513b-5p. BMC Cancer 2021;21:290. [Crossref] [PubMed]
- Chen GQ, Liao ZM, Liu J, et al. LncRNA FTX Promotes Colorectal Cancer Cells Migration and Invasion by miRNA-590-5p/RBPJ Axis. Biochem Genet 2021;59:560-73. [Crossref] [PubMed]
- Huang S, Zhu X, Ke Y, et al. LncRNA FTX inhibition restrains osteosarcoma proliferation and migration via modulating miR-320a/TXNRD1. Cancer Biol Ther 2020;21:379-87. [Crossref] [PubMed]
- Mills JD, Kavanagh T, Kim WS, et al. High expression of long intervening non-coding RNA OLMALINC in the human cortical white matter is associated with regulation of oligodendrocyte maturation. Mol Brain 2015;8:2. [Crossref] [PubMed]
- Benhammou JN, Ko A, Alvarez M, et al. Novel Lipid Long Intervening Noncoding RNA, Oligodendrocyte Maturation-Associated Long Intergenic Noncoding RNA, Regulates the Liver Steatosis Gene Stearoyl-Coenzyme A Desaturase As an Enhancer RNA. Hepatol Commun 2019;3:1356-72. [Crossref] [PubMed]
- Matboli M, Gadallah SH, Rashed WM, et al. mRNA-miRNA-lncRNA Regulatory Network in Nonalcoholic Fatty Liver Disease. Int J Mol Sci 2021;22:6770. [Crossref] [PubMed]
- Lee WJ, Shin CH, Ji H, et al. hnRNPK-regulated LINC00263 promotes malignant phenotypes through miR-147a/CAPN2. Cell Death Dis 2021;12:290. [Crossref] [PubMed]
- Liu S, Harmston N, Glaser TL, et al. Wnt-regulated lncRNA discovery enhanced by in vivo identification and CRISPRi functional validation. Genome Med 2020;12:89. [Crossref] [PubMed]
- Ren Y, Zhao C, He Y, et al. RPARP-AS1/miR125a-5p Axis Promotes Cell Proliferation, Migration and Invasion in Colon Cancer. Onco Targets Ther 2021;14:5035-43. [Crossref] [PubMed]
- Bu X, Liu J, Ding R, et al. Prognostic Value of a Pyroptosis-Related Long Noncoding RNA Signature Associated with Osteosarcoma Microenvironment. J Oncol 2021;2021:2182761. [Crossref] [PubMed]
- Zheng J, Zhao Z, Wan J, et al. N-6 methylation-related lncRNA is potential signature in lung adenocarcinoma and influences tumor microenvironment. J Clin Lab Anal 2021;35:e23951. [Crossref] [PubMed]
- Li XX, Wang LJ, Hou J, et al. Identification of Long Noncoding RNAs as Predictors of Survival in Triple-Negative Breast Cancer Based on Network Analysis. Biomed Res Int 2020;2020:8970340. [Crossref] [PubMed]
- Thomas JK, Mir H, Kapur N, et al. CC chemokines are differentially expressed in Breast Cancer and are associated with disparity in overall survival. Sci Rep 2019;9:4014. [Crossref] [PubMed]
- Lei Y, Takahama Y. XCL1 and XCR1 in the immune system. Microbes Infect 2012;14:262-7. [Crossref] [PubMed]
- Korbecki J, Simińska D, Kojder K, et al. Fractalkine/CX3CL1 in Neoplastic Processes. Int J Mol Sci 2020;21:3723. [Crossref] [PubMed]
- Wang Z, Liu Y, Mo Y, et al. The CXCL Family Contributes to Immunosuppressive Microenvironment in Gliomas and Assists in Gliomas Chemotherapy. Front Immunol 2021;12:731751. [Crossref] [PubMed]
- Zhou W, Guo S, Liu M, et al. Targeting CXCL12/CXCR4 Axis in Tumor Immunotherapy. Curr Med Chem 2019;26:3026-41. [Crossref] [PubMed]
- Unver N. Revisiting CCL-type chemokines in breast cancer and its milieu: prominent targetable chemokines, CCL8 and CCL21. Biosci Rep 2021;41:BSR20210033. [Crossref] [PubMed]
- Li X, Yao W, Yuan Y, et al. Targeting of tumour-infiltrating macrophages via CCL2/CCR2 signalling as a therapeutic strategy against hepatocellular carcinoma. Gut 2017;66:157-67. [Crossref] [PubMed]
- Liu J, Li SM. MiR-484 suppressed proliferation, migration, invasion and induced apoptosis of gastric cancer via targeting CCL-18. Int J Exp Pathol 2020;101:203-14. [Crossref] [PubMed]
- Andrews LP, Marciscano AE, Drake CG, et al. LAG3 (CD223) as a cancer immunotherapy target. Immunol Rev 2017;276:80-96. [Crossref] [PubMed]
- Lou Q, Liu R, Yang X, et al. miR-448 targets IDO1 and regulates CD8+ T cell response in human colon cancer. J Immunother Cancer 2019;7:210. [Crossref] [PubMed]
- Li D, Wang Y, Tang L, et al. CD200-CD200R1 signalling attenuates imiquimod-induced psoriatic inflammation by inhibiting the activation of skin inflammatory macrophages. Int Immunopharmacol 2020;78:106046. [Crossref] [PubMed]
- Li K, Ma L, Sun Y, et al. The immunotherapy candidate TNFSF4 may help the induction of a promising immunological response in breast carcinomas. Sci Rep 2021;11:18587. [Crossref] [PubMed]
- Xia S, Chen Q, Niu B. CD28: A New Drug Target for Immune Disease. Curr Drug Targets 2020;21:589-98. [Crossref] [PubMed]
- Tokunaga R, Zhang W, Naseem M, et al. CXCL9, CXCL10, CXCL11/CXCR3 axis for immune activation - A target for novel cancer therapy. Cancer Treat Rev 2018;63:40-7. [Crossref] [PubMed]
- Karin N. Chemokines and cancer: new immune checkpoints for cancer therapy. Curr Opin Immunol 2018;51:140-5. [Crossref] [PubMed]
- Arias M, Martínez-Lostao L, Santiago L, et al. The Untold Story of Granzymes in Oncoimmunology: Novel Opportunities with Old Acquaintances. Trends Cancer 2017;3:407-22. [Crossref] [PubMed]